HS2024 Lecture 3 - Learning to Know PDF
Document Details
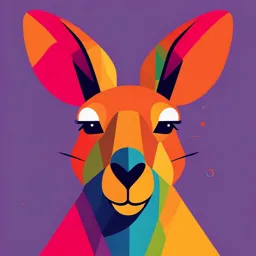
Uploaded by FreshFlerovium14
ETH Zurich
2024
Margarita Boenig-Liptsin
Tags
Related
- HS2024 AI and Human Values Lecture 1 PDF
- HS2024 Lecture 4 - Knowing Humans, Selves PDF
- HS2024 Lecture 5 - Dreams and Nightmares of Human-Computer Interaction PDF
- Lesson 6-Intelligent Environments PDF
- Artificial Intelligence & Human Values Lecture Notes PDF
- Lesson 11: Quest for Social Justice - AI & Human Values Fall 2024, ETH Zurich PDF
Summary
This ETH Zurich lecture covers artificial intelligence and human values, focusing on the use of generative AI in assignments and its implications for learning and knowledge-making. The lecture also discusses relevant contemporary issues and critical thinking in the AI era.
Full Transcript
Artificial Intelligence & Human Values Fall 2024, ETH Zürich Prof. Margarita Boenig-Liptsin Lecture 3: Learning to Know Use of Generative AI in AI&HV Assignments We have found instances of generated text—don't do it! Presenting generated content as one's own work can con...
Artificial Intelligence & Human Values Fall 2024, ETH Zürich Prof. Margarita Boenig-Liptsin Lecture 3: Learning to Know Use of Generative AI in AI&HV Assignments We have found instances of generated text—don't do it! Presenting generated content as one's own work can constitute plagiarism, is counter-productive to your learning in this class, and wasteful of instructors' time. Our course policy: If we suspect generated text, we will check, notify you and you will receive a 0 on that assignment. If you use translation/grammar correction tools, declare it. See ETH guidelines on use of Generative AI in scientific work. October events With Ruha Benjamin, Oct 29 UZH (Rämistrasse 71) 16:15 With Nicholas Christakis, Effy Vayena, Chris Luebkeman, With Sir Nigel Shadbolt, Oct 16 15:00 AudiMax M. Boenig-Liptsin, Oct 23 13:00 AudiMax From last week How do people make sense of Generative AI? Interpretive frames "assign meaning to and interpret relevant events and conditions" - Steve Epstein, Impure Science, 1996. Ways of framing the Political Economy Property issue Human & Machine Truth & Power Environment From last week Truth & Power Frame Prompt: "Historical footage of California during the gold rush." – Sora, Video accessed February 27, 2024 Context of fairness and data (since c. 2013) and concern about Who says what is? influence and manipulation (since How do they know? Cambridge Analytica controversy, What can they do with it? 2018, reaching back to 2016..and at least end of 19th c!) Where We Are AIxKnowledges Unit Reading for this week: Sheila Jasanoff, "The Power of Technology," Ethics of Invention, 2016. Optional: Donna Haraway, “Situated Knowledges: The Science Question in Feminism and the Privilege of Partial Perspective," 1988. Questions for "Learning to Know" lecture What are the contemporary issues in AI’s relationship to research and knowledge-making? How does the role of computing and AI in knowledge-making relate to debates about what is a good education? What is particularly important about critical thinking in the era of AI? Sphinx: "Which creature has one voice and yet becomes four-footed and two-footed and three-footed?" Why ask questions about "the human" at ETH? Oedipus: "Man" s l y ! s er iou to ols Take Detail from cover of A. Leroi-Gourhan La Geste et la Parole, 1964. Image: Espaces Libres. Detail of James Joyce monument in Dublin. Street art, Bologna, Italy. Image: Ashplant. Image: MBL 2023. s l y ! ri ou l s s e "What skills do we need? to o "How do we adapt?" e ak kind of a tool is this?" T"What "How do we preserve what we value?" "How can and should we accommodate it?" ETH communication about ChatGPT. March 2023. Technology Myths Exercise (also linked in Moodle) (5 min) Individually review the Jasanoff reading. ○ (Everyone) Setting up the myths, "Against Common Wisdom," p. 13 ○ "Technological Determinism" p. 14-19 ○ "Technocracy" p. 19-21 ○ "Unintended consequences" p. 21-26 (10 min) Discuss in your small group and write down for your myth: Definition; Examples; What's at stake. Use the text and your experience. (5 min) Add your content to shared doc. Class discussion. AI in learning and knowledge-making brings into focus: 1. Practices of how we think (and learn) → individual 2. Student-teacher relationships → interpersonal 3. Dominant ways of knowing → collective Thinking with computational tools— a pivotal moment and a long term development "The main aims are 1) to let computers facilitate formulative thinking as they now facilitate the solution of formulated problems" Licklider 1960 Patrick Suppes with a girl Children with LOGO Turtle. working on computer Image: Papert, 1980. (drill-and-practice), 1960s. Sparrow et al., Science, 2011 1. Practices of how we think (and learn) Mind the gap: What is the AI tool telling me in relation to what I might expect? What looks different? Why? 2. Student-teacher relationships Learning relationships: How does the tool re-configure the student-teacher relationship? What should this relationship ideally be like for "good learning" in a specific course; discipline? AI in learning and knowledge-making brings into focus 1. Practices of how we think (and learn) 2. Student-teacher relationships 3. Dominant ways of knowing Microsoft Research, 2009. "Back" to the age of Computation? Prof. Torsten Hoefler at the Inauguration of "Alps" Research Symposium, September 13, 2024 "The End of Theory" "The more we learn about biology, the further we find ourselves from a model that can explain it. [...] We can throw the numbers into the biggest computing clusters the world has ever seen and let statistical algorithms find patterns where science [i.e. models/theories] cannot" Anderson, Wired, 2008 A “new empiricism” Full resolution, granularity without sampling (“n=all”) Induction over deduction Beyond hypothesis testing Instrumentalist - Prediction and correlation over (causal) explanation and understanding “Data speak for themselves”; ML/AI find new patterns undetectable to human sight Critique of "end of theory" data always selective data always theory-laden (classification) Why was the data collected? With what instruments? How was it labelled and organized? The meaning of data, models, and inferences are always situated, always involve interpretation and domain knowledge Big Data, AI and conditions of knowledge at-large “Big Data” as sociotechnical fact (high volume,variety, velocity) as imaginary and “world schema” (“dataism”) Context or situation of data: world as laboratory How is data valued in different contexts? Political economy of data Balance shifts from academic to corporate context The Economist, February 2010 "insist on a better account of the world," p. 579 What's needed: "knowledge potent for constructing worlds less organized by axes of domination", p. 585 Haraway, Situated Knowledges, 1988 Situated AI? Domain-specific AI Reproducibility Transparency "We also don't want to theorize the world, much less act within it, in terms of Global Systems, but we do need an earth-wide network of connections, including the ability partially to translate knowledges among very different–and power-differentiated–communities" – Haraway, Situated Knowledges, p.580 Nature, September 14, 2023 3. Dominant ways of knowing What ways of knowing does AI depend upon? How are these integrated within a given discipline? What are the affordances and limits of ways of knowing with AI (for that discipline)? How can these be made visible and counter-balanced? Key Takeaways People learn to think and know with tools, in contexts of learning-relationships, and broader dominant ways of knowing. We need to take tools seriously and inquire into the specific affordances and limits of them for how we know. One aspect of taking tools seriously is being able to spot some common "myths" about technology in society: technological determinism, technocracy, and unintended consequences. As Jasanoff writes, "Each idea offers useful pointers for thinking about how to govern technology well, but each is limited and ultimately misleading. Most dangerously, each represents technology as politically neutral and outside the scope of democratic oversight" (Jasanoff, "The Power of Technology," p. 13). The introduction of AI in research and learning opens up many questions that pertain to what is knowledge: What does it mean to know? Who can say what is and what is knowledge? ○ It is also an invitation to examine the assumptions about existing practices (of thinking, of learning-relationships, and of ways of knowing) Key Takeaways II The AI way of knowing is dependent upon the existence and curation of large data sets, as well as the widespread imaginary that data are natural and necessary for knowledge and action. These assumptions are complex and contested, they privilege certain ways of knowing at the expense of others, and they tend to a form of "universal" knowledge that historically has been shown to be socially problematic. Considering and striving to be explicit about the "situatedness" of AI knowledge is a helpful corrective. Next Week AIxKnowledges Unit Reading for next week: Ursula Le Guin, "The Carrier Bag Theory of Fiction" (1996) Joanna Radin, "'Digital Natives': How Medical and Indigenous Histories Matter for Big Data" (2017)