Glossary RM Assignment 1 PDF
Document Details
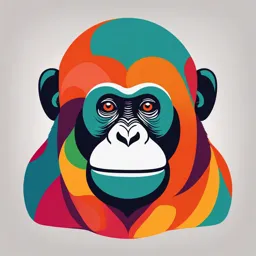
Uploaded by ImpartialPoplar
Alma Mater Studiorum - Università di Bologna
Tags
Summary
This document appears to be a glossary or assignment related to univariate descriptive statistics. It defines key terms and concepts in the field. The document includes various terms and definitions related to measurements, variables, associations, and dispersion in statistics.
Full Transcript
**ASSIGNMENT 1** **(Univariate Descriptive Statistic)** - A **population** is the entire set of what you wish to draw conclusions about. A **semple** is a subset of units in the population of interest**.** - **Conceptualization** is the process defining the concepts in which we ar...
**ASSIGNMENT 1** **(Univariate Descriptive Statistic)** - A **population** is the entire set of what you wish to draw conclusions about. A **semple** is a subset of units in the population of interest**.** - **Conceptualization** is the process defining the concepts in which we are interested. **Operationalization** is the process of measuring our concepts with empirical referents in the real world. - The **level of measurement** is a description of the type of data that comprise a variable which shapes the subsequent statistics techniques that can be used. **Nominal-level variables** are unordered values or categories. **Ordinal-level variables** are values or categories that, while having a clear order, do not lend themselves easily to statistical analysis as the intervals between each category are not the same mathematically. **Interval-level variables** have values and intervals that are mathematically tractable. - **Measures of central tendency** summarize variables with a representative value vs **Measures of dispersion** describe the distribution of values around the measure of central tendency in turn telling us how representative that measure is. - **Variance** describes how close or far the observations are to our summary statistic. The **mode** is a measure of central tendency used for nominal-level variables. It is the category with the most observations. **Relative frequencies** are the proportional frequency of each category relative to the total. **The variation ratio** is a measure of dispersion used for nominal-level variables. It describes the variation around the mode. The **median** is a measure of central tendency used for ordinal-level variables. It is the observation in the middle such that 50% of the data fall on both sides. The **range** is a measure of dispersion used for ordinal-level variables. It describes extent of values for the variable: i.e.: the highest to the lowest values. The **mean** is a measure of central tendency used for interval-level variables. It is the sum of all the values divided by the number of observations. The **standard deviation** is a measure of dispersion used for interval-level variables. It describes the variation around the mean by measuring the average distance of all the data from the mean. - **Outliers** are observations in the data that deviate from the rest of the data. - **Measures of Association** assess the existence, strength, and, on occasion, direction of a relationship between variables. **Cross-tabulation** is an explicit means to show the joint distribution of nominal- and ordinal-level variables. A.k.a.: \'cross-tab\'. **Joint distribution**: The distribution of responses as a function of the other distribution of responses. You can already see how this is helpful in understanding how one variable \'moves\' or is coordinated with another. - **Yule\'s Q** is the specific 2x2 form of Goodman and Kruskal\'s gamma. Goodman and Kruskal\'s **Lambda** is a proportional reduction of error (PRE) measure of association for nominal-level by ordinal-level variables (of any size; N x N). Goodman and Kruskal\'s **Gamma** is a proportional reduction of error (PRE) measure of association for ordinal-level variables. The **direction** of a relationship provided by gamma for ordinal-level variables tells us the nature of the relationship - whether the variables increase (and decrease) together - a positive relationship - or move in opposite directions (as one increases, the other decreases - a negative relationship). The **magnitude** of a relationship is how closely the two variables are associated. A high magnitude of association implies that two variables move together in a conspicuous and predictable way. A low magnitude of association implies that two variables do not move together in a meaningful or obvious way. - A **zero-order relationship** describes the relationship between two variables and nothing else. - **Controlled comparisons** are a means to begin to establish how much of the change in the dependent variable (Y) is due to changes in independent variable (X) and how much is due to something else such as another independent variable (e.g: Z). - **ASSIGNMENT 2** **(Measure of association and Bivariate)** - **Means Comparison** compares the means of interval-level variable across the categories of the nominal-level or ordinal-level variable. A **scatterplot** is a graph that displays the joint distribution of two interval-level variables as a set of points on a Cartesian coordinate system. - **Correlation** measures the extent of which two interval-level variables are linearly related to each other, indicating both strength and direction. The most used and well-known correlation is **Pearson\'s Product Moment Correlation** **Coefficient**. This is denoted by an \'***r***\' and often simply referred to as \'correlation\'. - A [positive correlation] means that the two interval-level variables increase and decrease together. - A [negative correlation] means that the two interval-level variables move in opposite directions (i.e.: as one increases, the other decreases). - Regression analysis a description of the linear relationship between a dependent and independent variable that is variable-specific. The **Regression Equation**, given a dependent variable, Y, and an independent variable X, is: \ [*y*= *α*+ *β*(*x*~*i*~) + *ε*]{.math.display}\ - **(Bivariate) linear regression** is a specific form of regression in which we examine the relationship between two interval-level variables. **Correlation** describes the relationship between two interval-level variables. [What then is the new information we get from (bivariate) regression? ] - (Bivariate) regression gives us variable-specific information -- a mathematical summary in the metric of the independent and dependent variables - on how the two variables move together. - (Bivariate) regression gives us results that respect the theoretical order of the independent and dependent variables. - (Bivariate) regression tells us where we can expect to find values of the dependent variable by knowing the independent variable. - (Bivariate) regression tells us how well a line summarizes the relationship. - **Regression coefficient** [*β*]{.math.inline} is a component of the regression equation that relates the average change in Y associated with a unit change in X. It also describes the relationship as negative or positive. It is sometimes called the ***\'slope\'*** of the line. **Intercept** ([*α*]{.math.inline} - alpha) is a component of the regression equation that indicates the point where the regression line \'intercepts\' the Y axis (i.e.: ***when X=0***). **Error term** ([*ε*]{.math.inline}) is a component of the regression equation that represents the presence of error of trying to match the regression analysis to the real world. - **Systematic Components** of the regression equation capture a deterministic relationship in which there is no randomness. It is the part that we try explain: [*α*+ *β*(*x*~*i*~)]{.math.inline}. **Random Components** of the regression equation refer to the error term [*ε*]{.math.inline} in which the impact of everything else in the world sums to zero. [Difference between the **systematic** and **random parts** of the regression equation? ] - In a regression equation, the systematic components refer to the intercept and the beta coefficient. - The random parts of a regression equation refer to the error term. - The systematic components are the part of the regression equation that we control by introducing an independent variable. - The random components of the regression equation represent the random effects that explain the dependent variable but are not included. - The **Coefficient of Determination** ([*R*^2^]{.math.inline}) represents the proportion of common variation between the two variables. - **Model Fit** is the appropriateness of the included independent variables as a solution for the variation in the dependent variable. A.K.A.: Goodness of Fit. Modelling is the process through which we fully examine the relationship in which we are interested. The steps move from scientific design, to the use of statistical techniques, to interpretation. The assumptions or criteria of regression analysis are necessary so that the estimation of the regression components provides reliable (i.e.: unbiased and efficient) estimates of the real regressionparameters. When met, the assumptions strenghten our confidence un the results of the regression. **ASSIGNMENT 3** **(Inference for Nominal and Ordinal Level Variable)** - **Inference** is the general process by which one uses observed data to learn about the social system and it outputs. **Inferential Statistics** involves making informed guesses about population values - or parameters - from a sample by estimating the probability that the result could be due to chance. - **Substantive significance** is the nature of the results in the sample. **Statistical significance** is wheter the results of the sample can be inferred to the population from which the sample was drawn (*more specific the difference is that Substantive significance describes the relationship in the sample. Statistical significance is whether these results can be inferred to the population from which the sample was drawn*). [Statistical significance means that what we have observed in the sample are unlikely to be a function of chance. Statistical significance tell us nothing about causation. Although statistical significance is necessary but not sufficient in order to identify a potential causal relationship.] Substantive significance is the size or magnitude of a description, relationship, or pattern in a data. The logic of (statistical) inference is that the results from our sample are so unlikely to be a function of chance that they must represent a real relationship in the population from which that sample was drawn. - [A **parameter** is a descriptive characteristic of a population. A statistic refers to the estimate of a parameter from sample data.] A **simple random sample** occurs when each unit in the population has an equal chance of becoming a part of the sample. The law of large numbers states that if we draw observations at random from any population, as number of observations increases, our sample statistics closes in on the population parameter. [A key element to being able to make proper inferences in statistical analysis is a sufficiently sized and random sample. ] - **Randomization** is the selection of units of analysis on the basis of chance and not design. **PROBABILITY** is a formal model of uncertainty that assigns a numerical measure of the chance, or likelihood, that a particular event will occur. The classical method assigns equiprobability for each outcome or event \[1/n where \'n\' is the number of possible events or outcomes\]. The relative frequency method assigns probabilities based on data available to estimate the likelihood of outcomes. A union of events contain all outcomes in which we are interested. The notation is: E, U Ej and the formula is: P(E, U Ej) = P(E;) + P(E;) - P(E; n E;). An intersection - a.k.a. the joint probability - contains the outcomes which belong to both E; and Ej. The notation is E; n Ej. The formula is: P(E; n Ej) = P(E;) \* P(E;). The complement of an event is all the other outcomes that are not part of the event: P(Ec) = 1 - P(E) Mutual exclusivity simply means that when one event occurs, the other cannot. Conditional probability is when the probability of an event is influented by whether or no a related event has occurred. If E, given Ej: P(E,\| Ej). The formula is: P(E;\| Ej) = P(E; n). **Independent events** are when an event occurs and does not affect the probability of another event. **Classical hypothesis testing** is a step-by-step procedure to determine statistical significance. It is also commonly referred to as significance testing. **Alternative hypothesis** or research hypothesis (H, or [*H*~*a*~]{.math.inline}) is a is a general, non-normative, directional statement of the expected relationship derived from theory. The **null hypothesis** ([*H*~*a*~]{.math.inline}) is the condition in which there is no relationship or dependence among your variables. Reject the null hypothesis indicates that there is sufficient evidence to conclude that observed relationships in the sample can be inferred to the population from which that sample was drawn. Fail to reject the null hypothesis indicates that there is insufficient evidence to conclude that observed relationships in the sample can be inferred to the population from which that sample was drawn. Substantive significance is the magnitude and sometimes direction of a relationship described in a sample. Statistical significance is ability to make inferences from sample to population with our results. - [**Χ**^**2**^ ]{.math.inline}is a test for independence that allows for inferential claims about the performance of relationships among nominal- and ordinal-level data. When is [**Χ**^**2**^]{.math.inline} appropriate as a test of statistical significance? When you want to make inferential claims about relationships among nominal- and ordinal-level variables. To determine statistical significance, χ2 compares the distribution of expected values versus the distribution of observed values. Why does this approach make sense? For example, if [**Χ**^**2**^ **=** **0**]{.math.inline}, the distribution of expected values would be the exact same as the distribution of observed values. As [**Χ**^**2**^]{.math.inline} grows larger, what -- in the language of statistical significance -- is [**Χ**^**2**^]{.math.inline} trying to tell us? As observed values increasingly differ from expected values, we are seeing something deviate from our expectation, something highly unlikely to occur by chance and therefore likely to exist in the population. - **Degrees of freedom** are a measure crucial to the selection of critical values that relate the number of observations or dimensions of the variables under investigation. Confidence is the extent a result is statistically significant or not. Its complement is alpha. A **test statistic** is calculated from our sample data and indicates the extent our result deviates from what is expected. **Alpha (**[**α**]{.math.inline}**)** is the level of significance ([**α** **=** **0.05** **→** **95%** \| **α** **=** **0.01** **→** **99%** \|**α** **=** **0.001** **→** **99.9%**]{.math.inline}**)** - [In order to determine statistical significance, we compare a sample-specific test statistic to an existing critical value. What is the role of the critical value]? **The critical value** defines the critical or rejection region in significance testing and is determined by a selected level of confidence and the sample-specific degrees of freedom. A critical value defines the critical region in the sampling distribution with which we decide to reject or fail to reject the test statistic. **ASSIGNMENT 4** **(Inference for Interval Level Variable)** - The three useful properties of the **Normal distribution** 1. **It is symmetric.** 2. **The total area under the curve is 1.** 3. **It has fixed proportions under the curve.** - How do **Z-scores standardize variables** so that they can be directly compared? By standardizing interval-level variables to units of their standard deviations. - The **Central Limit Theorem** makes a number of strong assumptions: Samples from the sampling distribution are actually samples of samples; the distribution of the sample means approaches a normal distribution as the sample size increases, regardless of the population distribution, as long as the sample size is sufficiently large (usually more than 30 observations). - We make inferences about population parameters from our sample in two ways, estimation and hypothesis testing. - One form of (point) estimation is constructing a **confidence interval** around our sample estimate of a population parameter. A confidence interval is range of values consisting of a margin of error -- based on sample size and our level of confidence - on either side of a sample estimate in which we expect to find the population parameter with the chosen level of confidence. \ [\$\$C.I. = sample\\ mean\\ \\pm {(z - score}\_{\\text{confidential\\ level}})(\\frac{\\text{standard\\ deviation}}{\\sqrt{\\text{n\\ observations}}})\$\$]{.math.display}\ - The **standard error** is the measure of dispersion that we use for sampling distributions, the standard error of the mean. - A **t-distribution** is a Standard Normal distribution that adjusts according to the number of observations in the sample. The (critical) **t-value** [defines the critical or rejection region by cleaving the t-distribution into probabilities according to the degrees of freedom and a chosen confidence level]. A (**t-test) t-statistic** - or t-score - is the test statistic generated from our sample for hypothesis testing - What does the **difference of means** test actually test? [That the difference between the means is zero or not]. What is **the null** in this difference of means test [The difference between the means is zero.] **ASSGNMENT 5 and 6** **(Multiple regression)** \ [*Y*= *α* + *β*~1~(*x*~1~)+ *β*~2~(*x*~2~) + ... + *β*~*n*~(*x*~*n*~)+ *ε* ]{.math.display}\ - **Multiple regression** is the technique to test several independent variables simultaneously thus controlling for the impact of each independent variable on the variation of the dependent variable. Three key elements of the regression equation: 1. The regression coefficients which estimate the average change in Y associated with a unit change in X 2. The intercept which is the value of Y when X = 0 3. The error term which contains the random or unaccounted for elements - Why do we not throw all the variables that we can into the multiple regression model? It is inefficient and costly to **adjusted-**[**R**^**2**^]{.math.inline}**.** The adjusted-[*R*^2^]{.math.inline} reports the explained variance in the dependent variable by the set of independent variables and 'adjusts' for both the number of independent variables as well as the number of observations. - Why are multiple regression coefficients referred to as **partials**? They represent each independent variable's partial contribution to the total explained variance of the dependent variable, controlling for the effect of the other included independent variables. - The **p-value** is calculated probability that -- in the case of multiple regression - the estimated regression coefficient is a function of chance. - [How does the **F-test** differ from R2?] The F-test tells us the joint probability that all the regression coefficients are simultaneously zero. - **Dummy variables** are dichotomous variables that allow us to include nominal- and ordinal- level variables in a multiple regression analysis. They essentially make multiple regression to perform a difference of means t-test for each category against a reference category. **The base** or reference category is the category of response against which all dummy variables are compared. - An interaction term captures the separate effects of different independent variables, as well as their interactive effect on the dependent variable. **ASSGNMENT 7** **(5 assumption)** - Primary problem of violating the **assumptions of the linear regression model** The regression coefficients become unreliable - The most correct response to a high level of **multicollinearity** It depends on the question and the state of the literature. - One way to assess the robustness of a model is to use a **proxy variable** in place of key independent variables and look to see how much the model solution changes. We will know whether our model is robust if [the regression coefficients change very little.] - **Heteroscedasticity** is non-constant variance of the error term and for the model it means that [the model is solving some parts of the relationship better than others]. - **Model specification** is a list of the included independent variables and their transformations. - **Biased estimates** create problem(s) for the model: [they misrepresent the relationship between the independent variables and the dependent variable]. - **Inefficient estimates** create problem(s) for the: [the regression coefficients become unreliable as they are poor estimates of the 'true' parameter.] - If the error term is '**well-behaved'** we mean that the **error term is normally distributed**, has a mean of zero, and has constant variance. - [Do these assumptions tell us which variables to include in our model]? To some extent, yes. The relationship among the independent variables and the error term is as important as their relationships with the dependent variable. There has to be a balance in what we choose to include and exclude in our model **ASSIGNMENT 8** **(Binary Logit Regression)** - Conceptually **binary logistic regression** [determines only] the **probability of the dependent variable = 1** Logistic regression gives us the probability of moving from 0 to 1. Conceptually, [it is the shift from one state to another, from the absence of something to the presence of it.] - Categorical (and limited) dependent variables are aclass of regressions ni which the dependent variables are not interval-level variables. Logistic regression produces **logits** as regression coefficients Logits are the **odds of the log**. In Logit regression, independent variables have a linear relationship with the logged odds of the dependent variable. The marginal effect si the ratio of the change in the dependent variable to the change in the independent variable. - The **discrete change** si the change in value from each value of the independent variable. - A **probability density function** (PDF) si a curve under which the area depicts the probability of particular values. A **cumulative density function** (CDF) si a curve under which the area describes the probabilities up to avalue. - In linear models, R2 can be very helpful as it tells us the amount of explained variance of the dependent variable. The output for a binary logistic regression model includes **pseudo-R2** and it is rarely used to show model fit. This beacuse the Logistic regression does not report or even assess explained variance. Thus, we are left with a comparative metric but not an absolute one. - You run a binary logistic regression predicting [whether an individual voted or not in the last election (coded 1 for voted)]. You have only one independent variable in your regression, sex, coded [1 for men] and [0 for women]. You obtain a **regression coefficient of 0.58**. Express the coefficient as an odds ratio and interpret it. *For men, we expect a 0.58 increase in the log-odds of having voted. This means that the odds of having voted increase by a factor of 1.786 (i.e.:* [**e**^**0.58**^]{.math.inline}*).* - **Maximum Likelihood Estimation** MLE selects estimates for population parameters for which the probability of the sample observations is the highest. - **Predicted probabilities** it is the probabilities of the dependent variable at different values of the independent variable(s). - **Ideal types** it summarizes the marginal effects of key variables into profiles in which we might be interested. - **Likelihood Ratio chi2** test determines whether our model is specified correctly with a Chi2 test. - The **Wald test** compares models in which regression coefficients are equal to zero, another number, or even each other. - Odds ratios give us the odds that ysi equal to 1over yequal to 0. - Predicted probabilities are summary probabilities given different assigned values to different independent variables. **ASSIGNMENT 9** **(Ordinal and Multinominal Logit Regression)** - In the Ordinal Regression Model, the **proportional odds** **assumption** asserts the intervals between each adjacent outcome in the dependent variable are uniform. - We use the proportional odds assumption in Ordinal Logistic Regression models so that the regression coefficient estimates [can apply to each 'jump' from one category to the next across the range of the dependent variable]. - How does a multinomial logit model estimate the model? A multinomial logit model handles a polychotomous nominal-level dependent variable by estimating binary logits for each outcome category against a base or reference category. - A crucial decision for Multinomial Logistic regression model that will shape the results of the model is the choice of **base or reference category**. - The most important basis for making this choice is the combination of theory and the potential number of observations in the base category. - The LR χ2 test gives us high confidence in the model as it is currently specified. - The **Ordinal Regression Model** (ORM) estimates dependent variables with more than two outcomes. - **Cutpoints** are when the probability of the impact of values of independent variables changes for outcome categories of the ordinal-level dependent variable. The proportional odds assumption alows the the ods of moving from hte base category ot the other categories ot be the same between each category. Amultinomial logit model handled apolychotomous nominal-level dependent variable by estimating binary logits for each outcome category against a base or reference category. - **Truncated data** have data in which some observations are systematically excluded from observation. **Censored data** have observation but unknown values often beyond some terminal value at either end of the range of outcomes. **Count data** are data that represent occurrences of an event within afixed period.