Quantitative Data Collection PDF
Document Details
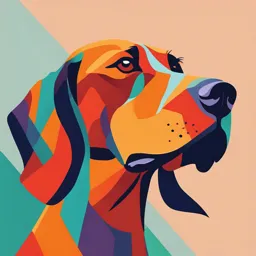
Uploaded by LavishHappiness
Holy Angel University
Elvira C. David
Tags
Summary
This document provides a comprehensive overview of quantitative data collection methods. It covers various sampling techniques like probability, stratified, and systematic sampling, along with important considerations in selecting and administering data collection instruments. The content is aimed at researchers and students involved in data collection and survey-based studies.
Full Transcript
Quantitative Data Collection Elvira C. David, PhD., RPsy, RGC., RPm Five Steps in the Process of Data Collection 1. Determine the participants to study 2. Obtain permissions needed from several individuals and organizations 3. Consider the types of information to collect from several sour...
Quantitative Data Collection Elvira C. David, PhD., RPsy, RGC., RPm Five Steps in the Process of Data Collection 1. Determine the participants to study 2. Obtain permissions needed from several individuals and organizations 3. Consider the types of information to collect from several sources available to the quantitative research 4. Locate and select instruments to use that will net useful data for the study 5. Administer the data collection process to collect data. I. Identifying Participants Identify your unit of analysis You must decide at what level (e.g., individual, family, school, school district) the data needs to be gathered Specify the Population and Sample Probability Sampling Probability Sampling involves the use of a random selection process to select a sample from members or elements of a population. The goal of probability sampling is to examine representative elements of populations. Probability Sampling 1. Simple Random Sampling is a type of probability sampling that ensures that each element of the population has an equal and independent chance of being chosen. 3 ways of selecting a simple random sample: Fishbowl Method Table of Random Numbers Method Calculator Probability Sampling Fishbowl Technique or Lottery Method Steps: 1. Write the numbers of all the participants in small pieces of papers, papers of the same, form and color. 2. Roll these papers and put them in container, where they can move freely in all directions. 3. The container is shaken very thoroughly and a number is drawn. 4. Stop when the desired the number of samples is picked from the container. Probability Sampling Procedures in Fishbowl Technique Random sample with replacement the drawn paper containing the number is returned to the container. Random sample without replacement the paper with the number is not returned to the container after it has been drawn. Probability Sampling Table of random numbers method includes a list of numbers that have been generated in such a manner that there is no order or sequencing of the numbers. Probabilistic Sampling Steps in the table of random numbers method 1. Assign a number to each element of the accessible population. 2. Enter the table of random numbers at an arbitrary or random starting point. This can be done through closing your eyes and blindly pointing to a number in the table. That number becomes your first selection. 3. Continue in a systematic way either up, down, to the left, right, or diagonally. The direction is not important, but the decision should be made before the process is started, and this direction should continue until the total sample is selected. 4. Continue to select numbers until the desired number of samples. The numbers are circled. 5. If a number is encountered more than once, the number is skipped. Table of Random Numbers 319 432 148 732 826 144 261 389 099 487 003 823 138 278 133 688 194 205 271 425 784 542 316 667 408 058 919 892 847 009 269 131 649 538 391 857 383 398 502 296 888 711 527 036 087 129 203 108 Probability Sampling Using a calculator to generate random numbers Use: NRan# (ex. 1000Ran#) Where: The first N stands for the population size Ran# - is the key used to generate random numbers Probability Sampling 2. Systematic Random Sampling involves selecting every kth element of the population, such as every fifth, eighth, or twenty-first element. K = N/n Where: K = interval N = Population size n = Sample size Probability Sampling Steps in Systematic Random Sampling: 1. Construct the population frame and assign a number to each participant randomly. 2. Compute the interval K: K = N/n 3. Enumerate the elements contained in the set of N. This set shall contain the number for the random start. 4. From this set of elements in N select a number using the Table of Random Numbers for a random start. 5. Using the number as a “system” select every nth member of the population (Determine the sampling interval or the standard distance between the selected elements) 6. Stop when the required number of participants has been obtained. Probability Sampling 3. Stratified Random Sampling the population is divided into subgroups, or strata, according to some variable or variables of importance to the research study. Steps: 1. Construct the population of the participants and determine the relevant strata. 2. Select the number using proportional or disproportional stratified sampling. 3. Choose the participants of the research within each of the categories according to simple random sampling methods. Probability Sampling 2 approaches in getting the samples from strata: a. Proportional Stratified Sampling involves obtaining a sample from each stratum that is in proportion to the size of that stratum in the total population. b. Disproportional Stratified Sampling involves the selection of members from strata where the number of members chosen from each stratum is not in proportion to the size of the stratum in the total population. Probability Sampling Probability Sampling 4. Multistage Cluster Sampling Also known as Cluster Random Sampling where large groups or “clusters” become the sampling units. Region Province Town Barangay Use Random or Systematic Random Sampling Using Power Analysis to Determine Sample size Power Analysis The power of a statistical test is the probability that the test will correctly reject a false null hypothesis. That is, power is the probability that the test will identify a treatment effect if one really exists. Factors that Influence Power Effect Size as the effect size increases, the probability of rejecting Ho also increases, which means that the power of the test increases. Measures of effect size such as Cohen’s d and measures of power both provide an indication of the strength or magnitude of a treatment effect. Sample Size A larger sample produces greater power for a hypothesis test. Because power is directly related to sample size, one of the primary reasons for computing power is to determine what sample size is necessary to achieve a reasonable probability for a successful research study. Factors that Influence Power Alpha Level Reducing the alpha level for a hypothesis test also reduces the power of the test. For example, lowering α from.05 to.01 lowers the power of the hypothesis test. One-Tailed vs. Two-Tailed Tests If the treatment effect is in the predicted direction, then changing from a regular two-tailed test to a one-tailed test increases the power of the hypothesis test. Power Analysis using G*Power G*Power is a tool to compute statistical power analyses for many different t tests, F tests, χ2 tests, z tests and some exact tests. G*Power can also be used to compute effect sizes and to display graphically the results of power analyses. The main purpose underlying statistical power analysis is to help the researchers to determine the smallest sample size that is suitable to detect the effect of a given test at the desired level of significance. How to Determine Sample size using G*Power https://www.statisticssolutions.com/how-to-determine-sample-size-from- gpower/ G*Power User Manual https://www.psychologie.hhu.de/fileadmin/redaktion/Fakultaeten/Mathematisch- Naturwissenschaftliche_Fakultaet/Psychologie/AAP/gpower/GPowerManual.pdf Power Analysis using G*Power Input the following Test family Select the appropriate test for your analysis Effect size Alpha error probability (.05) Estimated power (1-B probability) (.80 or 1-.20) Power Analysis using G*Power As an example, if you aim to conduct a cross-sectional study measuring the correlation between the number of overtime hours worked and burnout symptoms in a sample of emergency room nurses, you can estimate the sample size required to determine whether your correlation significantly differs from zero (e.g., one possible hypothesis is that there will be a significant positive association between number of hours worked and emotional exhaustion burnout symptoms). This power analysis requires just three pieces of information: 1. An estimate of the size of correlation (r). A common approach for generating this estimate is to find similar studies that have reported the size of the correlation between hours worked and burnout symptoms. Power Analysis using G*Power 2. A two-tailed alpha value (α). This value is called the Type I error rate and refers to the risk we want to take in saying we have a real non-zero correlation when in fact this effect is not real (and determined by chance), that is, a false positive effect. A commonly accepted alpha value is.05, which refers to a 5% probability (5/100) that we are comfortable making a Type I error, such that 5% of the time we will say that there’s a significant (non-zero) relationship between number of hours worked and burnout symptoms when in fact this effect occurred by chance and is not real. 3. A beta value (β). This value is called the Type II error rate and refers to the risk we want to take in saying we do not have a significant effect when in fact there is a significant association, that is, a false negative effect. Researchers commonly try to balance the risks of making Type I versus Type II errors, with a commonly accepted beta value being.20. Power analysis calculators will commonly ask for estimated power, which refers to 1 − beta (1 −.20 =.80). Power Analysis using G*Power You can then plug these numbers into a power analysis calculator to determine the sample size needed. If you assume that the estimated association is r =.25, with a two- tailed alpha value of.05 and a beta value of.20, the power analysis calculation indicates that you need at least 123 participants in the study you aim to conduct. To get some practice, try conducting this sample size determination power analysis using the G*Power software program with the following input parameters: Test family: Exact Statistical test: Correlation: Bivariate normal model Type of power analysis: A priori: Compute required sample size Tails: Two Correlation ρ H1:.25 α err prob:.05 Power (1 – β err prob):.8 Correlation ρ H0: 0 Nonprobability Sampling Nonprobability Sampling the sample elements are chosen from the population by nonrandom methods. individuals are representative of the population 1. Convenience Sampling involves choosing readily available people or objects for a study. also known as accidental or incidental sampling. 2. Snowball sampling (network or chain sampling) the researcher asks participants to identify others to become members of the sample Nonprobability Sampling 2. Quota Sampling is similar to stratified random sampling except for obtaining sample members. 3. Purposive Sampling involves “handpicking” of subjects. also known as judgmental sampling. Considerations in Sample Size in Quantitative Studies 1. The larger the sample, the more representative it is likely to be. 2. The larger the sample, the smaller the sampling error. 3. As the sample size increases, the probability of getting a deviant sample diminishes because large samples provide opportunity to counterbalance atypical values (sampling bias). 4. Power analysis determines how large the sample size is through statistics. Sampling Error - is the difference between data obtained from a random sample and the data that would be obtained if an entire population were measured. Sampling Bias - is the bias that occurs when samples are not carefully selected. Considerations in Sample Size in Quantitative Studies Sample size estimates (Creswell, 2019) Approximately 15 participants in each group in an experiment Approximately 30 participants for a correlational study that relates variables Approximately 350 individuals for a survey study, but this size will vary depending on several factors Sampling Designs in Qualitative Studies 1. Convenience and Snowball Sampling Volunteer Sampling – when researchers need participants to come forward to identify themselves. Nominated Sampling – relies on the nominations or referrals of others already in the sample. Sampling Designs in Qualitative Studies 2. Purposive Sampling The qualitative researchers strive to select sample members purposefully based on the information needs emerging from the early findings. Strategies for Purposive Sampling (Patton, 2015) a. Maximum variation sampling involves purposefully selecting cases with a range of variation on dimensions of interest. b. Homogenous sampling involves a deliberate reduction of variation to permit a more focused inquiry. c. Typical case sampling involves selecting participants who will illustrate or highlight what is typical or average. Sampling Designs in Qualitative Studies d. Extreme/deviant case sampling provides opportunities for learning from the most unusual and extreme informants (e.g., outstanding successes and notable features). e. Criterion sampling involves studying cases that meet a predetermined criterion of importance. f. Sampling of confirming and disconfirming cases Confirming cases are additional cases that fit researchers’ conceptualizations and offer enhanced credibility. Disconfirming cases are new cases that do not fit and serve to challenge researchers’ interpretations. Sampling Designs in Qualitative Studies 3. Theoretical Sampling is a method of sampling that is most often used in grounded theory studies. is a process of data collection for generating theory whereby the analyst jointly collects, codes, and analyzes his data and decides what data to collect next and where to find them, in order to develop his theory as it emerges (Glaser, 1978). The purpose is to discover categories and their properties and to offer interrelationships that occur in the substantive theory. Strategies for Selection of Samples and Cases Considerations in Sample Size in Qualitative Studies Data saturation is the guiding principle in qualitative sampling. A small number of informants can generate a large sample for analysis (i.e. the “incidents” or experiences are the basis for analysis) Final sampling decisions generally take place during data collection. For ethnographic studies, 25-50 informants; phenomenology, 10 or lower; grounded theory studies, 20-30 people. Sample Description in Qualitative Studies The research report should include a description of the following aspects of the sample: 1. The type of sampling procedure 2. The population under study and the eligibility (inclusion) criteria for sample selection 3. The number of participants in the study and a rationale for the sample size * Example for qualitative research II. Obtain Different Types of Permissions Institutions or organizations (e.g., school district) Specific sites (e.g., the secondary school) A participant or group of participants Parents of participants (e.g., 10th graders’ parents) The campus on which you conduct the research (i.e., permission from your university or college institutional review board) II. Obtain Different Types of Permissions Review Board Approval An institutional review board is a committee made up of faculty members Process of Obtaining Approval from Review Boards 1. Start by finding out about the review process used by the institutional review board on your campus. 2. Determine what information the review board needs about your project. 3. Develop an informed consent form for participants to sign before they participate in the study. III. Determine type of information to Collect III. Determine type of information to Collect III. Determine type of information to Collect III. Determine type of information to Collect IV. Selecting an Instrument Criteria for Choosing a Good Instrument Have authors developed the instrument recently, and can you obtain the most recent version? Instruments over 5 years old might be outdated. Is the instrument widely cited by other authors? Frequent use by other researchers will provide some indication of its endorsement by others. Are reviews available for the instrument? Look for published reviews about the instrument in the MMY or in journals such as Measurement and Evaluation in Counseling and Development. If reviews exist, it means that other researchers have taken the instrument seriously and seek to document its worth. Is there information about the reliability and validity of scores from past uses of the instrument? Does the procedure for recording data fit the research questions/hypotheses in your study? Does the instrument contain accepted scales of measurement? IV. Selecting an Instrument Modifying an instrument means locating an existing instrument, obtaining permission to change it, and making changes in it to fit your requirements. Typically, authors of the original instrument will ask for a copy of your modified version and the results from your study in exchange for your use of their instrument. Some only require that you cite them. Reliability and Validity of an Instrument Reliability means that scores from an instrument are stable and consistent. Scores should be nearly the same when researchers administer the instrument multiple times at different times. Factors that result in unreliable data: Questions on instruments are ambiguous and unclear Procedures of test administration vary and are not standardized Participants are fatigued, are nervous, misinterpret questions, or guess on tests (Rudner, 1993) Types of Reliability Reliability and Validity of an Instrument Validity is the development of sound evidence to demonstrate that the test interpretation (of scores about the concept or construct that the test is assumed to measure) matches its proposed use. This definition, in place since 1985, changes the traditional focus on the three-fold types of validity—construct, criterion-referenced, and content—and shifts the emphasis from “types” of validity to the “evidence” and “use” of the test or instrument (Thorndike, 1997b). Reliability and Validity of an Instrument Steps to employ in determining validity: 1. Identify an instrument (or test) that you would like to use 2. Look for evidence of validity by examining prior studies that have reported scores and use of the instrument 3. Look closely at the purpose for which the instrument was used in these studies 4. Look as well at how the researchers have interpreted (discussed if the instrument measured what it is intended to measure) the scores in light of their intended use 5. Evaluate whether the authors provide good evidence that links their interpretation to their use. V. The Collection Process Scales of measurement are response options to questions that measure (or observe) variables in categorical or continuous units. It is important to understand scales of measurement to assess the quality of an instrument and to determine the appropriate statistics to use in data analysis. Two Basic Types: 1. Categorical scales have two types: nominal and ordinal scales. 2. Continuous scales (often called scale scores) two types: interval/quasi-interval and ratio scales. V. The Collection Process Nominal Scales Researchers use nominal scales (or categorical scales) to provide response options where participants check one or more categories that describe their traits, attributes, or characteristics. They do not have any order. Ordinal Scales Researchers use ordinal scales (or ranking scales or categorical scales) to provide response options where participants rank from best or most important to worst or least important some trait, attribute, or characteristic. These scales have an implied intrinsic order. For example, first to last place; “highly important” to “of no importance”; “to a great extent” to “a little extent” V. The Collection Process Interval/Rating Scales Interval scales (or rating scales or continuous scales) provide “continuous” response options to questions with assumed equal distances between options. These scales may have three, four, or more response options. Likert scale ( strongly agree to strongly disagree) illustrates a scale with theoretically equal intervals among responses. It has become common practice to treat this scale as a rating scale and assume that the equal intervals hold between the response categories (Blaikie, 2003). However, we have no guarantee that we have equal intervals. Hence, often the Likert scale ( strongly agree to strongly disagree) is treated as both ordinal and interval data in educational research. Hence the term quasi-interval. V. The Collection Process Ratio Scale (or true zero scale) A response scale in which participants check a response option with a true zero and equal distances between units. Examples: height of individuals (e.g., 50 inches, 60 inches) and income levels (from zero dollars to $50,000 in increments of $10,000). Combined Scales In educational research, quantitative investigators often use a combination of categorical and continuous scales. Of these, interval scales provide the most variation of responses and lend themselves to stronger statistical analysis. The best rule of thumb is that if you do not know in advance what statistical analysis you will use, create an interval or continuous scale. Continuous scales can always be converted into ordinal or nominal scales (Tuckman, 1999), but not vice versa. Survey Research Three aspects of using mailed questionnaires: A cover letter to invite participants to complete the questionnaire The form and construction of the questionnaire Statistical procedures typically used to analyze data from a mailed questionnaire Survey Research Parts of a mailed questionnaire 1. A cover letter 2. Closed-ended questions asking participants about their background (i.e., demographic questions) 3. Closed-ended questions addressing practices or behaviors (e.g., “I claim responsibility when I’ve made a ‘bad call’ professionally”) and attitudes (e.g., “I benefi t from collaborative refl ection with colleagues”) 4. Open-ended questions permitting respondents to add their perceptions (e.g., “In your opinion, what defi nes a competent student affairs professional?”) 5. Closing instructions thanking the participant for taking part in the study Survey Research 1. Mail out the original questionnaire. 2. Follow it 2 weeks later with a second questionnaire to the individuals who have not responded (called nonrespondents). 3. After another 2 weeks, send a postcard to the nonrespondents, reminding them to complete the questionnaire. Common Problems in Item Construction in Surveys Common Problems in Item Construction in Surveys Common Problems in Item Construction in Surveys V. The Collection Process Standardization involves instruments and procedures When procedures vary, you introduce bias into the study and the data for individuals may not be comparable for analysis. V. The Collection Process Ethical Issues Data collection should be ethical, and it should respect individuals and sites. Obtaining permission before starting to collect data Protecting the anonymity of individuals by assigning numbers to returned instruments Keeping the identity of individuals confidential offers privacy to participants. Respect the wishes of individuals who choose not to participate in your study. Even when they consent to participate, people may back out or not show up for an observation or interview. Reduce disruption of the research site Obtain permissions and clearly communicate the purpose of the study before you collect data.