Statistics: Measures of Association (PDF)
Document Details
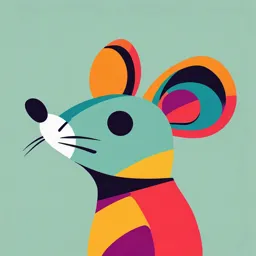
Uploaded by ConscientiousEvergreenForest1127
Toronto Metropolitan University
Tags
Related
- Stats Unit-3: Introduction to R PDF
- Sociology TYBA Paper 9 Research Methodology (Marathi) PDF
- Elementary Statistics in Social Research (12th Edition) PDF
- PSCI 2702 Textbook: Statistics for Social Research - PDF
- Chap 9 Exploring Statistics PDF
- Quantitative Methods in Social Science Research - 360-223-AB
Summary
This is a chapter from a textbook on statistics, focusing on measures of association for variables measured at the nominal level. It explains how to use conditional distributions, percentages, and measures like phi, Cramer's V, and lambda to analyze relationships in your data and introduces the concept of association in bivariate analysis.
Full Transcript
Book Title: eTextbook: Statistics: A Tool for Social Research and Data Analysis, Fifth Canadian Edition Chapter 8. Measures of Association for Variables Measured at the Nominal Level Chapter 8. Measures of Association for Variables Measured at the Nominal Level Learning Objectives 250 B...
Book Title: eTextbook: Statistics: A Tool for Social Research and Data Analysis, Fifth Canadian Edition Chapter 8. Measures of Association for Variables Measured at the Nominal Level Chapter 8. Measures of Association for Variables Measured at the Nominal Level Learning Objectives 250 By the end of this chapter, you will be able to 1. Explain how to use measures of association to describe and analyze the importance of relationships (versus their statistical significance) 2. Define association in the context of bivariate tables and in terms of changing conditional distributions 3. List and explain the three characteristics of a bivariate relationship: existence, strength, and pattern or direction 4. Investigate a bivariate association by properly calculating percentages for a bivariate table and interpreting the results 5. Compute and interpret three measures of association for variables measured at the nominal level: phi, Cramer’s V, and lambda Book Title: eTextbook: Statistics: A Tool for Social Research and Data Analysis, Fifth Canadian Edition Chapter 8. Measures of Association for Variables Measured at the Nominal Level 8.1. Introduction 8.1. Introduction As we saw in Chapter 7, tests of statistical significance are extremely important in social science research. As long as social scientists must work with samples rather than populations, these tests are indispensable for dealing with the possibility that our research results are the products of mere random chance. However, tests of significance are often merely the first step in the analysis of research results. These tests do have limitations, and statistical significance is not necessarily the same thing as relevance or importance. Furthermore, tests of statistical significance are affected by sample size, so large samples may result in decisions to reject the null hypothesis when, in fact, the observed relationship between variables is quite weak. Beginning with this chapter, we will be working with a class of descriptive statistics called measures of association. Whereas tests of significance detect non-random relationships, measures of association provide information about the strength and, where appropriate, the direction of relationships between variables in our data set—information that is more directly relevant for assessing the importance of relationships and testing the power and validity of our theories. The theories that guide scientific research are almost always stated in cause-and-effect terms (e.g., “variable X causes variable Y”). As an example, recall our discussion of the materialistic hypothesis in Chapter 1. In that theory, the causal (or independent) variable was social class and the effect (or dependent) variable was health status. The theory asserts that social class causes health status. Measures of association help us trace causal relationships among variables, and they are our most important and powerful statistical 251 tools for documenting, measuring, and analyzing cause-and-effect relationships. As useful as they are, measures of association, like any class of statistics, do have their limitations. Most important, these statistics cannot prove that two variables are causally related. Even if there is a strong (and significant) statistical association between two variables, we cannot necessarily conclude that one variable is a cause of the other. As an example, lobbyists for tobacco companies argued for years that correlation studies showing an association between smoking and lung cancer could not prove that smoking directly caused cancer. We will explore causation and how to assess it in more detail in Part 4, but for now you should keep in mind that causation and association are two different things. We can use a statistical association between variables as evidence for a causal relationship, but association by itself is not proof that a causal relationship exists. Another important use for measures of association is prediction. If two variables are associated, we can predict the score of a case on one variable from the score of that case on the other variable. For example, if social class and health are associated, we can predict that people who have high social class are healthier than those with low social class. Note that prediction and causation can be two separate matters. If variables are associated, we can predict from one to the other even if the variables are not causally related. In conclusion, this chapter begins by introducing the concept of association between variables in the context of bivariate tables and will stress the use of percentages to analyze associations between variables, as introduced in Chapter 7. We will then proceed to the logic, calculation, and interpretation of some widely used measures of association. By the end of the chapter, you will have an array of statistical tools you can use to analyze the strength and direction of associations between variables. 252 Book Title: eTextbook: Statistics: A Tool for Social Research and Data Analysis, Fifth Canadian Edition Chapter 8. Measures of Association for Variables Measured at the Nominal Level 8.2. Association between Variables and the Bivariate Table 8.2. Association between Variables and the Bivariate Table Most generally, two variables are said to be associated if the distribution of one of them changes under the various categories or scores of the other. For example, suppose that an industrial sociologist was concerned with the relationship between job satisfaction and productivity for assembly-line workers. If these two variables are associated, then scores on productivity will change under the different conditions of satisfaction. Highly satisfied workers will have different scores on productivity than workers who are low on satisfaction, and levels of productivity will vary by levels of satisfaction. This relationship will become clearer with the use of bivariate tables. As we discussed in Chapter 7, bivariate tables display the scores of cases on two different variables. By convention, the independent variable or X (i.e., the variable taken as causal) is arrayed in the columns, and the dependent variable or Y in the rows (for the sake of brevity, we will often refer to the independent variable as X and the dependent variable as Y in the material that follows). Each non-marginal column of the table (the vertical dimension) represents a score or category of the independent variable (X), and each non-marginal row (the horizontal dimension) represents a score or category of the dependent variable (Y). Table 8.1 displays the relationship between productivity and job satisfaction for a fictitious sample of 173 factory workers. We focus on the columns to detect the presence of an association between variables displayed in table format. Each column shows the pattern of scores on the dependent variable for each score on the independent variable. For example, the left-hand column indicates that 30 of the 60 workers who were low on job satisfaction were low on productivity, 20 were moderate on productivity, and 10 were high on productivity. The middle column shows that 21 of the 61 moderately satisfied workers were low on productivity, 25 were moderate on productivity, and 15 were high on productivity. Of the 52 workers who are highly satisfied (the right-hand column), 7 were low on productivity, 18 were moderate, and 27 were high. Table 8.1 Productivity by Job Satisfaction (Frequencies) By inspecting the table from column to column, we can observe the effects of the independent variable on the dependent variable (provided, of course, that the table is constructed with the independent variable in the columns). These 253 “within-column” frequency distributions are called the conditional distributions of Y; they display the distribution of scores on the dependent variable for each condition (or score) of the independent variable. Stokkete/shutterstock.com Simple bivariate tables can be used to conveniently describe the association between variables like job dissatisfaction and productivity. Table 8.1 indicates that productivity and satisfaction are associated: the distribution of scores on Y (productivity) changes across the various conditions of X (satisfaction). For example, half of the workers who were low on satisfaction were also low on productivity (30 out of 60), and over half of the workers who were high on satisfaction were high on productivity (27 out of 52). Although it is intended to be a test of significance, the chi square statistic provides another way to detect the existence of an association between two variables that are organized in table format. Any non-zero value for the obtained chi square indicates that the variables are associated. For example, the obtained chi square for Table 8.1 is 24.20, a value that affirms our previous conclusion, based on the conditional distributions of Y, that an association of some sort exists between job satisfaction and productivity. Often, the researcher will have already conducted a chi square test before considering matters of association. In such cases, it is not necessary to inspect the conditional distributions of Y to ascertain whether the two variables are associated. If the obtained chi square is zero, the two variables are independent and not associated. Any value other than zero indicates some association between the variables. Remember, however, that statistical significance (inferential statistics) and association (descriptive statistics) are two different things. It is perfectly possible for two variables to be associated (as indicated by a non-zero chi square) but still independent in the population (if we fail to reject the null hypothesis). In this section, we defined, in a general way, the concept of association between two variables. We have also shown two different ways to detect the presence of an association. In the next section, we will extend the analysis beyond questions of the mere presence or absence of an association and, in a systematic way, see how we can develop additional, very useful information about the relationship between two variables. Book Title: eTextbook: Statistics: A Tool for Social Research and Data Analysis, Fifth Canadian Edition 8.3. Three Characteristics of Bivariate Associations 8.3. Three Characteristics of Bivariate Associations Bivariate associations have three different characteristics, each of which must be analyzed for a full investigation of the relationship. Investigating these characteristics may be thought of as a process of finding answers to three questions: 1. Does an association exist? 2. If an association does exist, how strong is it? 254 3. If an association does exist, what are the pattern and/or the direction of the association? We will consider each of these questions separately. Book Title: eTextbook: Statistics: A Tool for Social Research and Data Analysis, Fifth Canadian Edition 8.3. Three Characteristics of Bivariate Associations Does an Association Exist? Does an Association Exist? We have already discussed the general definition of association, and we have seen that we can detect an association by using chi square or by observing the conditional distributions of Y in a table. In Table 8.1, we know that the two variables are associated to some extent because the conditional distributions of productivity (Y) are different across the various categories of satisfaction (X) and because the chi square statistic is non-zero. Comparisons from column to column in Table 8.1 are relatively easy to make because the column totals are roughly equal. This is not usually the case, and it is helpful to compute percentages to control for varying column totals. These column percentages, introduced in Chapter 7, are computed within each column separately and make the pattern of association more visible. The general procedure for detecting association with bivariate tables is to compute percentages within the columns (vertically or down each column) and then compare column to column across the table (horizontally or across the rows). We can conveniently remember this procedure with the following statement: “Percentage Down, Compare Across.” Table 8.2 presents column percentages calculated from the data in Table 8.1. Besides controlling for any differences in column totals, tables in percentage form are usually easier to read because changes in the conditional distributions of Y are easier to detect. Table 8.2 Productivity by Job Satisfaction (Percentages) In Table 8.2, we can see that the cell with the largest value changes position from column to column. For workers who are low on satisfaction, the cell with the single largest value is in the top row (low on productivity). For the middle column (moderate on satisfaction), the cell with the largest value is in the middle row (moderate on productivity), and for the right-hand column (high on satisfaction), it is in the bottom row (high on productivity). Even a cursory glance at the conditional distributions of Y in Table 8.2 reinforces our conclusion that an association does exist between these two variables. 255 If two variables are not associated, then the conditional distributions of Y do not change across the columns. The distribution of Y is the same for each condition of X. Table 8.3 illustrates a perfect non-association between age and productivity. Table 8.3 is only one of many patterns that indicate no association. The important point is that the conditional distributions of Y are the same. Levels of productivity do not change at all for the various age groups and, therefore, no association exists between these variables. Also, the obtained chi square computed from this table has a value of zero, again indicating no association. Table 8.3 Productivity by Age (an Illustration of no Association) The use of panelled pie charts and clustered bar charts can also help us recognize association between variables. Both of these bivariate graphs are visual representations of the conditional distributions of Y shown in the bivariate table. As we saw with the univariate pie and bar charts in Chapter 2, pie charts are especially useful for nominal and ordinal variables that do not have many response categories, and bar charts are very useful for nominal and ordinal variables with many response categories and when we want to preserve the ranked order of ordinal variable response categories in the chart. To construct a panelled pie chart, begin by computing the column percentages. Then draw a circle (a pie) for each response category of the independent variable. Each pie thus represents 100% of the cases in its respective response category. Next, divide each circle (each pie) into segments (slices) proportional to the percentages shown in its respective response category’s distribution of Y. Be sure that the chart and all segments are clearly labelled. Figure 8.1 shows the panelled pie chart for the conditional distributions of Y of Table 8.2. Figure 8.1 Panelled Pie Chart of Productivity by Job Satisfaction (Percentages) To detect variable relationships with the panelled pie chart, look for changes in the size of each dependent-variable (productivity) slice from one pie to the next, across all the values of the independent variable (job satisfaction). As shown in Figure 8.1, the high-productivity slice increases in size as job satisfaction increases, while the low-productivity slice decreases in size. A clustered bar chart is constructed in a somewhat similar way as we used for the panelled pie chart. To construct a clustered bar chart, begin by computing the column percentages. Then, place the dependent-variable response 256 categories along the horizontal axis (i.e., abscissa) and the percentages on the vertical axis (i.e., ordinate). Above each dependent-variable response category, construct a rectangle of constant width for every independent-variable response category. The height of each independent-variable response category should correspond to its conditional distribution of Y. Be sure that the chart and all bars are clearly labelled. Figure 8.2 shows the clustered bar chart for the conditional distributions of Y of Table 8.2. 257 Figure 8.2 Clustered Bar Chart of Productivity by Job Satisfaction (Percentages) To detect variable relationships with the clustered bar chart, look for changes in the height of each independent-variable (job satisfaction) value bar from one set of clustered bars to the next, across all values of the dependent variable (productivity). As shown in Figure 8.2, as the high job satisfaction bar increases in height, productivity increases, while the low-productivity bar decreases in height. Book Title: eTextbook: Statistics: A Tool for Social Research and Data Analysis, Fifth Canadian Edition 8.3. Three Characteristics of Bivariate Associations How Strong Is the Association? How Strong Is the Association? Once we establish the existence of the association, we need to develop some idea of how strong it is. This is essentially a matter of determining the amount of change in the conditional distributions of Y. At one extreme, of course, there is the case of no association, where the conditional distributions of Y do not change at all (see Table 8.3). At the other extreme is a perfect association, the strongest possible relationship. In general, a perfect association exists between two variables if each value of the dependent variable is associated with one and only one value of the independent variable. In a bivariate table, all cases in each column would be located in a single cell and there would be no variation in Y for a given value of X (see Table 8.4). Table 8.4 Productivity by Age (an Illustration of Perfect Association) A perfect relationship is taken as very strong evidence of a causal relationship between the variables, at least for the sample at hand. In fact, the results presented in Table 8.4 indicate that, for this sample, age is the sole cause of productivity. Also, in the case of a perfect relationship, predictions from one variable to the other can be made without error. If we know that a particular worker is between the ages of 25 and 34, for example, we can be sure that they are highly productive. Of course, the huge majority of relationships fall somewhere between the two extremes of no association and perfect association. We need to develop some way of describing these intermediate relationships consistently and meaningfully. For example, Tables 8.1 and 8.2 show that there is an association between productivity and job satisfaction. How could this relationship be described in terms of strength? How close to perfect is the relationship? How far away from no association is it? To answer these questions, researchers rely on statistics called measures of association, a variety of which are presented later in this chapter and in the 258 chapters to follow. Measures of association provide precise, objective indicators of the strength of a relationship. Virtually all of these statistics are designed so that they have a lower limit of 0.00 and an upper limit of 1.00 ( ±1.00 for ordinal and interval-ratio measures of association). A measure that equals 0.00 indicates no association between the variables (the conditional distributions of Y do not vary), and a measure of 1.00 ( ±1.00 in the case of ordinal and interval-ratio measures) indicates a perfect relationship. The exact meaning of values between 0.00 and 1.00 varies from measure to measure, but for all measures, the closer the value is to 1.00, the stronger the relationship (the greater the change in the conditional distributions of Y). We will begin to consider measures of association used in social research later in this chapter. At this point, let’s consider the maximum difference, a less formal way of assessing the strength of a relationship based on comparing column percentages across the rows. This technique is quick and easy to apply (at least for small tables) but limited in its usefulness. To calculate the maximum difference, compute the column percentages as usual and then skim the table across each of the rows to find the largest difference in any row between column percentages. For example, the largest difference in column percentages in Table 8.2 is in the top row between the “Low” column and the “High” column: 50.00% − 13.46% = 36.54%. The maximum difference in the middle row is between “moderates” and “lows” (40.98% − 33.33% = 7.65%) , and in the bottom row, it is between “highs” and “lows” (51.92% − 16.67% = 35.25%). Both of the latter values are less than the maximum difference in the top row. Once you have found the maximum difference in the table, you can use the scale presented in Table 8.5 to describe the strength of the relationship. Using this scale, we can describe the relationship between productivity and job satisfaction in Table 8.2 as strong. Table 8.5 The Relationship between the Maximum Difference and the Strength of the Relationship Maximum Difference Strength If the maximum difference is The strength of the relationship is between 0 and 10 weak percentage points moderate between 11 and 30 strong percentage points more than 30 percentage points You should be aware that the relationships between the size of the maximum difference and the descriptive terms (weak, moderate, and strong) in Table 8.5 are arbitrary and approximate. We will get more precise and useful information when we compute and analyze measures of association, beginning later in this chapter. Also, maximum differences are easiest to find and most useful for smaller tables. In large tables, with many (say, more than three) 259 columns and rows, it can be cumbersome to find the high and low percentages and it is advisable to consider only measures of association as indicators of the strength of relationships for these tables. Finally, note that the maximum difference is based on only two values (the high and low column percentages within any row). Like the range (see Chapter 3), this statistic can give a misleading impression of the overall strength of the relationship. Within these limits, however, the maximum difference can provide a useful, quick, and easy way of characterizing the strength of relationships (at least for smaller tables). As a final caution, do not mistake chi square as an indicator of the strength of a relationship. Even very large values for chi square do not necessarily mean that the relationship is strong. Remember that significance and association are two separate matters and that chi square, by itself, is not a measure of association. While a non-zero value indicates that there is some association between the variables, the magnitude of chi square bears no particular relationship to the strength of the association. However, as we will see later in this chapter, there are ways to transform chi square into other statistics that do measure the strength of the association between two variables. (For practice in computing percentages and judging the existence and strength of an association, see any of the problems at the end of this chapter.) Book Title: eTextbook: Statistics: A Tool for Social Research and Data Analysis, Fifth Canadian Edition 8.3. Three Characteristics of Bivariate Associations What Is the Pattern and/or the Direction of the Association? What Is the Pattern and/or the Direction of the Association? Investigating the pattern of the association requires that we ascertain which values or categories of one variable are associated with which values or categories of the other. We have already remarked on the pattern of the relationship between productivity and satisfaction. Table 8.2 indicates that low scores on satisfaction are associated with low scores on productivity, moderate satisfaction with moderate productivity, and high satisfaction with high productivity. When both variables are at least ordinal in level of measurement, the association between the variables may also be described in terms of direction. The direction of the association can be positive or negative. An association is positive if the variables vary in the same direction. That is, in a positive association, high scores on one variable are associated with high scores on the other variable, and low scores on one variable are associated with low scores on the other. In a positive association, as one variable increases in value, the other also increases, and as one variable decreases, the other also decreases. Table 8.6 displays, with fictitious data, a positive 260 relationship between education and use of public libraries. As education increases (as you move from left to right across the table), library use also increases (the percentage of “high” users increases). The association between job satisfaction and productivity, as displayed in Tables 8.1 and 8.2, is also a positive association. Table 8.6 Library Use by Education (an Illustration of a Positive Relationship) In a negative association, the variables vary in opposite directions. High scores on one variable are associated with low scores on the other, and increases in one variable are accompanied by decreases in the other. Table 8.7 displays a negative relationship, again with fictitious data, between education and television viewing. The amount of television viewing decreases as education increases. In other words, as you move from left to right across the top of the table (as education increases), the percentage of high television viewing decreases. Table 8.7 Amount of Television Viewing by Education (an Illustration of a Negative Relationship) Measures of association for ordinal and interval-ratio variables are designed so that they take on positive values for positive associations and negative values for negative associations. Thus, a measure of association preceded by a plus sign indicates a positive relationship between the two variables, with the value +1.00 indicating a perfect positive relationship. A negative sign indicates a negative relationship, with −1.00 indicating a perfect negative relationship. We will consider the direction of relationships in more detail in Chapters 9 and 13. (For practice in determining the pattern of an association, see any of the end-of- chapter problems. For practice in determining the direction of a relationship, see Problems 8.1 and 8.7.) 261 Book Title: eTextbook: Statistics: A Tool for Social Research and Data Analysis, Fifth Canadian Edition Chapter 8. Measures of Association for Variables Measured at the Nominal Level 8.4. The Importance of Percentages and Some Errors of Interpretation 8.4. The Importance of Percentages and Some Errors of Interpretation We have seen how percentages can be used to analyze associations between variables. While they are among the humblest of statistics, percentages can provide important information about the relationship between two variables. Of course, this information will be clear and accurate only when bivariate tables are properly constructed, percentages are computed within columns, and comparisons are made from column to column. Errors and misunderstandings can occur when there is confusion about which variable is the cause (or independent variable) and which is the effect (or dependent variable), or when the researcher asks the wrong questions about the relationship. To illustrate these problems, let’s review the process by which we analyze bivariate tables. When we compare column percentages across the table, we are asking: “Does Y (the dependent variable) vary by X (the independent variable)?” We conclude that there is evidence for a causal relationship if the values of Y change for the different values of X. To illustrate further, consider Table 8.8, which shows the relationship between age—15 to 44, 45 to 64, and 65 and older—and the importance of religion in people’s lives. The data are taken from the Canadian sample of the World Values Survey (Wave 5)—a global survey on people’s values, beliefs, and well- being. Age must be the independent or causal variable in this relationship since it may shape people’s attitudes and opinions. The reverse cannot be true: a person’s opinion cannot cause their age. Age is the column variable in the table, and the percentages are computed in the proper direction. A quick inspection shows that importance of religion varies by age group, indicating that there may be a causal relationship between these variables. The maximum difference between the columns is about 27 percentage points, indicating that the relationship is moderate to strong. Table 8.8 Importance of Religion in Life by Age Group (Frequencies and Percentages) Source: Data from World Values Survey Association, World Values Survey, Wave 5. What if we had misunderstood this causal relationship? If we had computed percentages within each row, for example, age would become the dependent variable. We would be asking “Does age vary by the importance of religion in 262 peoples’ lives?” Table 8.9 shows the results of asking this incorrect question. Table 8.9 Row Percentages A casual glance at the top row of the table seems to indicate a causal relationship since 41.03% of the respondents who said that religion is important in their lives are younger (15–44) adults, while 35.78% are middle- aged (44–64) adults, and just 23.19% are older (65+) adults. If we looked only at the top row of the table (as people sometimes do), we would conclude that younger persons attach more importance to religion than older persons. But the second row shows that younger adults are also the majority (56.85%) of those who said that religion is not important in their lives. How can this be? The row percentages in this table simply reflect the fact that younger adults outnumber the other age groups, especially older adults: for example, there are almost three times as many young adults as older adults in the sample. Computing percentages within the rows would make sense only if age could vary by attitude or opinion, and Table 8.9 could easily lead to false conclusions about this relationship. Professional researchers sometimes compute percentages in the wrong direction or ask a question about the relationship incorrectly, and you should always check bivariate tables to make sure that the analysis agrees with the patterns in the table. Book Title: eTextbook: Statistics: A Tool for Social Research and Data Analysis, Fifth Canadian Edition Chapter 8. Measures of Association for Variables Measured at the Nominal Level 8.5. Introduction to Measures of Association 8.5. Introduction to Measures of Association Conditional distributions, column percentages, and the maximum difference provide very useful information about the bivariate association and should always be computed and analyzed. However, they can be awkward and cumbersome to use, especially for larger tables. Measures of association, on the other hand, characterize the strength (and for ordinal and interval-ratio variables, also the direction) of bivariate relationships in a single number—a more compact and convenient format for interpretation and discussion. There are many measures of association, but we will confine our attention to a few of the most widely used ones. We will cover these statistics by the level of measurement for which they are most appropriate. In this chapter, we will consider measures appropriate for nominally measured variables. In the next chapter, we will cover measures of association for ordinal-level variables, and 263 in Chapter 13, we will consider Pearson’s r, a measure of association or correlation for interval-ratio-level variables. You will note that several of the research situations used as examples involve variables measured at different levels (e.g., one nominal-level variable and one ordinal-level variable). The general procedure in the situation of mixed levels is to be conservative and select measures of association that are appropriate for the lower of the two levels of measurement. However, special measures of association are sometimes available for special situations. For example, Chapter 12 includes measures of association for variables with mixed levels of measurement, when the independent variable is either nominal or ordinal and the dependent variable is interval-ratio. Book Title: eTextbook: Statistics: A Tool for Social Research and Data Analysis, Fifth Canadian Edition 8.6. Chi Square–Based Measures of Association 8.6. Chi Square–Based Measures of Association When working with nominal-level variables, social science researchers rely heavily on measures of association based on the value of chi square. While chi square per se is a test of statistical significance, it can be transformed into other statistics that measure the strength of the association between two variables. When the value of chi square is already known, these measures are easy to calculate. To illustrate, let us reconsider Table 7.5, which displayed, with fictitious data, a relationship between CASSW (Canadian Association of Schools of Social Work) accreditation and employment for social work graduates. For the sake of convenience, this table is reproduced here as Table 8.10. Table 8.10 Employment Status by CASSW-Accreditation Status We saw in Chapter 7 that this relationship is statistically significant ( χ2 = 10.78 , which is significant at the 0.05 alpha level), but the question now concerns the strength of the association. A brief glance at Table 8.10 shows that the conditional distributions of employment status do change, so the variables are associated. To emphasize this point, it is always helpful to calculate column percentages, as in Table 8.11. Table 8.11 Employment Status by CASSW-Accreditation Status (Percentages) So far, we know that the relationship between these two variables is statistically significant and that there is an association of some kind between CASSW accreditation and employment. To assess the strength of the association, we will compute phi (ϕ). This statistic is a frequently used chi 264 square–based measure of association appropriate for 2 × 2 tables (i.e., tables with two rows and two columns). Book Title: eTextbook: Statistics: A Tool for Social Research and Data Analysis, Fifth Canadian Edition 8.6. Chi Square–Based Measures of Association Calculating Phi Calculating Phi One attraction of phi is that it is easy to calculate. Simply divide the value of the obtained chi square by the total number of cases in the sample (n) and take the square root of the result. Expressed in symbols, the formula for phi is Formula 8.1 χ2 ϕ=√ n For the data displayed in Table 8.10, the chi square is 10.78. Therefore, phi is χ 2 10.78 ϕ=√ =√ = 0.33 n 100 For a 2 × 2 table, phi ranges in value from 0 (no association) to 1.00 (perfect association). The closer phi is to 1.00, the stronger the relationship; the closer it is to 0.00, the weaker the relationship. For Table 8.10, we already knew that the relationship was statistically significant at the 0.05 level. Phi, as a measure of association, adds information about the strength of the relationship. As for the pattern of the association, the column percentages in Table 8.11 show that graduates of CASSW-accredited programs were more often employed as social workers. Book Title: eTextbook: Statistics: A Tool for Social Research and Data Analysis, Fifth Canadian Edition 8.6. Chi Square–Based Measures of Association Calculating Cramer’s V Calculating Cramer’s V For tables larger than 2 × 2 (specifically, for tables with more than two columns and more than two rows), the upper limit of phi can exceed 1.00. This makes phi difficult to interpret, so a more general form of the statistic called Cramer’s V must be used for larger tables. The formula for Cramer’s V is Formula 8.2 χ2 V =√ n[min(r − 1), (c − 1)] where (min r − 1, c − 1) = the minimum value of r − 1 (number of rows minus and c − 1 (number of columns minus 1) 265 In words: To calculate V, find the lesser of the number of rows minus 1 (r − 1) and the number of columns minus 1 (c − 1) , multiply this value by n, divide the result into the value of chi square, and then find the square root. Cramer’s V has an upper limit of 1.00 for any size table and has the same value as phi if the table has either two rows or two columns. Like phi, Cramer’s V can be interpreted as an index that measures the strength of the association between two variables. To illustrate the computation of V, suppose you had gathered the data displayed in Table 8.12, which shows the relationship between membership in student clubs or organizations and academic achievement for a sample of university students. The obtained chi square for this table is 32.14, a value that is significant at the 0.05 level. Cramer’s V is χ2 V =√ n[min(r − 1), (c − 1)] 32.14 32.14 V =√ =√ 75(2) 150 V = √0.21 = 0.46 Because Table 8.12 has the same number of rows and columns, we may use either r − 1 or c − 1 in the denominator. In either case, the value of the denominator is n multiplied by 3 − 1 , or n multiplied by 2. Column percentages are presented in Table 8.13 to help identify the pattern of this relationship. Members of sports clubs tend to be moderate, members of non- sports clubs tend to be high, and non-members tend to be low in academic achievement. Table 8.12 Academic Achievement by Student-Club Membership (Fictitious Data) Table 8.13 Academic Achievement by Student-Club Membership (Percentages) Book Title: eTextbook: Statistics: A Tool for Social Research and Data Analysis, Fifth Canadian Edition 8.6. Chi Square–Based Measures of Association Interpreting Phi and Cramer’s V Interpreting Phi and Cramer’s V It is helpful to have some general guidelines for interpreting the value of measures of association for nominal variables, similar to the guidelines we 266 used to interpret the maximum difference in column percentages. Table 8.14 presents the general relationship between the value of the statistic and the strength of the relationship for phi and Cramer’s V. As was the case with Table 8.5, the relationships in Table 8.14 are arbitrary and meant as general guidelines only. Using these guidelines, we can characterize the relationship in Table 8.10 (ϕ = 0.33) and Table 8.12 (Cramer’s V = 0.46 ) as strong. Table 8.14 The Relationship between the Value of Nominal- Level Measures of Association and the Strength of the Relationship Value Strength If the value is The strength of the relationship is between 0.00 and 0.10 weak between 0.11 and 0.30 moderate greater than 0.30 strong Book Title: eTextbook: Statistics: A Tool for Social Research and Data Analysis, Fifth Canadian Edition 8.6. Chi Square–Based Measures of Association Limitations of Phi and Cramer’s V Limitations of Phi and Cramer’s V One limitation of phi and Cramer’s V is that they are only general indicators of the strength of the relationship. Of course, the closer these measures are to 0.00, the weaker the relationship, and the closer they are to 1.00, the stronger the relationship. Values between 0.00 and 1.00 can be described as weak, moderate, or strong according to the general guidelines presented in Table 8.14 but have no direct or meaningful interpretation. On the other hand, phi and V are easy to calculate (once the value of chi square is obtained) and are commonly used indicators of the importance of an association. (For practice in 267 computing phi and Cramer’s V, see any of the problems at the end of this chapter. Problems with 2 × 2 tables minimize computations. Remember that for tables with either two rows or two columns, phi and Cramer’s V have the same value.) One Step at a Time Computing and Interpreting Phi and Cramer’s V To Calculate Phi, Solve Formula 8.1 1: Divide the value of chi square by n. 2: Find the square root of the quantity you found in step 1. The resulting value is phi. 3: Consult Table 8.14 to help interpret the value of phi. To Calculate Cramer’s V, Solve Formula 8.2 1: Find the number of rows (r) and the number of columns (c) in the table. Subtract 1 from the lesser of these two numbers to find min ( r − 1 , c − 1 ). 2: Multiply the value you found in step 1 by n. 3: Divide the value of chi square by the value you found in step 2. 4: Take the square root of the value you found in step 3. The resulting value is V. 5: Consult Table 8.14 to interpret the value of V. Book Title: eTextbook: Statistics: A Tool for Social Research and Data Analysis, Fifth Canadian Edition Chapter 8. Measures of Association for Variables Measured at the Nominal Level 8.7. Proportional Reduction in Error Measures of Association 8.7. Proportional Reduction in Error Measures of Association In recent years, measures based on a logic known as proportional reduction in error (PRE) have been developed to complement the older chi square–based measures of association. Unlike their chi square– based counterparts, PRE-based measures of association provide a direct, meaningful interpretation. Most generally stated, the logic of PRE measures requires us to make two different predictions about the scores of cases. In the first prediction, we ignore information about the independent variable and, therefore, make many errors in predicting the score on the dependent variable. In the second prediction, we take account of the score of the case on the independent variable to help predict the score on the dependent variable. If there is an association between the variables, we will make fewer errors when taking the independent variable into account. The value of a PRE-based measure of association is that it has a precise, meaningful interpretation in the sense that it quantitatively measures the PRE between the two predictions. Applying these general thoughts to the case of nominal-level variables will make the logic clearer. Book Title: eTextbook: Statistics: A Tool for Social Research and Data Analysis, Fifth Canadian Edition 8.8. Lambda: A PRE Measure of Association 8.8. Lambda: A PRE Measure of Association For nominal-level variables, we first predict the category into which each case will fall on the dependent variable (Y) while ignoring the independent variable (X). Because we would, in effect, be predicting blindly in this case, we would make many errors (i.e., we would often incorrectly predict the category of a case on the dependent variable). 268 The second prediction allows us to take the independent variable into account. If the two variables are associated, the additional information supplied by the independent variable will reduce our errors of prediction (i.e., we should misclassify fewer cases). The stronger the association between the variables, the greater the reduction in errors. In the case of a perfect association, we would make no errors at all when predicting the score on Y from the score on X. But when there is no association between the variables, knowledge of the independent variable will not improve the accuracy of our predictions. We would make just as many errors of prediction with knowledge of the independent variable as we would without knowledge of it. To illustrate these principles, suppose you were placed in the rather unusual position of having to predict whether each of the next 100 people you meet will be shorter or taller than 174 centimetres under the condition that you have no knowledge of these people at all. With absolutely no information about these people, your predictions will be wrong quite often (you will often misclassify a tall person as short, and vice versa). Now assume that you must go through this ordeal twice, but on the second round, you know the gender of the person whose height you must predict. Because height is associated with gender and females are, on average, shorter than males, the optimal strategy is to predict that all females are short and that all males are tall. Of course, you will still make errors on this second round, but if the variables are associated, the number of errors on the second round will be less than the number of errors on the first. That is, using information about the independent variable will reduce the number of errors (if, of course, the two variables are related). How can these unusual thoughts be translated into a useful statistic? Book Title: eTextbook: Statistics: A Tool for Social Research and Data Analysis, Fifth Canadian Edition 8.8. Lambda: A PRE Measure of Association Lambda Lambda One hundred individuals have been categorized by gender and height, and the data are displayed in Table 8.15. It is clear, even without percentages, that the two variables are associated. To measure the strength of this association, we calculate a PRE measure called lambda (λ). Following the logic introduced above, we must find two quantities. First, we find the number of prediction errors made while ignoring the independent variable (gender). Then, we find the number of prediction errors made while taking gender into account. We then compare these two sums to derive the statistic. 269 Table 8.15 Height by Gender First, the information given by the independent variable (gender) can be ignored, in effect, by working with only the row marginals. Two different predictions can be made about height (the dependent variable) by using these marginals. We can predict either that all subjects are tall or that all subjects are short. For the first prediction (all subjects are tall), we make 48 errors. That is, for this prediction, all 100 cases are placed in the first row. Because only 52 of the cases actually belong in this row, this prediction results in 100 − 52 , or 48, errors. If we predict that all subjects are short, on the other hand, we make 52 errors (100 − 48 = 52). We take the lesser of these two numbers and refer to this quantity as E1 for the number of errors made while ignoring the independent variable. So, E1 = 48. In the second step in the computation of lambda, we predict the score on Y (height) again, but this time we take X (gender) into account. To do this, we follow the same procedure as in the first step but this time move from column to column. Because each column is a category of X, we thus take X into account in making our predictions. For the left-hand column (males), we predict that all 50 cases are tall and make 6 errors (50 − 44 = 6). For the second column (females), our prediction is that all females are short, and we make 8 errors. By moving from column to column, we took X into account and made a total of 14 errors of prediction, a quantity we refer to as E2 (6 + 8 = 14). If the variables are associated, we will make fewer errors under the second procedure than under the first. In other words, E2 will be smaller than E1. In this case, we made fewer errors of prediction while taking gender into account (E2 = 14) than while ignoring gender (E1 = 48) , so gender and height are clearly associated. Our errors were reduced from 48 to only 14. To find the proportional reduction in error, use Formula 8.3: Formula 8.3 E1 − E2 λ= E1 where E1 = the total number of cases minus the largest row total E2 = the sum of the following: for each column, the column total minus the largest cell frequency For the sample problem, the value of lambda is E1 − E2 48 − 14 34 λ= = = = 0.71 E1 48 48 The value of lambda ranges from 0.00 to 1.00. Of course, a value of 0.00 means that the variables are not associated at all ( E1 is the same as E2 ), and a value of 1.00 means that the association is perfect ( E2 is zero, and scores on the dependent variable can be predicted without error from the independent 270 variable). Unlike phi or Cramer’s V, however, the numerical value of lambda between the extremes of 0.00 and 1.00 has a precise meaning: it is an index of the extent to which the independent variable (X) helps us predict (or, more loosely, understand) the dependent variable (Y). When multiplied by 100%, the value of lambda indicates the strength of the association in terms of the percentage reduction in error. Thus, the lambda above is interpreted by concluding that knowledge of gender improves our ability to predict height by 71%, or we are 71% better off knowing gender when attempting to predict height. Book Title: eTextbook: Statistics: A Tool for Social Research and Data Analysis, Fifth Canadian Edition 8.8. Lambda: A PRE Measure of Association Calculating Lambda: Another Example Calculating Lambda: Another Example In this section, we will work through another example, based on actual data, to state the computational routine for lambda in more general terms. To help reduce carbon emissions, the Canadian federal government passed the Greenhouse Gas Pollution Pricing Act in 2018 and implemented it in the following year. The Act put in place a national minimum for carbon pricing to help reduce greenhouse gas emissions to targets set by the Paris Agreement adopted in 2015. Public opinion on this carbon tax has been mixed. We will use data from the 2019 Canadian Election Study, shown in Table 8.16, to examine whether public opinion at that time varied by region. To make the number of cases in this example more manageable, yet still representative, we randomly selected about 5% of cases (or 597 respondents) from the full sample of about 12,000 respondents. 271 Table 8.16 Opinion Toward Carbon Tax by Region Source: Data from Canadian Election Study. 2019 Canadian Election Study. arindambanerjee/Shutterstock.com Public opinion on the federal carbon tax has been mixed. We can use data to further examine public opinion by region. Step 1 To find E1 , the number of errors made while ignoring X (region, in this case), subtract the largest row total from n. For Table 8.16, E1 is Thus, we will misclassify 332 cases on opinion about the carbon tax while ignoring region. Step 2 Next, we must find E2 , the number of errors made when taking the independent variable into account. For each column, subtract the largest cell frequency from the column total and then add the subtotals together. For the data presented in Table 8.16, we have the following: We make a total of 290 errors predicting opinion about the carbon tax while taking region into account. Step 3 In step 1, 332 errors of prediction were made as compared to 290 errors in step 2. Because the number of errors is reduced, the variables are associated. To find the PRE, we can substitute the values for E1 and E2 directly into Formula 8.3: 332 − 290 42 λ= = = 0.13 332 332 Using our conventional labels (see Table 8.14), we would call this a moderate relationship. Using PRE logic, we can add more detail to the characterization: when attempting to predict opinion about the carbon tax, we make 13% fewer errors by taking region into account. Knowledge of a respondent’s region of residence improves the accuracy of our predictions by a factor of 13%. The slightly moderate strength of lambda indicates that factors other than region are associated with the dependent variable for the carbon tax. 272 Applying Statistics 8.1. Measures of Association The most recent (2017) Aboriginal Peoples Survey collected data on a range of topics including participation in the Canadian economy, use of information technology, and Indigenous language attainment. One of the survey questions asked respondents, “How important is it to you that you speak and understand an Indigenous language?” The majority said that it is very or somewhat important (as opposed to not very important or not important) to speak and understand an Indigenous language. The table below displays the data for this variable by gender of the respondent. (To make the number of cases more manageable for computation, yet still representative, we randomly selected about 5% of the cases from the full sample.) Is the importance of speaking and understanding an Indigenous language related to gender? Because this is a 2 × 2 table, we can compute phi as a measure of association. The chi square for the table is 13.44, so phi is χ2 13.44 ϕ=√ =√ = 0.12 n 994 which indicates a weak-moderate relationship between gender and importance of speaking and understanding an Indigenous language. Lambda, on the other hand, reveals no relationship between the two variables: The problem here is a limitation of lambda. Lambda can produce a “false” zero when the categories of the independent variable (male and female) have the same dependent-variable modal category. Because the mode for both males and females falls in the “very or somewhat” category, lambda can be misleading and should be disregarded and phi used instead. Source: Data from Statistics Canada. Aboriginal Peoples Survey, 2017. Book Title: eTextbook: Statistics: A Tool for Social Research and Data Analysis, Fifth Canadian Edition 8.8. Lambda: A PRE Measure of Association Limitations of Lambda Limitations of Lambda As a measure of association, lambda has two characteristics that should be stressed. First, lambda is asymmetric. This means that the value of the statistic varies depending on which variable is taken as dependent. For example, in Table 8.16, the value of lambda is about 0.04 if region is taken as the dependent variable (verify this with your own computation). Thus, you should exercise some caution when designating a dependent variable. If you consistently follow the convention of arraying the independent variable in the columns and the dependent variable in the rows and compute lambda as outlined previously, the asymmetry of the statistic should not be confusing. Second, as illustrated in Applying Statistics 8.1, lambda can be misleading. It can be 0.00 even when other measures of association are greater than 0.00 and the conditional distributions for the table indicate that there is an association between the variables. This problem of a “false” zero is a function of the way 273 lambda is calculated—lambda is zero whenever the mode for each category of the independent variable lands in the same category of the dependent variable. Great caution should be exercised in the interpretation of lambda when the modes appear in the same category. In fact, in these situations, a chi square– based measure of association is preferred. (For practice in computing lambda, see any of the problems at the end of this chapter. As with phi and Cramer’s V, it’s probably a good idea to start with small samples and 2 × 2 tables.) One Step at a Time Computing and Interpreting Lambda To Calculate Lambda, Solve Formula 8.3 1: To find E1 , subtract the largest row subtotal (marginal) from n. 2: Starting with the far left column, subtract the largest cell frequency in the column from the column total. Repeat this step for all columns in the table. 3: Add up all the values you found in step 2. The result is E2. 4: Subtract E2 from E1. 5: Divide the quantity you found in step 4 by E1. The result is lambda. To Interpret Lambda 1: Multiply the value of lambda by 100%. This percentage tells us the extent to which our predictions of the dependent variable are improved by taking the independent variable into account. Also, lambda may be interpreted using the descriptive terms in Table 8.14. Book Title: eTextbook: Statistics: A Tool for Social Research and Data Analysis, Fifth Canadian Edition Chapter 8. Measures of Association for Variables Measured at the Nominal Level Summary Summary 1. Analyzing the association between variables provides information that is complementary to tests of significance. The latter are designed to detect non-random relationships, whereas measures of association are designed to quantify the importance or strength of a relationship. 2. Relationships between variables have three characteristics: the existence of an association, the strength of the association, and the direction or pattern of the association. These three characteristics can be investigated by calculating percentages for a bivariate table in the direction of the independent variable (vertically) and then comparing in the other direction (horizontally). This procedure can be summarized in the following statement: “Percentage Down, Compare Across.” It is often useful (as well as quick and easy) to assess the strength of a relationship by finding the maximum difference in column percentages in any row of the table. The panelled pie chart and clustered bar chart make the “Percentage Down, Compare Across” procedure graphically visible. 3. Tables 8.1 and 8.2 can be analyzed in terms of these three characteristics. Clearly, a relationship does exist between job satisfaction and productivity because the conditional distributions of the dependent variable (productivity) are different for the three different conditions of the independent variable (job satisfaction). Even without a measure of association, we can see that the association is substantial in that the change in Y (productivity) across the three categories of X (satisfaction) is marked. The maximum difference of 36.54% confirms that the relationship is substantial (strong). Furthermore, the relationship is positive in direction: productivity increases as job satisfaction rises, and workers who report high job satisfaction also tend to be high on 274 productivity. Workers with little job satisfaction tend to be low on productivity. 4. Given the nature and strength of the relationship, it could be predicted with fair accuracy that highly satisfied workers tend to be highly productive (“happy workers are busy workers”). These results might be taken as evidence of a causal relationship between these two variables, but they cannot, by themselves, prove that a causal relationship exists— association is not the same thing as causation. In fact, although we have presumed that job satisfaction is the independent variable, we could have argued the reverse causal sequence (“busy workers are happy workers”). The results presented in Tables 8.1 and 8.2 are consistent with both causal arguments. 5. Phi, Cramer’s V, and lambda are measures of association, and each is appropriate for a specific situation. We use phi for 2 × 2 tables and Cramer’s V for tables larger than 2 × 2. While phi and Cramer’s V are chi square–based measures, lambda is a PRE-based measure and provides a more direct interpretation for values between the extremes of 0.00 and 1.00. These statistics express information about the strength of the relationship only. In all cases, be sure to analyze the column percentages as well as the measures of association to maximize the information you have about the relationship. Summary of Formulas Phi χ 2 ϕ=√ n Cramer’s V χ2 V =√ n[min(r − 1), (c − 1)] Lambda λ= E1 − E2 E1 Glossary Association Clustered bar charts Conditional distributions of Y Cramer’s V Dependent variable Independent variable Lambda (λ) Maximum difference Measures of association Negative association Panelled pie charts Phi (ϕ) Positive association Proportional reduction in error (PRE) X Y Multimedia Resources 275 Visit the companion website for the fifth Canadian edition of Statistics: A Tool for Social Research and Data Analysis to access a wide range of student resources: www.cengage.com/healey5ce. Problems 8.1 GER A survey of older adults who live in either a housing development specifically designed for retirees or an age-integrated neighbourhood is conducted. Calculate percentages for the table, and then describe the strength and pattern of the association between living arrangement and sense of social isolation. SHOW ANSWER 8.2 SOC The administration of a university has proposed an increase in the mandatory student fee in order to finance an upgrade of the varsity football program. A sample of the faculty has completed a survey on the issue. Is there any association between support for raising fees and the age, discipline, or tenure status of the faculty? Calculate percentages for each table, and then describe the strength and pattern of the association. a. Support for raising fees by age: b. Support for raising fees by discipline: c. Support for raising fees by tenure status: 8.3 PS How consistent are people in their voting habits? Do the same 276 people vote from election to election? Below are the hypothetical results of a poll in which people were asked if they had voted in each of the last two federal elections. Calculate percentages for the table, and assess the strength and pattern of this relationship. SHOW ANSWER 8.4 GER A needs assessment survey is distributed in a retirement community. Residents are asked to check off the services or programs they think should be added. Is there any association between age and the perception of a need for more social occasions? Calculate percentages for the table, and assess the strength and pattern of this relationship. 8.5 Compute a phi and a lambda for Problems 8.1, 8.2, 8.3, and 8.4. Compare the value of the measure of association with your impressions of the strength of the relationships based solely on the percentages you calculated for those problems. SHOW ANSWER 8.6 In any social science journal, find an article that includes a bivariate table. Inspect the table and the related text carefully, and answer the following questions: a. Identify the variables in the table. What values (categories) does each possess? What is the level of measurement for each variable? b. Is the table in percentage form? In what direction are the percentages calculated? Are comparisons made between columns or rows? c. Is one of the variables identified by the author as independent? d. How is the relationship characterized by the author in terms of the strength of the association? In terms of the direction (if any) of the association? e. Find the measure of association (if any) calculated for the table. What is the numerical value of the measure? What is the sign (if any) of the measure? 8.7 If a person’s political ideology (liberal, moderate, or conservative) is known, can we predict their position on issues? If liberals are generally progressive and conservatives are generally traditional (with moderates in between), what relationships would you expect to find between political ideology and these issues? a. support for same-sex marriage b. support for the death penalty c. support for the legal right to medical assistance in dying for people with incurable diseases d. support for traditional gender roles e. support for the legalization of illicit drugs The tables below show the results of a recent public opinion survey. For each table, compute column percentages and the maximum difference. Summarize the strength and direction of each relationship in a brief paragraph. Were your expectations confirmed? a. Support for same-sex marriage by political ideology: SHOW ANSWER b. Support for capital punishment by political ideology: 277 SHOW ANSWER c. Support for the right of people with an incurable disease to access medical assistance in dying by political ideology: SHOW ANSWER d. Support for traditional gender roles by political ideology: SHOW ANSWER e. Support for legalizing illicit drugs by political ideology: SHOW ANSWER 8.8 Problem 8.7 analyzed the bivariate relationships between political ideology, the independent variable, and five different dependent variables using only percentages. Now, with the aid of measures of association, these characterizations should be easier to develop. Compute a phi and a lambda for each table in Problem 8.7. Compare the measures of association with your characterizations based on the percentages. You Are the Researcher Using SPSS to Analyze Bivariate Association with the 2018 GSS The demonstrations and exercises below use the shortened version of the 2018 GSS data set supplied with this textbook. Start SPSS, and open the GSS_2018_Shortened.sav file. SPSS Demonstration 8.1 Does Health Vary by Income? What’s the relationship between income and health? To answer this question, we’ll run a Crosstabs on famincg2 (annual family income) and hm_01 (general health). We should first recode famincg2 because it has many (six) categories and thus it may be difficult to observe a relationship or patterns in a bivariate table. We will provide only a brief review here; a detailed guide to recoding, including adding labels to the values of the recoded variable, is given in Appendix F.5. Click Transform from the main menu, and choose Recode into Different Variables. Next, move the variable famincg2 to the Input Variable → Output Variable box, and then type a name—we suggest income4—in the Output Variable box. Click the Change button. Next, click on the Old and New Values button. We have decided to collapse the values of famincg2 into four categories. The recoding instructions that should appear in the Old → New dialog box are 1 thru 2 → 1 3 thru 4 → 2 5→3 6→4 278 Click Continue and then OK after inputting these recode instructions. We will also recode health status, hm_01. As a common rule, it is best to collapse a variable into logical groups. We decided to collapse hm_01 into a dichotomized variable: good health versus poor health. Click Transform and then Recode into Different Variables. Next, click the Reset button to reset all specifications in the dialog and sub-dialog boxes to their default state. Move the variable hm_01 to the Input Variable → Output Variable box. Give the recoded variable a new name in the Output Variable box (we used health), and then click the Change button. Click the Old and New Values button, and follow these recoding instructions: 1 thru 3 → 1 4 thru 5 → 2 Click Continue and then OK. Scores 1 (excellent), 2 (very good), or 3 (good) on hm_01 are grouped together into a score of 1 on health, and scores 4 (fair) or 5 (poor) on hm_01 are grouped into a score of 2 on health. We highly recommend that both new variables be added to the permanent data file because they will be used in future SPSS demonstrations and exercises. To do so, click Save from the File menu, and the updated data set with income4 and health added will be saved. To examine the effect of income4 on health, click Analyze, Descriptive Statistics, and Crosstabs, and then input health as the row variable and income4 as the column variable. Click the Cells button, and request column percentages by clicking the box next to Column in the Percentages box. With the dependent variable in the rows and the independent variable in the columns and with percentages calculated within columns, we can read the table by following the rules developed in this chapter. Click the Continue button to return to the Crosstabs dialog box. Also, request chi square by clicking the Statistics button. Click Continue and then OK, and the following output will be produced. (The output has been modified slightly, including adding labels to the values of income4 and health, as illustrated in Appendix F.5, to improve readability.) Inspecting the table column by column, you will see that there is a relationship and that it is weak in strength. The maximum difference is the same in both the top and bottom rows, and it occurs in each row between the highest and lowest income columns. In the top row, the maximum difference is 89.1% − 80.0% = 9.1%. In the bottom row, the maximum difference is 20.0% − 10.9% = 9.1%. 279 Both variables are measured at the ordinal level, so we can also describe the direction. Is this relationship positive or negative? Remember that in a positive association, high scores on one variable are associated with high scores on the other, and low scores are associated with low. In a negative relationship, high scores on one variable are associated with low scores on the other. Looking at the table again, we see that the relationship is negative: as the code value of income increases, the code value of health decreases. This is a good opportunity to draw attention to the fact that SPSS calculates relationship direction on the basis of the relationship between the variables’ response category code values. What does this mean, in our example? Remember how we recoded famincg2 and hm_01. For income4, the code value 1 represents the lowest income category (< $50,000), while the code value 4 represents the highest income category ($125,000+). An increasing income code value corresponds to an increasing income category amount. The opposite is true for health, where 1 represents good health and 2 represents poor health. In other words, an increasing health code value corresponds to declining health. So a negative relationship in our example means that as the income code value increases, the health code value decreases. Looking at the meaning of our codes, what this shows us is that as the amount of income increases, people’s health gets better, while as income decreases, people’s health becomes poorer. We can provide a visual representation of the conditional distributions of Y in the above cross-tabulation by a creating a panelled pie chart and a clustered bar chart. To create the panelled pie chart, click on Graphs, select Legacy Dialogs, and then select Pie. Keep Data in Chart Are Summaries for groups of cases, and click Define. At the top of the drop-down menu, click on Slices Represent N of cases. Next, click on health in the left variable name column, and move this dependent variable into the Define slices by box. Similarly, click on income4 in the left variable column, but now move this independent variable into the Panel by columns box. When you click OK, the panelled pie chart appears in the output window. Next, double-click anywhere on the panelled pie chart to open Chart Editor, and then click on the Show Data Labels icon in the toolbar menu. When you 280 do this, the Properties window opens and the raw frequency counts appear in each slice; however, we want the percentages to appear, so that we can see the direct connection between our chart and the conditional distributions of Y. So, scroll across the top of the Properties window until you arrive at Data Value Labels. Count shows up in the Labels Displayed box. Click on Count, and then click on the small red “x” (located to the right of the Labels Displayed box). This will remove the frequency count from the pie slices and transfer it to the Not Displayed box. Next, click on Percent and then on the small green arrow (located to the right of the Not Displayed box). This will move the percentage to the Labels Displayed box. Click on Apply, and then on Close, to close the box. The raw frequency counts will now change to percentages in the panelled pie chart in the output window as follows (slightly edited for readability): Pie Chart Showing the Impact of Income on Health Next, to create the clustered bar chart, click on Graphs, select Legacy Dialogs, and then select Bar. Click on Clustered, keep Data in Chart Are Summaries for groups of cases, and then click Define. At the top of the drop-down menu, click on Bars Represent % of cases. Next, click on health in the left variable name column, and move this dependent variable into the Category Axis box. Similarly, click on income4 in the left variable column, but now move this independent variable into the Define Clusters By box. When you click OK, the clustered bar chart appears in the output window. Then, double-click on the clustered bar chart to open Chart Editor, and then click on the Show Data Labels icon. When you do this, the percentages will automatically appear in each bar, and the Properties window will open. Since we want the percentages, in order to see the direct connection between our chart and the conditional distributions of Y, we do not need to make any further changes. Simply close the Properties window, as well as the Chart Editor editing window. The percentages will now appear in the clustered bar chart in the output window as follows (slightly edited for readability): 281 Bar Graph Showing the Impact of Income on Health The panelled pie chart and the clustered bar chart represent the conditional distributions of Y directly, so they can help us interpret our cross-tabulation. In the panelled pie chart, we see four pies. Each pie represents 100% of the cases in one of the income categories, while the slices in each pie show how the health categories are distributed within that pie. So, for example, we can see how the “poor health” slice is largest for the “< $50,000” income category, but this slice becomes smaller as the income level increases; conversely, the size of the “good health” slice increases as income increases. Comparing the sizes of the independent-variable slices for each dependent-variable pie is equivalent to comparing column percentages across in a cross-tabulation in order to identify variable relationships. At the same time, in every pie, we see that the largest pie slice always comprises people who said that their health is good. In other words, most people, across all income categories, have good health; however, we are more likely to find people with poor health among people with low income than we are to find them among people with high income. Turning to the clustered bar chart, the bars represent the income categories, such that each of the bars of a single colour represents 100% of the cases in that income category. Comparing the heights of the bars, for each dependent- variable value on the x-axis, is equivalent to comparing column percentages 282 across in a cross-tabulation in order to identify variable relationships. So, for example, among people with good health, we can see that the bar representing people with incomes of $125,000 and over is the tallest, while the bar representing people with incomes of < $50,000 is the shortest; this pattern is reversed for people with poor health. At the same time, the greater height of all the bars in the “good health” category, compared with all the bars in the “poor health” category, shows us that across all income categories, most people said that their health is good. Finally, the exact probability value of chi square is 0.003, below the standard alpha indicator of a significant result, 0.05, so we reject the null hypothesis that the variables are independent and conclude that there is a statistically significant relationship between income and health. However, this is a situation where we have a statistically significant finding but not necessarily an important one (recall that the maximum percentage point difference was only 9.1%). As noted in Chapter 7, a statistically significant finding does not guarantee that it is important in any other sense, especially if the sample size is large, as in our case. SPSS Demonstration 8.2 Does Volunteer Behaviour Vary by Gender? Another Look In Demonstration 7.1, we used the Crosstabs procedure to examine the relationship between fvisvolc (volunteered in the past 12 months) and gndr (respondent’s gender). We saw that the relationship was statistically significant, and that females were more likely than males to have volunteered in the past 12 months. In this demonstration, we will re-examine the relationship and have SPSS compute some measures of association. Click Analyze, then Descriptive Statistics, and then Crosstabs. Move fvisvolc into the Row(s) box and gndr into the Column(s) box. Click the Cells button, and request column percentages. Click the Statistics button, and request chi square, phi, Cramer’s V, and lambda. Click Continue, and then OK, and the following output, slightly edited to improve readability, will be produced. 283 The measures of association are reported after the crosstab and chi square tables. Three values for lambda are reported in the “Directional Measures” output block. Remember that lambda is asymmetric and changes value depending on which variable is taken as dependent. (Symmetric lambda is more or less an average of the two asymmetric lambda values and should only be used when it is not possible to determine which variable is dependent and which is independent.) In this case, fvisvolc (volunteered in the past 12 months) is the dependent variable, so lambda is 0.000, a value that indicates no relationship between the variables. Looking at the first block in the output “Cross-tabulation,” we see, however, that the conditional distributions do 284 change, indicating that there actually is a relationship. This is a problem of a “false” zero (see Section 8.8), and lambda should be disregarded. Note that the Goodman and Kruskal tau is similar to lambda and is based on the logic of PRE. It is also an asymmetric measure of association. Phi and Cramer’s V are reported in the “Symmetric Measures” output block. The statistics are identical in value, 0.097, as they will be whenever the table has either two rows or two columns. (We can ignore the directional sign of phi.) The measures reveal an association between the variables, albeit a weak one. The significance value of chi square, 0.000 (or more precisely, < 0.001), reported in the “Chi-Square Tests” output block, is lower than 0.05, so we reject the null hypothesis and conclude that the relationship between gender and volunteering behaviour is statistically significant. Even though phi and Cramer’s V tell us that the relationship between gender and volunteering behaviour is rather weak, chi square indicates that it is statistically significant. This reminds us once again that association and statistical significance are two different things. Exercises (using GSS_2018_Shortened.sav) 8.1 As long as hm_01 has already been recoded, examine the relationship between recoded hm_01 as the dependent or row variable and dh1ged (education) as the independent or column variable using the Crosstabs procedure. Be sure to request column percentages in the cells and the chi square test. To assist your interpretation of the column percentages in the cross-tabulation, also produce a panelled pie chart and a clustered bar chart. Write a paragraph summarizing the results. Describe the relationships in terms of strength and pattern. 8.2 Following up on Demonstration 8.2, select two more variables that you think might be related to fvisvolc. Run the Crosstabs procedure with fvisvolc as the dependent or row variable and your other variables as the independent or column variables. Be sure to request column percentages in the cells, as well as phi and lambda. Write a few sentences describing each relationship. 285 Book Title: eTextbook: Statistics: A Tool for Social Research and Data Analysis, Fifth Canadian Edition Chapter 8. Measures of Association for Variables Measured at the Nominal Level Summary of Formulas Summary of Formulas Phi χ 2 ϕ=√ n Cramer’s V χ2 V =√ n[min(r − 1), (c − 1)] Lambda λ= E1 − E2 E1 Book Title: eTextbook: Statistics: A Tool for Social Research and Data Analysis, Fifth Canadian Edition Chapter 9. Measures of Association for Variables Measured at the Ordinal Level Chapter 9. Measures of Association for Variables Measured at the Ordinal Level Learning Objectives By the end of this chapter, you will be able to 1. Calculate and interpret gamma, Kendall’s tau-b and tau-c, Somers’ d, and Spearman’s rho 2. Explain the logic of pairs as it relates to measuring association 3. Use gamma, Kendall’s tau-b and tau-c, Somers’ d, and Spearman’s rho to analyze and describe a bivariate relationship in terms of the three questions introduced in Chapter 8 4. Test gamma and Spearman’s rho for significance Book Title: eTextbook: Statistics: A Tool for Social Research and Data Analysis, Fifth Canadian Edition Chapter 9. Measures of Association for Variables Measured at the Ordinal Level 9.1. Introduction 9.1. Introduction 286 There are two common types of ordinal-level variables. Some have many possible scores, and they look, at least at first glance, like interval-ratio-level variables. We will call these continuous ordinal variables. An attitude scale that incorporates many different items and, therefore, has many possible values produces this type of variable. The second type, which we call a collapsed ordinal variable, has only a few (no more than five or six) values or scores and can be created either by collecting data in collapsed form or by collapsing a continuous ordinal scale. For example, we could produce collapsed ordinal variables by measuring social class as upper, middle, or lower or by reducing the scores on an attitude scale to just a few categories (such as high, moderate, and low). A number of measures of association have been developed for use with collapsed ordinal-level variables. The most commonly used statistics in this group include gamma (G), Somers’ d ( dyx ), Kendall’s tau-b ( τb ), and a variant of Kendall’s tau-b called Kendall’s tau-c ( τc ). These statistics are covered in the first part of this chapter. For continuous ordinal variables, a statistic called Spearman’s rho ( rs ) is typically used. We will cover this measure of association toward the end of the chapter. This chapter will expand your understanding of how bivariate associations can be described and analyzed, but it is important to remember that we are still trying to answer the three questions raised in Chapter 8: Are the variables associated? How strong is the association? What is the direction of the association? Book Title: eTextbook: Statistics: A Tool for Social Research and Data Analysis, Fifth Canadian Edition Chapter 9. Measures of Association for Variables Measured at the Ordinal Level 9.2. The Logic of Pairs 9.2. The Logic of Pairs Gamma, Kendall’s tau-b and tau-c, and Somers’ d are conceptually similar to one another. They each measure the strength and direction of association by comparing each respondent to every other respondent, called a pair of respondents or more simply a pair, in terms of their rankings on the independent and dependent variable. The total number of unique pairs of respondents in a data set can be found by the following formula: Formula 9.1 n(n − 1) Total number of unique pairs of respondents = 2 where n = the total number of respondents Pairs can be further divided into five subgroups. A pair of respondents is similar if the respondent with the larger value on the independent variable also has the larger value on the dependent variable. A pair is dissimilar if the respondent with the larger value on the independent variable has the smaller value on the dependent variable. A pair is tied if respondents have the same value on either the independent or dependent variable, or on both. Specifically, a pair is tied on the independent variable if both respondents have the same 287 score on the independent but not the dependent variable, a pair is tied on the dependent variable if both respondents have the same score on the dependent but not the independent variable, and a pair is tied on both variables if both respondents have the same independent and dependent variable scores. As an example, let’s assume that a researcher is concerned about the causes of “burnout” (i.e., demoralization and loss of commitment) among elementary school teachers and wonders about the relationship between years of service (independent or X variable) and level of burnout (dependent or Y variable). To examine this relationship, five elementary school teachers were sampled and asked about number of years employed as a teacher (1 = low, 2 = moderate, or 3 = high) and level of teacher burnout (1 = low, 2 = moderate, or 3 = high). Their scores are reported in Table 9.1. Table 9.1 Data on Length of Service and Burnout for Five Teachers Teacher Length (X) Burnout (Y) Amira 1 1 Camil 2 2 Joseph 2 3 Karina 3 3 Steven 3 3 For length of service: 1 = low , 2 = moderate , 3 = high. For burnout: 1 = low , 2 = moderate , 3 = high. Using Formula 9.1, we find that there are 10 unique pairs among the five teachers: 5(5 − 1) 20 = = 10 2 2 Table 9.2 lists each of the 10 pairs by type of pair. So, for example, looking at Camil and Steven in Table 9.1, we see that Steven is ranked above Camil on 288 length of service. Steven is also ranked above Camil on burnout. Camil–Steven is therefore a similar pair. On the other hand, Joseph–Steven is a tied pair on the dependent variable, since they each have the same score on burnout but not on length of service, while Camil–Joseph is a tied pair on the independent variable since they each have the same score on length of service but not on burnout. We also see that Karina–Steven is a tied pair on both variables, because both respondents have the same independent and dependent variable scores. Table 9.2 Pairs of Teachers by Type of Pair Pair Type of Pair Amira–Camil Similar Amira–Joseph Similar Amira–Karina Similar Amira–Steven Similar Camil–Joseph Tied on the independent variable Camil–Karina Similar Camil–Steven Similar Joseph–Karina Tied on the dependent variable Joseph–Steven Tied on the dependent variable Karina–Steven Tied on both variables An example of a dissimilar pair is a teacher who is ranked above another teacher on length of service, but below that teacher on burnout. (As you can see, however, this table does not include any dissimilar pairs.) For example, if the teacher Sidney were included in Table 9.1, and they had a high length of service (score of 3) but a low level of burnout (score of 1), then Joseph–Sidney and Camil–Sidney would both be examples of dissimilar pairs. Book Title: eTextbook: Statistics: A Tool for Social Research and Data Analysis, Fifth Canadian Edition 9.3. Analyzing Relationships with Gamma, Kendall’s tau-b and tau-c, and Somers’ d 9.3. Analyzing Relationships with Gamma, Kendall’s tau-b and tau-c, and Somers’ d Determining the Strength of Relationships Gamma, Kendall’s tau-b and tau-c, and Somers’ d measure the strength of association between variables by considering the number of similar versus dissimilar pairs. When there is an equal number of similar and dissimilar pairs, the value of these statistics is equal to 0.00, indicating no association between the independent and dependent variable. As the number of similar relative to dissimilar or dissimilar relative to similar pairs increases, the value moves closer to 1.00. Thus, the larger the difference between the number of similar and dissimilar pairs, the stronger the association. When all pairs are either similar or dissimilar, the value of these statistics is equal to 1.00, indicating a perfect relationship. Table 9.3 provides some additional assistance with interpreting the values of gamma, Kendall’s tau-b and tau-c, and Somers’ d in a format similar to Tables 8.5 and 8.14. As with the latter tables, the relationship between the values and 289 the descriptive terms is arbitrary, so the outline presented in Table 9.3 is intended as a general guideline only. Table 9.3 The Relationship between the Value of Ordinal- Level Measures of Association and the Strength of the Relationship Value Strength If the value is The strength of the relationship is between 0.00 and 0.10 weak between 0.11 and 0.30 moderate greater than 0.30 strong Book Title: eTextbook: Statistics: A Tool for Social Research and Data Analysis, Fifth Canadian Edition 9.3. Analyzing Relationships with Gamma, Kendall’s tau-b and tau-c, and Somers’ d Gamma Gamma Gamma, Kendall’s tau-b, and Somers’ d are computed by subtracting the number of similar pairs from the number of dissimilar pairs and then dividing this result by the total number of pairs. Where these statistics differ is in how they treat tied pairs. (In other words, the value of all these statistics is identical when there are no tied pairs.) Gamma is the number of similar over dissimilar pairs as a proportion of all pairs excluding ties. The formula for gamma is 291 Formula 9.2 ns − nd G= ns + nd where ns = number of pairs of respondents ranked the same on both variables (i.e., similar pairs) nd = number of pairs of respondents ranked differently on both variables (i.e., dissimilar pairs) Gamma ranges from −1.00 for a negative relationship to +1.00 for a positive relationship. A value of zero indicates no relationship. Gamma is a symmetrical measure of association, so its value is the same regardless of which variable is taken as dependent. To illustrate the computation of G, let’s consider the relationship between levels of burnout and years of service for the five elementary school teachers in Table 9.1. To compute gamma, we must find the number of similar pairs, ns , and dissimilar pairs, nd. Turning to Table 9.2, we see that there are a total of six similar pairs and zero dissimilar pairs. Next, we substitute these numbers into the numerator and denominator of the formula: ns − nd 6−0 6 G= = = = +1.00 ns + nd 6+0 6 Thus, ignoring ties, there is perfect agreement between the teachers: A teacher with a higher length of service also has a higher level of burnout. That is, there is a perfect positive association between the variables. Gamma as a PRE Measure In addition to its interpretation of strength and direction of association, gamma