Inferential Statistics PDF
Document Details
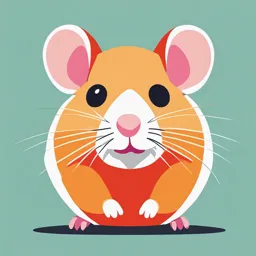
Uploaded by CourteousPrairieDog849
Maynooth University
Pedro Valero Mora
Tags
Summary
This document is a lecture on Inferential Statistics, covering topics such as astronomical errors, political polls, and convenience sampling. The content describes how to deal with measurement errors and how to generalize conclusions from samples to populations.
Full Transcript
Inferential Statistics Pedro Valero Mora 2025-01-22 1 Table of contents i 1 Introduction 2 Inferential Statistics 3 Statistical error in four situations 3.1 Astronomical errors 3.2 From Samples to the Population: Political Polls...
Inferential Statistics Pedro Valero Mora 2025-01-22 1 Table of contents i 1 Introduction 2 Inferential Statistics 3 Statistical error in four situations 3.1 Astronomical errors 3.2 From Samples to the Population: Political Polls 3.3 Just one sample, and sometimes quite small 3.4 Convenience Samples 4 Summary of the Topic 2 1 Introduction Three levels of knowledge through statistics: Descriptive statistics: Allows you to draw conclusions about the data you actually have. Inferential statistics: Tries to generalize your conclusions to other people or situations for which you donβt have data. Causality: Seeks to demonstrate that a treatment or action causes an effect. This course focuses on inferential statistics. 3 2 Inferential Statistics Generalizing from a sample to a population involves error: statistical error. Error calculation: Based on mathematical assumptions. These assumptions are diο¬icult to justify in practice. Statistics courses often assume that these assumptions are true 4 3 Statistical error in four situations Astronomical errors From sample to population: Political surveys Only one sample, and sometimes quite small Convenience sampling 5 3.1 Astronomical errors The instruments used to measure the position of the stars gave different results in each measurement At sea, an incorrect result could be fatal! 6 3.1.1 The measurement problem: π¦π = π + ππ , where: π¦π : The measurement we actually take. π: The true position of the star. ππ : The error that causes the measurement to be inaccurate. What we measure in each case is the true value we want to measure plus an associated error. Causes of error: Factors such as hand tremors, air turbulence, waves at sea, among others. 7 What we want is π, but what we have is π¦π. Now, we can assume that: ππ Μ π=1 = 0: The average of the errors is zero, if the error varies randomly from one side to another. This is called random error. π Therefore, π¦π=1 Μ = π + 0 for a number π of repetitions of the measurement. That is, if we measure a value suο¬iciently many times and calculate the average, we can obtain the correct value or at least a good approximation to it. 8 3.1.2 What value should π have? If π is small, we might be unlucky and have all the errors in the same direction. To be completely sure, π = β, but life is not that long. Depending on the context, π can be as small as 2 or 3, or it can be several hundreds or thousands. A suο¬icient value of π depends on the size of the error. 9 3.1.3 Example of error size Device 1 Device 2 We measured blood pressure with two devices, 6 times each. 135 165 Both devices give an average of 105 150 120 (an acceptable blood 120 120 pressure). 110 110 Device 2 has more variability. 130 90 120 85 π₯1Μ = 120 π₯2Μ = 120 With device 1, only two measurements always give a reasonable approximation. If we donβt take many measurements with device 2, we might end up with one or two very high or low values. 10 3.1.4 Calculation of the average (standard) error size Device 1 Device 2 If we subtract the mean (120) 15 45 from the values in the previous -15 30 table, we get this new table. 0 0 If we calculate the mean using the -10 -10 usual formula, the result would be 10 -30 zero. 0 -35 π₯1Μ = 0 π₯2Μ = 0 11 The solution is to square, sum, and take the square root so that the number doesnβt become too large: ππ·ππ£πππ1 = β(152 + (β15)2 + 02 + (β10)2 + 102 + 02 )/6 = 10.41 ππ·ππ£πππ2 = β(452 + 302 + 02 + (β10)2 + (β30)2 + (β35)2 )/6 = 29.3 In fact, this is the same as if we had applied the standard deviation formula to the original measurements. The standard deviation in this context can be called average measurement error because we consider the mean as the true value. The average error is called standard error. ΔΉ The normal distribution of errors Gauss proposed that measurement errors follow the normal probability distribution and described its properties and mathematical function. Using this distribution, we can calculate the probability and, therefore, the amount of errors we can expect if we know the standard deviation population (π). A demonstration of the normal distribution 12 3.1.5 Summary We have a value that we want to measure, but we canβt do it with accuracy If we assume that the errors are random and have a mean of zero, we can approximate that value by calculating the mean of several measurements. The larger the errors, the more measurements we will need. It is common to assume or take for granted that the errors follow a normal distribution. The standard deviation/typical error gives us an idea of the size of the error. 13 3.2 From Samples to the Population: Political Polls Measurement problems laid the foundation for statistical inference, but these ideas need to be adapted to be applicable in other contexts. Political polls have been, and continue to be, an important field for the practical application of Statistics and Social Sciences. This case is particularly interesting because many polls are conducted before elections. This allows us to test statistical theory with real data. Additionally, we have a criterion to compare estimates against: the election results. The 1936 error highlighted the importance of proper statistical sampling to avoid incorrect predictions. 14 3.2.1 Contents of this Section Estimating the population value from multiple polls. What is good statistical sampling? Sample size (π). Error is not random in this case. Calculating Confidence Intervals with Multiple polls 15 3.2.2 Estimating the Population Value from Multiple Polls Suppose we have π polls, and each one produces a value ππ=π when we ask people whether they favor a candidate: In this case: ππ=π = π + ππ=π , where: ππ=π is the percentage obtained in a poll π. π is the true percentage in the population (the population parameter). ππ=π is the error introduced by that poll. In other words, each poll produces the true population value plus an error. 16 If we average the percentages from all the polls, we get an estimate of π, the population value (assuming all polls have the same sample size, π, so no weighting is needed). This estimate will be more precise if there is low variability in the errors, which is equivalent to saying that there is low variability between polls. Similarly, by calculating the standard deviation of the percentages obtained from the polls (or the errors, which is equivalent), we get the sampling standard error (ππΈπ ). If we were working with means, this error would be ππ₯Μ. 17 3.2.3 What Is Good Statistical Sampling? All the above assumes that the data were obtained through proper sampling. Much of statistical theory is based on data sampled using simple random sampling with replacement. ΔΉ Definition of Simple Random Sampling with Replacement Simple random sampling with replacement is a method of selecting ele- ments from a population where: 1. Random selection: Every element in the population has an equal probability of being selected in each draw. 2. Replacement: Once an element is selected, it is returned to the population, so it can be selected again in subsequent draws. 18 For large populations, simple random sampling can be expensive and diο¬icult to implement. Alternatives such as cluster sampling can reduce costs and are also acceptable. When the population is large, sampling without replacement produces results very similar to sampling with replacement. The main goal is to obtain a representative sample of the population: This is a highly relevant topic in applied research. However, in Psychology, we often lack expertise in this area compared to Sociology or Political Science. 19 3.2.4 Sample Size (π) In the earlier example on measurement error, we observed that small samples could be used. In the case of political polls, it is clear that larger samples are required. While it is diο¬icult to provide concrete rules for π in many contexts, in the field of polling, there are some useful starting points: 20 In the simplest case, we want to calculate the percentage (π) of people who support or oppose a candidate: The standard deviation is largest when ππ π’πππππ‘ = 50% and ππππππ π = 50% (converted to proportions, 0.5). An acceptable margin of error (π πΈ ) is typically Β±2.5%. Assuming the sampling error follows a normal distribution and we want 95% confidence, we use π = 1.96. 21 Applying the formula: π 2 β π β (1 β π) π= = 1537 π πΈ2 Rounding up, π = 1600. This sample size applies to very large populations if sampling is done without replacement: For example, the entire United States, Europe, or Spain. It is also valid for small populations if sampling is done with replacement. Note that population size does not affect this calculation: the recommended sample size π remains the same. However, if we want to provide results for subpopulations (e.g., provinces or European countries), we need to draw a sample of this size for each subpopulation if we want the same error margin 22 3.2.5 Error Is Not Random in This Case When comparing political poll results with election outcomes, we often find that: All polls either overestimate or underestimate a candidate. This results from bias, as the sampling fails to be representative. The error distribution does not always follow a normal distribution and may show skewness or kurtosis. This complicates the calculation of the standard error using conventional methods. 23 To correct bias, experts use strategies informally referred to as βcooking the resultsβ: In Spain, the Center for Sociological Research (CIS) used to βcookβ poll results, but it was often accused of favoring the ruling party. They no longer do this, and their results are now less accurate. To correct variability, results from multiple polls can be used 24 3.2.6 Calculating Confidence Intervals with Multiple polls Political polls often present their results as an interval within which the election results are expected to fall. Specifically, 95% confidence intervals are commonly used, which reflect the range in which the true population parameter is expected to lie with 95% confidence. These intervals are calculated based on the conducted polls. Providing an interval makes it easier to be correct, at the expense of being less precise. 25 Method for Calculating Confidence Intervals with multiple surveys When multiple surveys are available on the same topic, there are two common ways to calculate confidence intervals. The first steps are the same for both methods: 1. Calculate the percentage of people who would vote for a candidate in each survey. 2. Average the percentages from all the surveys to obtain πΜ, where: πΜ is the estimate of the parameter π, the true percentage in the population. 26 3. Calculate the confidence interval (in this case at 95%). There are two ways to do this: Method 1: Using Percentiles 1. Calculate the 2.5% and 97.5% percentiles of the percentages obtained from the surveys. 2. The interval between these percentiles contains 95% of the survey results. Method 2: Using the Standard Error (SE) 1. Calculate the standard error (SE) from the standard deviation of the percentages obtained in the surveys. 2. Assuming the errors follow a normal distribution, calculate the margin of error (ME) using ππΈ = Β±π§ β ππΈ , where π§ is the value that leaves 95% of the area under the normal curve (Β±1.96), and compute: πΆπΌ95% = πΜ Β± 1.96 β ππΈ 27 Both methods have their advantages: The first method is more robust if the normality assumption does not hold The second method relies on the normality assumption and is commonly used in practice. 28 An example In the table, most of the Candidate(%) percentages are between 45% and 55 55%, but there is a lot of 48 50 variability. 53 There are two that seem unusual: 50 80% and 25%, but we will use 80 them even though a statistician 25 45 might remove them. 55 60 To calculate the voting intention, we can take the average of the percentages (πΜ = 52.1%), or if we donβt really trust the two unusual values, we could remove them (πΜ = 52%; the result doesnβt change much) 29 The confidence interval calculated using the percentile formula would be: [29.5%, 79.5%]: 95% of the surveys give a result between these percentages The standard error or mean sampling error would be 13.61. We can use this value to calculate the confidence interval. 30 To calculate the confidence interval from the mean sampling error, we rely on the assumption that errors follow a normal distribution. 95% of the values in a normal distribution lie between -1.96 and 1.96 standard deviations (in this case, standard errors). By multiplying the standard error (13.61) by 1.96, we get 26.7. This is called the Margin of Error (ME). We add and subtract 26.7 from the point estimate (52 Β± 26.7). The confidence interval calculated this way would be [25.3%, 78.7%]. The result in this case is quite similar to the one obtained with the previous method, but we have had to assume something. In contrast, the previous method used data from actual surveys, which makes it more credible. 31 3.2.7 Summary In political surveys (and surveys in general), large samples are often used, but a reasonable maximum size is around 1600 cases. The sample size is important, but it is more crucial that the sample is representative. If a sample is not representative, it is said to have bias. In political surveys, we can compare the results with the election results and use them to correct the findings. 32 If we have several surveys measuring the same thing and they are not all biased in the same direction, we can average them to calculate an estimate of the true value or parameter. If we calculate the standard deviation of the results obtained from all the surveys, we can estimate the standard sampling error. If we calculate the 5th and 95th percentiles of the results obtained from all the surveys, we can obtain an interval within which we have 95% confidence that the parameter value will fall. If we assume that errors follow a normal distribution, we can also calculate the confidence interval, but we must keep in mind that it is based on a speculation. 33 3.2.8 A real example: Election polls in the United States This example is taken from Nate Silverβs website (in Spain, Kiko Llaneras does something similar). Can you interpret this graph? Keep in mind that it is published in a newspaper, so it is intended for the general public. 34 3.3 Just one sample, and sometimes quite small Political surveys are special for the following reasons: We had several studies that more or less asked the same thing. There was a clear population to generalize the results to. The samples usually consist of several hundred or thousand cases. There is a criterion (the elections) that serves as a basis to evaluate our result. They are descriptive studies; they donβt try to prove anything, but instead aim to find facts. 35 In scientific studies in fields like Psychology and Health Sciences, on the other hand: There is only one study on a topic, and we want to generalize from it. The population sometimes doesnβt exist in a real sense, but in a theoretical or future sense. Example: A new treatment is applied to a sample of subjects, but a population of treated individuals doesnβt yet exist. The samples are often between 10 to 100 subjects, although sometimes they are large as well. There is no criterion or parameter that serves as a real value to evaluate the estimate. These are typically experimental studies that aim to prove an effect or consequence. 36 ΔΉ Types of Scientific Studies An experiment is a research design in which independent variables are manipulated to observe their effect on dependent variables, under controlled conditions. A randomized controlled trial (RCT) is a specific type of experiment where participants are randomly assigned to groups (e.g., treatment group and control group) to compare the effects of an intervention. A randomized clinical trial (RCT) is a type of experimental study used in healthcare to assess the effectiveness and safety of treatments, medications, or medical interventions. It is considered the gold standard in medical and psychological research due to its ability to reduce biases. RCTs in medicine often have very large samples, and a significant economic investment is made in them. In Psychology, they tend to be more modest: Here is an example. 37 3.3.1 Characteristics of Statistical Analyses in Scientific studies They use simple random sampling or make a strong effort to achieve something quite similar. The most important parameter is usually (although not always) the difference between the mean in the control group and in the treatment group. The parameter cannot be calculated by averaging several studies because there is only one, but refer to the definition of meta-analysis. ΔΉ Note Definition of Meta-analysis A meta-analysis is a type of research study that combines and analyzes the results of multiple previous studies addressing the same question or topic, with the goal of obtaining more precise and reliable conclusions. It is primarily used in fields such as medicine, psychology, education, and social sciences to synthesize existing evidence and evaluate the overall effect of an intervention, treatment, or variable. Meta-analyses are funda- mental in systematic reviews. 38 3.3.2 Studentβs t distribution The problems described before can be solved thanks to student The development of the Studentβs t-distribution is considered one of the most important milestones in statistics and has had a profound impact on science in general. It was developed by William Sealy Gosset, who wrote under the pseudonym βStudent,β while working as a chemist for the Guinness brewery in the early 20th century. Gosset created this distribution as a solution to statistical analysis problems with small samples, a very common issue in the industrial and experimental field of his time. The way he arrived at this solution was by studying the sampling distribution of the mean, which we will look at next. 39 3.3.3 Example We want to know the average score of Spanish students aged 16 on a mathematics test. We use a sample of π = 25 students. The mean score of those 25 students on the test is π¦ Μ = 490, the standard deviation is π π¦ = 10. From this, we know that π, the value for all of Spain, will be close to 490, but it may not be exactly 490: 25 students are too few, so the error could be quite large. We would like to obtain an approximate interval within which it is reasonable (likely) that π lies. 40 The difference between π and π¦ Μ is the sampling error π, or the difference between the value in the sample and the value in the population: how can we know π if we canβt know π? Answer: Itβs impossible to know an individual value of π without knowing π. In political surveys, we had the elections as π, but here we donβt. Can we at least know π,Μ the average error we would get if we did many surveys? In political surveys, we had many values of π, but now we only have one. How did Student solve this problem? 41 Studentβs Simulation Student solved the problem using simulation: 1. Created a population by generating data with a specific mean (π) and a specific standard deviation (π). 2. Took a sample (π) of a given size (for example, π = 25 as in the previous example) using simple random sampling with replacement. 3. Calculated the mean (π₯πΜ ) in the sample and the standard deviation (π π₯π ), and stored it. 4. Calculated the difference between the population mean and the sample mean (ππ = π β π₯πΜ ). Stored that value. 5. Repeated steps 2 to 4 until he got tired (say π times). He repeated the previous steps with different sample sizes (π = 1, 2, 3, 4, 5,..., 100,...). Then, with the means for all samples of a given sample size, he calculated the mean (to see if he got π), its standard deviation (to calculate the average or standard sampling error), and studied its probability distribution (to see if it followed a normal distribution). 42 3.3.4 How Were the Calculations Done Before Student? We saw that the first advancements in statistics were related to astronomy: Gauss and Laplace showed that a formula applicable for estimating the average or standard sampling error (ππΈ ) is π ππΈ = β π This formula has a problem: part of the population standard deviation (π), which if we have only one sample like in our example with students, we donβt know and itβs impossible to know. To solve this, what was done in practice was to estimate π with π π₯ , the standard deviation of the sample. It was thought to be correct if the sample size was large (π > 100 was considered adequate, but there was no evidence). 43 We also saw that, to calculate a confidence interval for the mean, we could assume that the errors (ππ ) followed a normal distribution. However, Gosset worked with smaller samples, and that didnβt seem to be true in his experience. 44 What Did Student Discover by Exploring the Results of His Simulation? Two main things: a) The standard deviations in the samples were usually smaller than the population standard deviation. π This led to a correction that consisted of: π πβ1 = πβ1.π π₯ , and then π ππΈ = πβ1 β π , which is Gaussβs formula but replacing π with π πβ1. b) The errors did not follow the normal distribution, although the difference was only noticeable when the samples were quite small. This led him to discover a new probability distribution called the Studentβs t distribution, which could be applied when the samples were small instead of the normal distribution. These two discoveries showed that confidence intervals based on traditional calculations with small samples were too small. 45 3.3.5 An Example of the Application of Studentsβs Discoveries In the mathematics test I described earlier, we want to know if Spain is above the threshold considered acceptable for that test. The value is considered acceptable if itβs above 500 with a 95% confidence level. If Spain is not below, the government will change the curriculum so students get more hours of mathematics (this example is not fictional). 46 Letβs suppose we get the same result as in the previous example (π¦ Μ = 490 and the standard deviation is π π¦ = 10) with samples of different sizes. π π πβ1 ππΈπΊππ’π π ππΈππ‘π’ππππ‘ π§95% π‘πβ195% 95%πΌπΆπΊππ’π π 95%πΌπΆππ‘π 5 10. 54 4.47 5.60 Β±1.96 Β±2.77 [468 β [451 β 512] 528] 10 10. 10 9 3.16 3.51 Β±1.96 Β±2.26 [474 β [470 β 505] 510] 25 10. 25 24 2.00 2.08 Β±1.96 Β±2.06 [480 β [479 β 500] 501] 50 10. 50 49 1.41 1.44 Β±1.96 Β±2.01 [483 β [483 β 487] 487] 100 10. 100 99 1.00 1.01 Β±1.96 Β±1.98 [485 β [485 β 495] 495] The results show that Gaussβs method with samples below 25 produces smaller standard errors, the value of the theoretical distribution (π§ versus π‘ in this case) is lower, and the confidence intervals are narrower. With π = 25, the differences are minimal. Starting from 50, although there are still differences, they are not 47 3.3.6 Summary When we only have one sample and want to estimate a confidence interval within which the population mean will fall, we have to rely on statistical theory. The statistical theory was developed by Gauss, but it was based on large samples, and it was unknown what happened with smaller samples. Student demonstrated that with small samples, the formula for the standard error of the mean needed to be corrected, and that the sampling distribution of the mean followed a probability distribution different from the normal distribution, which was called the t distribution. Studentβs result paved the way for: The ability to conduct studies with smaller samples. Studying the sampling distributions of other estimators: variance, correlation, difference between means, etc. The above, along with the work of other statisticians, particularly Fisher, paved the way for the development of significance tests, which will be the focus of this course. 48 3.4 Convenience Samples A convenience sample is a type of non-probability sample that is selected based on: ease of access, proximity or availability of participants. In this case, researchers select elements or subjects that are easily available, rather than using a random or representative method. The vast majority of studies published in the Social Sciences and Psychology use convenience samples rather than simple random sampling. These studies should be taken with considerable skepticism unless successive studies confirm the findings. 49 3.4.1 Problems with Convenience Samples in Psychology and Social Sciences Sample errors that are too small due to the lack of simple random sampling. Participants may be special for many reasons: Voluntary response bias: volunteers tend to be more extreme. Accessibility bias: those closer to the researchers are likely to think similarly. Temporal bias: certain issues may be more prominent at certain times, but may not be relevant at other times. Etc. To me, one way to summarize the problem in one line is: in a convenience sample, it is unknown to which population the results are being generalized. 50 3.4.2 Table One Table 1 is called so because it is typically the first table that appears in the results section of scientific studies. Table 1 is a chart that summarizes the demographic, clinical, or other characteristics of the study participants. Its main purpose is to describe the sample so that readers understand who the participants were and evaluate the generalizability of the results. You need to review Table 1 to see if the study is biased and if it is reasonable to apply it to your specific case. 51 This table appears in this article and it is a Table 1 even though it is called Table(2016) Mindfulness 2. 7:1396β1407 Table 2 Comparison of demographic characteristics and Socio-demographics Experimental (n = 212) Control (n = 182) Statistical baseline psychometric measures N % N % between control and experimental Gender (% female) 163 76.9 147 80.8 Ο2 =.880 groups Education (University degree) 164 77.4 140 76.9 Ο2 =.011 Income (below household income 105 49.5 95 52.2 Ο2 =.279 over $35,000 a year) M SD M SD Age 41.31 11.51 40.32 11.08 t =.864 Meditation experience in years 2.80 3.04 2.58 2.77 t =.751 Well-being Stress (PSS) 28.54 6.82 28.38 6.18 t =.242 Depression (BDI-II) 13.46 9.48 12.55 8.58 t =.982 Well-being (PHI-PIS) 6.44 1.74 6.36 1.76 t =.435 Mindfulness (FMI) 33.08 7.71 34.23 8.26 t = β1.428 Gratitude (GQ6) 35.15 5.85 35.04 5.59 t =.181 Self-compassion (SCS) 3.02 0.73 3.05 0.80 t = β.358 Self-efficacy (GSE) 30.65 4.50 30.58 4.39 t =.844 Autonomy (APWB) 57.98 12.08 56.91 12.56 t =.319 Meaning (MLQP) 23.70 6.92 23.77 7.22 t = β.096 Positive relations (COS) 70.48 9.14 71.52 8.75 t = β1.123 Engagement (APM) 39.59 7.01 38.99 7.97 t =.765 (Meaning in life, with no interaction found), two for the COS duration of the participantsβ previous meditation e (Compassion for others, with interaction) and three for the did statistically significantly co-variate with the52slop 2 at are Another thing to look at is the section that describes how the participants Method 007). were contacted. per- Participants ecia- The study used a randomised wait-list controlled design. leas- Our main between-group independent variable was the most allocation to a control or an experimental group. A con- posi- venience sample, composed by three different population leas- groups, was targeted in the recruitment process: educa- fully tors, office workers and meditators. BEducators^ included able people who were educating others in a group setting, e.g. n and school teachers. BOffice workers^ were people working pro- for at least 7 h a week in an office setting. BMeditators^ iman were people who had meditated at least once a week for at n the least 1 year. Meditation, in this context, was defined as ls of any activity where a conscious attempt is made to focus cally attention in a non-analytical way; examples included g in- breathing and walking meditation, body-scan, and yoga. wing The inclusion of this subsample intended to allow ndful analysing whether previous practice of meditation had 53 s the Participants were recruited online through social networks ances and forums. The program was advertised as voluntary and was described as a combination of mindfulness and positive psy- sitive chology exercises. No incentives were offered. Four hundred ll-be- fifty-five participants were initially recruited, of whom 15 well- were excluded for severe levels of depression (as measured ssion, with the Beckβs depression inventory (BDI) cut off established ll-be- by Beck et al. 1996) following initial completion of question- gage- naires. This screening was deemed necessary based on studies pres- indicating that meditation can have adverse effects on severely sitive depressed individuals (Shapiro 1992b). Another criterion ex- PMP cluded participants under the age of 18, but none appeared on , two the initial recruit list. We Of the remaining 440 participants, 394 completed at least PMP one questionnaire. The number of participants who completed both all the questionnaires finally reached 168; 115 were in the ables. control group and 53 in the experimental group. The partici- lower pants included citizens of 20 countries, with most of them n will from the UK (32 %), Canada (24 %), the USA (13.5 %) and Australia (11 %). All participants were English-speaking. 54 4 Summary of the Topic In the previous course, you studied that the standard deviation is the way to measure the extent of variability of a variable. If the data consists of measurements of the same thing with an instrument and there is variability, we can use the same formula: The value is called standard or typical measurement error. 55 If what we want is to estimate the value of a population and we have several samples drawn through simple random sampling from that population (as in political polls), we can apply the same formula and call it standard sampling error. As in political polls, it is important not to give a result that is too different from the actual election outcome, so the results are presented as an interval within which the true value is likely to fall. This is called confidence intervals, and we can calculate them directly using percentiles from the survey results or under the assumption that errors follow a normal distribution. If we only have one study, we can estimate the same standard sampling error using a formula proposed by Student. If we want to calculate a confidence interval for the population value, we can do so assuming that the error distribution follows the t distribution of Student. 56 In many Psychology studies, convenience samples are used that are not drawn using simple random sampling, yet still present estimates of sampling error and confidence intervals as if they had. These studies are questionable and should always be taken with skepticism. Examining the descriptive statistics of the sample (Table 1) is a convenient step to assess the relevance of a study. 57