HDO Exam 1 Study Guide PDF
Document Details
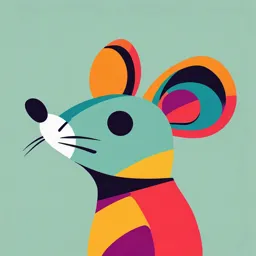
Uploaded by SpectacularWilliamsite4259
University of Texas Health
Tags
Summary
This document is a study guide for an HDO (likely Human Development and Organizations) exam. The guide covers various topics in research methods, including the scientific approach, different types of logic, data collection, and research design. Key concepts such as variables, and sampling are explained in detail.
Full Transcript
Week 1 Introduction to the Course What are some of the reasons why we do research (versus taking other approaches to understanding people-centered problems in organizations)? Research helps reduce bias and subjectivity. It provides systematic and replicable findings rather than relying on...
Week 1 Introduction to the Course What are some of the reasons why we do research (versus taking other approaches to understanding people-centered problems in organizations)? Research helps reduce bias and subjectivity. It provides systematic and replicable findings rather than relying on intuition, anecdotes, or assumptions. It allows organizations to identify patterns, make informed decisions, and develop strategies based on evidence. The Benefits of Research What makes an approach “scientific”? A scientific approach is systematic, empirical, and based on evidence. It follows a structured process of observation, experimentation, and analysis. Scientific Process: Four Major Features 1. Empirical Observation – Based on measurable evidence rather than personal beliefs. 2. Logical Reasoning – Uses inductive and deductive logic to form conclusions. 3. Skepticism – Findings must be tested and challenged. 4. Transparency & Replicability – Other researchers must be able to reproduce results. Theory and Data: Definition & Examples Theory: An interconnected set of propositions about how or why something occurs (story or abstract ideas about how the world works). Data: Information recorded from observation; may be in numerical or nonnumerical form (Observations of what is happening in your case) Hypothesis A testable statement predicting a relationship between variables. ○ Example: “Employees who receive more feedback from managers will have higher job satisfaction.” Data: What Does It Mean to Be Verifiable & Systematically Collected? Verifiable: must be observable to the researcher and others in the scientific community (this is also called “empirical evidence”) ○ Information must be tangible, observed, or sensed in some way: through sight, hearing, taste, smell, or touch Systematically Collected: scientific collection and analysis of verifiable data follows a typical process or series of steps ○ The point? The skeptics are more likely to believe your answers are correct ○ Reduces influence of personal values or bias in the research process ○ This course: devoted to learning the agreed-upon “series of steps” involved in different research methods Empirical Pattern A recurring trend found in data. ○ Example: Research shows that remote workers tend to have higher job satisfaction but lower team cohesion. Logical Reasoning: Inductive vs. Deductive Logic Inductive Logic: you start with specific observations and form general conclusions Example: Observing that many startups with flexible work hours succeed → concluding that flexible work boosts productivity. ○ Inductive logic of inquiry: you start with making observations in a setting (data), recognize patterns, and arrive at a more general conclusion that goes beyond the specifics of the case (theory) Data → patterns → theory Deductive Logic: Moves from general principles to specific predictions. you progress from general ideas to specific conclusions Example: Theory states that social interaction improves well-being → conducting a study to test if workplace collaboration improves employee satisfaction. ○ Deductive logic of inquiry: you start with a theory, derive a hypothesis, and observe if it holds up in a specific setting (data) Theory → hypothesis → data When is a Scientific Approach Especially Useful in an Organizational Setting? When making high-stakes decisions (e.g., hiring policies, marketing strategies). When identifying patterns to predict future trends (e.g., customer behavior). Limitations of a Scientific Approach Not all human behavior can be quantified. Ethical concerns can limit experimentation. Example of a Question That Can’t Be Answered Using Scientific Research “What is the meaning of life?” – It’s philosophical, not empirical. Why Does Inductive Reasoning Lead to Weaker Conclusions Than Deductive Reasoning? Inductive: the conclusion is still uncertain even if the evidence is true because the content of the conclusion goes beyond the evidence Benefits of Deductive vs. Inductive Research Deductive: More precise, allows hypothesis testing. Inductive: More exploratory, useful when there’s little prior research. Week 2 Research Design: Setting the Stage Unit of Analysis: Definition & Example “the level of social life on which a research question is focused” OR entities such as people, nations, and artifacts that are studied, which are described and compared in terms of variables ○ Example: Individuals, groups, towns, or nations. Ecological Fallacy: Definition & Example An error in reasoning in which incorrect conclusions about individual-level processes are drawn from group-level data. ○ Example: Assuming all employees in a company with high productivity are highly motivated. ○ Ex: The crime rate in Suzy’s neighborhood is 3x higher than the rest of the city. Therefore, Suzy is 3x more likely to commit crime than someone who doesn’t live in her neighborhood. Cross-Sectional Research Design: Definition & Example Examines data at a single point in time. one sample, one moment in time ○ Example: Surveying employees about job satisfaction in 2024. Repeated Cross-Sectional Research Design: Definition & Example Collecting data from different people at multiple time points. ○ Example: Conducting employee satisfaction surveys in 2020, 2022, and 2024. Repeated cross sections allow researchers to track continuity and change in adolescent attitudes & behaviors across time & generation, but they do not allow consideration of what happens to youth in the sample in the years after they take the survey Panel Data Research Design: Definition & Example Follows the same individuals over time. ○ Example: Tracking the career progression of graduates from 2015 to 2025. Panel data allows researchers to gauge basic pathways that youth take as they move through adulthood, but it does not allow any historical comparison of this youth cohort to those who came before or after Cross-Sectional vs. Longitudinal Research Designs Cross-Sectional: Faster, cheaper, but limited in tracking changes. Longitudinal: Tracks change over time but takes longer and costs more. Qualitative Research: Definition & Example LANGUAGE. deepen understanding through providing in-depth knowledge - questions ask about social processes or the meaning and cultural significance of people’s actions ○ Aim is to establish context-specific meaning or contribute to the development of theory ask about social processes or the meaning and cultural significance of people’s actions Great for understanding meaning, process, and for developing theory Quantitative Research: Definition & Example NUMBERS. uncover general relationships - questions ask about the empirical relationship between two or more variables ○ Aim is to test hypotheses or establish causality ○ Example: Measuring sales performance before and after training. ask about social processes or the meaning and cultural significance of people’s actions Focuses on relationships between variables, often with the goal of establishing causal relationships Variable: Definition & Example A variable is a measured concept: something that varies across cases or across time ○ Question: Are older people more afraid of crime than younger people? Variables: age / fear of crime Independent Variable: Definition & Example The cause in an experiment. ○ Example: Amount of training received. Dependent Variable: Definition & Example The effect being measured. ○ Example: Employee performance scores. Causal Evidence: Association, Direction of Influence, Non-Spuriousness Association: Variables must be related. (More training → higher productivity) Direction of Influence: The cause precedes the effect. (Training happens before productivity increases) Non-Spuriousness: No third factor causing the change. Antecedent & Intervening Variables: Definitions & Examples Antecedent: Comes before the independent variable. ○ Example: Company culture influences training programs → training affects performance. Intervening: Comes between the independent and dependent variable. ○ Example: Training increases motivation → motivation improves performance. Is Qualitative Data Systematically Collected? Yes, through structured interviews, focus groups, or ethnography. Week 3 Developing Research Questions Qualities of a Good Research Question Clear, focused, and answerable using data. Two Basic Rules of Scientific Research Questions 1. Must be testable. 2. Must be based on empirical observation. Week 4 Research Ethics The Belmont Report Principles 1. Respect for Persons: Informed consent required. a. Acknowledge autonomy of people. People have agency and can consider and make decisions for themselves in their own interests b. Protect vulnerable populations i. Diminished autonomy: children, prisoners ii. Diminished cognitive ability: stroke patients iii. Oppressed groups: political dissidents 2. Beneficence: Minimize harm, maximize benefits. a. Beneficence = the responsibility to do good – to maximize benefits for science, for humanity, and for research subjects – and to prevent subjects from suffering any harm as a result of the research. 3. Justice: Fair distribution of research benefits. a. Justice requires that the risks and potential benefits of the research be equally distributed among subjects. Four major concerns researchers must consider when it comes to the treatment of human subjects 1. Potential harm (respect for persons, beneficence) a. Life, physical health – but also emotional distress b. How to manage it: informed consent, screening out those most likely to be harmed, resources to ameliorate harm 2. Informed consent (respect for persons) a. Freedom of choice matters: give participants enough information about the research (risks and benefits) to make a rational decision about whether to participate b. No coercion – participation must always be voluntary 3. Deception (respect for persons, beneficence) a. Intentionally misleading or misinforming participants about aspects of a study b. Q: Is it ever okay? 4. Invasion of privacy (respect for persons) a. Right to privacy: individual decides when, where, to whom, and to what extent their attitudes, beliefs, and behavior will be revealed b. Anonymity & confidentiality Ethical Issues in HDO Research A study should be designed in a way that will get an understandable answer to an important research question Invalid research is unethical because it is a waste of resources and exposes people to risk for no purpose. It can also lead to bad policy and bad science The Rosenhan Experiment: Why Unethical? Psychologists faked mental illness to test psychiatric hospitals → risked harm to real patients. Measurement and Measurement in Action Conceptualization defining and clarifying the meaning of a given concept. ○ What do you mean by __________? Conceptualization is a process that involves producing clear, concise definitions of what we seek to measure with our empirical observations Operationalization process of identifying the empirical indicators and procedures for applying them to measure a concept. ○ How are we going to capture this concept? Indicator: definition? Example? something that points to, provides evidence of, or otherwise measures a concept ○ Shows the presence, absence, or degree to which the concepts exist Observable characteristics of your concept What indicates your concept in real life? Many concepts have multiple facets, or dimensions - - you may need measures of EACH dimension ○ Example: religiosity may be composed of beliefs, rituals, knowledge, community engagement, etc. Level of measurement: process of assigning numbers or labels to units of analysis to represent their conceptual properties. ○ Capturing/recording the concept you’ve operationalized. (doing it) All operational definitions specify procedures for sorting units into different categories – but categories vary in their level of measurement The level at which you measure a variable determines what you can and can’t say about a relationship Also impacts how you can analyze your data ○ If unsure, err on the side of a HIGHER level of measurement You can always collapse categories into a lower level of measurement, but you can never disaggregate categories to a higher level of measurement after the data has been collected CAN go higher → lower CANNOT go lower → higher How do we know if a measure is a “good measure”? What are the two principles researchers agree on? Reliability (consistency) ○ Can the results be reproduced under the same conditions? Validity (accuracy) ○ Do the results really measure what they are supposed to measure? What is the US Census? The U.S. Census is a count of every person living in the United States, conducted by the U.S. Census Bureau every 10 years. It's mandated by the Constitution. The dangers of not including enough/the right categories in a measure (Hansi Lo Wang article) – what’s the problem? Erasure of populations Undercounting Masking heterogeneity Inadequate policy responses Reinforcing outdated concepts Misallocation of resources Week 5 Sampling Population vs. Sample Population: Entire group of interest. complete set of persons, objects, or events that share some characteristic Sample: Subset of the population studied. subset of a population Random Selection vs. Non-Random Selection Random: Everyone has equal chance of being chosen. Non-Random: Bias may be present (e.g., convenience sampling). Why Is a Bigger Sample Better? Reduces error and improves accuracy. Sampling Techniques & Examples Simple Random Sample: Every individual has an equal chance. Stratified Random Sample: Groups split based on characteristics, then sampled. Cluster Sampling: Randomly selecting groups rather than individuals. Standard error: is the standard deviation of a statistic's sampling distribution or an estimate of that standard deviation1. A larger standard error indicates less precision and is generally considered less desirable, as it suggests more variability in the estimate Simple random sample: definition? Example? Every unit of the population has the same chance of being selected. Your sampling frame should contain EVERYONE in the population. ○ Basically drawing names out of a hat Stratified random sample – and pros/cons versus simple random sample Intentionally divide up the total population into strata, then randomly or systematically sample from each strata (e.g. by age, race, gender, etc.) This technique is good for heterogeneous populations and makes sure you get a combined sample that more closely approximates the population. Cheaper and reduced margin of error Proportionate stratified random sample: definition, example the sample size of each stratum/group is proportional to the stratum’s share in the population Disproportionate stratified random sample: definition, example some groups may be more/less present in the sample than they are in the population Multistage cluster sampling: definition? Example? Intentionally divide up the total population into clusters. ○ Cluster samples allow researchers to conduct many more surveys at a much lower cost than they would incur with simple random sampling ○ Cluster sampling allows researchers to take probability samples when a sampling frame – a list of all the members of a population – doesn’t exist Ex: Demographic & Health Surveys (DHS) - - surveys conducted in dozens of developing countries to obtain vital information; e.g. the proportion of the nation’s adults who are HIV positive Conflict over sampling at the Census: what happened (broadly speaking) and how does this connect to what we have learned about probability sampling? (to be further discussed on Mon) Non-probability sample: ○ methods of selecting cases other than random selection Why do we use it? Sometimes we have no choice – random selection isn’t possible Useful when the aim is to develop a holistic understanding of complex social units ○ When we are only studying a single unit or small sample of units – usually better to leave the selection of cases to expert judgment ○ How likely is the case to enhance my insight & understanding? Purposive sample, snowball sample, theoretical sample (to be discussed on Mon) ○ sampling is guided by researcher expertise on which units are best suited to provide information on particular questions/topics UNLIKE probability sampling (where picking cases has nothing to do with the characteristics of your cases), here picking cases has EVERYTHING to do with their characteristics ○ Snowball sampling: Members of target population are located & they then refer other members of that population ○ Common in hidden/stigmatized/deviant populations for whom a sampling frame does not exist Ex: the super-rich, intravenous drug users, illegal gun sellers, computer hackers ○ Theoretical sampling: iterative sampling strategy in which the researcher continually recruits participants to test emerging theories based on new data and emerging theory Statistical vs. theoretical inference (to be discussed on Mon) ○ Statistical inference: Uses data to make conclusions about populations Relies on probability and statistics Deals with real-world data and uncertainty ○ Theoretical inference: Based on logical reasoning and math models Works with abstract concepts Doesn't directly use real-world data Statistical inference uses actual data and accounts for uncertainty Theoretical inference uses abstract models and often assumes ideal conditions Saturation (to be discussed on Mon) ○ the point at which no new insights are generated from data collection, & responses become redundant continue to sample cases until little or no new information can be extracted from the data