Game Theory Maxmin Strategies PDF
Document Details
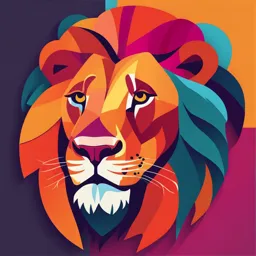
Uploaded by DeadCheapGuqin685
Tags
Summary
This document discusses maxmin and minmax strategies in game theory, focusing on their application to zero-sum games. It explains the concept of maximizing one's own payoff while minimizing the other player's gain. The text also considers the graphical representation of strategies and provides an example of a penalty kick game to illustrate the application of these strategies.
Full Transcript
We will now speak about Maxmin Strategies. These make particular sense in the context of zero sum games but actually are applicable quite to, to all games. What is a maxmin strategy? It's simply put; a player's strategy that maximizes their pay off assuming the other player is out to get them. we wi...
We will now speak about Maxmin Strategies. These make particular sense in the context of zero sum games but actually are applicable quite to, to all games. What is a maxmin strategy? It's simply put; a player's strategy that maximizes their pay off assuming the other player is out to get them. we will def, we will concentrate primarily on the two player case here, again because when we get to zero sum games, those really make only sense in the case of two players. but keep in mind that one could define this kind of more generally when we speak about maxmin strategy. So the maxmin strategy is a strategy that maximizes my worst case outcome and my maxmin value or, safety level is that payoff that's guaranteed by the maxmin strategy. And here it is defined formally, maximum strategy for player i is the strategy s 1 that maximizes the minimum that the other player, remember the minus i, the player other than i would hold player one down to. And the maximum value is defined similarly to be the value of that maxmin strategy. Now, why, why you would you want to think about the maxmin strategy? one can think of it either as a simply as sort of a certain cautionary, maybe the other people will make some mistakes or not act in their own best interests. maybe, I'm not sure exactly what their payoffs are, they're up for interpretation, though you can simply be paranoid. about about, about them and think that they're out to get you and you know the saying, you know even the paranoids have enemies. That's the maxmin strategy and, just to confuse things, we'll also speak about the minmax strategy. The minmax strategy is strategy against, if you wish, the other player in the two player game, is the strategy that minimizes their payoff, on the assumption that they're trying to maximize it. And so here is the formal definition, the minmax strategy for player i is playing against the other guy we denote by minus i, is the strategy that minimizes the maximum pay-off as attempted by the other guy of the payoff to the other guy. And the minmax value is simply the value of that minmax strategy, the value to play one. Now, why, why would, player one, want to, want to har, harm the other guy? Well, you could, you could just, be out to get him that, that's a possibility or, they could be playing a zero sum game. And in a zero sum game hurting the guy is tantamount to improving your own your own payoff. And so in, in the setting of zero-sum games, maxmin and minmax strategies make a lot of sense and in fact, in a very famous theorem, due to John von Neumann. it proved that in a zero-sum game, by definition, you consider only two player such games. In any Nash equilibrium the players sees a payoff that's equal to both his maxmin value and his minmax value. And and that means that so we'll call it the value of the game, the value for player one called the value of the game that means that the, the set of maxmin strategies are really the same as the set of the minmax strategies. That is, trying to improve your worst case situation is the same as trying to minimize the other guy's best case situation. And any maxmin strategy profile or min max strategy profile, because they're the same constitute a Nash equilibrium and furthermore there's order in the Nash equilibrium that exists. And so the payoffs in all Nash equilibrium is the same mainly the value of the gain. One way to get a concrete feel for it is graphically and here is again a matching pennies. This is a game where you, each of us chooses heads and tails on probability, and if we if it comes up either if we, both, if, if, if, if the result of our randomization I end up choosing head, and you end up playing tail, you win and con, and vice versa, if I chose tail and you head. But if we both chose head, or both chose tail, I win and so here are the payoffs. you see here the strategy spaces this is player 2 is kind of increasing the probability of playing heads. And this is player one and, with this dimension, you have the value of the game with payoff to player one. And the only Nash equilibrium is for both to randomize, 50/50, it's just right here, and it's conveniently located by slicing the the three-dimensional structure in this way. And you sort of see that it's got to be an equilibrium in the sense that player, player 1 could be moving along this this curve. But as he does it his payoffs would only drop and so he's trying to maximize the value you would do it, and conversely player two can only traverse around, along this but if he does that the chaos would only increase. And he's trying to minimize the value so so so, you get a, a stable point which for obvious reasons is called a saddle point. So although our general purpose procedures for finding Nash equilibrium in particular in in 2 by 2 games, we can use the, the maxmin, definition, to, to find it, directly in zero-sum games. And, the, let's see how it, how it happens in the, game we'll call the, the penalty kick game. So in this game, we have a, a, a, penalty kicker and a goalie it's a zero sum game and the goal of the, kicker is to score a goal. And the goal, the goal of the goalie, is to, prevent it. And let's assume that they each have two strategies. Kick to the left and kick to the right for the kicker, and jump to the left and jump to the right for the goalie. The payoffs will be the, each of them will determinate probability of the the kicker scoring a goal. And we'll have that probability being the pay off, the value of the game. This is the payoff to player one, and therefore minus the pay-off to player two, namely the goalie. So here we are, so for example, if the kicker kicks left and the goalie guesses correctly and jump left also. Then the goalie has not too bad a change of stopping the shot namely, probably, 0.4. If he jumps to the wrong side, his probability of stopping it, is much lower, it's 0.2, similarly, if the, kicker decides to kick to the right. If the goalie guesses wrong, his probability is low, even lower than if he guessed wrong in the other case. And if he guesses right his probability is higher, although not quite as high had he guessed right in the left case. So he's, he's better at, stopping, shots when the kicker kicks to the left. So, how does the kicker, maximize his minimum? That's what we're after. So, here is the expression, right? we want the maxmin value of the following. So they each have some mixed strategy of playing left and right with some probability, so s1 L is the probability that the kicker kicks to the left, and s2 L is the probability that the goalie jumps to the left and as we saw in that case, the value is 0.6. And similarly, the value of 0.8 is, if we end up in this situation, 0.9, and 0.7 in these situations. Okay? So this is expression that we somehow need to compute. So, what is the minimum that the kicker should keep in mind? So, the kicker says, I'm going to pick, I'm going to play my strategy s1, whatever it is. When I play it, player 2 is going to play s2 as to minimize my payoff. So let me write down this entire expression, and I will just, this is simply just copying it over, replacing s, expressed in such as, s1 R by 1 minus s1 L, that's all it's doing and the same, same for s2 R. And so we have this is our expression, and player 1 is saying to himself, what do player 2 do if I were to play my strategy s 1. And this is simply rearranging the terms, nothing else going on here, as a function of s 2 L because player one says, I'm going to, I'm going to pick s 1. What would s 2's be best response, namely its minimum? And so this is arranging it as a function of s 2 strategy. And now all that remains is to look for the minimum of the strategy. And the minimum is taken by, look, taking first derivative in respect to s 2, holding s 1 as a constant and so we have this expression. And then we solved for for s1, and we get that s1, of L is, is half, and therefore s1 of R is half as well. And so by this maxmin calculation, we see that player, or the kicker figures out that in equilibrium they'd better randomize half-half between left and right. What does what does the goalie kind of figure out? Well, he's trying to minimize the kicker's maximum, right? That's one way to look at it, he's doing the min max strategy. And so, here is the min max value for player two, just writing it down. And as before, we'll simply, we'll simply, rewrite, s1 of r as 1 minus s1 of l, and so on, for, s2 of r. And we'll rearrange the term, this time as a function of s1, s1 of L because player 2 is saying player, player 1, player 2 says player 1 is, whatever I choose namely s2, player one will want to best responding to maximize. So if you write it down as a function of s1's choice and now figure out what the maximum would be. Well, here's the maximum and when you solve for L2 now, you get that the randomization in equilibrium for press two for pair two is a quarter and three quarters. So this illustrates how we can use the max min theory to actually compute the the the Nash equilibrium zero sum games atlest two by two games. In general, we can use the min max theorem to compute the equilibria of the zero sum game. And we do it by simply laying out a linear program that captures the game and here it is. So U1* is going to be the value of the gain, that is payoff to player one in equilibrium and so we're going to specify from players two's point of view, we could have done it the other way around also. So what player two is saying is simply, says, for each of the actions of player one, each action that player one might consider, I want to find, a mixed strategy s2. So here's my mixed strategy s2, it will look at all my pure strategies k, and make sure that the probability that, this is the probability distribution over the sum, say sum to one and they're non negative. So what I'd like to do is that the best response to my strategy by player one for any of these actions will never exceed this value of the game because I'm trying to minimize it, so I'm going to find the lowest view that has a property. That player 1 doesn't have a profitable deviation by any of his of his of his pure strategies, so when I look at the payoff for player 2 when I play a 2 k and he plays a 1 j, that j that I'm considering right now, and I multiply the probability of, in my mixed-strategy playing a 2 k, I don't want that other player, player 1, to have a profitable deviation. So it's gotta be that the expected payoff will be no greater than the value U1 star. So, clearly this is a correct formulation of the game, and it is a linear program. As we know, linear programs are efficiently solvable. In theory, by interior methods that is provably, but no real in practice by procedures that are worst case exponential but in practice work well.