EXAM Study Guide 2022 PDF
Document Details
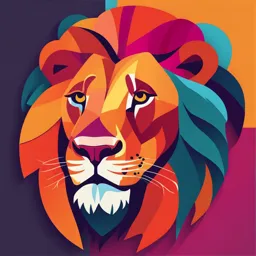
Uploaded by BrighterMiami2098
2022
Tags
Summary
This is a study guide for a semester review and covers topics such as categorical versus quantitative data, analyzing categorical data, describing/comparing distributions of quantitative data, the effect of shape on measures of centers, resistant measures, interpreting standard deviation, and z-scores. It also includes a section on interpreting probability and Random Variables.
Full Transcript
Semester Review UNIT 1 – Describing Distributions & the Normal Distribution Categorical vs. Quantitative Data -- Data are categorical if they place individuals into groups or categories and data are quantitative if they take number values that are amounts...
Semester Review UNIT 1 – Describing Distributions & the Normal Distribution Categorical vs. Quantitative Data -- Data are categorical if they place individuals into groups or categories and data are quantitative if they take number values that are amounts— counts or measurements. o Use bar graphs, pie graphs, or segmented bar charts for categorical variables such as color or gender. o Use dotplots, stemplots, histograms, or boxplots for quantitative variables such as age or weight. Analyzing Categorical Data -- Two variables have an association if knowing the value of one variable helps to predict the value of the other. If knowing the value of one variable does not help predict the value of the other variable, there is no association between the two variables. o Marginal Distribution: Gives the proportion of individuals that have a specific value for one categorical variable. o Joint Distribution: Gives the proportion of individuals that have a specific value for one categorical variable and a specific value for another categorical variable. o Conditional Distribution: Gives the proportion of individuals that have a specific value for one categorical variable among individuals who share the same value of another categorical variable (the condition). Left-handed Pitcher Right-handed Pitcher Joint Distribution of “Walk” AND “Left” Hit 123 60 183 24/745 = 0.032 Walk 24 46 70 Out 117 375 492 201 745 544 Conditional Distribution Marginal Distribution 123/544 = 0.226 183/745 = 0.246 46/544 = 0.085 70/745 = 0.094 375/544 = 0.689 492/745 = 0.660 1|Page Describing/Comparing Distributions of Quantitative Data -- Discuss the following, in context (using variable name) with comparison phrases (e.g., greater than) for center and variability o Shape – Skewed Left, Skewed Right, Approximately Mound-Shape Symmetric, Uniform, Single-peaked (Unimodal), Double-peaked (Bimodal) o Center – Mean or Median o Spread (Variability) – Standard Deviation or IQR (as well as Range) o Outliers – Discuss them if there are obvious ones. Also, look for gaps and clusters. Outliers are any observation 𝑥 where 𝑥 < 𝑄1 − 1.5 ∗ 𝐼𝑄𝑅 or 𝑥 < 𝑄3 + 1.5 ∗ 𝐼𝑄𝑅 Outliers can also be defined as 𝑥 where 𝑥 < 𝑚𝑒𝑎𝑛 − 2 ∗ 𝑠𝑡𝑑. 𝑑𝑒𝑣. or 𝑥 > 𝑚𝑒𝑎𝑛 + 2 ∗ 𝑠𝑡𝑑. 𝑑𝑒𝑣., especially if the distribution is approximately mound-shape symmetric The Effect of Shape on Measures of Centers -- In general the following are true o Skewed Left implies Mean < Median* o Skewed Right implies Mean > Median* o Fairly Symmetric implies Mean ≈ Median* *The comparison of mean and median supports shape but does not guarantee it. Resistant Measures -- A resistant measure is not affected much by outliers. o Resistant measures include: median, IQR, Q1, Q3 o Non-resistant measures include: mean, SD, range Interpret Standard Deviation -- Standard Deviation measures variability by giving the “typical” distance that the observations are away from the mean. EXAMPLE: “The heights of students at our school typically vary by about 3.1 inches from the mean, on average.” Interpret a z-score -- A z-score (also called a standardized score) describes how many standard deviations a value falls from the mean of the distribution and in what direction. value − mean 𝑧= standard deviation EXAMPLE: “Jessica’s test score was 2.3 standard deviations below the mean (z = –2.3).” Percentiles -- The pth percentile of a distribution is the point that has p% of the values less than or equal to that point. EXAMPLE: A student who scores at the 90th percentile got a score greater than or equal to 90% of the other test takers. 2|Page Transforming Data -- Adding “a” to every member of a data set adds “a” to the measures of center/position, but does not change the measures of variability or the shape. Multiplying every member of a data set by a positive constant “b” multiplies the measures of center/position by “b” and multiplies most measures of variability by “b,” (multiplies variance by 𝑏 2 ) but does not change the shape. Density Curve -- Curve that is always on or above the horizontal axis and has area exactly 1 underneath it. A density curve describes the overall (idealized) pattern of a distribution. The area under the curve and above any interval of values on the horizontal axis is the proportion of all observations that fall in that interval. Standard Normal Distribution (z Distribution) -- The Normal distribution with mean = 0 and SD = 1. Finding Areas under a Normal Distribution – 1. Draw a Normal distribution 2. Perform calculations (i) Standardize each boundary value (calculate a z-score) and use Table A or technology. (ii) Use technology without standardizing: normalcdf(lower, upper, mean, SD). Include labels if you are using your calculator! Finding Boundaries in a Normal Distribution – 1. Draw a Normal distribution 2. Perform calculations (i) Use Table A or technology to find the value of z with the appropriate area to the left of the boundary, then “unstandardize.” (ii) Use technology without standardizing: invnorm(area, mean, SD). Include labels if you are using your calculator! 3|Page UNIT 2 – Gathering Data Census -- Study that attempts to collect data from every individual in the population. Bias -- The design of a statistical study shows bias if it is very likely to underestimate or very likely to overestimate the value you want to know. Convenience samples, voluntary response samples, undercoverage, nonresponse, and other issues (e.g., wording of questions) can result in bias. Simple Random Sample (SRS) -- A sample taken in such a way that every set of n individuals has an equal chance to be the sample actually selected. Using a Random Digit Table – o Label. Give each member of the population a numerical label with the same number of digits. o Randomize. Read consecutive groups of digits of the appropriate length from left to right across a line in table. Ignore any group of digits that was not used as a label or that duplicates a label already in the sample. Continue until you have chosen n different labels. o Select. Your sample contains the individuals whose labels you find. Stratified Random Sampling -- Split the population into homogeneous groups (strata), select an SRS from each stratum, and combine to form the overall sample. Stratified random sampling guarantees that each of the strata will be represented. When strata are chosen properly, a stratified random sample will produce better (less variable/more precise) information than an SRS of the same size. Cluster Sampling -- Split the population into groups (often based on location) called clusters, and randomly select whole clusters for the sample. When clusters are chosen properly, cluster sampling is more efficient than simple random sampling. Experiment vs. Observational Study -- A study is an experiment only if researchers impose a treatment upon the experimental units. Well-designed experiments allow for cause and effect conclusions. In an observational study, researchers do not attempt to influence the results and cannot conclude cause and effect. 4|Page Confounding -- Two variables are confounded if it cannot be determined which variable is causing the change in the response variable. EXAMPLE: if people who choose to take vitamins have less cancer, we may not be able to say for sure that the vitamins are causing the reduction in cancer. It could be other characteristics of vitamin takers, such as diet or exercise that caused the reduction. Control Groups & Blinding -- A control group often gets a placebo (fake treatment) and gives researchers a comparison group to evaluate the effectiveness of the treatment(s). When the subjects do not know which treatment they are receiving, they are blind. When the people interacting with the subjects and measuring the response variable also do not know, they are blind. If both groups are blind, the study is double-blind. Random Assignment -- The purpose of random assignment is to create groups of experimental units that are roughly equivalent at the beginning of the experiment. If treatments are assigned to experimental units completely at random (i.e., with no blocking), the result is a completely randomized design. Blocking & Matched Pairs Designs -- Prior to random assignment, divide experimental units into groups (blocks) of experimental units that you expect to respond similarly. Then, randomly assign treatments within blocks. Blocking helps account for the variability in the response variable caused by the blocking variable. A matched pairs design uses blocks of size 2 or gives both treatments to each subject in random order. Scope of Inference: Generalizing to a Larger Population -- We can generalize the results of a study to a larger population if we randomly select from that population. However, be aware of sampling variability—the fact that different samples of the same size from the same population will produce different estimates. Scope of Inference: Cause-and-Effect -- Cause-and-effect conclusions are possible if we randomly assign treatments to experimental units in an experiment and find a statistically significant difference. A difference is statistically significant if it is larger than what would be expected to happen by chance alone. Conducting a Simulation – o Describe how to use a chance device to imitate one trial (repetition) of the simulation. Tell what you will record at the end of each trial. o Perform many trials of the simulation. o Use the results of your simulation to answer the question of interest. 5|Page UNIT 3 – Probability & Random Variables Interpreting Probability – The probability of an event is the proportion of times the event would occur in a very large number of repetitions. It must be a number between 0 and 1. EXAMPLE: “If I were to flip a coin many, many times, I would expect to get heads in about 50% of the flips.” Law of Large Numbers – The Law of Large Numbers says that if we observe many repetitions of a chance process, the observed proportion of times that an event occurs approaches a single value, called the probability of that event. Probability Rules – o General Addition Rule (“or” means add): P(A or B) = P(A∪B) = P(A) + P(B) – P(A∩B) (on the formula sheet) o General Multiplication Rule (“and” means multiply) P(A and B) = P(A∩B) = P(A)⋅P(B|A) o Complement Rule: P(AC) = 1 – P(A) Conditional Probability – Probability that one event occurs given that another event is already known to have occurred. 𝑃(𝐴∩𝐵) 𝑃(𝐴 given 𝐵) = 𝑃(𝐴|𝐵) = 𝑃(𝐵) (on formula sheet) Mutually Exclusive Events – Events A and B are mutually exclusive if they share no outcomes (they cannot happen at the same time). P(A and B) = P(A∩B) = 0 Independent Events – Events A and B are independent if knowing that Event A has occurred (or has not occurred) does not change the probability that event B occurs. P(B) = P(B | A) = P(B | AC) or P(A) = P(A | B) = P(A | BC) Continuous and Discrete Random Variables – o A discrete random variable has a fixed set of possible values with gaps between them. Display its probability distribution with a table or histogram. o A continuous random variable can take any value in an interval on the number line. Display its probability distribution with a density curve. 6|Page Mean/Expected Value of a Random Variable – The mean/expected value describes the long run average of a random variable. 𝜇𝑥 = 𝐸(𝑋) = ∑ 𝑥𝑖 𝑝𝑖 SAMPLE INTERPRETATION: “If I played the game many times, I would win about $0.05 per game, on average.” Standard Deviation of a Random Variable – The standard deviation measures how much a random variable typically varies from the mean. 𝜎𝑥 = ∑(𝑥𝑖 − 𝜇𝑥 )2 𝑝𝑖 SAMPLE INTERPRETATION: “If I played the game many times, the amount I win typically varies by about $0.15 from the mean.” Transforming Random Variables – If 𝑌 = 𝑎 + 𝑏𝑋, o 𝜇𝑌 = 𝑎 + 𝑏𝜇𝑥 o 𝜎𝑌 = |𝑏|𝜎𝑋 Combining Random Variables – o 𝜇𝑋+𝑌 = 𝜇𝑋 + 𝜇𝑌 𝜇𝑋−𝑌 = 𝜇𝑋 − 𝜇𝑌 If X and Y are independent: o 𝜎𝑋+𝑌 = √𝜎𝑋2 + 𝜎𝑌2 𝜎𝑋−𝑌 = √𝜎𝑋2 + 𝜎𝑌2 Binomial Setting and Random Variable – Bernoulli? Each trial can be classified as success/failure. Independent? Trials must be independent. Number? The number of trials (n) must be fixed in advance. Same probability of success? The probability of success (p) must be the same for each trial. X = number of successes in n trials Calculating Binomial Probabilities – 𝑛 𝑃(𝑋 = 𝑥𝑖 ) = (𝑥 ) (𝑝) 𝑥𝑖 (1 − 𝑝)𝑛−𝑥𝑖 = binompdf(𝑛, 𝑝, 𝑥𝑖 ) 𝑖 𝑃(𝑋 ≤ 𝑥𝑖 ) = Binomcdf(𝑛, 𝑝, 𝑥𝑖 ) Remember to label n, p, and 𝑥𝑖 Mean, Standard Deviation, and Shape of a Binomial Distribution – o Center: 𝜇𝑥 = 𝑛𝑝 o Spread: 𝜎𝑥 = √𝑛𝑝(1 − 𝑝) o Shape: Approximately Normal if 𝑛𝑝 ≥ 10 and 𝑛(1 − 𝑝) ≥ 10 If not approximately Normal and 𝑝 is closer to 0 than 1, then skewed right. If not approximately Normal and 𝑝 is closer to 1 than 0, then skewed left. 7|Page Geometric Setting and Random Variable – Arises when we perform independent trials of the same chance process and record the number of trials it takes to get one success. On each trial, the probability p of success must be the same. X = number of trials needed to achieve one success (includes the one success) 𝑃(𝑋 = 𝑥𝑖 ) = 𝑝(1 − 𝑝)𝑥𝑖−1 1 √1 − 𝑝 𝜇𝑋 = , 𝜎𝑋 = 𝑝 𝑝 UNIT 4 – Sampling Distributions Parameters and Statistics – A parameter measures a characteristic of a population, such as a population mean μx, population SD σx, or population proportion p. A statistic measures a characteristic of a sample, such as a sample mean 𝑥̅ , sample SD 𝑠𝑥 , or sample proportion 𝑝̂. Statistics are used to estimate parameters. Sampling Distribution – A sampling distribution is the distribution of a statistic in all possible samples of the same size. It describes the possible values of a statistic and how likely these values are. Contrast with the distribution of the population and the distribution of a sample. Unbiased Estimator – A statistic is an unbiased estimator of a parameter if the mean of its sampling distribution equals the true value of the parameter being estimated. In other words, the sampling distribution of the statistic is centered in the right place. Sampling Distribution of 𝒑 ̂– o Center: 𝜇𝑝̂ = 𝑝 𝑝(1−𝑝) o Spread: 𝜎𝑝̂ = √ 𝑛 o Shape: Approximately Normal if 𝑛𝑝 ≥ 10 and 𝑛(1 − 𝑝) ≥ 10 Sampling Distribution of 𝒙 ̅– o Center: 𝜇𝑥̅ = 𝜇𝑥 𝜎 o Spread: 𝜎𝑥̅ = 𝑥 √𝑛 o Shape: Normal if the population is Normal Or Approximately Normal by the CLT if 𝑛 ≥ 30 Central Limit Theorem – If the population distribution is not Normal, the sampling distribution of the sample mean 𝑥̅ will become more and more Normal as n increases. Point Estimate – The single-value “best guess” for the value of a population parameter. EXAMPLE: 𝑝̂ = 0.63 is a point estimate for the population proportion 𝑝. 8|Page