Medical Imaging & Engineering in Medicine PDF
Document Details
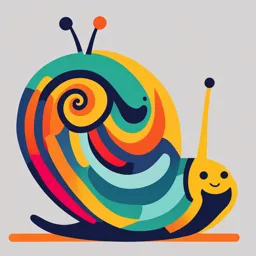
Uploaded by EthicalIsland2905
null
Tags
Summary
This document discusses various aspects of medical imaging, including X-rays and CT scans, and explores trending topics in 'engineering in medicine', such as AI and machine learning in medical diagnostics, wearable devices, and nanotechnology. It also delves into machine learning algorithms used in medical imaging, focusing on support vector machines (SVM). Furthermore, it highlights the importance of ethical considerations in medical research and data privacy. The document includes examples and specific applications in areas like tumor detection with MRI.
Full Transcript
Reading – 15 mins X-ray, CT An X-ray, also called a radiograph, sends radiation through the body. Areas with high levels of calcium (bones and teeth) block the radiation, causing them to appear white on the image. Soft tissues allow the radiation to pass through. They appear gray or black on the im...
Reading – 15 mins X-ray, CT An X-ray, also called a radiograph, sends radiation through the body. Areas with high levels of calcium (bones and teeth) block the radiation, causing them to appear white on the image. Soft tissues allow the radiation to pass through. They appear gray or black on the image. Fractures Dislocations Misalignments Narrowed joint spaces X-ray An X-ray won’t show subtle bone injuries, soft tissue injuries or inflammation. Portrait of Wilhelm Conrad Röntgen. TRENDING TOPICS IN ‘ENGINEERING IN MEDICINE’ according to chatGPT 1. Artificial Intelligence (AI) and Machine/Deep Learning in Medicine - AI in diagnostics (e.g., image analysis for cancer detection) - Predictive analytics for patient outcomes 2. Wearable and Implantable Medical Devices - Smart wearables for health monitoring (e.g., heart rate, glucose levels) - Brain-computer interfaces (BCIs) for rehabilitation 3. Biomedical Imaging and Advanced Imaging Techniques - Functional and molecular imaging - Real-time imaging advancements - Portable imaging devices for remote healthcare 4. Nanotechnology in Medicine - Nanomedicine for targeted drug delivery - Nanosensors for disease detection - Nanomaterials for regenerative medicine 5. Biomechanics and Bio-robotics - Robotic surgery advancements - Prosthetics with sensory feedback - Exoskeletons for rehabilitation Artificial Intelligence (AI) and Machine/Deep Learning in Medicine - AI in diagnostics (e.g., image analysis for cancer detection) - Predictive analytics for patient outcomes Chatbots (e.g., healthcare virtual assistants). Autonomous vehicles. Supervised Learning (e.g., classification and regression). Unsupervised Learning (e.g., clustering, anomaly detection). Ability to process unstructured data (e.g., images, text, audio). End-to-end learning (automatically extracts features from raw data). Machine Learning in Medical Imaging Pattern Recognition: Identifying anomalies or disease-specific features in imaging data. Predictive Modeling: Using imaging data to predict patient outcomes or disease progression. Automation: Assisting in repetitive tasks such as image segmentation, registration, or classification. Image Segmentation Image Classification Disease Detection and Diagnosis Popular ML Algorithms in Medical Imaging Support Vector Machines (SVMs): For binary classification tasks, like separating diseased from non- diseased tissues. Random Forests: For robust feature selection and multi- class classification. K-Nearest Neighbors (KNN): For identifying similar patterns in images. Linear Regression/Logistic Regression: For predictive modeling based on image-derived features Popular ML Algorithms in Medical Imaging Support Vector Machines (SVMs): For binary classification tasks, like separating diseased from non- diseased tissues. Random Forests: For robust feature selection and multi- class classification. K-Nearest Neighbors (KNN): For identifying similar patterns in images. Linear Regression/Logistic Regression: For predictive modeling based on image-derived features Face detection, image classification, text categorization Linear SVM: Linear SVM is used for linearly separable data, which means if a dataset can be classified into two classes by using a single straight line, then such data is termed as linearly separable data, and classifier is used called as Linear SVM classifier. Non-Linear SVM: If data is linearly arranged, then we can separate it by using a straight line, but for non-linear data, we cannot draw a single straight line. Consider the below image: Importance of Training Set/Labeling Learning Patterns in Tumor Characteristics - The training set provides the data for the model to learn tumor-specific patterns such as shape, size, texture, and contrast in medical images (e.g., MRI, CT, X-rays). - It helps the model distinguish between healthy tissue and abnormalities like tumors or lesions. Generalization to Real-World Data Robustness to Variability To evaluate the use of multi-parametric (mp) MRI quantitative features with a multiregion-of-interest approach in machine learning-based glioma grading. - Imaging Protocol: T1, T2- -Regions of Interest (ROIs): weighted, diffusion- Tumor, immediate - Participants: 43 newly weighted, diffusion tensor, periphery, and distant diagnosed glioma patients. MR perfusion, and MR peritumoral edema/normal spectroscopic imaging. tissue. - A support vector machine - Analysis: Normalized mp- (SVM) with recursive MRI features were used to feature elimination was differentiate low-grade applied for feature (WHO I–II) and high-grade selection. (WHO III–IV) gliomas. The SVM model (linear kernel) achieved: - Accuracy: 93.0% - Specificity: 86.7% - Sensitivity: 96.4% In-Class Reading (Writing) Assignment: SVM Applications in MRI Objective: Analyze and summarize applications of SVM classification in MRI for medical diagnosis. 1. 15-Minute Independent Reading 2. Literature search on ML/SVM & MRI (e.g., Brain tumor detection, lesion detection in multiple sclerosis (MS). 3. Writing Task - In max. 2 pages, summarize the role of ML/SVM in MRI-based medical diagnosis. Include: - applications (add one more application). - Advantages of using SVM in medical imaging. - Any challenges or limitations noted in your reading. BM402: ENGINEERING IN MEDICINE 19th Dec 2024 M 2170 – South Campus Where were we? Machine Learning Deep learning Bio/Nanomaterials Biomechanics Lab Tour EEG Tour MIMLAB EEG LAB Experiment with EEG and pulse oximeter Drops in the pulse amplitute during breatholding During eyes open and closed: change in EEG alpha power We also had system noise due to electric wires, hardwares in the space. The systems needs upgrade. But managed to filter out (50 Hz) with processing. All of the electronic devices work with a fixed frequency and voltage of What is the source of 50Hz noise in EEG recording? alternative current (AC) with the frequency of 50 Hz is the power line An oscillating voltage in a conductor generates a magnetic field that will frequency. induce a small oscillating voltage in nearby conductors, and this is how We can use a faraday cage to eliminate the noise easily. We can build the electrical noise in the environment shows up in the EEG. cage. Any box with conducting wire making grid and connecting the box into a grpund. And we can put out device inside the box. EEG Tour All of the electronic devices work with a fixed frequency and voltage of What is the source of 50Hz noise in EEG recording? alternative current (AC) with the frequency of 50 Hz is the power line An oscillating voltage in a conductor generates a magnetic field that will frequency. induce a small oscillating voltage in nearby conductors, and this is how We can use a faraday cage to eliminate the noise easily. We can build the electrical noise in the environment shows up in the EEG. cage. Any box with conducting wire making grid and connecting the box into a grpund. And we can put out device inside the box. Preclinical Tour 7T Preclinical MRI CT Portable PET Wonderful and in-depth intro and presentation by Dr. Uluç Preclinical Tour Bio/Nano-materials Tour Bio/Nano-materials Tour Biomechanis Tour Biomechanis Tour Ethics, Data Sharing, and Rights in Biomedical Engineering / Medicine Tuskegee Syphilis Study Ethics, Data Sharing, and Rights in Biomedical Engineering / Medicine Tuskegee Syphilis Study Ethics, Data Sharing, and Rights in Biomedical Engineering / Medicine Tuskegee Syphilis Study The study (1932–1972) was a U.S. Public Health Service experiment that aimed to observe the natural progression of untreated syphilis in African American men. They were misled into believing they were receiving free medical care, but they were not informed about their diagnosis or provided treatment, even after penicillin became the standard therapy in the 1940s. The study continued for 40 years, causing unnecessary suffering, long-term health complications, and deaths. It is widely regarded as a gross violation of medical ethics, emphasizing the importance of informed consent, patient rights, and equitable treatment in research. Ethics, Data Sharing, and Rights in Biomedical Engineering / Medicine Search and reading on Willowbrook Experiments – 15 minutes Ethics, Data Sharing, and Rights in Biomedical Engineering / Medicine Willowbrook Experiments Hepatitis, form the eye of a microscope Ethics, Data Sharing, and Rights in Biomedical Engineering / Medicine Willowbrook Experiments Hepatitis, form the eye of a microscope Ethics, Data Sharing, and Rights in Biomedical Engineering / Medicine Willowbrook Experiments Hepatitis, form the eye of a microscope It is morally wrong to perform an experiment on either a normal or a mentally retarded child when no benefit can result for that child. There was no additional risk for the subjects. Under the normal conditions at the institution the subjects would have been exposed to the same strains of hepatitis. Ethics, Data Sharing, and Rights in Biomedical Engineering / Medicine Willowbrook Experiments Findings: The studies helped differentiate between hepatitis A and B. Researchers discovered insights into the modes of hepatitis transmission and potential treatments. The experiments are widely criticized for violating ethical principles: Lack of informed consent: Many parents were not adequately informed about the risks. Exploitation of vulnerable populations: The children were unable to consent, and their status as institutionalized minors made them highly vulnerable. Questionable necessity. Data Privacy and Patient Confidentiality Risks with Wearable Devices and Apps Collecting Sensitive Health Data: Vast amounts of sensitive personal health data, including heart rate, activity levels, sleep patterns, and even menstrual cycle tracking. Data Privacy and Patient Confidentiality Risks with Wearable Devices and Apps Collecting Sensitive Health Data: Vast amounts of sensitive personal health data, including heart rate, activity levels, sleep patterns, and even menstrual cycle tracking. This data is often shared with third-party companies for analytics, marketing, or research without the user's explicit understanding or consent. Data Privacy and Patient Confidentiality Genomic Data and Biobanking Risks Genomic data collected for research or direct-to- consumer genetic testing services (e.g., 23andMe, AncestryDNA) poses significant privacy concerns. The long-term storage of genomic data in biobanks also raises concerns about consent, ownership, and unauthorized future uses, particularly as technologies advance. Data Privacy and Patient Confidentiality Genomic Data and Biobanking Risks Popular direct-to-consumer genetic testing company that provides insights into ancestry and health-related genetic traits by analyzing a person's DNA from a saliva sample Recently raised significant privacy concerns related to the collection, storage, and use of genomic data. Data Privacy and Patient Confidentiality Genomic Data and Biobanking Risks Sample Collection Saliva Collection Kit: Companies like 23andMe provide a specialized kit to collect saliva. Preservation Solution: The kit includes a solution that stabilizes the DNA during transportation. Cells in Saliva: The DNA comes primarily from epithelial cells shed from the lining of the mouth. Data Privacy and Patient Confidentiality Genomic Data and Biobanking Risks DNA Extraction DNA extraction is the process of isolating DNA from the cells of an organism isolated from a sample, typically a biological sample such as blood, saliva, or tissue. It involves breaking open the cells, removing proteins and other contaminants, and purifying the DNA so that it is free of other cellular components. Data Privacy and Patient Confidentiality Genomic Data and Biobanking Risks Genotyping or Whole Genome Sequencing Genotyping: Identifies specific genetic markers associated with traits, health risks, and ancestry. Whole Genome Sequencing: Maps the entire DNA sequence to provide a comprehensive view of the genome. Involves high-throughput sequencing technologies like Illumina or Oxford Nanopore. Data Analysis: Ancestry analysis, heart rate etc. Data Privacy and Patient Confidentiality Genomic Data and Biobanking Risks Data Sharing with Third Parties: In 2018, 23andMe partnered with GlaxoSmithKline, giving the pharmaceutical giant access to its genetic database for drug development. Even though the data is anonymized, advancements in technology could potentially re-identify individuals. Data Privacy and Patient Confidentiality Genomic Data and Biobanking Risks Data Sharing with Third Parties: In 2018, 23andMe partnered with GlaxoSmithKline, giving the pharmaceutical giant access to its genetic database for drug development. Even though the data is anonymized, advancements in Data Breaches: technology could potentially re-identify individuals. As with any large database, 23andMe's genetic database is at risk of cyberattacks. A breach could expose sensitive information about individuals and their families, given that DNA data is uniquely identifying and permanent. Data Privacy and Patient Confidentiality Genomic Data and Biobanking Risks On September 17, 2024, all seven independent directors of the company resigned, voicing concerns about the strategic direction of the company and intention to take the company private. Users were concerned about the security of their genetic Data Breaches: data, and were trying to delete it from the company's As with any large database, 23andMe's genetic archives. database is at risk of cyberattacks. A breach could expose sensitive information about individuals and their families, given that DNA data is uniquely identifying and permanent. Researcher view: Data sharing Benefits of Data Sharing: Enhances reproducibility and transparency in science. Promotes global collaboration and accelerates innovation. Challenges in Data Sharing: Balancing transparency with the risk of misuse (e.g., re-identification of patients). Institutional Requirements: Overview of mandates by NIH, EU Horizon, and other agencies requiring data sharing for funded research. Researcher view: Data sharing Researcher view: Data sharing Benefits of Data Sharing: Enhances reproducibility and transparency in science. Promotes global collaboration and accelerates innovation. Challenges in Data Sharing: Balancing transparency with the risk of misuse (e.g., re-identification of patients). Institutional Requirements: Overview of mandates by NIH, EU Horizon, and other agencies requiring data sharing for funded research. Researcher view: Data sharing Benefits of Data Sharing: Enhances reproducibility and transparency in science. Promotes global collaboration and accelerates innovation. Challenges in Data Sharing: Balancing transparency with the risk of misuse (e.g., re-identification of patients). Institutional Requirements: Overview of mandates by NIH, EU Horizon, and other agencies requiring data sharing for funded research. Data Sharing in Research Labs Data sharing is an essential part of advancing science, fostering collaboration, and verifying results. However, sharing data, particularly sensitive data like genetic or health information, raises several issues that laboratories must address to ensure ethical and responsible practices. Key issues: Informed Consent De-identification Data Misuse Data Sharing in Research Labs Example: De-identification of Brain MRI: - involves removing or modifying personal information in MRI scans to protect patient privacy. 1. Removal of Personal Identifiers: Removing direct identifiers (e.g., name, date of birth) and indirect identifiers (e.g., age, gender). 2. Metadata Scrubbing: Deleting sensitive metadata embedded in the MRI file (e.g., patient ID, hospital name, scan date). 3. Facial Feature Removal: Masking facial features to prevent re-identification in scans involving the skull/nose. 4. Pseudonymization: Replacing personal identifiers with pseudonyms or codes, while ensuring the mapping is kept separate. Open Data Example: De-identification of Brain MRI: Facial Feature Removal: Masking facial features to prevent re-identification in scans involving deatures like ears. Open Data Example: De-identification of Brain MRI: Facial Feature Removal: Masking facial features to prevent re-identification in scans involving deatures like ears. Process of (A) developing the facial feature detector, which is a deep learning model that can detect the eyes, nose, and ears in 3-dimensional (3D) magnetic resonance (MR) images, and (B) distorting the facial features in nonanonymized cranial MR images. Open Data ADNI database Structural MRI Functional MRI PET Scans EEG Open Data ADNI database Open Access Longitudinal Data The database contains a large collection of clinical, neuroimaging, genetic, and biomarker data that is used to understand the progression of Alzheimer's disease, identify early biomarkers, and evaluate potential treatments. Open Data Example: De-identification of Brain MRI: Facial Feature Removal: Masking facial features to prevent re-identification in scans involving deatures like ears. Process of (A) developing the facial feature detector, which is a deep learning model that can detect the eyes, nose, and ears in 3-dimensional (3D) magnetic resonance (MR) images, and (B) distorting the facial features in nonanonymized cranial MR images. Open Data Example: De-identification of Brain MRI: Facial Feature Removal: Masking facial features to prevent re-identification in scans involving deatures like ears. Process of (A) developing the facial feature detector, which is a deep learning model that can detect the eyes, nose, and ears in 3-dimensional (3D) magnetic resonance (MR) images, and (B) distorting the facial features in nonanonymized cranial MR images. 15 minutes Search ADNI Reading Limitations Among the facial features, wrinkles or the mouth can be identifiers but were not considered in this study. To train the deep learning model, we needed to manually draw labels that mark facial features. We are planning to construct a training dataset that takes into account additional facial features for further study. Once labeled training data comprising any desired facial feature have been constructed, our facial feature detector can evolve through deep learning. Data Sharing in Research Labs what we do? Data Sharing in Research Labs what we do? Anonymze Organize Annotate Describe e.g. Structural, functional, EEG-fMRI & phyio & eyecam data Data Sharing in Research Labs –especially if you’re a young PI ☺ Intellectual Property Concerns - Sharing data, especially pre-publication, risks intellectual property theft. Competing researchers may use the shared data without giving proper attribution or acknowledgment. - This creates tension between the need for open science and protecting the original researchers' efforts. Completing a power analysis to ascertain the smallest group size required to obtain statistically significant data Performing multiple experiments simultaneously so the same control group can be used for all experiments Using newer instrumentation that improves precision and reduces animals needed per data point Sharing tissues with other investigators at the completion of an experiment Refinement refers to employing methods that reduce pain or distress in experimental animals and include: Improving surgical techniques to reduce loss and recovery time Modification of research procedures to be less invasive, painful, or stressful Utilizing up-to-date anesthetics and analgesics that reduce complications, stress, and recovery time Provide environmental enrichment Replacement refers to replacing the experimental animals with non- animal techniques and include: Use of cell culture or organoids Use of bench assays to replace bioassays involving animals Use of in silica computer modeling Drug Delivery Systems Nanoparticles: Testing nanoparticle-based drug carriers to deliver medications directly to target tissues (e.g., tumors). Controlled Release: Developing implants or hydrogels for sustained drug delivery. Gene Therapy: Using viral vectors to deliver therapeutic genes to specific organs or systems. Boğaziçi Universtiy – Example EEG & Physio Lab Ongoing review for BAP starting grant Approved ethics from the university BM 402 TENTATIVE BM597 OUTLINE Human brain development Human nervous system Brain and physiology Biology of behavior Sensory areas EEG-fMRI Emotions; stress, fear, pain Attention and consciousness Brain rhythms and sleep Memory and learning Decision making Addiction Cognitive impairment South Campus BM402: ENGINEERING IN MEDICINE 5th Dec 2024 M 2170 – South Campus Case Study: Engineering Solutions for Traumatic Brain Injury (TBI) Rehabilitation – Focus on Brainstem Damage Recovery Rehabilitation Strategies and Engineering Treatment Timeline: Solutions: Acute Phase (0-6 weeks): Immediate medical In class assignment based on 3 rehabs (1 given) intervention to stabilize the brain injury. The patient patient needs: was placed on a ventilator to assist with breathing, - Brainstorm on challenge, solution, outcome Those who were not available during the Case Study: and a feeding tube was used for nutrition. - Work in groups (2-3 p.) You can submit it as a report on your own (2-3 pages). Rehabilitation Phase (6 weeks - 6 months): - 30 minutes Introduction of physical, occupational, and speech therapy to address motor, respiratory, and swallowing 1. Speech and Swallowing Rehabilitation difficulties. Use of engineering solutions to promote 2. XX Rehabilitation neuroplasticity and recovery of lost functions. 3. YY Rehabilitation - Group presentations TRENDING TOPICS IN ‘ENGINEERING IN MEDICINE’ according to chatGPT TRENDING TOPICS IN ‘ENGINEERING IN MEDICINE’ according to chatGPT 1. Artificial Intelligence (AI) and Machine/Deep Learning in Medicine - AI in diagnostics (e.g., image analysis for cancer detection) - Predictive analytics for patient outcomes 2. Wearable and Implantable Medical Devices - Smart wearables for health monitoring (e.g., heart rate, glucose levels) - Brain-computer interfaces (BCIs) for rehabilitation 3. Biomedical Imaging and Advanced Imaging Techniques - Functional and molecular imaging - Real-time imaging advancements - Portable imaging devices for remote healthcare 4. Nanotechnology in Medicine - Nanomedicine for targeted drug delivery - Nanosensors for disease detection - Nanomaterials for regenerative medicine 5. Biomechanics and Bio-robotics - Robotic surgery advancements - Prosthetics with sensory feedback - Exoskeletons for rehabilitation Artificial Intelligence (AI) and Machine/Deep Learning in Medicine - AI in diagnostics (e.g., image analysis for cancer detection) - Predictive analytics for patient outcomes Chatbots (e.g., healthcare virtual assistants). Autonomous vehicles. Supervised Learning (e.g., classification and regression). Unsupervised Learning (e.g., clustering, anomaly detection). Ability to process unstructured data (e.g., images, text, audio). End-to-end learning (automatically extracts features from raw data). Machine Learning in Medical Imaging Pattern Recognition: Identifying anomalies or disease-specific features in imaging data. Predictive Modeling: Using imaging data to predict patient outcomes or disease progression. Automation: Assisting in repetitive tasks such as image segmentation, registration, or classification. Image Segmentation Image Classification Disease Detection and Diagnosis Popular ML Algorithms in Medical Imaging Support Vector Machines (SVMs): For binary classification tasks, like separating diseased from non- diseased tissues. Random Forests: For robust feature selection and multi- class classification. K-Nearest Neighbors (KNN): For identifying similar patterns in images. Linear Regression/Logistic Regression: For predictive modeling based on image-derived features Face detection, image classification, text categorization Face detection, image classification, text categorization Linear SVM: Linear SVM is used for linearly separable data, which means if a dataset can be classified into two classes by using a single straight line, then such data is termed as linearly separable data, and classifier is used called as Linear SVM classifier. Non-Linear SVM: If data is linearly arranged, then we can separate it by using a straight line, but for non-linear data, we cannot draw a single straight line. Consider the below image: Importance of Training Set/Labeling Learning Patterns in Tumor Characteristics - The training set provides the data for the model to learn tumor-specific patterns such as shape, size, texture, and contrast in medical images (e.g., MRI, CT, X-rays). - It helps the model distinguish between healthy tissue and abnormalities like tumors or lesions. Generalization to Real-World Data Robustness to Variability To evaluate the use of multi-parametric MRI quantitative features with a multiregion-of-interest approach in machine learning-based glioma grading. - Imaging Protocol: T1, T2- -Regions of Interest (ROIs): weighted, diffusion- Tumor, immediate - Participants: 43 newly weighted, diffusion tensor, periphery, and distant diagnosed glioma patients. MR perfusion, and MR peritumoral edema/normal spectroscopic imaging. tissue. - A support vector machine - Analysis: Normalized mp- (SVM) with recursive MRI features were used to feature elimination was differentiate low-grade applied for feature (WHO I–II) and high-grade selection. (WHO III–IV) gliomas. The SVM model (linear kernel) achieved: - Accuracy: 93.0% - Specificity: 86.7% - Sensitivity: 96.4% Reminder for assignment: SVM Applications in MRI Objective: Analyze and summarize applications of SVM classification in MRI for medical diagnosis. 1. Reading Materials 2. Literature search on ML/SVM & MRI (e.g., Brain tumor detection, lesion detection in multiple sclerosis (MS). 3. Writing Task - In max. 2 pages, summarize the role of ML/SVM in MRI-based medical diagnosis. Include: - applications (add one more application). - Advantages of using SVM in medical imaging. - Any challenges or limitations noted in your reading. Notable applications include: Image recognition (e.g., facial recognition, object detection). Natural language processing (e.g., chatbots, language translation). Healthcare (e.g., disease diagnosis through medical imaging). Why Deep Learning? Limitations of Traditional Machine Learning: Traditional ML methods like Support Vector Machines (SVMs) or decision trees rely heavily on manual feature extraction. Example: In image processing, features such as edges, textures, or shapes must be manually designed by experts, making it time- consuming and potentially biased. Advantages of Deep Learning: Automated Feature Learning: Deep Learning models, such as Convolutional Neural Networks (CNNs), can automatically extract and learn hierarchical features from raw data. For example, in image recognition, early layers detect edges and textures, while deeper layers identify objects or faces. Key Components of Deep Learning Neural Networks Basics: The foundational unit of deep learning is the artificial neuron, modeled after biological neurons. Neural networks are organized into layers: Input Layer: Takes raw data (e.g., pixel values for images). Hidden Layers: Perform feature extraction using activation functions and weights. Output Layer: Provides predictions or classifications (e.g., cat vs. dog in an image). Importance of Large Datasets: Deep learning models are data-hungry, requiring large and diverse datasets for effective training. Example: ImageNet, with millions of labeled images, was pivotal in the success of deep learning models like AlexNet. Key Components of Deep Learning What is an Artificial Neuron? An artificial neuron is inspired by biological neurons in the brain. It: Receives Inputs: Takes signals (numerical values) from other neurons or raw data. Processes the Input: Combines the inputs with learned weights (importance of each input) and adds a bias(adjustment term). Applies an Activation Function: Determines whether the neuron "fires" (produces output) by applying a mathematical function (e.g., sigmoid). Sends an Output: Passes the processed signal to other neurons or as the final result The sigmoid function is a mathematical function often used in machine learning, particularly in logistic regression and neural networks. Its main purpose is to map any real-valued input to a value between 0 and 1, making it suitable for tasks like binary classification. Key Components of Deep Learning What is an Artificial Neuron? An artificial neuron is inspired by biological neurons in the brain. It: Receives Inputs: Takes signals (numerical values) from other neurons or raw data. Processes the Input: Combines the inputs with learned weights (importance of each input) and adds a bias(adjustment term). Applies an Activation Function: Determines whether the neuron "fires" (produces output) by applying a mathematical function (e.g., sigmoid). Sends an Output: Passes the processed signal to other neurons or as the final result The sigmoid function is a mathematical function often used in machine learning, particularly in logistic regression and neural networks. Its main purpose is to map any real-valued input to a value between 0 and 1, making it suitable for tasks like binary classification. Key Components of Deep Learning What is an Artificial Neuron? An artificial neuron is inspired by biological neurons in the brain. It: Receives Inputs: Takes signals (numerical values) from other neurons or raw data. Processes the Input: Combines the inputs with learned weights (importance of each input) and adds a bias(adjustment term). Applies an Activation Function: Determines whether the neuron "fires" (produces output) by applying a mathematical function (e.g., sigmoid). Sends an Output: Passes the processed signal to other neurons or as the final result The sigmoid function is a mathematical function often used in machine learning, particularly in logistic regression and neural networks. Its main purpose is to map any real-valued input to a value between 0 and 1, making it suitable for tasks like binary classification. Key Components of Deep Learning What is an Artificial Neuron? An artificial neuron is inspired by biological neurons in the brain. It: Receives Inputs: Takes signals (numerical values) from other neurons or raw data. Processes the Input: Combines the inputs with learned weights (importance of each input) and adds a bias(adjustment term). Applies an Activation Function: Determines whether the neuron "fires" (produces output) by applying a mathematical function (e.g., sigmoid). Sends an Output: Passes the processed signal to other neurons or as the final result The sigmoid function is a mathematical function often used in machine learning, particularly in logistic regression and neural networks. Its main purpose is to map any real-valued input to a value between 0 and 1, making it suitable for tasks like binary classification. Key Components of Deep Learning What is an Artificial Neuron? An artificial neuron is inspired by biological neurons in the brain. It: Receives Inputs: Takes signals (numerical values) from other neurons or raw data. Processes the Input: Combines the inputs with learned weights (importance of each input) and adds a bias(adjustment term). Applies an Activation Function: Determines whether the neuron "fires" (produces output) by applying a mathematical function (e.g., sigmoid). Sends an Output: Passes the processed signal to other neurons or as the final result The sigmoid function is a mathematical function often used in machine learning, particularly in logistic regression and neural networks. Its main purpose is to map any real-valued input to a value between 0 and 1, making it suitable for tasks like binary classification. Real-World Example: Imagine teaching a computer to identify a cat: Input Layer: Feeds the computer raw image data (pixel values). Hidden Layers: Teach it to recognize whiskers, ears, fur texture, and then combine them to identify "catness.» Real-World Example: Imagine teaching a computer to identify a cat: Input Layer: Feeds the computer raw image data (pixel values). Hidden Layers: Teach it to recognize whiskers, ears, fur texture, and then combine them to identify "catness.» Hidden Layers: These layers sit between the input and output layers. They extract patterns, such as: Early layers: Detect basic features (e.g., edges, corners). Deeper layers: Recognize more complex patterns (e.g., shapes, objects). Hidden layers use weights and biases to refine the data and activation functions to decide the strength of signals passed forward. Real-World Example: Imagine teaching a computer to identify a cat: Input Layer: Feeds the computer raw image data (pixel values). Hidden Layers: Teach it to recognize whiskers, ears, fur texture, and then combine them to identify "catness.» Hidden Layers: These layers sit between the input and output layers. They extract patterns, such as: Early layers: Why AreDetect Hiddenbasic features Layers Important? Hiddencorners). (e.g., edges, layers create a hierarchical representation of data: Deeper layers: Recognize Simple more patterns (e.g., lines, textures) complex patternsbuild (e.g., shapes, up to more complex ideas (e.g., a objects). face or an object). Hidden layers use weights This automation eliminates the need and biases to for manual feature extraction, which refine the data and activation was requiredfunctions in older machine learning to decide the strengthtechniques. of signals passed forward. Real-World Example: Imagine teaching a computer to identify a cat: Input Layer: Feeds the computer raw image data (pixel values). Hidden Layers: Teach it to recognize whiskers, ears, fur texture, and then combine them to identify "catness.» Hidden Layers: These layers sit between the input and output layers. They extract patterns, such as: Early layers: Why AreDetect Hiddenbasic features Layers Important? Hiddencorners). (e.g., edges, layers create a hierarchical representation of data: Deeper layers: Recognize Simple more patterns (e.g., lines, textures) complex patternsbuild (e.g., shapes, up to more complex ideas (e.g., a objects). face or an object). Hidden layers use weights This automation eliminates the need and biases to for manual feature extraction, which refine the data and activation was requiredfunctions in older machine learning to decide the strengthtechniques. of signals passed forward. Deep learning in medicine – any thoughts? 10 minutes open source, each of you one application Deep learning in medicine – any thoughts? Medical Imaging - Identifying conditions like cancer, pneumonia, and bone fractures from diagnostic images such as X-rays, CT scans, and MRIs. - to automate tumor identification in histopathology slides, reducing manual effort. Drug Discovery - Predicting interactions between drugs and their targets, as well as determining their chemical properties. - Employing generative models to design new molecules for specific therapeutic needs. - Solving protein structures with AI systems like AlphaFold to accelerate biomedical research. Deep learning in medicine – any thoughts? Medical Imaging - Identifying conditions like cancer, pneumonia, and bone fractures from diagnostic images such as X-rays, CT scans, and MRIs. - to automate tumor identification in histopathology slides, reducing manual effort. Drug Discovery - Predicting interactions between drugs and their targets, as well as determining their chemical properties. - Employing generative models to design new molecules for specific therapeutic needs. - Solving protein structures with AI systems like AlphaFold to accelerate biomedical research. AlphaFold2 developed by DeepMind, revolutionized protein structure prediction by using deep learning techniques to achieve near-experimental accuracy in determining the 3D structure of proteins from their amino acid sequences. AlphaFold2 integrates evolutionary information, geometry, and deep learning to predict protein structures. 1. Protein Folding Problem: Proteins are made of amino acids that fold into specific 3D shapes, which determine their function. Predicting these shapes from a linear sequence is complex due to the vast number of possible configurations. 2. Sequence-Structure Relationship: The amino acid sequence of a protein dictates its final structure, but the folding process involves intricate physical and biochemical interactions. Input Data - Protein Sequence: The primary amino acid sequence of the protein to be folded. - Multiple Sequence Alignments (MSA): Uses evolutionary data from related protein sequences to infer conserved structural features. - Templates (optional): Existing structures of similar proteins to guide predictions. AlphaFold2 integrates evolutionary information, geometry, and deep learning to predict protein structures. Neural Network Architecture - Evoformer: - Processes the MSA to identify patterns of evolutionary conservation and co-evolution. - Captures pairwise relationships between amino acids (distance constraints, contact maps). - Structure Module: - Builds the 3D coordinates of the protein by iteratively refining a structure. - Utilizes geometric reasoning to predict angles, bond lengths, and residue positions. AlphaFold2 integrates evolutionary information, geometry, and deep learning to predict protein structures. Neural Network Architecture - Evoformer: - Processes the MSA to identify patterns of evolutionary conservation and co-evolution. - Captures pairwise relationships between amino acids (distance constraints, contact maps). - Structure Module: - Builds the 3D coordinates of the protein by iteratively refining a structure. - Utilizes geometric reasoning to predict angles, bond lengths, and residue positions. MJ Pietal et al. Bioinformatics 2015 AlphaFold2 integrates evolutionary information, geometry, and deep learning to predict protein structures. Input Data - Protein Sequence: The primary amino acid sequence of the protein to be folded. - Multiple Sequence Alignments (MSA): Uses evolutionary data from related protein sequences to infer conserved structural features. - Templates (optional): Existing structures of similar proteins to guide predictions. Neural Network Architecture - Evoformer: - Processes the MSA to identify patterns of evolutionary conservation and co- evolution. - Captures pairwise relationships between amino acids (distance constraints, contact maps). - Structure Module: - Builds the 3D coordinates of the protein by iteratively refining a structure. - Utilizes geometric reasoning to predict angles, bond lengths, and residue positions. AlphaFold2 integrates evolutionary information, geometry, and deep learning to predict protein structures. Training Data and Techniques 1. Databases Used: - Trained on publicly available protein databases like PDB (Protein Data Bank). 2. Loss Function: - Optimized to minimize the difference between predicted and actual structures. 3. Computation: - Extensive use of GPUs/TPUs for training and inference. Applications of AlphaFold2 1. Drug Discovery: - Identifying targets and designing inhibitors for diseases. 2. Enzyme Engineering: - Creating enzymes for industrial and environmental purposes. 3. Disease Understanding: - Studying the role of protein misfolding in conditions like Alzheimer’s. Deep learning in medicine – simple example from MIMLAB Automated Brain Extraction for Multi-Contrast MRI in Rats Using Deep Learning Deep learning in medicine – simple example from MIMLAB Automated Brain Extraction for Multi-Contrast MRI in Rats Using Deep Learning Leen Hakki, Melisa Özakçakaya, Belal Tavashi, Uluç Pamuk, Oğuzhan Hüraydın, Esin Öztürk Işık, Pınar Senay Özbay MRI is a powerful tool for studying rodent brain structure and function, but preprocessing steps like skull stripping (removal of non-brain tissue) are essential for reliable group-level analyses. Skull stripping is critical for accurate atlas registration, and segmentation method. Current rodent methods are often manual and inconsistent. Animal studies are not that common as human, so limited source is available. The aim is to develop an automatic brain extraction method for rodent MRI scans with multiple contrasts, supporting preprocessing pipelines for aging studies that require consistent and reliable brain extraction Automated Brain Extraction for Multi-Contrast MRI in Rats Using Deep Learning Data Acquisition - Subjects: 36 female Wistar rats. - Imaging System: 7T preclinical MRI scanner - (MR Solutions Ltd. @Kandilli). - MRI Sequences for multiparametric mapping: - T1-weighted - T2-weighted - Multi Gradient Echo (MGE) - Data for Model Training: Only T2-weighted images were used. - Segmentation: - Scans were (auto)-manually segmented using 3D Slicer. - Segmentation followed the Tohoku Rat Brain Atlas. - Assessment was performed by two operators for reliability. Automated Brain Extraction for Multi-Contrast MRI in Rats Using Deep Learning Preprocessing: MRI images and their corresponding masks were sliced and saved individually as JPEG files to prepare the input data for the U-Net model. A total of 26 brain images (572 slices) were used for training, 5 images (129 slices) for testing, and 5 images (123 slices) for validation. The images were resized to 256x256 pixels and normalized. Before inputting the data into the model, data augmentation was applied to improve model robustness across multi-contrast MRI data. Automated Brain Extraction for Multi-Contrast MRI in Rats Using Deep Learning 3x3 Convolutions: What it does: Applies a small 3x3 filter (or kernel) across the input data (image or feature map) to extract spatial features. Why it's used: The 3x3 size strikes a balance between capturing fine details (local patterns) and computational efficiency. It is small enough to reduce computation while large enough to capture important spatial relationships in an image. Automated Brain Extraction for Multi-Contrast MRI in Rats Using Deep Learning Automated Brain Extraction for Multi-Contrast MRI in Rats Using Deep Learning 3x3 Convolutions: What it does: Applies a small 3x3 filter (or kernel) across the input data (image or feature map) to extract spatial features. Why it's used: The 3x3 size strikes a balance between capturing fine details (local patterns) and computational efficiency. It is small enough to reduce computation while large enough to capture important spatial relationships in an image. Automated Brain Extraction for Multi-Contrast MRI in Rats Using Deep Learning Automated Brain Extraction for Multi-Contrast MRI in Rats Using Deep Learning Automated Brain Extraction for Multi-Contrast MRI in Rats Using Deep Learning The importance of accurate brain extraction for the correct registration can be seen in Figure 4, as U-Net-based brain extraction effectively registered the Tohoku atlas on the MGE image, however, the FSL mask was misaligned due to less accurate brain extraction. This will ease our calculations in future analysis, e.g. multiparametric imaging during aging.