Insights & Big Data Foundations PDF
Document Details
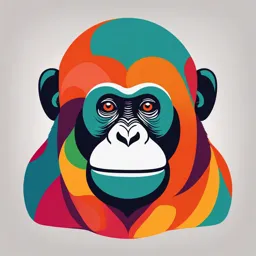
Uploaded by CheeryAstrophysics9707
KEDGE Business School
2024
Jana Gross
Tags
Summary
This document, from KEDGE Business School, provides a foundation of big data. It covers definitions of big data and data-driven marketing, including its characteristics and examples of implementation. The presentation is designed for students of business and marketing at a postgraduate level.
Full Transcript
Insights & Big Data Prof. Dr. Jana Gross 2024-2025 Prof. Dr. Jana Gross 1 Foundations of big data Agenda Foundations of big data 1 Definition of data-driven marketing 2 Definition of big data 3 The five/eight “Vs” of big data 4...
Insights & Big Data Prof. Dr. Jana Gross 2024-2025 Prof. Dr. Jana Gross 1 Foundations of big data Agenda Foundations of big data 1 Definition of data-driven marketing 2 Definition of big data 3 The five/eight “Vs” of big data 4 Creating value of big data in marketing 5 Opportunities and challenges with big data in marketing © Prof. Dr. Jana Gross 3 Definition of data-driven marketing Data-driven marketing Big data Insights drawn from data Big data is collected, are used for improved analyzed, and used for decision marking. marketing purposes. Decision making is based Insights & decision on evidence and facts from making data. Data-driven marketing is a marketing approach of using big data to improve marketing processes. © Prof. Dr. Jana Gross 4 Definition of data-driven marketing Insights & Big data decision making Increase efficiency Increase performance Data collection Improve customer Data preparation retention and loyalty Data storage Reach customers Data analytics more efficiently more Social listening and monitoring more © Prof. Dr. Jana Gross 5 Definition of data-driven marketing Example of data-driven marketing communication © Prof. Dr. Jana Gross 6 Source: Disney (2022) Definition of data-driven marketing Example of data as a product © Prof. Dr. Jana Gross 7 Source: https://www.livingwinelabels.com Definition of data-driven marketing Example of shopping in the metaverse © Prof. Dr. Jana Gross 8 Source: Walmart (2022) The rise of data-driven marketing © Prof. Dr. Jana Gross 9 Source: https://mediapeanut.com/how-fast-is-technology-growing-statistics-facts/ Process of data flow in companies Business Intelligence Data Data Data Data Data generation & collection warehousing analytics visualizations reporting CRM / CE Google Data Studio © Prof. Dr. Jana Gross 10 Foundations of big data Holistic definition of big data Big data is a cultural, technological, and scholarly phenomenon that rests on the interplay of: Technology: The ability to increase computation power and algorithmic accuracy. Analysis: The ability to draw on large data sets. Mythology: The belief that large data sets offer a higher form of intelligence and knowledge. © Prof. Dr. Jana Gross 11 Foundations of big data Broad parameters of big data Data types: The ability to capture and integrate different data types into a comprehensive and readily accessible source. Data sources: Systems that have the ability to handle and integrate diverse sources of data. Data volume: Hardware and software systems with the ability to store, process, analyze, display, and evaluate data in a given organization. Processing strategies: Statistical tools and techniques that can reveal patterns and insights that can yield rich benefits to an organization. © Prof. Dr. Jana Gross 12 Foundations of big data Data Big Data Data is a set of qualitative or Data that is so complex that quantitative variables about one Definition or more individuals and/or traditional techniques and methodologies cannot handle it. objects. String Unstructured data Integer Structured data Types Float Semi-structured data Character Internal data Boolean External data Metrics that focus on the Value Volume behavior of the individual Veracity Characteristics customer in the relationship with Velocity Validity Vision Variety the company. Visibility © Prof. Dr. Jana Gross 13 Foundations of big data Example of big data in practice © Prof. Dr. Jana Gross 14 Source: Google (2024) Big data characteristics Volume Volume refers to Velocity refers to the volume of the frequency of Velocity data generation data generation and/or delivery. and/or delivery. Value refers to Big Data Variety refers to value that the complexity of Value without big data Variety data generation would simply not and/or delivery. be possible. Veracity refers to the messiness and trustworthiness of data. Veracity © Prof. Dr. Jana Gross 15 Big data characteristics Volume Volume refers to the volume of data generation and/or delivery. Benefit: More holistic understanding of customers and marketing Challenge: Need for models that are scalable Velocity Velocity refers to the frequency of data generation and/or delivery. Benefit: Rapid and instant generations of data, (virtually) real-time marketing decision making Challenge: Speed of data analytics to add value and offer a competitive advantage © Prof. Dr. Jana Gross 16 Big data characteristics Variety Variety refers to the complexity of data generation and/or delivery. Benefit: Combining and analyzing data from multiple sources to create value Challenge: Statistical tools and techniques to draw valuable insights from different types and formats of data Veracity Veracity refers to the messiness and trustworthiness of data. Benefit: Higher data quality, reducing wrong insights and poor decision-marking Challenge: Capacity of verifying data on an ongoing basis © Prof. Dr. Jana Gross 17 Big data characteristics Value Value refers to value that without big data would simply not be possible. Benefit: Significant competitive advantage Challenge: Subjective and time-dependent, function of the preferences and shifting needs of customers Validity Validity refers to the integrity and accuracy of the data being used to derive insights. Benefit: Higher data quality and reliability Challenge: Requirement of business and data knowledge © Prof. Dr. Jana Gross 18 Big data characteristics Visibility Visibility refers to data that an authorized user can locate, access, process and secure in a reliable and timely manner. Benefit: Valuable intelligence and insights Challenge: Visibility at the right time, in the right way, to the right user Vision Vision refers to an organization's most visible and valuable roadmap for its future. Benefit: Increases the chances of success for big data projects Challenge: Collective journey that all stakeholders must undertake © Prof. Dr. Jana Gross 19 Big data characteristics © Prof. Dr. Jana Gross 20 What big data is and what it is not Big data does not have a consistent and concise definition. Data that is so complex that traditional techniques and methodologies cannot handle it. Big Data refers to the ability to collect, structure, analyze, and process data from a wide variety of sources. Data with greater volume, velocity, variety, value, veracity (validity, visibility, and vision). Big Data is less about data that is big than it is about the capacity to search, aggregate, and cross-reference large data sets (Boyd and Crawford 2021). At the end, big data is just data. © Prof. Dr. Jana Gross 21 Value creation of big data strategies For companies to successful adopt big data, they need: to embrace big data and be open to rely more on analytics in their marketing decision making. an understanding of how to manage data that is already available. a cultural shift towards an evidence-based decision making. Value is created on a market, brand/product, and customer level. © Prof. Dr. Jana Gross 22 Source: Kooge et al. (2016) Value creation of big data strategies Value-to-customer Value-to-firm (V2C) (V2F) Creating value to Extracting value from customers customers Examples Examples High quality Higher price premium Attractive brand propositions Lower churn rates Appropriate customer Higher revenues/customer relationships Stronger customer advocacy © Prof. Dr. Jana Gross 23 Source: Kooge et al. (2016) Value creation of big data strategies Value-to-firm (V2F) Value-to-customer (V2C) © Prof. Dr. Jana Gross 24 Source: Kooge et al. (2016) Value-to-customer (V2C) and Value-to-firm (V2F) Value-to-customer (V2C) metrics V2C metrics focus on the delivered value to customers What companies achieve in a customer's mind Whether these achievements results in positive cognitive and affective responses Value-to-firm (V2F) metrics V2F metrics focus on the delivered value by customers to companies Often of behavioral and financial nature © Prof. Dr. Jana Gross 25 Source: Kooge et al. (2016) Value-to-customer (V2C) Value-to-customer Example of a big data (V2C) metric Analysis of online Metrics that focus on conversation on products and Market level products and its usage their usage on Amazon Reviews Sentiment analysis of online Metrics that focus on brand conversations on brands on a Brand level performance outcomes companies' social media fanpages Metrics that focus on Analysis of online customer Customer level customer feedback and reviews on TripAdvisor experience © Prof. Dr. Jana Gross 26 Source: Kooge et al. (2016) Value-to-firm (V2F) Value-to-firm Example of a big data (V2F) metric Metrics that focus on the Market size metrics Market level attractiveness of the market Market attractiveness metrics Brand market performance Metrics that focus on the Brand level performance of the brand metrics Brand valuation metrics Metrics that focus on the CLV behavior of the individual Customer level customer in the relationship Customer engagement Digital customer journey with the company © Prof. Dr. Jana Gross 27 Source: Kooge et al. (2016) Value creation of big data strategies Value creation requires data capabilities People, systems, processes, organizations Opportunities Creation of important new insights Development of more effective marketing campaigns Development of big data-based customer solutions Challenges Dominant role of IT and CRM implementation Data privacy and security Creating value from big data © Prof. Dr. Jana Gross 28 Source: Kooge et al. (2016) Value creation of big data strategies Analytics focus on on gaining insights Analytics aim to develop models to improve decision making Insights and models create values for companies in the following ways: Decision support for marketing Improve actions and campaigns Information-based products and solutions © Prof. Dr. Jana Gross 29 Literature Kooge, E., Walk, N., and Verhoef, P.C. (2016). Creating Value with Big Data Analytics: Making Smarter Marketing Decisions. Bernard Marr (2016). Big Data in Practice: How 45 Successful Companies Used Big Data Analytics to Deliver Extraordinary Results, WILEY Edition. Bernard Marr (2015). Big Data: Using SMART Big Data, Analytics and Metrics To Make Better Decisions and Improve Performance. Knaflic, C.N. (2015). Storytelling with Data: A Data Visualization Guide for Business Professionals. Sherman, R. (2014). Business Intelligence Guidebook: From Data Integration to Analytics. Sathi, A. (2014). Engaging Customers using Big Data: How Marketing Analytics are Transforming Business. Barnes, S. B. (2006). A privacy paradox: Social networking in the United States. First Monday. Barth, S., & De Jong, M. D. (2017). The privacy paradox–Investigating discrepancies between expressed privacy concerns and actual online behavior–A systematic literature review. Telematics and informatics, 34(7), 1038-1058. Gupta, U.G., and Gupta, A. (2016). Vision: A missing key dimension of the 5V Big Data framework. Journal of International Business Research and Marketing. 1(3): 46-52. © Prof. Dr. Jana Gross 30