HE201 Research Methods in Health Sciences PDF
Document Details
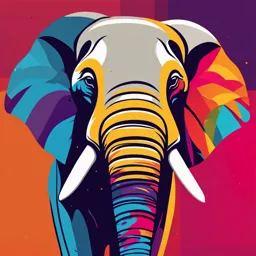
Uploaded by BenevolentAntigorite9283
Wilfrid Laurier University
Nicolas Rouleau
Tags
Related
- Statistics in Health Sciences PDF
- ANM150 AY2324 Week 6 Research 8 Statistics (1) PDF
- HE2801A Week 3 Measurement, Reliability, and Validity (Research Methods in Health Sciences) PDF
- Orientation to Health Research PDF
- HE 201 Research Methods Past Paper October 2024 (PDF)
- Health Research Methodology PDF
Summary
This document provides a lecture outline for HE201: Research Methods in Health Sciences, focusing on topics like measurement, reliability, validity, and descriptive statistics. The material covers different types of measurements and analysis methods. It's geared towards an undergraduate level health sciences course.
Full Transcript
HE201: Research Methods in the Health Sciences Measurement, Bias, Reliability, Validity, Descriptive Statistics Copyright © 2024 by Dr. Nicolas Rouleau, Ph.D., Waterloo, Ontario What is measurement? → Measurement is the quantification of objects or events → A measurable...
HE201: Research Methods in the Health Sciences Measurement, Bias, Reliability, Validity, Descriptive Statistics Copyright © 2024 by Dr. Nicolas Rouleau, Ph.D., Waterloo, Ontario What is measurement? → Measurement is the quantification of objects or events → A measurable phenomenon can be/is: 1) Pointed to 2) Enumerated 3) Subject to inter-observer agreement → All measurements affect the signal they are measuring → What are data? Reliability and validity unreliable Reliability in assessment → Reliability is consistency of measurement → There are several types of reliability → A person’s score on a test should be repeatable, consistent with similar measures, consistent across testers → How do you know if the test is changing or the person is changing? reliable Inter-rater reliability → The degree to which two independent observers agree; how congruent their measures are → Examples: 1) If two clinicians can assess the same patient and make the same diagnosis, their method is reliable 2) If two TAs can follow the same rubric and assign the same grades to a student, the method is reliable Test-retest reliability → If the same person is observed twice using the same assessment tool, the result should be the same → This assumption excludes the possibility that the person changed – which is also possible Alternate-form reliability → Rather than using the same assessment tool twice, clinicians can use alternate forms to test reliability → If there is congruence across forms that are rated to test the same feature (e.g., mood, anxiety, etc.), then it is reliable → This is especially useful if the clinician suspects the person can remember their answers and simply reproduce their results from memory Internal consistency reliability → The interrelatedness of items on a questionnaire should be highly correlated if they are all testing the same basic feature (e.g., anxiety) → If the correlation is too high, the test can be reduced to one question; this is redundancy Validity in assessment → Validity is the extent to which a tool is measuring the feature it is purported to measure → Validity may be superficial, pragmatic, or veridical → If a tool is not valid, no conclusion can be drawn from the results valid invalid Face validity → The degree to which a test is subjectively viewed as measuring what it purports to measure → It “looks like” an X test, superficially → It is the least important form of validity when determining what is true → It might be the most important form of validity for the person being tested Content validity → The extent to which a measure adequately samples the domain of interest → If you are assessing stress and you only ask questions about a person’s workplace situation, you will miss the phenomenon → You need to cast a wider net → Content should be comprehensive Criterion validity → Whether a new measure is associated in an expected way with some other established measure (the criterion) → If you know X and Y correlate, your test that you claim tests X should correlate with results from a test that measures Y → Depression and distorted thoughts should correlate → IQ predicts school performance and both predict lifetime earnings Predictive validity → Does the measure predict the phenomenon? → Regardless of face validity, measures can be powerful predictors → E.g. ice cream sales predict murders → E.g. red sky at night, sailor’s delight → E.g. a chromosomal test would powerfully predict likelihood of committing violent crime Construct validity → A construct is an inferred attribute or characteristic (e.g., intelligence, personality) → Construct validity is a measure of how accurately the inferred attribute is being measured → Are you actually measuring anxiety, or are you just the amount of times someone blinks? → Direct vs indirect measures matter What is a variable? → A variable is not a constant → Variables are values with many possible states that can be measured → An independent variable is manipulated → A dependent variable is observed Continuous vs. Discrete variables → Continuous variables are numeric variables that can take on any value within a range → Discrete variables are numeric variables that can only take on quantized unit that is definite Ratio variables → Measures on a scale → Has a meaningful 0 value (true zero) → Examples: height, weight, blood pressure Interval variables → Measures on a scale → Does not have a meaningful 0 value → Example: temperature in C or F (0 =/= absence of heat) Ordinal variables → Rank order variable → Numbers between ordered values are not meaningful → Only the order is meaningful → Example: education level, pain scales Nominal variables → Nominal or “categorical” variables do not hold rank or order → Example: employment status, blood type, sex, marital status, colour of hair Measures of central tendency → Descriptive statistics allow scientists to characterize datasets → Central tendency is a typical value for a probability distribution → Means are averages → Medians are middle numbers → Modes are the most frequent numbers What is the mean, median, and mode? 25 30 30 40 50 62 Measures of dispersion → Dispersion or variability can also be described for any given dataset or distribution → Also known as “scatter” or “spread” → Standard deviation describes variation about the mean → How many variability is in the sample or population? → Other measures: coefficient of variation, SEM Range and Quartiles Minimum (lowest value of the data) Maximum (highest value of the data) Range (lowest and highest value) Quartiles (dividing data into quarters) Deciles (dividing data into 10) IQR (interquartile range) What is the maximum, minimum, and range? 25 30 30 40 50 62 Displaying distributions → Distributions of data can be displayed in several ways: a) Histograms b) Boxplots c) Bar charts d) Pie charts Histograms Bar charts and pie charts Boxplots The normal distribution (of data) Other distributions (of data) “Skewness” and “Kurtosis” Descriptive statistics → Descriptive statistics allow scientists to characterize their datasets → How do you report the kinds of data that you have collected? → Give measures of central tendency, distribution type, measures of variability, etc. → E.g., ratio/interval data reported as means and SDs → E.g., categorical data reported as % of participants Statistical honesty Researchers are obligated to describe their data accurately and to correctly report the results of statistical tests Three of the most serious forms of research misconduct are: Fabrication: the creation of fake data Falsification: the misrepresentation of results Plagiarism: the use of other people’s ideas, words, or images without proper attribution Statistical honesty requires adherence to accepted statistical practices. Typical measures in health sciences → Weight, height, waist circumference → Temperature, blood pressure, pulse, pH → Breath sounds, range of motion, mental state → EEG, EKG, spirometry of lung function → Genetic/bio-markers in blood, saliva, urine → Imaging results from CT, MRI, PET, fNIRS, etc. → Strength, endurance, flexibility → Pain scores Scans Biomarkers LDH Psychometrics Humoral model of health and disease → The humoral theory was the primary theory of health in the West for ~2000 years → Humors, which refer to bodily fluids (blood, phlegm, yellow bile, and black bile), were thought to underly all vital functions → Balanced humours promoted good health, imbalances caused disease → Temperament and personality could also be explained by humors → Relationships between the humors and elemental forces were drawn → The theory fell out of favour in the 17 th and 18th centuries as the germ theory was established Mutagenesis model of cancer Microenvironment model of cancer Cattell-Horn-Carrol (Factorial) model Bias in science → Bias is a property that erodes the accuracy of a measurement → How data are collected, analyzed, reported, interpreted, or communicated can be influenced by bias → Consider motivations, incentives, and goals → Bulwarks include blinding procedures, pre-registration, replication, etc.