INF411 Business Intelligence and Analysis Final Exam Material PDF
Document Details
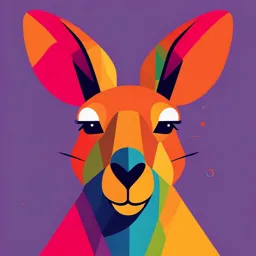
Uploaded by ManageableBowenite695
Dr. Ahmed Youssef
Tags
Summary
This document is a presentation outlining the content of the INF411 Business Intelligence and Analysis course. It discusses topics like motivation, course objectives, and various business intelligence techniques.
Full Transcript
INF411 Business Intelligence and Analysis COMPUTER ASSISTED APPLICATIONS FOR REPORTING AND VISUAIZAATION INTRODUCTION TO DECISION SUPPORT AND BUSINESS INTELLIGENCE PRESENTED BY: DR. AHMED YOUSSEF Motivation Market Basket Analysis How Target Figured Out A Teen Girl Was Pr...
INF411 Business Intelligence and Analysis COMPUTER ASSISTED APPLICATIONS FOR REPORTING AND VISUAIZAATION INTRODUCTION TO DECISION SUPPORT AND BUSINESS INTELLIGENCE PRESENTED BY: DR. AHMED YOUSSEF Motivation Market Basket Analysis How Target Figured Out A Teen Girl Was Pregnant Before Her Father Did (forbes.com) What do computers and phones know about you? Motivation Main From data to decision objective Motivation & intro كيف نجحت الصين في النجاة؟YouTube Overall aims of course The aim of this course is to Educate students on the foundational theories and practical skills in business intelligence and decision support systems. This includes understanding data warehousing, ETL (Extract, Transform, Load) processes, and OLAP (Online Analytical Processing). Provide hands-on experience in designing and constructing a business intelligence system. This system will integrate complex, multi-agency databases, allowing students to apply BI concepts and data management techniques in a practical setting. Introduce students to advanced topics such as data, text, and web mining, artificial intelligence, and expert systems. The course will also cover the impact of BI on business process improvement and explore case studies that illustrate deriving business value from BI implementations. Address the managerial challenges associated with implementing BI systems, including the strategic decision-making process and the adoption of open-source BI tools like Pentaho. This aims to prepare students to overcome common obstacles and successfully lead BI projects in diverse organizational environments. Text books Ho to use this slides Each slide theme represent some books WHAT IS Definitions BI , DA DB, IS Youssef Tawfik – Business Intelligence Analyst - YouTube Business Intelligence: What is Business Business refers to an organization or entity involved in commercial, industrial, or professional activities. These activities like production, buying and selling of goods or services etc. Businesses can be for-profit entities aiming to generate financial gain, or non-profit organizations focused on social or charitable purposes. Business Intelligence (BI) Overview Organizations need to analyze large amounts of data for decision-making. BI helps convert data into actionable insights. BI is essential for: o Tracking performance o Identifying problems o Increasing profits o Forecasting market needs and competition What Is Business Intelligence? – [Broad Definition] – An umbrella term that combines architectures, tools, databases, analytical tools, applications, and methodologies for Reporting decision support. – A broad term that encompasses the strategies, technologies, and practices used by organizations to collect, analyze, and interpret data to gain valuable insights that inform business decisions. – [Narrow Definition] – Descriptive analytics tools and techniques – (i.e., reporting and visualization tools) What Is Business Intelligence? Methods used by the organization to Turn data into knowledge. Turn data into actionable insights Using data to understand what’s happening in the business (e.g., who, how, when, and why people interact with it). How you can turn data into knowledge? Business Intelligence Systems I/O Business Intelligence Systems I/O where OLAP = On-Line Analytic Processing, DW=Data Warehouse, DM=Data Mining, EIS = Executive Information Systems, and ERP = Enterprise Requirement Planning. Systems Prior to BI Evolution of Computerized Decision Support to Business Intelligence, Analytics, Data Science Evolution of Computerized Decision Support to Business Intelligence, Analytics, Data Science Applications of BI (example) Applications of BI (example) Customer Analytics Human Capital Productivity Analysis Business Productivity Analytics Sales Channel Analytics Supply Chain Analytics Behavior Analytics And more…….. Business Value of BI Analytical Applications Applications of BI (example) Analytic Application Business Question Business Value Customer What market segments do my Personalize customer relationships for higher segmentation customers fall into, and what are their satisfaction and retention. characteristics? Propensity to buy Which customers are most likely to Target customers based on their need to increase respond to my promotion? their loyalty to your product line. Also, increase campaign profitability by focusing on the most likely to buy. Customer What is the lifetime profitability of my Make individual business interaction decisions profitability customer? based on the overall profitability of customers. Fraud detection How can I tell which transactions are Quickly determine fraud and take immediate likely to be fraudulent? action to minimize cost. Customer attrition Which customer is at risk of leaving? Prevent loss of high-value customers and let go of lower-value customers. Channel What is the best channel to reach my Interact with customers based on their optimization customer in each segment? preference and your need to manage cost. Who Uses Business Intelligence? BI is used by various roles within an organization, including: Executives: Use BI to gain insights into overall business performance, track key metrics, and make strategic decisions. Managers: Utilize BI to monitor departmental performance, identify areas for improvement, and make data-driven operational decisions. Analysts: Use BI tools to analyze data, generate reports, and identify trends to support decision-making across the organization. Sales and Marketing Teams: Leverage BI to understand customer behavior, target marketing campaigns more effectively, and improve sales forecasting. Benefits of Business Intelligence ? Some Benefits of Business Intelligence: Improved Decision-Making: BI empowers businesses to make data-driven decisions based on insights gleaned from historical data and current trends. This can lead to better resource allocation, optimized marketing campaigns, and overall improved business strategies. Enhanced Efficiency: BI automates data analysis tasks, freeing up valuable time and resources that can be directed towards other areas. It also streamlines processes by providing a centralized view of data, eliminating the need for manual data gathering and reconciliation. Increased Productivity: By providing easy access to relevant data through reports and dashboards, BI allows employees to work more productively and focus on higher-value activities. Reduced Costs: Data-driven decision-making can help businesses identify areas for cost savings and optimize resource allocation. Additionally, BI can help prevent fraud and waste by providing better visibility into financial activities. Competitive Advantage: Organizations that leverage BI effectively gain a competitive edge by understanding their customers, market trends, and internal performance better. This allows them to adapt to changing market conditions and deliver superior products and services. Benefits of Business Intelligence: Faster reporting, analysis or planning Improve customer relationships & loyalty More accurate reporting, analysis or Increased competitive advantage planning Reduced costs Improved data and Knowledge management Increase revenue and profit Improved data quality Overcoming cognitive limits in processing Better business decisions with greater speed and storing information and confidence Align effort with firm strategy Improved employee satisfaction Recognize and maximize firm’s strengths Improved customer satisfaction Shorten marketing efforts BI development Stages Business Intelligence (BI) Career Roadmap Business Intelligence (BI) Career Roadmap (youtube.com) BI Process Stages 1. Data Modeling 3. Data Analysis o Data Architect designs the Data Warehouse. o Analyst analyzes data to support business decisions. o Data from multiple sources (databases, files, APIs) is o Methods include: structured. ▪ Text Analysis (Data Mining) o Common tools: Azure Synapse, SQL Server, Redshift, Oracle. ▪ Statistical Analysis (Descriptive/Inferential) o Requires advanced SQL skills. ▪ Diagnostic Analysis ▪ Predictive Analysis 2. Data Integration ▪ Prescriptive Analysis o Data Engineer extracts, transforms, and loads (ETL/ELT) data. o Tools: Excel, SAS, Azure Analysis Services, SQL Server. o Ensures data is suitable for the Data Warehouse. 4. Data Visualization & Reporting o Common tools: Azure Data Factory, Talend, Databricks. o Data Visualization Developer presents analyzed data. o Programming skills: SQL, Python, Java, C++, etc. o Formats: Detailed reports, charts, interactive dashboards. o Tools: Power BI, Tableau, Cognos, Excel. The BI Process 1. Data Acquisition: The first step involves collecting data from various internal and external sources. This may include sales figures, customer information, marketing campaign data, social media data, and financial records. 2. Data Integration: The collected data may be in different formats and reside in various systems. BI tools integrate this data into a centralized repository for analysis. 3. Data Cleaning and Transformation: Data may contain errors or inconsistencies. BI processes clean and transform the data to ensure accuracy and consistency for analysis. 4. Data Warehousing: A data warehouse is a central repository that stores historical data from various sources, enabling analysis of trends over time. 5. Data Analysis: Once the data is prepared, BI tools are used to analyze it. This may involve techniques like data visualization, statistical analysis, and online analytical processing (OLAP) for multidimensional analysis. The 5 Stages of BI development BI development typically follows the 5 stages listed below: 1. The Data: defining which data will be loaded into the system and analyzed. 2. The ETL (Extract, Transform, and Load) Engine: moving the source data to the Data Warehouse. This can be a complex step involving modifications and calculations on the data itself. 3. Data Warehousing: connects electronic data from different operational systems so that the data can be queried and analyzed over time for business decision making. 4. Analytic Engine: analyzes multidimensional data sets found in a data warehouse to identify trends, outliers, and patterns. 5. Presentation Layer: dashboards, reports and alerts that present findings from the analysis Phases in the development of a business intelligence system Business intelligence architecture components BI Devices and Technologies BI professionals use a range of software tools rather than specialized hardware. Common BI Tools (types) Data Visualization Tools: These tools help create charts, graphs, and other interactive and visually appealing dashboards of data to make it easier to analysis and understand trends and patterns. Examples: Tableau: Known for its ease of use, user-friendly data visualizations, Power BI: Microsoft's competitor to Tableau, offering robust features for data visualization. QlikView Reporting Tools: These tools allow users to generate reports that summarize key business metrics and performance indicators. (Examples: Microsoft Power BI, Jaspersoft, SAP Crystal Reports) Data Mining Tools: These tools uncover hidden patterns and relationships within large data sets. (Examples: RapidMiner, KNIME, SAS Enterprise Miner) Online Analytical Processing (OLAP) Tools: These tools enable multidimensional analysis of data, allowing users to drill down into specific details and analyze data from different perspectives. (Examples: Microsoft Analysis Services, IBM Cognos Analysis) Common BI Tools Excel revolutionized data interaction by allowing users to create and manage their own datasets. Despite its widespread use, Excel has limitations when handling large datasets, requiring the use of more powerful tools for BI analysis. Databases are essential for managing large amounts of data efficiently. BI professionals often use SQL (Structured Query Language) to interact with databases. Knowing SQL can make working with data visualization tools easier. Common BI Tools ( brands) Microsoft: Excell, Power BI, SQL Server, Azure. Amazon: Redshift, AWS Databricks. IBM: Cognos Analytics, DB2 Warehouse. Others: Tableau, Qlik, PostgreSQL, Teradata. How BI Tools Work (Lab) Both Tableau and Power BI connect to databases (such as SQL) and Excel files to pull data. After gathering data, they visualize it using charts, graphs, and dashboards. Tableau Workflow Example: 1.Open Tableau on your desktop. 2.Connect to a SQL database to access data. 3.Create visualizations. 4.Publish the dashboard to a server for users to access via a web browser. Key Roles in BI Key Roles in BI Data Modeler/Architect: Designs data structures. Data Engineer: Integrates data. Data Analyst: Analyzes and interprets data. Analyzes collected data to predict trends. Data Visualization Developer: Presents data in a user-friendly format. Database Administrator (DBA): Designs back-end data warehouses. SQL Developer: Works with SQL (Structured Query Language) to manage and query databases. SQL Reporter: Develops reports and dashboards from SQL queries. Key Roles in BI (BI Engineers vs BI Developers) BI Developers: BI Developers vs BI Engineers: Skill Sets o Create dashboards that visualize data (e.g., pie BI Developers: charts, line charts). o Front-end role: analyzing data and presenting o Make data easy to understand, interactive, and trends clearly. accessible. o Focus on visualization and user interaction. o Users can answer their own questions through interactive dashboards. BI Engineers: BI Engineers: o Back-end role: understanding business rules and organizing data. o Focus on organizing and collecting data from various sources. o Focus on data collection, cleaning, and standardization. o Standardize data to make it usable for BI Developers. o Ensure that different data formats are unified into a central place. Key Takeaways BI involves multiple stages, each requiring specialized tools and skills. Professionals should be proficient in at least one tool per stage. Collaboration between different BI roles ensures effective decision-making. the use of data to manage day-to-day operational management within a business. Leaders employ business intelligence tools and experts when they want to collect and house data about current operations, maximize workflow, produce informative reports, and achieve their current business goals. Conclusion Business BI tools can include a variety of software tools and other systems. Some of these include spreadsheets, online analytical processing, reporting software, business activity monitoring software, and Intelligence data mining software. Some experts would also argue that business intelligence tools also include the more predictive and statistical tools used in business analytics. Overall, BI helps leaders navigate organizational and industry-related challenges and ensures that companies stay focused on their primary target to successfully get where they want to go Decision Support System Business-Intelligence-Data-Mining-and-Optimization-for-Decision-Making-by-Carlo-Vercellis-z Characteristics of Data for Good Decision Making Data Types and Structures Flat File: Data stored in a single file (like Excel or CSV). As data grows, it's often spread across multiple files to organize and speed up data retrieval. Database: Data is spread out across multiple tables to allow for quick access to specific pieces of data. Data Warehouse: A database designed specifically for reporting and dashboarding. It organizes data to make it more useful for analysis. Big Data: Refers to large volumes of data spread across various locations. Tools like Hadoop are used to manage and analyze this type of data. Data, Information, Knowledge, and Wisdom Decision Support System Business-Intelligence-Data-Mining-and-Optimization-for-Decision-Making-by-Carlo-Vercellis-z The needs for DSS Representation of the decision-making process In order to build effective DSSs, we first need to describe in general terms; Rationality and problem solving The decision-making process Types of decisions Approaches to the decision-making process Changing Business Environments And Evolving Needs For Decision Support And Analytics Decision types: Big-bet, high-risk decisions. These are strategic choices that can significantly impact a company's future, such as launching a new product or forming a strategic partnership. They require in-depth analysis of available data and information. Cross-cutting decisions, which are repetitive but high risk that require group work. Ad hoc decisions that arise episodically. These decisions arise unexpectedly and require quick, informed judgments. Delegated decisions to individuals or small groups. necessitating tools and systems to support sound decision-making Why are these challenges significant? Dynamic business environments: Technological advancements, shifting consumer behaviors, and intense competition create increasingly complex and volatile business landscapes. Need for data and analytics: To make informed decisions in such environments, businesses must rely on data and analytics to assess risks and opportunities. What are the solutions? Decision support systems: These systems gather, analyze, and provide insights to aid in decision-making. Artificial intelligence and machine learning: These technologies can analyze vast amounts of data to uncover patterns and trends that humans might miss. Data-driven culture: Organizations should foster a data- driven culture where data analysis becomes integral to the decision-making process. Decision Support System DSSs are a basic component in the development of a business intelligence architecture. A decision support system (DSS) is an interactive computer-based application that combines data and mathematical models to help decision makers solve complex problems faced in managing the public and private enterprises and organizations. Definition of system A system receives a set of input flows and returns a set of output flows through a transformation process regulated by internal conditions and external conditions. Abstract representation of a system Business-Intelligence-Data-Mining-and-Optimization-for-Decision-Making-by-Carlo-Vercellis-z Definition of system an abstract definition of the notion of system: Any is made up of a set of components that are in some way connected to each other to provide a single collective result and a common purpose. Any given system receives specific input flows, carries out an internal transformation process and generates observable output flows. Every system is characterized by boundaries that separate its internal components from the external environment. A system is said to be open if its boundaries can be crossed in both directions by flows of materials and information. When such flows are lacking, the system is said to be closed. Definition of system A system will often incorporate a feedback mechanism. Feedback occurs when a system component generates an output flow that is fed back into the system itself as an input flow, possibly as a result of a further transformation. The effectiveness and efficiency of a system are assessed using measurable performance indicators that can be classified into different category. Effectiveness metrics indicate whether the right action is being carried out or not. Efficiency metrics show whether the action is being carried out in the best possible way or not. Representation of the decision-making process In order to build effective DSSs, we first need to describe in general terms; Rationality and problem solving The decision-making process Types of decisions Approaches to the decision-making process 1. Rationality and problem solving A decision is a choice from multiple alternatives, usually made with a fair degree of rationality. The decision-making process is part of a broader subject usually referred to as problem solving, DSS refers to the process through which individuals try to bridge the gap between the current operating conditions of a system (as is) and the supposedly better conditions to be achieved in the future (to be). The structure of the problem-solving process Problem: The issue or challenge that needs to be addressed. Environment: The context in which the decision is made, including factors like resources, constraints, and stakeholders. Decision: The chosen course of action based on the evaluation of alternatives against the criteria. Rational approach to decision making implies that the option fulfilling the best performance criteria is selected out of all Logical flow of a problem-solving process possible alternatives. The structure of the problem-solving process Alternatives represent the possible actions aimed at solving the given problem and helping to achieve the planned objective. The possible solutions or courses of action to the problem. Criteria are the measurements of effectiveness of the various alternatives and correspond to the different kinds of system performance The factors or standards used to evaluate Logical flow of a problem-solving process the alternatives. We will talk about 1- Problem identification phase 2- decision-making process 1- Problem identification phase Root Cause Analysis (tool 1 for Problem identification) What is Root Cause Analysis (5 Whys)? Root Cause Analysis (5 Whys) is a simple yet effective problem-solving tool where you ask "why" at least five times to investigate the root cause of a problem. The goal of this method is to move from the visible symptoms to the real root of the problem, allowing for effective corrective actions and preventing the problem from recurring in the future. IC-5-WHYs-Root-Cause-Template-10551.xlsx - Microsoft Excel Online (live.com) 5 whys Case Studies Case study: Production Line Shutdown Problem: The production line was shut down for two hours. 1. Why did the production line stop? 1. Because there was a malfunction in the main engine. 2. Why did the main engine malfunction? 1. Because it overheated. 3. Why did the engine overheat? 1. Because there was an obstruction in the cooling system. 4.Why was there an obstruction in the cooling system? 1. Because of a buildup of dirt and debris in the filter. 5. Why wasn't the filter cleaned regularly? 1. Because there was no regular maintenance schedule for the filter. Root cause: Lack of a regular maintenance schedule for the filter. Solution: Establish a regular maintenance schedule for cleaning the filter. Train employees on how to inspect and clean the filter. Keep spare filter parts on hand. Another Example: Increase in Customer Complaints Why did the number of complaints increase? Because of late order deliveries. Why were the orders late? Because of a shortage of drivers. Why is there a shortage of drivers? Because of a high turnover rate among drivers. Why is the turnover rate high? Because drivers are dissatisfied with their salaries and working conditions. Root cause: Driver dissatisfaction with salaries and working conditions. Solution: Increase driver salaries. Improve working conditions. Offer incentives to drivers. Case Study: Incorrect Item Shipped In this case, J&J Shipping Company uses the 5 Whys analysis to determine why an incorrect item was shipped to a customer. Here's a breakdown of each step: Problem: The wrong item was shipped to the customer. This is the initial, most obvious question. 1. Why? The wrong item was pulled from inventory. Here, we start digging deeper into the cause. 2. Why? The item from the inventory was mislabeled. We move to a deeper level of analysis. 3. Why? The supplier mislabeled the item prior to shipping it to the warehouse. We reach an external cause. 4. Why? A worker from the supplier incorrectly labeled the product. We identify the person responsible for the mistake. 5. Why? Labels for different orders vary but are pre-printed, increasing the possibility of mislabeled items. Here, we reach the root cause of the problem, which is a labeling system that is prone to errors. Advantages of Root Cause Analysis (5 Whys) Simplicity: Anyone can use this tool without specialized training. Effectiveness: Helps identify the root cause of the problem accurately. Preventing problem recurrence: By addressing the root cause, the problem can be prevented from recurring in the future. Process improvement: Helps identify weaknesses in processes and improve them. Important Notes Number of questions: It is not necessary to ask "why" exactly five times. You may need to ask more or less depending on the complexity of the problem. Focus on the root cause: The goal is to reach the underlying cause of the problem, not just the visible symptoms. Teamwork: It is best to perform root cause analysis as a team, as you can benefit from different perspectives. Documentation: The results of the analysis should be documented to take corrective actions and track progress. Ishikawa Diagram Ishikawa Diagram (tool 2 for Problem identification) also known as a cause-and-effect diagram or fishbone diagram, is a visual tool used to identify the potential causes of a specific problem. The diagram resembles the shape of a fish, where the head represents the main problem, and the spines are the different categories of causes that contribute to this problem. Why Use an Ishikawa Diagram? Identifying root causes: The diagram helps identify all potential causes of a problem, not just the symptoms. Critical thinking: It encourages systematic and organized thinking about the problem. Teamwork: It can be used in brainstorming sessions to gather ideas from the team. Improved decision-making: It helps gain a deeper understanding of the problem and make more informed decisions. How to Draw an Ishikawa Diagram? 1. Define the problem: Write the main problem at the end of the main arrow (the fish's mouth). 2.Identify categories: Define the main categories of potential causes, such as: 1. Materials: The raw materials used in the process. 2. Method: The methods and procedures followed. 3. Machine: The equipment and tools used. 4. Measurement: Measurement and control processes. 5. Environment: The surrounding conditions. 6. Manpower: The people involved in the process. 3.Identify causes: Write potential causes for each category on the spines of the fish. 4.Analyze causes: Use "why" questions to identify the root causes for each cause. Ishikawa Diagram Ishikawa free Templates Free customizable fishbone diagram templates | Canva Ishikawa Diagram Fishbone (Cause and Effect) - Template Excel (topexceltemplates.com) Free Excel Fishbone Templates (smartsheet.com) Root Cause Analysis and Ishikawa Diagram These tools can be used in the "Problem" stage of this decision-making flowchart. These tools are particularly useful for: 1. Precisely defining the problem 2.Understanding the root causes of the problem 3.Analyzing the various factors contributing to the problem By using these tools in the problem identification phase, you can: Gain a deeper understanding of the problem before moving to the Alternatives stage. Identify key areas of focus that may lead to more effective solutions. Ensure that the alternatives proposed later address the root causes of the problem, not just the surface symptoms. Adding these tools in the "Problem" stage will enhance the entire decision-making process by providing a solid foundation for identifying and evaluating alternatives. Suggested Steps for problem solving: 1. Problem Identification: Identifying the specific issue that requires resolution. Data collection Root Cause Analysis: 1. 5 Whys: Repeatedly asking "why" to delve into the fundamental cause of the problem. 2. Ishikawa Diagram: Utilizing a fishbone diagram to identify potential causes and categorize them into categories (e.g., materials, method, machine, measurement, environment, manpower). 2.Environmental Analysis: Identifying external factors influencing the problem, with a focus on factors uncovered in the root cause analysis. 3.Suggesting Alternatives: Proposing solutions that directly address the identified root causes. Suggesting potential solutions to the problem. 4.Criteria Establishment: Setting criteria for evaluating solutions, emphasizing the impact of proposed solutions on the root causes. 5.Decision Making: Selecting the most suitable solution based on the established criteria. Root cause Illustrative Example: Problem: A surge in customer complaints regarding delayed order deliveries. 5 Whys: Why are deliveries delayed? Due to a driver shortage. Why is there a driver shortage? High turnover rate. Why is the turnover rate high? Low wages. Ishikawa Diagram: Identifying additional potential causes such as: planning issues, vehicle malfunctions, or inadequate training. Environmental Analysis: Analyzing external factors like competition, increased demand, or traffic changes. Suggesting Alternatives: Increasing wages, improving working conditions, hiring more drivers, enhancing planning systems. Establishing Criteria: Reducing the number of complaints, improving delivery times, lowering operational costs. Decision Making: Selecting the best solution based on the defined criteria. Benefits of Integrating These Tools Deeper Problem Understanding: Provides a more thorough understanding of the root causes. More Effective Solutions: Focuses solutions on addressing the fundamental causes. Improved Decision Making: Facilitates informed decisions based on comprehensive analysis. Preventing Recurrence: By addressing root causes, the likelihood of the problem reoccurring is reduced. In essence, integrating root cause analysis and Ishikawa diagrams into the early stages of the problem-solving process enables a more profound comprehension of the issue and fosters the development of more effective solutions. Lab 2- decision-making process Representation of the decision-making process In order to build effective DSSs, we first need to describe in general terms; Rationality and problem solving The decision-making process Types of decisions Approaches to the decision-making process Logical structure of the decision-making process After problem-solving process (or problem- identification process) decision-making process take place Business-Intelligence-Data-Mining-and-Optimization-for-Decision-Making-by-Carlo-Vercellis-z Factors influencing a rational choice. to prevail in the decision-making process within companies, it is however Ethical possible to identify other factors influencing a rational choice. Economic Besides being compliant with the law, a decision should abide by the ethical principles and social rules of the community to which the system Economic factors are the most influential in decision-making processes belongs. Adherence to moral principles and social norms. and are often aimed at the minimization of costs or the maximization of profits. Procedural For example, an annual logistic plan may be preferred over alternative plans if it achieves a reduction in total costs. A decision may be considered ideal from an economic, legal and social standpoint, but it may be unworkable due to practices and cultural Technical limitations of the organization in terms of prevailing procedures and common practice. Options that are not technically feasible must be discarded. Political For example, a production plan that exceeds the maximum capacity of a plant cannot be regarded as a feasible option. The decision maker must also assess the political consequences of a specific decision among individuals, departments and organizations. Legal Legal rationality implies that before adopting any choice the decision makers should verify whether it is compatible with the legislation in force within the application domain. i.e Compliance with laws and regulations. Evaluating the alternatives The process of evaluating the alternatives may be divided into two main stages, Exclusion: Eliminating alternatives that are not feasible or compatible. Evaluation: Comparing feasible alternatives based on During the exclusion stage, compatibility rules and restrictions are applied to the alternative actions that were originally identified. Within this assessment process, some alternatives will be dropped from consideration, while the rest represent feasible options that will be promoted to evaluation. In the evaluation phase, feasible alternatives are compared to one another based on the performance criteria, in order to identify the preferred decision as the best opportunity. “ Alternatives Evaluation tools SCORING MATRIX DECISION MATRIX DECISION TREE ” Tools used to evaluate options and make decisions Scoring matrix Case study 1 choosing ERP Evaluating the alternatives Objective: A manufacturing company wants to choose an ERP system to improve its operations at an affordable cost (budget 5000,000) while ensuring compatibility with current systems Specify three Alternatives: ERP systems Like (odoo, SAP…) Criteria: affordable cost compatibility with current systems Values Evaluating the alternatives Alternatives & criteria: 2. Odoo Cost: $600,000 1. SAP: 3. Oracle: 4. Microsoft Dynamics: 1. Cost: $500,000 1. Cost: $400,000 1. Cost: $350,000 2. Compatibility: 70% 2. Compatibility: 85% 2. Compatibility: 90% 3. Technical Support: 3. Technical Support: 3. Technical Support: Good (7/10) Excellent (9/10) Excellent (8/10) 4. Ease of Use: 4. Ease of Use: Good 4. Ease of Use: Excellent Moderate (6/10) (8/10) (9/10) Values for each criterion of an alternative To get the values for each criterion of an alternative, you can gather information from several sources: 1. Vendor Documentation: Review product specifications, pricing, and performance metrics from official vendor websites. 2. Industry Reports: Look at comparative studies or benchmark reports from third-party sources like Gartner, Forrester, or IDC. 3. Customer Reviews: Analyze user feedback on sites like G2, Capterra, or Trustpilot for real- world performance and user satisfaction. 4. Internal Testing: Conduct your own tests and assessments based on your organization's specific needs and context. 5. Etc. Each of these sources can help in assigning objective values for decision-making criteria. Evaluating the alternatives Exclusion Phase: Odoo is excluded due to its high cost. Evaluation Phase: SAP, Oracle and Microsoft Dynamics are compared based on performance criteria. Microsoft Dynamics is chosen for its better ease of use and compatibility at a lower cost. Evaluating the alternatives Case study Decision matrix for the ERP system example comparing SAP, Oracle, and Microsoft Dynamics based on key criteria: Microsoft Criteria SAP Oracle Dynamics Cost 2 3 5 Compatibility 3 4 5 Technical Support 4 5 4 Ease of Use 3 4 5 Total Score 12 16 19 Scale: 1 (lowest) to 5 (highest) Decision: Microsoft Dynamics is the top choice with a total score of 19 due to its high compatibility, ease of use, and lower cost. Odoo (excluded) Scoring matrix Case study 2 choosing CRM Evaluating the alternatives Let’s take a case of choosing a CRM (Customer Relationship Management) system for a multinational retail company, considering both cost, scalability, user adoption, and customer data security: Objective: The company is looking for a scalable CRM solution that enhances customer engagement, ensures data security, and allows for seamless integration with current marketing tools across different regions. Evaluating the alternatives Alternatives: Salesforce: HubSpot CRM: Zoho CRM: Cost: $1,200,000 (lower initial Cost: $900,000 (includes Cost: $2,000,000 (including cost, but customization options are customization for multi-regional customization and training) limited) operations) Scalability: 95% (highly Scalability: 75% (suitable for mid- Scalability: 80% (Scalable to some scalable, fits the company’s size but might require extra extent, but lacks certain advanced future expansion plans) upgrades for scaling) features required for large-scale operations) Data Security: 10/10 (Offers full Data Security: 8/10 (Complies with Data Security: 7/10 (Basic security encryption and regional data GDPR but lacks some advanced encryption features) features, moderate encryption but compliance) lacks advanced compliance for certain regions) User Adoption Rate: 70% User Adoption Rate: 90% (easier to (requires complex training and use with faster user onboarding) User Adoption Rate: 85% (User- integration) friendly with intuitive features and faster learning curve) Evaluating the alternatives Exclusion Phase: Salesforce is not excluded due to high security and scalability, but the high cost and lower adoption rate might be an issue for rapid rollout. Zoho CRM is excluded because it doesn’t provide enough data security and scalability for the company’s long-term goals. Evaluation Phase: Salesforce and HubSpot CRM are compared based on performance criteria. Salesforce is chosen for its better scalability and strong data security, despite its higher cost and lower adoption rate. HubSpot CRM has higher user adoption, but might not be the right choice for long- term scalability. Evaluating the alternatives Decision: Salesforce is chosen due to its better data security, scalability, and ability to support the company's global expansion, even though it requires more upfront investment and user training. The company plans to mitigate user adoption challenges by conducting extensive training sessions. Evaluating the alternatives Case study 2 Decision matrix for selecting the CRM system based on multiple criteria (cost, scalability, security, user adoption): Criteria Salesforce HubSpot CRM Zoho CRM Cost 3 4 5 Scalability 5 3 3 Data Security 5 4 3 User Adoption 3 5 4 Rate Total Score 16 16 15 Scale: 1 (lowest) to 5 (highest)Outcome: Salesforce and HubSpot CRM are tied, but Salesforce is preferred for long-term scalability and security. “ Decision matrix & Decision tree ” tools used to evaluate options and make decisions Scoring Matrix and a Decision Matrix Key Differences Complexity: A scoring matrix is generally simpler and focuses purely on scoring, while a decision matrix often includes weights for criteria and combines scores to provide a comprehensive analysis. Application: Scoring matrices are more suited for straightforward evaluations, while decision matrices are better for complex decision-making scenarios where criteria importance varies. In summary, both matrices are valuable for decision- making, but they differ in their structure and the complexity of the evaluation process. Decision matrix A decision matrix is a tool that helps evaluate and prioritize different options based on multiple criteria. It is particularly useful for making complex decisions by assigning scores to each option based on how well it meets certain criteria. Steps to Create a Decision Matrix: 1. List the Criteria: Identify the factors (e.g., cost, ease of use, quality). 2. List the Alternatives: Write down the options you're considering. 3. Score Each Option: For each criterion, assign a score (e.g., 1 to 5). 4. Total the Scores: Add up the scores for each alternative. 5. Analyze: The option with the highest total score is often the best choice. Decision matrix (+ve& -ve evaluation) When evaluating both positive and negative options in a decision matrix, follow these steps: 1. Define criteria: Choose factors that include both positive (e.g., benefits) and negative (e.g., risks, costs). 2. Score each option: Assign higher scores for positive outcomes and lower scores for negative outcomes. For example, a high benefit might score 5, while a high cost might score 1. 3. Apply weightings: You can add weights to the criteria based on their importance (e.g., cost might be more important than ease of use). 4. Calculate totals: Sum the weighted scores. The option with the highest total score indicates the best overall balance between positives and negatives. Decision matrix (+ve& -ve evaluation) Example of Positive and Negative Evaluation: Criteria Weight Option 1 Option 2 Option 3 Cost (lower is 0.3 5 3 2 better) Performance 0.5 4 5 3 (higher is better) Risk (lower is 0.2 3 4 5 better) Total Score 4.1 4.4 3.4 In this example, Option 2 has the highest score and offers the best overall trade-off between positives (performance) and negatives (cost, risk). Decision matrix (Weights calculation) Criterion Ranking & pairwise comparison methods Decision matrix (Weights calculation) To calculate weights for criteria in a decision matrix, follow these steps: 1. Identify the Criteria: List all criteria you're evaluating (e.g., cost, performance, ease of use). 2. Assign Relative Importance: Rank each criterion based on its importance (e.g., from 1 to 5, where 5 is very important and 1 is less important). 3. Normalize the Weights: Divide each criterion's rank by the sum of all rankings to get the weight. 4. Formula: Decision matrix (Weights calculation) Another way to calculate weights pairwise comparison method A Each criterion (A, B, C, D, E) is B compared with every other B criterion to determine which is C C more important. D D You then count how many E times each criterion is preferred E and use that count to calculate its weight as a percentage (marked in blue). Pairwise comparison method A A A=2 20% B=2 20% B B A B B C=0 ZERO C D=3 30% C C A B C E=3 30% D D D B D D D E EE E E E D E Decision matrix (Weights calculation) pairwise comparison method Suitability: This method is great when you want a more subjective assessment based on your personal or organizational priorities. It helps quantify relative importance. Note: If the process is consistently applied and criteria are logically compared, this method works well for decision- making, as shown in the image. Decision matrix (Weights calculation) To calculate weights for decision criteria, follow these steps: List the Criteria: Identify all criteria (e.g., cost, performance, ease of use). Rank the Criteria: Assign a ranking based on importance. The most important criterion gets the highest rank. Sum of Ranks: Add all the ranks together. Calculate the Weights: Divide each criterion’s rank by the sum of all ranks to get its weight. Weight of a criterion=Rank of that criterionSum of all ranks\te xt{Weight of a criterion} = \frac{\text{Rank of that criterion}}{\text{Sum of all ranks}}Weight of a criterion=Sum of all ranksRank of that crite rion This gives you normalized weights that add up to 1 Decision matrix (Weights calculation) Example: If you have three criteria ranked 4, 3, and 2: Sum of ranks = 4 + 3 + 2 = 9. Weight of criteria 1 = 4/9= 0.4494=0.45 Weight of criteria 2 = 3/9= 0.3393 =0.34 Weight of criteria 3 = 2/9= 0.2292 =0.23 These weights are then multiplied by the scores in the matrix to find the weighted score for each option. Break-Even Analysis Case Study Title: Choosing the Right Mobile Phone Plan Problem Statement: A person is unsure about which mobile phone plan (prepaid or subscription) is best suited to their needs. Objective: To analyze the costs and benefits of each plan and recommend the optimal choice based on the individual's usage patterns. Case Study Title: Choosing the Right Mobile Phone Plan Case Background Current Plan: The individual currently uses a prepaid card system. Changing Needs: Due to increased work calls, the individual needs a more reliable and cost-effective plan. Plan Details (alternatives): Prepaid Card: Cost per call: $1.75 Monthly fee: $30 every 3 months ($10 per month) Subscription: Cost per call: $0.5 Monthly fee: $60 Case Study Title: Choosing the Right Mobile Phone Plan Analysis Break-Even Point: Calculating the point at which the total cost of both plans becomes equal. Equation: 60 + 0.5x = 10 + 1.75x Solving for x: x = 40 minutes Cost Comparison: For less than 40 minutes per month, the prepaid card is more cost-effective. For more than 40 minutes per month, the subscription is more cost-effective. Case Study: Break-Even Point Analysis for a New Product Problem Statement A company is considering launching a new product. They need to determine the sales volume required to cover all costs and start generating profits. Data Fixed Costs: Rent for manufacturing facility: $5,000 per month Salaries of production staff: $20,000 per month Utilities: $1,000 per month Other fixed costs: $1,000 per month Variable Costs: Raw materials cost per unit: $5 Direct labor cost per unit: $3 Packaging and shipping cost per unit: $2 Selling Price per Unit: $20 Case Study: Break-Even Point Analysis for a New Product Calculation Total Fixed Costs: $5,000 + $20,000 + $1,000 + $1,000 = $27,000 per month Contribution Margin per Unit: Selling Price - Variable Costs = $20 - $5 - $3 - $2 = $10 per unit Break-Even Point (Units): Total Fixed Costs / Contribution Margin per Unit = $27,000 / $10 = 2,700 units Break-Even Point (Sales Revenue): Break-Even Point (Units) * Selling Price = 2,700 units * $20 = $54,000 Case Study: Break-Even Point Analysis for a New Product Interpretation The company needs to sell 2,700 units of the new product to cover all its costs and reach the break-even point. If they sell fewer than 2,700 units, they will incur a loss. If they sell more than 2,700 units, they will start making a profit. Case Study: Break-Even Point Analysis for a New Product Sensitivity Analysis To understand the impact of changes in fixed and variable costs on the break-even point, we can perform a sensitivity analysis: If fixed costs increase: The break-even point will increase, meaning the company will need to sell more units to cover its costs. If variable costs decrease: The break-even point will decrease, meaning the company will need to sell fewer units to cover its costs. If selling price increases: The break-even point will decrease, meaning the company will need to sell fewer units to cover its costs. Conclusion Based on the break-even analysis, the company should carefully evaluate the expected sales volume for the new product. If they believe they can sell more than 2,700 units, launching the product is likely to be profitable. However, if they anticipate selling fewer units, they may need to reconsider their pricing strategy or production costs to improve profitability. Assignment Time Series Analysis Trends Scenarios Analysis (What-If Analysis) Cost benefits analysis Risk/Uncertainty Analysis Data visualization By mastering these techniques, businesses can make data-driven decisions and gain a competitive edge. Time series & trends analysis Time Series Analysis Time Series Analysis is a statistical technique used to analyze data points collected or recorded at specific time intervals. It helps in understanding patterns, trends, and seasonal variations over time. Key Components: Trend: Long-term movement in data (e.g., increasing sales over years). Seasonality: Regular patterns or cycles (e.g., higher sales during holidays). Noise: Random variations in data. Example: A retail company analyzes monthly sales data over the past 5 years to forecast future sales and plan inventory. Solved Problem: Problem: A company wants to predict next quarter’s revenue based on past data. Solution: Use time series models like ARIMA or Exponential Smoothing to analyze historical revenue data and forecast future trends. Time Series Analysis Types 1. Descriptive Analysis: Summarizes historical data (e.g., mean, median, variance). 2. Decomposition Analysis: Breaks data into trend, seasonality, and noise components. 3. Forecasting Models: 1. ARIMA (AutoRegressive Integrated Moving Average): For stationary data. 2. Exponential Smoothing: For data with trends and seasonality. 3. Prophet (by Facebook): For business time series with strong seasonality. Dolar gold time series dataset Code BI_TimeAnalysis.ipynb - Colab Trends analysis Trends represent the general direction in which data is moving over time. They can be upward, downward, or stationary. Example: An e-commerce platform observes an upward trend in online sales due to increased internet penetration. Solved Problem: Problem: A business notices a decline in customer retention. Solution: Analyze customer data to identify the trend and implement strategies like loyalty programs to reverse it. Trends analysis Types 1. Upward Trend: Data shows a consistent increase over time (e.g., growing sales). 2. Downward Trend: Data shows a consistent decrease over time (e.g., declining customer retention). 3. Stationary Trend: Data remains constant over time (e.g., stable market demand). 4. Cyclical Trend: Long-term fluctuations not tied to seasonality (e.g., economic cycles). Scenarios Analysis (What- If Analysis) Scenarios Analysis (What-If Analysis) Scenarios Analysis involves evaluating different hypothetical situations to understand their potential impact on business outcomes. Example: A manufacturing company analyzes how a 10% increase in raw material costs would affect profitability. Solved Problem: Problem: A company wants to assess the impact of a new competitor entering the market. Solution: Use What-If Analysis to simulate different market share scenarios and develop strategies to maintain competitiveness. What-If (Scenario – Outcome – Mitigation) Analysis What-If : The dataset used for training contains errors or biases. Outcome: Reduced accuracy in grading. Potential increase in manual interventions. Mitigation: Regular dataset audits and updates. Incorporate diverse and high-quality data sources. Scenario: System scaled to accommodate more users. Outcome: Potential slowdown in processing due to high demand. Mitigation: Implement cloud-based scalability. Optimize algorithms for performance under load. What-If Scenarios for Automated Checking and Grading Tool | Gamma Scenarios Analysis (What-If Analysis) types: Best-Case Scenario: Assumes optimal conditions (e.g., maximum sales, lowest costs). Worst-Case Scenario: Assumes unfavorable conditions (e.g., economic downturn, high costs). Most Likely Scenario: Assumes realistic conditions based on historical data. Sensitivity Analysis: Examines how changes in one variable impact outcomes (e.g., how price changes affect profit). Cost-Benefit Analysis Cost-Benefit Analysis Cost-Benefit Analysis is a process of comparing the costs and benefits of a decision or project to determine its feasibility. “Costs” can be categorized as “Benefits” might be tangible or follows: intangible. These could include: Development costs Higher revenue and sales Hardware and Infrastructure Improved employee safety and morale Maintenance costs Greater customer satisfaction Training costs Competitive advantage Opportunity costs Scalability Data insights Cost-Benefit Analysis Example: A company evaluates whether to invest in a new software system by comparing the implementation costs with the expected efficiency gains. Solved Problem: Problem: Should a business expand to a new region? Solution: Conduct a Cost-Benefit Analysis to compare expansion costs (e.g., setup, marketing) with potential revenue and market growth. Scenario: Adding support for a new programming language. Costs: Development effort, testing time, documentation updates. Benefits: Broader user base, increased adoption, higher satisfaction. Calculate the Result: If benefits outweigh costs → Proceed with the feature. Cost-Benefit Analysis types: 1. Financial CBA: Focuses on monetary costs and benefits (e.g., ROI, NPV). 2. Social CBA: Includes non-monetary factors (e.g., environmental impact, public health). 3. Qualitative CBA: Uses subjective judgment for intangible factors (e.g., brand reputation). 4. Quantitative CBA: Uses numerical data for measurable factors (e.g., cost savings, revenue growth). 1] NET PRESENT VALUE (NPV): N P V = T o tal B e n e f i ts - T otal Cos ts NPV is a financial metric used to determine the profitability of an investment. It calculates the present value of all future cash flows generated by the project, minus the initial investment. If the NPV is positive, the project is expected to be profitable and is generally considered a good investment. If the NPV is negative, the project is expected to result in a loss and is typically not considered a good investment. 2] BENEFIT COST RATIO (BCR): B CR = T o tal B e n e f i ts / T otal Cos ts in real-world scenarios, NPV calculations can be more complex and may involve additional factors such as inflation, taxes, and risk. Assumptions: Project Duration: 5 years Costs: Development Costs: $100,000 Hardware and Infrastructure: $50,000 Maintenance Costs: $10,000 per year Training Costs: $20,000 Opportunity Costs: $30,000 per year Benefits: Higher Revenue and Sales: $100,000 per year Improved Employee Safety and Morale: $20,000 per year (estimated monetary value) Greater Customer Satisfaction: $15,000 per year (estimated monetary value) Competitive Advantage: $25,000 per year (estimated monetary value) Scalability: $5,000 per year (estimated monetary value) Data Insights: $10,000 per year (estimated monetary value) Calculations: 1. Total Costs: Greater Customer Satisfaction (5 years): $15,000/year * 5 years = $75,000 Development Costs: $100,000 Competitive Advantage (5 years): $25,000/year * 5 Hardware and Infrastructure: $50,000 years = $125,000 Training Costs: $20,000 Scalability (5 years): $5,000/year * 5 years = $25,000 Maintenance Costs (5 years): $10,000/year * 5 years = Data Insights (5 years): $10,000/year * 5 years = $50,000 $50,000 Opportunity Costs (5 years): $30,000/year * 5 years = Total Benefits = $875,000 $150,000 3. NPV Calculation: Total Costs = $370,000 NPV = Total Benefits - Total Costs NPV = $875,000 - $370,000 = $505,000 2. Total Benefits: Higher Revenue and Sales (5 years): $100,000/year * 5 4. BCR Calculation: years = $500,000 BCR = Total Benefits / Total Costs BCR = $875,000 / Improved Employee Safety and Morale (5 years): $370,000 = 2.36 $20,000/year * 5 years = $100,000 Interpretation: NPV: The positive NPV of $505,000 indicates that the project is expected to generate a significant profit over its 5-year lifespan. BCR: The BCR of 2.36 suggests that for every dollar invested, the project is expected to generate $2.36 in benefits. Risk/Uncertainty Analysis Risk/Uncertainty Analysis Risk/Uncertainty Analysis involves identifying and assessing potential risks and uncertainties that could impact business decisions. Example: A financial institution evaluates the risk of loan defaults under different economic conditions. Solved Problem: Problem: A project manager wants to assess the risk of delays in a construction project. Solution: Use Monte Carlo simulation to model different scenarios and estimate the probability of delays. Risk/Uncertainty Analysis types 1. Qualitative Risk Analysis: Uses expert judgment to assess risks (e.g., risk matrices). 2. Quantitative Risk Analysis: Uses numerical data and models (e.g., Monte Carlo simulation). 3. Scenario-Based Risk Analysis: Evaluates risks under different scenarios (e.g., economic recession). 4. Sensitivity Analysis: Measures how changes in input variables affect outcomes. Risk Matrices Risk matrices are a visual tool used in risk management to assess and prioritize risks based on their likelihood and impact. They are widely used in industries like project management, finance, healthcare, and engineering to make informed decisions about risk mitigation. When to Use a Risk Matrix During project planning to identify potential risks. In decision-making to evaluate the impact of risks. For communicating risks to stakeholders. Risk Matrices Components 1.Likelihood (Probability): 1. How likely is the risk to occur? 2. Measured on a scale (e.g., Low, Medium, High). 2.Impact (Severity): 1. What is the potential consequence if the risk occurs? 2. Measured on a scale (e.g., Low, Medium, High). 3.Risk Level: 1. Combines likelihood and impact to determine the overall risk (e.g., Low, Medium, High, Critical). How to Create a Risk Matrix 1.Define Likelihood and Impact Scales: Create clear definitions for each level (e.g., "High Impact" = significant financial loss). 2.Identify Risks: List all potential risks for the project or business. 3.Assess Likelihood and Impact: Evaluate each risk based on the defined scales. 4.Plot Risks on the Matrix: Place each risk in the appropriate cell based on its likelihood and impact. 5.Prioritize Risks: Focus on risks in the High- Likelihood/High-Impact quadrant first. Risk Matrix Quantitative risk analysis For each scenario, determine the likelihood and impact of the risk, calculate the risk score, and identify the risk level using the following matrix Likelihood (L) Impact (I) Risk Score (L * I) Risk Level 1 (Rare) 1 (Insignificant) 1 Low 2 (Unlikely) 2 (Minor) 4 Low 3 (Possible) 3 (Moderate) 9 Medium 4 (Likely) 4 (Major) 16 High 5 (Almost Certain) 5 (Catastrophic) 25 Very High Risk Assessment Matrix: What It Is and How to Use It Risk examples A cyberattack compromising sensitive customer data for a large e-commerce company. A sudden economic recession impacting a manufacturing company's sales and profitability. A natural disaster (e.g., flood, earthquake) damaging a hospital's critical infrastructure. A key supplier experiencing production delays, leading to disruptions in a manufacturing company's production process. A new competitor entering the market with a significantly lower-priced product. A competitor launching a superior product before your company can release its own. A major earthquake occurring in the region where a construction project is underway. A key employee suddenly leaving the company without proper notice. A software bug causing a major system outage for an e-commerce company during peak shopping season. A minor data breach affecting a small number of customer records. Risk Matrix Advantages of Risk Matrices Visual and Easy to Understand: Simplifies complex risk data into a clear format. Prioritization: Helps focus resources on high-priority risks. Flexibility: Can be adapted for qualitative or quantitative analysis. Limitations of Risk Matrices Subjectivity: Likelihood and impact assessments can be biased. Oversimplification: May not capture complex interdependencies between risks. Static Nature: Does not account for changes in risk over time. Expected Monetary Value (EMV) EMV is a statistical technique used in risk management to quantify the potential financial impact of uncertain events. It helps decision-makers assess the expected gain or loss associated with different choices or scenarios. How does EMV work? 1. Identify Potential Outcomes: Determine the possible outcomes of a decision or event. These outcomes can be positive (gains) or negative (losses). 2.Assign Probabilities: Estimate the probability of each outcome occurring. The sum of all probabilities should equal 1. EMV Formula 1.Calculate EMV: For each outcome, multiply the probability by its monetary value. Then, sum the results across all possible outcomes. Formula: EMV = Σ (Probability of Outcome * Monetary Value of Outcome where: Σ represents the sum of all outcomes Probability of Outcome is the likelihood of a specific outcome occurring Monetary Value of Outcome is the financial gain or loss associated with that outcome EMV Example Let's say a company is considering launching a new product. They estimate the following potential outcomes: Outcome 1: High success, 20% probability, profit of $1,000,000 Outcome 2: Moderate success, 50% probability, profit of $500,000 Outcome 3: Failure, 30% probability, loss of $200,000 Calculation: EMV = (0.20 * $1,000,000) + (0.50 * $500,000) + (0.30 * - $200,000) EMV = $200,000 + $250,000 - $60,000 EMV = $390,000 Based on the EMV calculation, the expected financial outcome of launching the new product is a profit of $390,000. “ Business Intelligence Terms Everyone Should Know ” Business Intelligence Terms Everyone Should Know Data Silo Data Warehouse ETL RDBMS SQL KPI Dashboard Data Silo Definition: A standalone data source not connected to others or a central repository. Example: Different departments (Sales, Marketing, Finance) may have separate systems (silos). Excel files, databases, social media profiles Goal in BI: Integrate data from various silos to perform comprehensive analysis. Data Warehouse Definition: A centralized database that stores data from multiple sources. Purpose: Helps with faster, easier analysis by storing a copy of current and historical data. Process: Data is extracted from sources, cleaned, and transformed before loading into the warehouse. ETL (Extract, Transform, Load) Definition: A process for moving data between systems. Steps: 1. Extract: Pull data from various sources. 2. Transform: Standardize, clean, and format the data. 3. Load: Store it in a centralized system like a data warehouse. RDBMS (Relational Database Management System) Definition: Software used to manage and query relational databases. Examples: MySQL, SQL Server, PostgreSQL. Use in BI: Manages data warehouses, which are relational databases made up of tables with relationships between them. SQL (Structured Query Language) Definition: A language used to query and manage relational databases. Usage in BI: SQL is used to filter, aggregate, and join data tables for analysis. Note: Different databases have slightly different versions of SQL. Slight syntax variations between different RDBMS. KPI (Key Performance Indicator) Definition: Visual representation of KPIs and aggregated data Metrics used to measure the success or performance of a business activity. Displays key metrics like revenue, stock levels, social media engagement At-a-glance snapshot of business performance Often single-screen and connected to live data – Constantly updated Examples of KPIs: Revenues, stock levels, social media engagement. Importance in BI: KPIs are crucial for tracking business goals and objectives. KPI Examples Activity Dashboard example Data Visualization Data Visualization is the graphical representation of data to help stakeholders understand patterns, trends, and insights. Tools: Charts (bar, line, pie) Dashboards (Tableau, Power BI) Heatmaps, Scatterplots Example: A marketing team uses a dashboard to visualize campaign performance metrics like click-through rates and conversions. Usage: A company struggles to communicate sales performance to stakeholders. Solution: Create interactive dashboards using tools like Power BI to visually represent sales data and trends. Data visualization Types 1. Exploratory Visualizations: Used for data analysis (e.g., scatterplots, histograms). 2. Explanatory Visualizations: Used to communicate insights (e.g., bar charts, line graphs). 3. Geospatial Visualizations: Represents data on maps (e.g., heatmaps, choropleth maps). 4. Interactive Visualizations: Allows users to explore data (e.g., dashboards, drill- down charts). 5. Specialized Visualizations: 1. Network Graphs: For relationships (e.g., social networks). 2. Tree Maps: For hierarchical data. 3. Gantt Charts: For project timelines. 1. Exploratory Visualizations 1. Exploratory Visualizations Used for data analysis to uncover patterns, trends, and outliers. Scatterplots: Visualize relationships between two continuous variables. For example, plotting study hours against test scores to identify correlations. Using Visualizations for Your Exploratory Data Analysis - StrataScratch 1. Exploratory Visualizations 1. Exploratory Visualizations Used for data analysis to uncover patterns, trends, and outliers. Scatterplots: Visualize relationships between two continuous variables. For example, plotting study hours against test scores to identify correlations. Using Visualizations for Your Exploratory Data Analysis - StrataScratch 1. Exploratory Visualizations 1. Exploratory Visualizations Histograms: Show the distribution of a single variable. For instance, displaying the age distribution of survey respondents. Using Visualizations for Your Exploratory Data Analysis - StrataScratch 1. Exploratory Visualizations 1. Exploratory Visualizations Box Plots: Summarize data distribution and identify outliers, useful for comparing multiple groups Using Visualizations for Your Exploratory Data Analysis - StrataScratch 2. Explanatory Visualizations Used to communicate insights clearly and effectively. Bar Charts: Compare categories, such as sales across different product types. 10 types of charts and graphs You Should Know for data visualization (Examples included) - PaperGen AI 2. Explanatory Visualizations Line Graphs: Display trends over time, like stock price fluctuations. 10 types of charts and graphs You Should Know for data visualization (Examples included) - PaperGen AI 2. Explanatory Visualizations Pie Charts: Show proportions of a whole, such as market share by brand. 10 types of charts and graphs You Should Know for data visualization (Examples included) - PaperGen AI 3. Geospatial Visualizations Represent data on maps to highlight spatial patterns. Heatmaps: Use color gradients to show density, such as crime incidents in a city. Choropleth Maps: Color regions based on data values, like population density by country. Interactive Maps: Allow users to zoom and filter, such as the Johns Hopkins COVID-19 Dashboard 10 Examples of Powerful Geographic Visualizations | Reality Pathing Heatmaps in Data Visualization: A Comprehensive Introduction - Inforiver 4. Interactive Visualizations Enable users to explore data dynamically. Dashboards: Combine multiple visualizations for real-time data exploration, like Tableau or Power BI dashboards. Visual representations of business metrics (KPIs). Data Visualization Makes complex data easy to read and understand. Key to BI: Effective visualization helps identify strengths and areas that need improvement. Drill-Down Charts: Allow users to navigate from high-level summaries to detailed data, such as company performance metrics. Hover Effects: Display additional information when users hover over data points, like a map showing regional details. Dashboard example Internet In Real Time | See How Many Users the Most Popular Apps Google Trends - Hot Searches Activity: Reporting & Dashboards in Close CRM Activity Reporting & Dashboards in Close CRM - YouTube 5. Specialized Visualizations Designed for specific types of data or use cases. Network Graphs: Visualize relationships, such as social networks or protein interactions. Tools like NetworkX can create these graphs. Tree Maps: Display hierarchical data, like budget allocations across departments. Gantt Charts: Track project timelines, showing task durations and dependencies Insights from data Process: Once data is analyzed and visualized, insights can be derived. Example: Identifying trends, understanding correlations, and comparing time periods. Benefit: Helps businesses adapt strategies based on real insights. Instagram Dashboard Example- KPI Insights: 1. Most posts in August had the least interactions – possibly due to over-posting or holiday seasons. 2. Posts on Tuesday and Wednesday received the highest interactions – suggests better days to post. 3. Using the "Amaro" filter resulted in more engagement – a tactic to test further. Summary Each type of visualization serves a unique purpose, from uncovering insights in data (exploratory) to communicating findings (explanatory) and representing spatial or hierarchical relationships (geospatial and specialized). Interactive visualizations enhance user engagement by allowing dynamic exploration. For more detailed examples and tools, refer to the sources cited above. Summary There are numerous types of charts and graphs, each suited to different kinds of data and purposes. Here are some common types: 1. Bar Charts: Ideal for comparing categories or showing changes over time. 2. Line Graphs: Perfect for displaying trends and changes over continuous periods. 3. Pie Charts: Effective for showing proportions of a whole. 4. Scatter Plots: Useful for showing relationships between two variables. 5. Area Charts: Similar to line graphs but with the area below the line filled in. 6. Histograms: Used to show the distribution of numerical data. 7. Box Plots: Helpful for displaying the distribution of data across different groups. 8. Heatmaps: Excellent for showing patterns in complex data sets. 9. Treemaps: Useful for displaying hierarchical data. 10. Bubble Charts: Effective for comparing three variables simultaneously. Summary Summary Time Series Analysis: For forecasting and understanding patterns over time. Trends: To identify long-term movements in data. Scenarios Analysis: To evaluate hypothetical situations. Cost-Benefit Analysis: To compare costs and benefits of decisions. Risk/Uncertainty Analysis: To assess potential risks. Data Visualization: To communicate insights effectively. By mastering these techniques, businesses can make data-driven decisions and gain a competitive edge. Conclusion Key Points: The decision-making process involves a systematic approach to problem- solving. Effective decision-making involves a structured process that considers various factors and stages. The number of alternatives can vary widely, from a few to infinite. By carefully evaluating and selecting the best option, organizations can make informed choices that lead to desired outcomes. The environment plays a significant role in influencing the decision. Criteria are used to assess the suitability of each alternative. Business-Intelligence-Data-Mining-and-Optimization-for-Decision-Making-by-Carlo-Vercellis-z