Biostatistics 4th Seminar 2017-18 PDF
Document Details
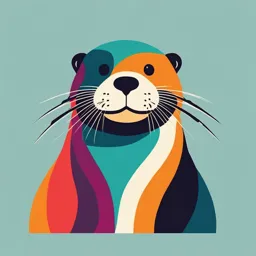
Uploaded by ComfortingAestheticism
University of Debrecen Faculty of Medicine
Tags
Summary
This document details examples of binomial and Poisson distribution in statistics for an undergraduate-level seminar. It includes various examples of calculations and probability problems related to different scenarios.
Full Transcript
4th seminar ▪ Binomial distribution ▪ Poisson distribution ▪ Examples Week 5 Discrete distributions. ❖ Binomial distribution (Bernoulli-distribution). there are EXACTLY two outcomes in the outcom...
4th seminar ▪ Binomial distribution ▪ Poisson distribution ▪ Examples Week 5 Discrete distributions. ❖ Binomial distribution (Bernoulli-distribution). there are EXACTLY two outcomes in the outcome space: Event „A”, and „NOT A”. Many variables in medicine have exactly 2 (dichotomous) outcomes, such as survival, gender, test results being positive or negative, presence or absence of a disease each observation falls into one of just two categories. Let the probability of event A be signed by P(A)=p, and event Ā by P(Ā) = 1 – p = q the n observations are all independent (independence assumption) the probability of a given outcome is constant over all trials (homogeneous assumption) the number of trials n is fixed and specified in advance. Bn,k gives the probability that a given event occurs k times in n independent trials with two possible outcomes with probabilities p and q: n! n Bn ,k = pk = P ( = k ) = p k q n−k = p k q n−k where n is the number of trials k ! ( n − k ) ! k the mean and the standard deviation of the binomial M ( ) = = n p distribution are: D ( ) = n p q Discrete distributions II. ❖ Poisson distribution. in the case of an event which is rather unlikely to happen (small p), but has a lot of opportunities to do so (large n) the expected number of total occurances (λ) is non-negligible: np= λ, the parameter of the Poisson-distribution k − lim Bn ,k = pk = e if ( lim n×p = λ ) > 0 k → k! if λ is the expected number of occurrences of an event, the probability that it occurs k times is given as k pk = P ( = k ) = e− k = 0, 1, 2,... k! the mean and the standard deviation of the Poisson distribution are: M ( ) = D ( ) = Example Roll a fair dice five times and record the number of dots on the up face of the dice. What is the probability that six dots on the up face occur exactly three times? Example Roll a fair dice five times and record the number of dots on the up face of the dice. What is the probability that six dots on the up face occur exactly three times? Solution The binomial distribution has to be used, since there are two outcomes (6 or any other side facing up), and the experiment is independently repeated 5 times. The number of elements, n, is 5. If this is considered to be a Bernoulli process, the probability of one of the outcomes, i.e. the probability of six dots coming up, is 1/6. The probability of the complementary event, i.e. any number of dots other than six facing up, is 5/6. Each time the dice is rolled the probability of six dots on the up face is 1/6. However, we perform this experiment 5 times, and we would like to determine the probability of obtaining the event of six dots facing up exactly 3 times, i.e. k=3 out of 5 experiments. Summarizing the above and applying the binomial formula: n= 5 3 2 k=6 n! 5! 1 5 P ( k = 3) = p k q n−k = = 0.03215 p = 1/6 = 0.167 k ! ( n − k ) ! 3! 2! 6 6 q = 5/6 = 0.833 Conclusion: The probability that six dots on the up face occurs exactly three times is 0.03215. Example 2. A quiz consists of five multiple-choice questions, each with four possible answer choices (A, B, C, or D), one of which is correct. Suppose that an unprepared student does not read the question, but simply makes one random guess for each question. What is the probability that the student will get all the five questions correct? Example 2. A quiz consists of five multiple-choice questions, each with four possible answer choices (A, B, C, or D), one of which is correct. Suppose that an unprepared student does not read the question, but simply makes one random guess for each question. What is the probability that the student will get all the five questions correct? Solution 1 5! ( 0.25) ( 0.75) = 0.00097 5 0 n = 5, p = = 0.25, P(k = 5) = 4 5!0! Example 3. 80% of a population are immune from a certain disease. If a random sample of 10 people is drawn from this population what is the probability that exactly 6 will be immune from the disease? Example 3. 80% of a population are immune from a certain disease. If a random sample of 10 people is drawn from this population what is the probability that exactly 6 will be immune from the disease? Solution the binomial distribution has to be used, since there are two outcomes (immune or not immune from the disease), and the experiment is independently repeated 10 times. the number of elements, n, is 10 the probability of one of the outcomes, i.e. the probability of immunity, is 0.8 the probability of the complementary event is 0.2 each time a person is drawn the probability of immunity is 0.8 However, we perform this experiment 10 times, and we would like to determine the probability of obtaining the event of immune from the disease exactly 6 times, i.e. k=6 out of 10 experiments. summarizing the above and applying the binomial formula: n= 10 n! 10! k=6 P ( k = 6) = p k q n−k = 0.86 0.24 = 0.088 p = 0.8 k !( n − k )! 6!4! q = 0.2 conclusion: the probability that exactly 6 people will be immune from the disease is 0.088. Example 4. Suppose that 13 percent of a certain population is diabetic. If a random sample of 7 people is drawn from this population find the probability that more than two will be diabetic? Example 4. Suppose that 13 percent of a certain population is diabetic. If a random sample of 7 people is drawn from this population find the probability that more than two will be diabetic? Solution the sum of the probabilities where k is more than 2 has to be calculated: P ( k 2 ) = P ( k = 3) + P ( k = 4 ) + + P (k = 7) this would require the calculation of 5 binomial formulas. In such cases it is often worth considering the following equation: P ( k = 0 ) + P ( k = 1) + P ( k = 2 ) + + P (k = 7) = 1 P ( k 2 ) = 1 − P ( k 2 ) = 1 − P ( k = 0 ) + P ( k = 1) + P ( k = 2 ) therefore, we can reduce the number of terms to be summed to three, i.e only 3 binomial formulas have to be calculated summarizing the above and applying the binomial formula: p = 0.13 7! 7! 7! P ( k 2) = 1 − 0.130 0.877 − 0.1310.876 − 0.132 0.875 = 0.0521 q = 0.87 0!7! 1!6! 2!5! conclusion: the probability that more than two people will be diabetic is 0.0521. Example 5. Suppose researchers determine that a new drug has a 40 percent chance of preventing infection by a certain flu strain. If the drug is administered to 8 subjects, what is the probability that the drug will be effective in preventing the infection a) in exactly 6 of the subjects? b) in fewer than 6 subjects? Example 5. Suppose researchers determine that a new drug has a 40 percent chance of preventing infection by a certain flu strain. If the drug is administered to 8 subjects, what is the probability that the drug will be effective in preventing the infection a) in exactly 6 of the subjects? b) in fewer than 6 subjects? Solution 8! a) P ( k = 6) = 0.46 0.62 = 0.0413 n= 8 6! 2! p = 0.4 q = 0.6 b) P ( k 6 ) = 1 − P ( k 6 ) = 1 − P ( k = 6 ) + P ( k = 7 ) + P ( k = 8 ) 8! P (k = 7) = 0.47 0.61 = 0.0078 7!1! 8! P ( k = 8) = 0.480.60 = 0.000655 8!0! P ( k 6 ) = 1 − 0.0413 − 0.0078 − 0.000655 = 0.95 Example 6. Albinism is a rare recessive inherited disorder. It is estimated that the probability that someone suffers from albinism is 1/20000. Let us assume that we investigate a population of 10000 people. What is the probability that there will be a. exactly three albino, b. at least three albino individuals in the population? Example 6. Albinism is a rare recessive inherited disorder. It is estimated that the probability that someone suffers from albinism is 1/20000. Let us assume that we investigate a population of 10000 people. What is the probability that there will be a. exactly three albino, b. at least three albino individuals in the population? Solution a.) we can use the Poisson distribution to solve this problem, since n is large and p is small (n – the number of patients in the population, p – the probability that some is albino). 1 determine the mean of the Poisson distribution: = np = 10000 = 0.5 20000 k 0.53 −0.5 the probability: P ( k = 3) = e − = e = 0.0126 k! 3! conclusion: the probability that exactly three albino individuals in the population is 0.0126. Example 6. Albinism is a rare recessive inherited disorder. It is estimated that the probability that someone suffers from albinism is 1/20000. Let us assume that we investigate a population of 10000 people. What is the probability that there will be a. exactly three albino, b. at least three albino individuals in the population? Solution b.) let us look at the statement “more than or equal to 3 (i.e. at least..)” more closely: P ( k 3) = P ( k = 3) + P ( k = 4 ) + P ( k = 5 ) + it is not obvious at which k the summation is to be finished. But if we notice that P ( k 3) + P ( k 3) = 1 therefore we can reduce the number of terms to be summed to three: P ( k 3) = 1 − P ( k 3) = 1 − P ( k = 0 ) − P ( k = 1) − P ( k = 2 ) 0.50 −0.5 0.51 −0.5 0.52 −0.5 −0.5 0.52 P ( k 3) = 1 − e − e − e = 1 − e 1 + 0.5 + = 0.014 0! 1! 2! 2! conclusion: the probability that the number of albino persons is at least 3 in the population of 10000 persons is 0.014. Example 7. The mean number of patients arriving at the emergency room of a university hospital on Saturday nights between 10:00 and 12:00 (2-hour interval) is 6.5. Assuming that the patients arrive randomly and independently, what is the probability that on a given Saturday night a) exactly two patients arrive at the emergency room in the 2-hour interval between 10:00 and 12:00? b) the number of patients arriving at the emergency room in the 2-hour interval between 10:00 and 12:00 is less than or equal to 2? c) the number of patients arriving at the emergency room in the 1-hour interval between 10:00 and 11:00 is more than or equal to 2? Example 7. The mean number of patients arriving at the emergency room of a university hospital on Saturday nights between 10:00 and 12:00 (2-hour interval) is 6.5. Assuming that the patients arrive randomly and independently, what is the probability that on a given Saturday night a) exactly two patients arrive at the emergency room in the 2-hour interval between 10:00 and 12:00? b) the number of patients arriving at the emergency room in the 2-hour interval between 10:00 and 12:00 is less than or equal to 2? c) the number of patients arriving at the emergency room in the 1-hour interval between 10:00 and 11:00 is more than or equal to 2? Solution a.) We have to use the Poisson distribution to solve this problem. For problems involving the Poisson distribution the mean (parameter ) of the distribution has to be determined. λ = 6.5 6.52 −6.5 P ( k = 2) = e = 0.032 2! b.) We have to sum three probabilities: the probability that no, one or two patients arrive at the emergency room in the 2- hour interval: 6,50 −6,5 6,51 −6,5 6,52 −6,5 P ( k 2 ) = P ( k = 0 ) + P ( k = 1) + P (k = 2) = e + e + e = 0, 043 0! 1! 2! Example 7. The mean number of patients arriving at the emergency room of a university hospital on Saturday nights between 10:00 and 12:00 (2-hour interval) is 6.5. Assuming that the patients arrive randomly and independently, what is the probability that on a given Saturday night a) exactly two patients arrive at the emergency room in the 2-hour interval between 10:00 and 12:00? b) the number of patients arriving at the emergency room in the 2-hour interval between 10:00 and 12:00 is less than or equal to 2? c) the number of patients arriving at the emergency room in the 1-hour interval between 10:00 and 11:00 is more than or equal to 2? Example 7. The mean number of patients arriving at the emergency room of a university hospital on Saturday nights between 10:00 and 12:00 (2-hour interval) is 6.5. Assuming that the patients arrive randomly and independently, what is the probability that on a given Saturday night a) exactly two patients arrive at the emergency room in the 2-hour interval between 10:00 and 12:00? b) the number of patients arriving at the emergency room in the 2-hour interval between 10:00 and 12:00 is less than or equal to 2? c) the number of patients arriving at the emergency room in the 1-hour interval between 10:00 and 11:00 is more than or equal to 2? Solution c.) We have to consider that a 1-hour interval has to be investigated in this part of the problem requiring a calculation of . Since patients arrive randomly and independently if the length of the time interval is reduced 2-fold, the mean number of patients arriving to the unit will be. 6.5 = = 3.25 2 let us look at the statement “more than or equal to 2” more closely: P ( k 2 ) = P ( k = 2 ) + P ( k = 3) + P ( k = 4 ) + It is not obvious at which k the summation is to be finished. But we can reduce the number of terms to be summed to two if we notice that: P ( k 2) + P ( k 2) = 1 P ( k 2 ) = 1 − P ( k 2 ) = 1 − P ( k = 0 ) − P ( k = 1) 3.250 −3.25 3.251 −3.25 P ( k 2) = 1 − e − e = 1 − e −3.25 (1 + 3.25 ) = 0.835 0! 1! Example 8. We introduce the gene of a fluorescent protein into cells so we can investigate the subcellular localization of this protein in the microscope. The transfection efficiency is 1%. In an average field of view, usually there are 100 cells. What is the probability that when we look in the microscope we are so lucky as to see at least 3 transfected (brightly fluorescing) cells? Example 8. We introduce the gene of a fluorescent protein into cells so we can investigate the subcellular localization of this protein in the microscope. The transfection efficiency is 1%. In an average field of view, usually there are 100 cells. What is the probability that when we look in the microscope we are so lucky as to see at least 3 transfected (brightly fluorescing) cells? Solution Can we use the Poisson distribution? Borderline case, p is small, but not extremely, and the number of chances for the occurrence of the event is not infinite, although 100 is quite large. =np = 100×0.01 = 1, on average we see 1 bright cell per field of view P ( k 3) = 1 – P ( k 3) = 1 – P ( k = 0 ) + P ( k = 1) + P ( k = 2 ) = With binomial: Using Poisson-distribution: 100! 10 –1 P ( k = 0) = 0.010 0.99100 = 0.36603 P ( k = 0 ) = e = 0.3678 0!100 ! 0! 100! 11 –1 P ( k = 1) = 0.011 0.9999 = 0.36972 P ( k = 1) = e = 0.3678 1!99 ! 1! 100! 12 –1 P ( k = 2) = 0.012 0.9998 = 0.18486 P ( k = 2 ) = e = 0.1839 2!98! 2! P ( k 3) = 0.07939 P ( k 3) = 0.08046 With Poisson: 8.04%, with binomial: 7.94%, so approximating the binomial with the Poisson was good. Example 9. The chance that someone suffers an adverse reaction from a dangerous medical treatment is 0.4. What is the probability that in a sample of 5 patients at least two will suffer an adverse reaction using a) the binomial distribution b) the Poisson approximation to the binomial distribution Example 9. The chance that someone suffers an adverse reaction from a dangerous medical treatment is 0.4. What is the probability that in a sample of 5 patients at least two will suffer an adverse reaction using a) the binomial distribution b) the Poisson approximation to the binomial distribution Solution With binomial n=5 (total number of individuals in the sample) k=0 or 1 (i.e. numbers of people with adverse reaction in the sample, “inverse” approach) p=0.4 (probability of a bad reaction) q=0.6 (probability of not having a bad reaction) 5 5 P ( k 2 ) = 1 − P ( k = 0 ) − P ( k = 1 ) = 1 − 0.4 0 0.65 − 0.41 0.64 = 1 − 0.6 5 − 5 0.4 0.6 4 = 0.663 0 1 Using Poisson-distribution: = np = 5 0.4 = 2 20 21 P ( k 2 ) = 1 − P ( k = 0 ) − P ( k = 1 ) = 1 − e + = 1 − e −2 (1 + 2 ) = 0.594 −2 0! 1! Conclusion: The probability that at least 2 persons suffer an adverse reaction in a sample of 5 is 0.663 and 0.594 according to the binomial and Poisson distribution, respectively. It can be seen that the Poisson distribution is not a good approximation to the binomial distribution in this case due to the small value of n and the large value of p. Example 10. A solution of 1 dl is contaminated with 3×105 bacteria. If a sample of 1 l is taken, what is the probability that there will be at least one bacterium in it? Example 10. A solution of 1 dl is contaminated with 3×105 bacteria. If a sample of 1 l is taken, what is the probability that there will be at least one bacterium in it? Solution Determination of , method 1: The value of the parameter has to be determined for a sample of 1 l: = np where n is the number of bacteria in the sample of 1 dl (3×105), p is the probability that an arbitrarily chosen bacterium gets into a sample of 1 l. This is obviously equal to the volume ratio: 1 μl 1 μl p= = 5 = 10 −5 = 3 105 10 −5 = 3 1 dl 10 μl Determination of , method 2: Since is the expected number of bacteria in a 1 l sample, the density of bacteria has to be calculated. If the density is determined in l, it will be equal to the expected number of bacteria in 1 l: n 3 105 = = = 5 =3 V 10 μl Determination of , method 3: can be determined with a proportion: in 105 l – 3×105 bacteria in 1 l – bacteria the required probability: 1 = 3 105 5 = 3 0 10 P ( k 1) = 1 − P ( k = 0 ) = 1 − e − = 1 − e − = 1 − e −3 = 1 − 0.49787 = 0.950213 0! Example 10. A solution of 1 dl is contaminated with 3×105 bacteria. If a sample of 1 l is taken, what is the probability that there will be at least one bacterium in it? Solution With binomial n=3×105 (number of bacteria) k=0 p=10-5 (the probability that any bacterium gets into the 1 l sample) q=1–10-5 = 99999/100000 (the probability that any bacterium does NOT get into the sample) 3105 3105 3 105 −5 0 99999 99999 P ( k 1) = 1 − P ( k = 0 ) = 1 − (10 ) =1− = 1 − 0.049786 = 0.950214 0 100000 100000