Mathematics In The Modern World Pdf
Document Details
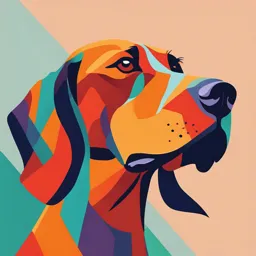
Uploaded by BestMagenta673
Tags
Summary
This document provides an introduction to data management and statistics in mathematics. It covers descriptive and inferential statistics, variables, levels of measurement, and sampling techniques. The document also details different types of graphs.
Full Transcript
MATHEMATICS IN THE MODERN WORLD | REVIEWER FOR FINALS CHAPTER 4: DATA MANAGEMENT (STATISTICS) INTRODUCTION One of the branches of Mathematics that is used in our daily life is Statistics. The word Statistics came from the Latin word “Status” or the Italian word “...
MATHEMATICS IN THE MODERN WORLD | REVIEWER FOR FINALS CHAPTER 4: DATA MANAGEMENT (STATISTICS) INTRODUCTION One of the branches of Mathematics that is used in our daily life is Statistics. The word Statistics came from the Latin word “Status” or the Italian word “Statista”, meaning of these words is “Political State” or a Government. It is a set of mathematical equations that we used to analyze things. It keeps us informed about what is happening in the world around us. Statistics are the method of conducting a study about a particular topic by collecting, organizing, interpreting, and finally presenting data. Statistical tools derived from mathematics are useful in processing and managing numerical data in order to describe a phenomenon and predict values. This module deals with the management of data on how to collect, organize, interpret, and present. You will be presented with exercises which you will analyze and answer so that you will appreciate more the importance of data management as you go through this module. STATISTICS It means “a collection of methods for planning experiments, obtaining data and then organizing, summarizing, analyzing, interpreting, presenting and drawing conclusions based on data.” DESCRIPTIVE STATISTICS It is a type of statistics that aims at summarizing and presenting data in the form which will make them easier to analyze and interpret. It includes tables, graphs, collection, extraction, summarization, presentation, measures of central tendency, variability, and location. INFERENTIAL STATISTICS It is a type of statistics that aims at drawing and making decisions on the population based on evidence obtained from a sample. It includes estimation and hypothesis testing. POPULATION It is the complete and entire collection of elements to be studied. SAMPLE It is defined as any subgroup of the population drawn by some appropriate method from the population. TYPES OF VARIABLES DATA are the values that the variables can assume while a variable is a characteristic of a population or sample which makes one different from the other. Variables can be classified into two – qualitative and quantitative variables. QUALITATIVE VARIABLE has values that are intrinsically non-numerical (categorical). These can be separated into different categories that are distinguished by some non-numeric characteristics. These are words or codes that represent a class or category, or these are attributes which cannot be subjected to meaningful arithmetic. QUANTITATIVE VARIABLE has values that are intrinsically numerical. It consists of numbers representing counts or measurements. These are numerical in nature and therefore meaningful arithmetic can be done. LEVELS OF MEASUREMENTS MEASUREMENT LEVELS It refer to different types of variables that imply how to analyze them. From low to high, these are nominal variables; ordinal variables; interval variables; ratio variables. The “higher” the measurement level, the more information a variable holds. NOMINAL It is characterized by that consist of names, labels, or categories only. ORDINAL involves data that may be arranged in some order, but differences between data values either cannot be determined or are meaningless. INTERVAL It is the same as the ordinal level, with an additional property that we can determine meaningful amounts of differences between the data. RATIO An interval level modified to include the inherent zero starting point is called Ratio level which is the highest of measurement levels. SAMPLING It is related to the selection of a subset of individuals from within a population to estimate the characteristics of the whole population. These processes of selection of subsets are called sampling techniques and these are grouped into two – probability sampling techniques and non-probability sampling techniques. SAMPLE SIZE Sample size are to be taken and these are the number of observations or individuals included in a study or experiment 𝑁 Slovin’s Formula which is denoted as: 𝑛 = 1 + 𝑁(𝑒)² where e = Margin of Error PROBABILITY SAMPLING These are techniques refers to a sampling method that uses some form of random selection. To have a random selection, one must set up some procedure that assures that the different units in the population have equal probabilities of being included. TYPES OF PROBABILITY SAMPLING SIMPLE RANDOM SAMPLING consists of choosing a sample from a set of all possible samples, giving each individual an equally likely chance of being the selected one. The following are different methods of using simple random sampling. a. LOTTERY METHOD/FISHBOWL METHOD is a method that is easy to carry out especially if both population and sample are small but can be tedious and time consuming for large populations or large samples. b. TABLE OF RANDOM NUMBERS is a set of random digits arranged in-groups both horizontally and vertically. c. RAN function of a calculator is a function in a scientific calculator that has the capacity to generate random samples. d. SOFTWARE refers to any program used to generate random samples using a computer (e.g. EXCELL, SPSS, SAS, among others). SYSTEMATIC RANDOM SAMPLING This method of probability sampling involves the selection of the desired sample in a list by arranging them systematically or logically in either alphabetical arrangement or any acceptable arrangement. It is a method of selecting a sample by taking every kth individual from the ordered population, where only the first individual was selected at random and the rest will be selected in a systematic manner. 𝑵 The formula for k is k = 𝒏 , where k = sampling interval, N = population size and n = sample size. STRATIFIED RANDOM SAMPLING The probability sampling in which the population is divided into a number of non-overlapping strata, so that the samples within a stratum are more or less homogenous and samples among strata are heterogeneous. After stratifying the population, random samples can be selected using either the simple random sampling or systematic random sampling. CLUSTER SAMPLING It is used when the population is very large and widely spread out over a wide range of geographical areas. In this method, the population is divided into clusters, which may not be on the same size. The random samplings (simple, systematic, and stratified) may be chosen to select the sample clusters. MULTISTAGE SAMPLING It is a sampling process that uses more than one kind of sampling. NON-PROBABILITY SAMPLING These are techniques refers to a sampling method which does not involve random selection, that is, the probabilities of selection are not specified for each individual in the population. TYPES OF NON-PROBABILITY SAMPLING ACCIDENTAL (HAPHAZARD) SAMPLING It refers to a non-probability sampling method wherein the samples are selected by chance or variability. For instance, a person who is obtaining opinions for a political poll at a shopping mall by randomly selecting passers-by. PURPOSIVE (JUDGMENTAL) SAMPLING It is done wherein the samples are chosen based on an expert’s opinion. For example, you may be conducting a study on why senior high school students choose public college over private university. You might canvas senior high school students and your first question would be “Are you planning to attend college?” People who answer “No,” would be excluded from the study. CONVENIENCE SAMPLING It is a sampling method wherein the samples are readily or easily accessible. For instance, an agricultural business student needs to get feedback on the “scope of content marketing in 2020.” The student may quickly create an online survey, send a link to all the contacts on your phone, share a link on social media, and talk to people you meet daily, face-to-face. QUOTA SAMPLING It is a non-probability sampling wherein the samples are chosen according to some fixed quota, whereby the concern is to come up with the required number of samples no matter how they are selected. For example, a researcher wants to survey individuals about what smartphone brand they prefer to use. He/she considers a sample size of 500 respondents. Also, he/she is only interested in surveying ten barangays in the Municipality of Magalang. Here’s how the researcher can divide the population by quotas: (a) Gender: 250 males and 250 females; (b) Age: 100 respondents each between the ages of 16-20, 21-30, 31-40, 41-50, and 51+; (c) Employment status: 350 employed and 150 unemployed people. (researcher may apply further nested quotas, like, out of the 150 unemployed people, 100 must be students.); and (d) Location: 50 responses per barangay. SNOWBALL SAMPLING OR CHAIN-REFERRAL SAMPLING It is defined as a non-probability sampling technique in which the samples have traits that are rare to find. This is a sampling technique, in which existing subjects provide referrals to recruit samples required for a research study. For example, if obtaining samples for a study that wants to observe a rare disease, the researcher may opt to use snowball sampling since it will be difficult to obtain samples. It is also possible that the patients with the same disease have a support group; being able to observe one of the members as your initial subject will then lead you to more subjects for the study. METHODS OF DATA COLLECTION There are different ways on how to collect data from your sample. The following are guides for collecting data. 1. INTERVIEW METHOD – the researcher’s direct and personal contact with the interviewee. 2. QUESTIONNAIRE METHOD – the researcher distributes the questionnaires either personally or by mail and collects them by the same process. 3. REGISTRATION METHOD – this method of collecting data is governed by our existing laws. Examples are PSA and COMELEC. 4. EXPERIMENTAL METHOD – it is used to find out the cause and effect relationship of certain phenomena under controlled conditions. 5. OBSERVATION METHOD – the researcher may observe subjects individually or group of individuals to obtain data and information related to the objectives of the investigation. 6. TEXTING METHOD – the researcher may ask or invite individuals to send text opinions, emails or google forms on certain issues or send their choices using their devices. PRESENTATION OF DATA PRESENTATION OF DATA It refers to the organization of data into tables, graphs, or charts, so that logical and statistical conclusions can be derived from the collected measurements. There are three ways to present data – textual, tabular, and graphical presentation. TEXTUAL PRESENTATION OF DATA In a textual presentation of data, the data is simply mentioned as mere text, that is generally in a paragraph. This is commonly used when the data is not very large. TABULAR PRESENTATION It refers to a method of presenting data consisting of columns and rows. When used alongside with textual form, the textual presentation or discussion must come either before or after the table. Pointers needed in the construction of table: A. Every table should be self-explanatory. B. Position the table after the text where it is first cited. C. Unit of measurement must be clearly stated. D. Show total, subtotals, percentages, and the like if necessary. E. Number of variables in a table must be at most three. F. Provide a source of the data when taken from another publication. GRAPHICAL PRESENTATION OF DATA GRAPH PRESENTATION It is a visual representation of data that can present complex information quickly and clearly and assist the reader to see patterns and trends in data. There are different kinds of graphs and charts and these are the following: BAR GRAPH These graphs are ideal for presenting categorical data. Bar graphs use rectangular bars to visually display each value and how it compares to other values in the graph – the greater the length of the bar, the greater the value. This provides a simple and easy way to interpret the data. Bar chart uses: I. When you want to display data that are grouped into nominal or ordinal categories. II. To compare data among different categories. III. Bar charts can also show large data changes over time. TAKE NOTE: Bar charts are ideal for visualizing the distribution of data when we have more than three categories. CIRCLE GRAPH/ PIE CHART These graphs are used to show a relationship of the parts to a whole. Percentages are used to show how much of the whole each category occupies, and it should be used for 3-7 categories only. Pie Chart uses: I. When you want to create and represent the composition of something. II. It is very useful for displaying nominal or ordinal categories of data. III. To show percentage or proportional data. IV. When comparing areas of growth within a business such as profit. V. Pie charts work best for displaying data for 3 to 7 categories. LINE GRAPH These graphs are used to illustrate trends over time for continuous data. They can also be used to compare two different variables over time. Uses of line graphs: I. When you want to show trends. II. When you want to make predictions based on a data history over time. III. When comparing two or more different variables, situations, and information over a given period of time. SCATTER PLOT These graphs are used to plot data points on a horizontal and a vertical axis to show relationships between two variables (or how much one variable is affected by another). They are particularly useful when comparing two continuous variables in situations where there are many data points, the measurement intervals on the x and/or y-axis may be uneven, and/or the reader is looking for trends and groupings in the data. HISTOGRAM In a histogram, the data are grouped into ranges (e.g. 10–19, 20–29) and then plotted as connected bars. Each bar represents a range of data. A histogram looks remarkably similar to a bar chart except that the bars are touching and may not be of equal width. In a bar chart, the spaces between the bars visually indicate that the categories are separate. Histogram uses: I. When the data is continuous. II. When you want to represent the shape of the data’s distribution. III. When you want to see whether the outputs of two or more processes are different. IV. To summarize large data sets graphically. V. To communicate the data distribution quickly to others. SHAPES OF HISTOGRAM SYMMETRIC Histogram is symmetric around its location since the densities are the (almost) same for any two intervals that are equally distant from the center. A distribution is said to be symmetric about the mean if the distribution to the left of the mean is the “mirror image” of the distribution to the right of the mean. A histogram with symmetrical in shape is said to be Normally Distribution or in Bell-shaped curve. SKEWNESS It refers to how asymmetric the shape of the distribution is. That is, are there more extreme values out to the right or left. More specifically, we call them left-skewed if they are stretched to the left, or right- skewed if they are stretched to the right. KURTOSIS It describes the extent of peakedness or flatness of the distribution of the data. MEASURES OF CENTRAL TENDENCY It is the summary measure that describes a whole set of data with a single quantity that represents the middle or center of its distribution the way in which a group of data clusters around a central value. The measures of central tendency are the mean, median and mode. MEAN It is the most commonly used measure of central tendency. To find the mean for a set of data, find the sum of the data values and divide by the number of data values. MEDIAN It is the middle number or the mean of the two middle numbers in a list of numbers that have been arranged in numerical order from smallest to largest or from largest to smallest. Any list of numbers that is arranged in numerical order from smallest to largest or from largest to smallest is a ranked list. MODE It is a list of numbers is the number that occurs most frequently. The mean, the median, and the mode are all averages; however, they are generally not equal. The mean of a set of data is the most sensitive of the averages. A change in any of the numbers changes the mean, and the mean can be changed drastically by changing an extreme value. In contrast, the median and the mode of a set of data are usually not changed by changing an extreme value. When a data set has one or more extreme values that are very different from the majority of data values, the mean will not necessarily be a good indicator of an average value. MEASURES OF VARIABILITY Measures of variability known as the range and standard deviation. VARIABILITY refers to how spread apart or dispersed the values of the distribution are or how much the values vary from each other. RANGE It is the difference between the highest value and lowest value. It is the simplest measure of variability. The range of a set of data is easy to compute, but it can be deceiving. The range is a measure that depends only on the two most extreme values, and as such it is very sensitive. But it suggests that a much larger range suggests greater variation or dispersion. STANDARD DEVIATION (SD) A measure of dispersion that is less sensitive to extreme values is the standard deviation. The standard deviation (SD) of a set of numerical data makes use of the individual amount that each data value deviates from the mean. It is determined by calculating the positive square root of variance. It is a measure of dispersion of a set of data from its mean. CORRELATION It is a statistical method used to determine if there is a relationship or association between variable and the strength of the relationship. The measure of the degree of correlation is known as the correlation coefficient 𝑟 or the Pearson Product Moment Correlation or simple called as the Pearson 𝑟. The range of values of the correlation coefficient is from – 1 to + 1. LINEAR REGRESSION REGRESSION ANALYSIS It is the process of formulating a mathematical model that can be used to predict or determine one variable by another variable/s. In LINEAR REGRESSION, only a straight-line relationship between the two variables is examined. CHAPTER 5: GRAPH THEORY GRAPH A graph is a set of points called vertices and line segments or curves called edges that connect vertices. TAKE NOTE: Vertices are always clearly indicated with a “dot.” Edges that intersect with no marked vertex are considered to cross over each other without touching. CONNECTED GRAPHS graphs in which any vertex can be reached from any other vertex by tracing along edges. Otherwise, it is DISCONNECTED GRAPH. NULL GRAPH a graph that has no edges. MULTIPLES EDGES two or more edges that connect the same vertices. LOOP edges that loop back to the same vertex. COMPLETE GRAPH is a connected graph in which every possible edge is drawn between vertices (without any multiple edges). EQUIVALENT GRAPH are graphs where the edges form the same connections of vertices in each graph. PATH it is a movement from one vertex to another by traversing edges. CIRCUIT or CLOSED PATH a path that ends at the same vertex at which it started. DEGREE the number of edges that meet at a vertex. EULER CIRCUIT a circuit that uses every edge, but never uses the same edge twice. EULERIAN GRAPH THEOREM A connected graph is Eulerian if and only if every vertex of the graph is of even degree. EULER PATH a path (not necessarily a circuit) that uses every edge once and only once. HAMILTONIAN CIRCUIT is a path that uses each vertex of a graph exactly once. A graph that contains a Hamiltonian circuit is called Hamiltonian. PLANAR GRAPH (PLANARITY) a planar graph is a graph that can be drawn so that no edges intersect each other (except at vertices). If the graph is drawn in such a way that no edges cross, we say that we have a planar drawing of the graph. FACES in a planar drawing of a graph, the edges divide the graph into different regions. INFINITE FACE the region surrounding the graph, or the exterior, is also considered a face. EULER’S FORMULA In a connected planar graph drawn with no intersecting edges, let v be the number of vertices, e the number of edges, and f the number of faces. Then v + f = e + 2 FOUR-COLOR THEOREM It states that every planar graph is 4-colorable. GOOD LUCK ON YOUR FINAL EXAMINATION!