Epidemiology 2024 PDF
Document Details
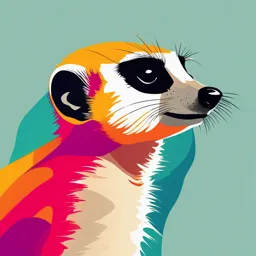
Uploaded by FinerLimeTree
University of Southern Denmark - SDU
Tags
Related
- Medical Terminology in Epidemiology and Public Health PDF
- Al-Quds University Faculty of Medicine, Introduction to Public Health Lecture 5, 2024-2025 PDF
- Epidemiology: Cornerstone of Public Health - PDF
- Medicine and Society 1 Review PDF
- MED 106 Research Methods and Medical Statistics: Introduction to Biomedical Research (PDF)
- PubHealth Exam 1 Notes PDF
Summary
This document introduces epidemiology, discussing causality, study designs, and measures of association in the field. It covers various types of studies, from ecological studies to randomized controlled trials, and explores different measures, such as relative risk.
Full Transcript
Topic 1; Intro to Epidemiology, Causality & Epidemiol Study Design What is epidemiology and what is its relevance to biomedicine? Epidemiology is the study of factors affecting human health and diseases. It uses quantitative research methods to analyze disease patterns and risk fac...
Topic 1; Intro to Epidemiology, Causality & Epidemiol Study Design What is epidemiology and what is its relevance to biomedicine? Epidemiology is the study of factors affecting human health and diseases. It uses quantitative research methods to analyze disease patterns and risk factors. Epidemiology helps in understanding the spread and prevention of diseases. It identifies associations between exposures and health outcomes. The field investigates cause-effect relationships in health. Epidemiology provides study designs for biomedical research. It involves biostatistics for data analysis. The discipline emphasizes communication of research findings. Epidemiology contributes to public health research and interventions. The concept of causality, cause-effect relations, and the concept of association Causality in epidemiology pertains to the cause-effect relationship between an exposure and an outcome. Cause-effect relations involve establishing a direct link between a specific cause and its effect through research and analysis. Association in epidemiology refers to the statistical relationship between an exposure and an outcome without implying causation. Epidemiologists use measures like relative risk, incidence rate ratio, and risk difference to quantify associations between exposures and outcomes. Establishing causality is complex and requires rigorous study designs and statistical analyses. The process of establishing causality involves inferring causal links from identified associations. Epidemiological study designs and the hierarchy of evidence; ecological studies, cross-sectiona l studies, case-control studies, cohort studies, and randomized controlled trials Ecological Studies: Study populations as a whole, useful for generating hypotheses but limited in establishing causality. Cross-Sectional Studies: Capture data at a single point in time, provide a snapshot of prevalence but cannot establish causality. Case-Control Studies: Compare cases to controls retrospectively, useful for studying rare diseases but prone to recall bias. Cohort Studies: Follow individuals over time, strong for establishing causality due to temporal relationships. Randomized Controlled Trials (RCTs): Gold standard for establishing causality, participants randomly assigned to groups. The hierarchy of evidence ranks study designs based on their strength: 1. Randomized Controlled Trials (RCTs): Highest level of evidence for establishing causality. 2. Cohort Studies: Provide strong evidence for causal relationships. 3. Case-Control Studies: Useful for studying rare diseases but retrospective and prone to biases. 4. Cross-Sectional Studies: Offer a snapshot of prevalence but cannot establish causality. 1 5. Ecological Studies: Limited in establishing causality due to the ecological fallacy. Measures of frequency; prevalence proportion (PP), cumulative incidence (Cl), incidence rate (IR), and odds Measures of frequency in epidemiology include: Prevalence Proportion (PP): Represents the proportion of individuals with a disease at a specific point in time in a given population. Cumulative Incidence (CI): Indicates the probability of an event occurring within a specified time period in a cohort. Incidence Rate (IR): Reflects the rate at which new cases of a disease occur in a population over a defined time period. Odds: The ratio of the probability of an event occurring to the probability of it not occurring, commonly used in case-control studies to assess the association between exposure and outcome. Measures of association; preva lence proportion ratio (PPR), prevalence proportion difference (PPD), re lative risk (RR), risk difference (RD)/cumu lat ive incidence difference (CID), incidence rate ratio (IRR), incidence rate difference (IRD), and odds ratio (OR) Measures of association in epidemiology include: Prevalence Proportion Ratio (PPR): Compares the prevalence of a condition between exposed and unexposed groups. Prevalence Proportion Difference (PPD): Calculates the absolute difference in prevalence between exposed and unexposed groups. Relative Risk (RR): Compares the risk of an outcome between exposed and unexposed groups in cohort studies. Risk Difference (RD) or Cumulative Incidence Difference (CID): Measures the absolute difference in risk between exposed and unexposed groups in cohort studies. Incidence Rate Ratio (IRR): Compares the incidence rates of an outcome between exposed and unexposed groups. Incidence Rate Difference (IRD): Calculates the absolute difference in incidence rates between exposed and unexposed groups. Odds Ratio (OR): Assesses the strength of association between exposure and outcome in case-control studies. 2 Topic 2, 3 & 4; Observational Study Designs Details regarding the cross-sectional study design Cross-sectional studies collect data on exposure and outcome at the same time. The sample in cross-sectional studies should be representative of the study population. They are suitable for investigating short-term effects or health conditions in a population at the present moment. Cross-sectional studies are good for investigating stable exposures like genotypes, biological sex, eye color, blood type, or short-term stable effects. Survival selection occurs in cross-sectional studies, affecting the frequency of exposure at different ages. In the past, cross-sectional studies involved investigating exposure and outcome simultaneously in a selected population. Currently, cross-sectional studies gather data at a single point in time without following individuals over time. Prevalence proportion (PP), prevalence proportion ratio (PPR), and prevalence proportion difference (PPD) Prevalence proportion (PP) in epidemiology is calculated as the number of individuals with a disease at a given point in time divided by the total number of individuals in the study population at the same point in time. Prevalence proportion ratio (PPR) is calculated as the ratio of the prevalence proportion in the exposed group to the prevalence proportion in the non-exposed group. Prevalence proportion difference (PPD) is calculated as the difference between the prevalence proportion in the exposed group and the prevalence proportion in the non-exposed group. PPR provides a relative measure of the prevalence of a disease between two groups, while PPD gives an absolute measure of the difference in prevalence between the groups. These measures help in understanding the burden of a disease in different populations or groups based on their exposure status. Details regarding the case-contro l study design Case-control studies involve selecting cases and controls retrospectively to assess past exposures. They allow for the investigation of causality and are particularly useful for studying rare diseases or outcomes with long latency periods. Controls should be selected from the same source population as cases and should be at equal risk of the disease and exposure. Case-control studies are prone to recall bias but are less expensive and quicker to conduct compared to cohort studies. Odds and odds ratio (OR) Odds in epidemiology represent the probability of an event occurring compared to the probability of it not occurring. The odds ratio (OR) is a measure used in case-control studies to assess the strength of association between an exposure and an outcome. OR is calculated as the odds of exposure among cases divided by the odds of exposure among controls. An OR of 1 suggests no association, OR greater than 1 indicates a positive association, and OR less than 1 suggests a negative association between exposure and outcome. 3 OR helps in understanding the likelihood of an outcome occurring in the presence of an exposure compared to its absence. OR values greater than 1 indicate a positive association between exposure and outcome. Details regarding the cohort study design Cohort studies are prospective observational studies that follow a group of individuals over time to assess the development of new cases of a disease or outcome. They can also be retrospective if data is collected from past records but still follow a prospective timeline in the study. Cohort studies are considered the gold standard for observational studies as they allow for the assessment of causality. Three major types of cohort studies include survey- or population-based studies, studies based on exposed or high-risk groups, and studies based on selected samples. Cohort studies can be conducted in closed (fixed) populations where participants are followed from start to end, or in open (dynamic) populations where individuals enter and exit based on eligibility criteria. Measures of frequency in cohort studies include cumulative incidence (risk/chance) and incidence rate to assess the probability and speed of disease occurrence. Measures of association in cohort studies include relative risk (RR), cumulative incidence difference (CID), incidence rate ratio (IRR), and incidence rate difference (IRD) to quantify the association between exposure and outcome. Cohort studies involve selecting a group of individuals without the disease at the beginning of the study and following them over time to observe the development of the disease. They are prospective in nature, starting with exposure assessment and then following participants to assess outcomes. Cohort studies are useful for studying rare exposures, multiple outcomes, and establishing temporal relationships between exposure and outcome. They allow for the calculation of incidence rates and relative risks to measure the association between exposure and outcome. Cohort studies are less prone to bias compared to case-control studies but can be expensive and time-consuming to conduct. Cumulative incidence (Cl), incidence rate (IR), relat ive risk (RR), risk difference (RD)/ cumulative incidence difference (CID), incidence rate ratio (IRR), and incidence rate difference (IRD) Cumulative Incidence (CI) is the proportion of individuals who develop a specific outcome within a defined period. Incidence Rate (IR) is the rate at which new cases of a disease occur in a population over a specified period. Relative Risk (RR) compares the risk of an outcome in the exposed group to the risk in the unexposed group. Risk Difference (RD)/Cumulative Incidence Difference (CID) is the absolute difference in the cumulative incidence of an outcome between two groups. Incidence Rate Ratio (IRR) compares the incidence rates of an outcome in two different groups. Incidence Rate Difference (IRD) is the absolute difference in the incidence rates of an outcome between two groups. These measures are essential in epidemiological studies to quantify relationships between exposures and outcomes, assess interventions' impact, and understand the risk of developing certain conditions in different populations. 4 Topic 5; Randomized controlled trials and meta-analysis Details on randomized controlled tria ls (RCT) The document provides detailed information on randomized controlled trials (RCTs): 1. Definition of RCTs: o RCTs are described as a cohort study with intervention where the researcher allocates the treatment or exposure. o Randomized clinical trials are a type of RCT with a clinical hypothesis, such as testing a drug on a specific patient group. 2. Randomization: o Randomization is a key feature of RCTs where study participants are randomly assigned to different treatment or exposure groups. o The purpose of randomization is to ensure that baseline characteristics and confounding factors are equally distributed between groups, making them 'exchangeable' and independent of outcome characteristics. o The goal is to attribute any observed differences between groups at the end of the trial to the treatment differences. 3. Blinding: o Blinding involves masking treatments, often using placebos, to prevent knowledge about the treatment from influencing outcomes or evaluations made by researchers. o The aim of blinding is to ensure unbiased behavior and similar measurement errors in the compared groups. 4. Compliance: o Compliance refers to whether study participants adhere to the treatment they were randomized to and for how long. o Factors affecting compliance include participant regret, dropout rates, changes in treatment, and severe side effects necessitating treatment cessation. o Different data analysis methods like intention-to-treat or per-protocol analysis are used based on compliance considerations. 5. Intention-to-treat Analysis: o In intention-to-treat analysis, participants are analyzed based on the treatment they were supposed to receive regardless of actual compliance. o This method maintains randomization in data analysis to attribute differences between groups to treatment effects, even if some participants did not comply. 6. Per-protocol Analysis: o Per-protocol analysis involves analyzing participants based on the treatment they actually received, potentially leading to loss of randomization and comparability between groups. o Differences observed in per-protocol analysis may be due to compliance variations rather than treatment effects. 7. Ethical Considerations: o Ethical principles in RCTs include ensuring participants understand the consequences of participation, focusing on beneficial outcomes, and maintaining equipoise between compared treatments. o It is unethical to continue an experiment if there is clear evidence of effectiveness, harm, or no effect. Details on meta-analyses 1. Definition: 5 o A meta-analysis is a statistical method used to combine and analyze findings from multiple studies that investigate the same association or research question. 2. Steps in Meta-analysis: o Step 1: Identification of relevant published studies based on predefined selection criteria, including methodological quality. Publication bias, where the publication of results may depend on the significance and direction of effects, is a concern. o Step 2: Collocation of effect estimates, typically measures of association with confidence intervals. o Step 3: Calculation of a joint estimate across the studies to provide an overall conclusion. 3. Publication Bias: o Publication bias occurs when the publication of research results is influenced by factors beyond research quality, such as the hypothesis tested or the significance of effects. o This bias can lead to the file drawer effect, where negative results are less likely to be published, skewing the overall research findings. 4. Results Presentation: o Results of a meta-analysis are often summarized in a forest plot. o The forest plot displays effect estimates and confidence intervals for each study, with the size of each study's marker indicating its weight in the analysis. o The joint effect estimate and confidence interval are typically shown at the bottom of the plot. 5. Example: o An example from the document involves a meta-analysis by Weiss et al. in 2000 on the association between circumcision of men and the risk of HIV infection in Sub-Saharan Africa, incorporating findings from 15 studies. 6. Pros and Cons: o Pros:  Utilizes existing data from published articles.  Rapid method of analysis.  Consistency in direction of effects across multiple studies can support associations. o Cons:  Difficulty in selecting relevant and high-quality studies.  Publication bias can impact the conclusion on causality, potentially leading to an overestimation of effects.  The quality of a meta-analysis is limited by the quality of the individual studies included. 6 Topic 6; Bradford-Hill's causal criteria 1. Strength of Association: o The study reports odds ratios for behavioral problems in children with both prenatal and postnatal exposure to cell phones. The odds ratio for a higher overall behavioral problems score was 1.80 (95% confidence interval 1.45–2.23) in children with combined exposure. This indicates a moderate to strong association between cell phone exposure and behavioral problems in children. 2. Consistency: o The study mentions that greater odds ratios for behavioral problems were observed for children with possible prenatal or postnatal exposure to cell phone use. This consistency in the association across different exposure scenarios adds weight to the argument for a potential causal relationship. 3. Specificity: o The study focuses on the specific relationship between cell phone exposure during pregnancy and early childhood with behavioral problems in children. It examines this particular association rather than a broad range of outcomes, aligning with the specificity criterion. 4. Temporality: o The study design involves assessing exposure to cell phones during pregnancy and early childhood and then evaluating behavioral problems in children at the age of 7. This temporal sequence supports the idea that exposure precedes the outcome, meeting the temporality criterion. 5. Biological Gradient: o The study explores possible dose-response patterns by considering proxies of prenatal exposure intensity such as the number of times spoken per day, location of the phone when not in use, and proportion of time the phone was turned on. This analysis to evaluate dose-response relationships aligns with the biological gradient criterion. 6. Plausibility: o While the study mentions that the associations observed may be noncausal and could be due to unmeasured confounding, it also highlights the vulnerability of fetuses and children to external exposures during critical stages of brain development. This suggests a plausible biological mechanism for how cell phone exposure could potentially impact behavioral outcomes in children. 7. Coherence: o The study discusses the importance of cognitive abilities and learning in early development, which aligns with the observed behavioral difficulties in children exposed to cell phones prenatally and postnatally. This coherence between exposure and outcome supports a potential causal relationship. 8. Experiment: o Experimental research mentioned in the document indicates that exposure to radiofrequency fields might affect nonspecific neurologic performance such as attention. This experimental evidence supports the plausibility of a causal relationship between cell phone exposure and behavioral problems in children. In conclusion, the study on prenatal and postnatal exposure to cell phone use and behavioral problems in children demonstrates several aspects of Bradford-Hill's causal criteria, suggesting a potential association between cell phone exposure and behavioral difficulties in children. 7 Topic 7; Sources of error in epidemiological studies Precision and validity 1. Precision: o Precision as random error. o Table shows the relationship between size (mm) and % for precision (random error). o Precision is associated with random error, which can affect the accuracy of measurements in epidemiological studies. 2. Validity: o Validity as systematic error. o Table illustratung the relationship between size (mm) and % for validity (systematic error). o Validity is linked to systematic error, which can impact the reliability and consistency of results in epidemiological studies. Internal and external validity 1. Internal Validity: o Internal validity as a process at any stage of inference that tends to produce results departing systematically from the true values. o Errors in the conception, design, collection, analysis, interpretation, publication, or review of data can lead to results or conclusions that are systematically different from the truth, impacting internal validity. o Various biases can affect internal validity, such as drop-outs, bad memory, non-responders, and a list of biases like recall bias, interviewer bias, and others. o The Danish 1905 Cohort study is cited as an example, where differences between participants and non-respondents led to potential biases affecting internal validity. o Strategies to address confounding, such as restriction, stratification, matching, and multiple variable regression analysis, are discussed in the context of maintaining internal validity. 2. External Validity (or generalization): o Importance of studying diseases in humans to gain accurate insights, rather than relying solely on studies conducted in mice. o Genetic differences between mice and humans, particularly in immune system, stress response, and metabolism, are factors affecting external validity. o Diseases are a complex interplay between genes and the environment, underscoring the need to consider both aspects for valid conclusions. o Issues related to biases in research findings from studies in mice, overselling results, and simplification of stories. o Significance of considering the generalizability of research findings to ensure that conclusions drawn from studies are applicable and relevant beyond the specific study population. Selection bias, information bias, and confounding 1. Selection Bias: o Selection bias is defined as errors caused by systematic differences between individuals who participate in a study and those who do not. o Selective recruitment of individuals based on certain characteristics for exposure and outcome can lead to differences in the relationship between exposure and outcome among study participants compared to those who could theoretically participate but do not. 8 o The importance of considering selection bias in comparative studies, its association with both exposure and outcome, and its impact on study conclusions are highlighted. o Examples from the Danish 1905 Cohort study are provided to illustrate how differences between participants and non-respondents can introduce selection bias. o Need to address selection bias based on the scientific question, study design, and data used to ensure the validity of study findings. 2. Information Bias: o Errors caused by systematically incorrect information about study participants. o Misclassification, both non-differential and differential as a form of information bias that can affect the classification of individuals, exposure, outcome, or levels of disease/exposure. o Examples of recall bias, interviewer bias, and the impact of inaccurate information on study results are discussed in the document. o The importance of accurate information about study participants to avoid over- or underestimation of associations and draw correct conclusions. o An example of misclassification in a cohort study related to alcohol intake and self-rated health is provided to illustrate the impact of information bias on study outcomes. 3. Confounding: o Confounding is defined as the confusion of the effect of one factor with another factor in the context of epidemiological studies. o The document explains that a confounding variable is associated with both the exposure and outcome, distributed unequally among groups being compared, and not part of the causal pathway from exposure to outcome. o Classic confounders like age, sex, social class, and disease risk factors are mentioned as factors that can introduce confounding in studies. o Methods to avoid confounding, such as restriction, stratification, matching, and multiple variable regression analysis, are discussed in the document. o The document highlights the importance of identifying and controlling for confounding to ensure accurate interpretation of study results and avoid misleading conclusions. Effect modification and interaction 1. Effect Modification: o Effect modification is defined as the phenomenon where the effect of an exposure on an outcome is modified by the levels of another factor, known as an effect modifier. o Effect modification is also referred to as statistical interaction and is due to complex causality. o Effect modification should be described rather than eliminated, as it provides valuable insights into how different factors influence the relationship between exposure and outcome. o Strategies to address effect modification involve stratifying the analysis based on the suspected effect modifier to examine the association separately for each level of the effect modifier. o Examples of effect modification include factors like sex, age, UV radiation, genetic markers, and specific diseases that can modify the effect of an exposure on an outcome. 2. Interaction: o Interaction, synonymous with effect modification, is described as a statistical concept where the effect of one variable on an outcome is dependent on the level of another variable. o Interaction should be reported as a finding rather than a bias to be avoided, and epidemiological analysis aims to discover and describe interaction effects. o Examples of interactions, such as those between alcohol and lung cancer, smoking and osteoporosis, and genetic markers in disease treatment illustrate how the relationship between exposure and outcome can be influenced by other factors. 9 o Understanding and reporting interaction effects are essential for a comprehensive analysis of epidemiological data and for gaining insights into the complexity of causal relationships. 10 Topic 8; Diagnostics Sensitivity 1. Definition: o Sensitivity is defined as the proportion of individuals with the disease who have a positive test result. o It is calculated using the formula: Sensitivity = TP / (TP + FN), where TP represents true positives and FN represents false negatives. 2. Importance: o Sensitivity is crucial for ruling out disease. o A highly sensitive test will rarely miss people with the disease, making it useful when false negatives are undesirable. 3. Trade-off: o The document mentions that there is typically a trade-off between sensitivity and specificity in diagnostic tests. o Balancing sensitivity and specificity is essential to ensure the accuracy and reliability of a diagnostic test. 4. Example: o The document includes examples illustrating the relationship between sensitivity and disease prevalence in diagnostic tests. In conclusion, sensitivity plays a vital role in diagnostic testing by determining the test's ability to correctly identify individuals with the disease. Understanding sensitivity is essential for evaluating the performance and reliability of diagnostic tests in clinical and research settings. Specificity The document provides information on specificity in the context of diagnostics. Here are the key points explicitly mentioned in the document regarding specificity: 1. Definition: o Specificity is defined as the proportion of individuals without the disease who have a negative test result. o It is calculated using the formula: Specificity = TN / (TN + FP), where TN represents true negatives and FP represents false positives. 2. Importance: o Specificity is crucial for confirming a diagnosis. o A highly specific test will rarely misclassify individuals as sick when they are not, making it useful when false positives are undesirable. 3. Trade-off: o The document notes that there is typically a trade-off between sensitivity and specificity in diagnostic tests. 11 o Balancing sensitivity and specificity is essential to ensure the accuracy and reliability of a diagnostic test. 4. Example: o The document includes examples illustrating the relationship between specificity and disease prevalence in diagnostic tests. Understanding specificity is essential for evaluating the performance and reliability of diagnostic tests, as it indicates the test's ability to correctly identify individuals without the disease. Specificity complements sensitivity in assessing the overall effectiveness of a diagnostic test in clinical and research settings. ROC Curves 1. Definition: o An ROC curve expresses the relationship between sensitivity and specificity of a diagnostic test. o It is a graphical representation that illustrates the trade-off between sensitivity and specificity at various thresholds of a test. 2. Purpose: o ROC curves can be used to determine the best cut-off point for a test. o They help in evaluating the performance of a diagnostic test by showing how well it distinguishes between true positives and false positives. 3. Interpretation: o A test that performs well will have an ROC curve lying in the upper left corner of the graph. o ROC curves that fall closer to the diagonal line indicate a test that is no better than chance. 4. Comparison: o ROC curves are valuable for comparing different tests for the same diagnosis. o The overall accuracy of a test can be described by the area under the ROC curve, where a larger area indicates a better test performance. Understanding ROC curves is essential for assessing the diagnostic accuracy and effectiveness of tests in distinguishing between individuals with and without a particular condition. ROC curves provide a visual representation of how well a test can discriminate between true positives and false positives, aiding in the evaluation and comparison of diagnostic tests in clinical and research settings. Predictive Values 1. Definition: o Predictive values, also known as posterior or post-test probabilities, indicate the probability of having or not having a disease based on a test result. o There are two types of predictive values: positive predictive value (PPV) and negative predictive value (NPV. 2. Calculation: o Positive Predictive Value (PPV) is calculated as TP / (TP + FP), where TP represents true positives and FP represents false positives. o Negative Predictive Value (NPV) is calculated as TN / (TN + FN), where TN represents true negatives and FN represents false negatives. 3. Importance: o PPV indicates the probability of having the disease if the test result is positive. o NPV indicates the probability of not having the disease if the test result is negative. 12 o Predictive values are not constant and change with the prevalence of the disease. 4. Example: o The document includes examples illustrating the calculation and interpretation of predictive values based on test results and disease prevalence. Understanding predictive values is crucial in clinical decision-making as they provide insights into the likelihood of disease presence or absence based on test results. PPV and NPV help healthcare professionals interpret diagnostic test outcomes and make informed decisions regarding patient care and treatment strategies. 13 Topic 9; Genetic epidemiology The human genome, genetic variation, haplotypes, and linkage disequilibrium The human genome encompasses all genetic information in humans, including genes and non-coding sequences. Genetic variation refers to diversity in DNA sequences among individuals, including SNPs, insertions, deletions, and structural variations. Haplotypes are sets of closely linked genetic markers on a chromosome inherited together, aiding in identifying genetic regions associated with traits or diseases. Linkage disequilibrium is the non-random association of alleles at different loci, providing insights into population genetics and recombination patterns. Genetic epidemiology involves studying genetic factors and their interactions to understand health and disease patterns. Linkage analysis helps identify markers associated with genes of interest, while LD analysis aids in tracking genes within families. These concepts are crucial for investigating inherited causes of diseases and traits in populations and families. Genetic epidemiology; familial clustering of diseases and traits, heritability, modes of inheritance, linkage analysis, and association studies Genetic epidemiology involves studying how genetic factors contribute to the occurrence of diseases and traits within populations. Familial clustering of diseases and traits refers to the tendency for certain conditions to run in families due to shared genetic and environmental factors. Heritability quantifies the proportion of phenotypic variation in a population that is due to genetic differences. Modes of inheritance, such as autosomal dominant, autosomal recessive, X-linked, or mitochondrial, describe how genes are passed down from parents to offspring. Linkage analysis is a method used to identify genetic markers that are inherited together with a gene of interest within families. Association studies investigate the relationship between genetic variants and traits or diseases in populations to identify genetic risk factors. Understanding these concepts is essential for unraveling the genetic basis of diseases and traits, informing prevention, diagnosis, and treatment strategies. Genetic epidemiology involves evaluating inherited causes of diseases in families and populations. Familial clustering studies examine the recurrence risk and familial risk ratios to assess the genetic contribution to disease. Heritability estimates the proportion of a trait's variance attributable to genetic factors. Modes of inheritance, such as Mendelian patterns (autosomal dominant, recessive, X-linked) or non- Mendelian inheritance, determine how traits are passed down. Sources of error in genetic epidemiology; population stratification Population stratification is a significant source of error in genetic epidemiology. It can lead to false positive or false negative findings in association studies. 14 Systematic differences in allele frequencies among subpopulations contribute to this error. Mitigation strategies include restricting analyses to homogeneous ancestral groups, using genomic control methods, and incorporating genetic ancestry information through techniques like principal components analysis. Proper consideration and adjustment for population stratification are essential for ensuring the validity and reliability of genetic association studies in diverse populations. 15 Topic 10; Type I and type II errors, power, and multiple testing Type I and type II errors Type I error: o Definition: The error of rejecting a null hypothesis when it is actually true. o Also known as a ‘false positive’. o Equals the significance level. Type II error: o Definition: The error of not rejecting (accepting) a null hypothesis when the alternative hypothesis is true. o Also known as a ‘false negative’. o Equals 1 minus the statistical power. Trade-off between type I and type II errors: o Example given using the story of "The boy who cried wolf" to illustrate the trade-off. o Villagers can avoid Type I errors by never believing the boy, but that will always cause a Type II error when there is a wolf around. o Similarly, they can always believe him and never make a Type II error, but that will cause lots of Type I errors (when the boy is lying). Exercise on preferences: o Question posed about preferring a small Type I error at the expense of a larger Type II error or a small Type II error at the expense of a larger Type I error. o Scenarios provided:  Testing a treatment against a deadly disease - the treatment is cheap and harmless.  Considering factors like treatment cost, side effects, and disease severity.  Performing a genetic association study. Power and power analysis 1. Strength of Association: o The study reports odds ratios for behavioral problems in children with both prenatal and postnatal exposure to cell phones. The odds ratio for a higher overall behavioral problems score was 1.80 (95% confidence interval 1.45–2.23) in children with combined exposure. This indicates a moderate to strong association between cell phone exposure and behavioral problems in children. 2. Consistency: o The study mentions that greater odds ratios for behavioral problems were observed for children with possible prenatal or postnatal exposure to cell phone use. This consistency in the association across different exposure scenarios adds weight to the argument for a potential causal relationship. 3. Specificity: o The study focuses on the specific relationship between cell phone exposure during pregnancy and early childhood with behavioral problems in children. It examines this particular association rather than a broad range of outcomes, aligning with the specificity criterion. 4. Temporality: o The study design involves assessing exposure to cell phones during pregnancy and early childhood and then evaluating behavioral problems in children at the age of 7. This temporal sequence supports the idea that exposure precedes the outcome, meeting the temporality criterion. 5. Biological Gradient: o The study explores possible dose-response patterns by considering proxies of prenatal exposure intensity such as the number of times spoken per day, location of the phone when not in use, and 16 proportion of time the phone was turned on. This analysis to evaluate dose-response relationships aligns with the biological gradient criterion. 6. Plausibility: o While the study mentions that the associations observed may be noncausal and could be due to unmeasured confounding, it also highlights the vulnerability of fetuses and children to external exposures during critical stages of brain development. This suggests a plausible biological mechanism for how cell phone exposure could potentially impact behavioral outcomes in children. 7. Coherence: o The study discusses the importance of cognitive abilities and learning in early development, which aligns with the observed behavioral difficulties in children exposed to cell phones prenatally and postnatally. This coherence between exposure and outcome supports a potential causal relationship. 8. Experiment: o Experimental research mentioned in the document indicates that exposure to radiofrequency fields might affect nonspecific neurologic performance such as attention. This experimental evidence supports the plausibility of a causal relationship between cell phone exposure and behavioral problems in children. In conclusion, the study on prenatal and postnatal exposure to cell phone use and behavioral problems in children demonstrates several aspects of Bradford-Hill's causal criteria, suggesting a potential association between cell phone exposure and behavioral difficulties in children. Multiple testing Power: o Definition: The probability of correctly rejecting the null hypothesis when the alternative hypothesis is true. o Represents the ability to detect an effect if the effect actually exists. Power calculation: o Example given for power calculation in a simple case-control design. o Reference to a power calculator link for a study of 500 cases and controls for a disease with a population frequency of 0.01. o Factors mentioned for power calculation include significance level, disease model, genotype relative risk, and disease allele frequency. Indirect allelic association: o Mentioned in the document. GWAS - sample size requirements: o Imaginary GWA study details provided in a table format. o Information includes the need for cases and controls to reach a genome-wide significance level (P < 5 x 10-8) with a power of ≥ 0.8. o Factors considered are case-control study design, disease prevalence, allelic relative risks, and risk allele frequencies. Power as a function of sample size, risk allele frequency, and OR: o Reference to a special issue on statistical inference focusing on different aspects of the issue. 17 Topic 11; Critical Assessment of Research - are All Research Find False? Critical assessment of research The authors had full access to the data and contributed to the design, analysis, and interpretation of the study. Stratifying by baseline characteristics showed varying risks, with different educational levels impacting the risk of death from any cause. The research focused on the Danish population above 40 years of age from 1980 to 2006. It examined the association between skin cancer (non-melanoma and cutaneous malignant melanoma) and the risk of myocardial infarction, hip fracture, and death from any cause. The study utilized a large sample size and data from national registries. Statistical analysis was robust, incorporating odds ratios, hazard ratios, multifactorially adjusted hazard ratios, and log-rank tests. Findings indicated varying risks of health outcomes for individuals with different types of skin cancer, with individuals with non-melanoma skin cancer having a lower risk of death from any cause compared to those without skin cancer. The study suggested a potential beneficial effect of sun exposure on health and emphasized the need for further research to draw causal conclusions. Limitations included the lack of information on smoking status and potential biases in outcome assessment. The study was supported by the Danish Heart Foundation, the Faculty of Health Sciences at the University of Copenhagen, and Herlev Hospital, Copenhagen University Hospital. The authors had full access to the data and contributed to the design, analysis, and interpretation of the study. Stratifying by baseline characteristics showed varying risks, with different educational levels impacting the risk of death from any cause. The document explicitly mentions that individuals with skin cancer, used as a marker of sun exposure, live on average around 6 years longer than individuals without skin cancer. Relevant Content from Document: o Individuals with skin cancer, indicating sun exposure, live around 6 years longer on average than those without skin cancer. Critical Assessment: o The study's finding of individuals with skin cancer living longer could be influenced by various factors:  Confounding Variables: There might be other variables not accounted for in the study that could be affecting the results. For example, lifestyle factors like diet, exercise, or socioeconomic status could play a role.  Selection Bias: The individuals included in the study may not be representative of the general population, leading to biased results.  Reverse Causation: It is possible that the relationship is not causal, and there could be underlying factors that both increase sun exposure and contribute to longevity.  Sample Size: The size of the study population could impact the reliability of the results. A larger sample size would provide more robust conclusions.  Study Design: The study design, such as retrospective or prospective, could influence the validity of the findings.  Data Collection Methods: The accuracy and reliability of data collection methods used in the study could affect the outcomes. 18 Conclusion: While the study's result of individuals with skin cancer living longer is intriguing, it is essential to critically analyze the methodology, potential biases, and other factors that could influence the observed association. Further research with rigorous study designs and controls would be necessary to confirm or refute these findings. Critical assessment of research findings involves considering factors like bias, statistical power, and prior probability of the relationship being true. Acknowledging biases and improving research standards are crucial for ensuring the validity of research findings. It is important to focus on the totality of evidence rather than individual significant findings to draw accurate conclusions. The document highlights concerns about the validity of many published research claims and emphasizes the prevalence of false research claims. Relying solely on statistical significance may not provide a complete picture of the truthfulness of research findings. Researchers should aim to present a more comprehensive representation and summary of research outcomes to enhance transparency and accuracy. Consider pre-study odds when designing experiments. Emphasize the need for multiple independent studies to validate research claims. Encourage upfront registration of studies, especially for randomized trials. Target large-scale evidence for research questions with high pre-study probability. Challenge narrow criteria for conducting large studies, especially related to specific drug marketing. Enhance understanding of the range of pre-study odds in research efforts. Factors influencing the truthfulness of research findings are highlighted, including study power, bias, number of studies on the same question, and the ratio of true to no relationships in a scientific field. Limitations of relying solely on statistical significance, especially with a p-value less than 0.05, are discussed as an ill-founded strategy for claiming conclusive research findings. 1. The study faced criticism primarily due to discrepancies between the stated protocol and the actual implementation of the study. 2. One of the methodological issues that led to criticism was the deviation from the protocol in participant recruitment. The protocol mentioned recruitment through advertising in media to individuals and organizations, but in reality, participants were selected from specific supermarket employees. 3. This deviation raised concerns about the representativeness of the sample and the generalizability of the study's findings. 4. The discrepancy between the planned recruitment method and the actual selection process likely undermined the scientific rigor and validity of the study. 5. Such deviations from the study protocol can introduce bias and affect the reliability of the research outcomes. 6. The criticism within the scientific community was likely fueled by these methodological issues that compromised the integrity and credibility of the study. The document outlines a randomized controlled trial called DANMASK-19, which aims to investigate the efficacy of face masks in reducing the risk of SARS-CoV-2 infection among individuals in the community. 1. Study Design: 19 o The trial is a two-arm, unblinded, randomized controlled trial involving 6,000 participants randomly assigned to either use face masks or not for a 30-day period. o The study design allows for a direct comparison between individuals wearing face masks and those not wearing them, which is essential for evaluating the effectiveness of face masks in preventing SARS-CoV-2 infection. 2. Inclusion Criteria: o The inclusion criteria specify that adults above 18 years of age, spending more than three hours per day outside the home with exposure to other people, and without prior COVID-19 infection are eligible to participate. o These criteria help in selecting a relevant sample population for the study, focusing on individuals who are at risk of exposure to the virus. 3. Endpoints: o The primary endpoint of the study is the difference in the number of infected individuals between the two study groups based on positive swabs, antibody tests, or hospital-based diagnoses. o Secondary endpoints include assessing other respiratory viral infections, providing a comprehensive evaluation of the impact of face masks on various infections. 4. Interventions: o Participants are provided with face masks and testing kits, along with detailed instructions for self- testing and monitoring. o The study ensures standardized procedures for both groups, enhancing the reliability of the results. 5. Data Collection and Analysis: o Data collection involves surveys, swabs, and antibody testing, with statistical analysis planned to compare outcomes between the face mask and control groups. o The statistical methods outlined in the document, including power calculations and statistical significance levels, demonstrate a rigorous approach to data analysis. 6. Ethical Considerations: o The study obtained ethical approval and adheres to data protection regulations, ensuring participant confidentiality and informed consent. o These ethical considerations are crucial for maintaining the integrity of the research and protecting the rights of the participants. 7. Potential Limitations: o The study acknowledges challenges related to mask compliance, durability, and behavioral changes, which could impact the effectiveness of face masks. o The document highlights the need for further research on mask durability and reuse, indicating areas where the study findings may have limitations. In conclusion, the DANMASK-19 trial is a well-structured research endeavor that aims to provide valuable insights into the efficacy of face masks in preventing SARS-CoV-2 infection. By addressing key research questions and following rigorous methodologies, the study has the potential to inform global recommendations on the use of face masks during the COVID-19 pandemic and future viral outbreaks. The critical assessment of research findings emphasizes the prevalence of false research findings and the factors contributing to this issue. Factors Influencing False Research Findings: o Smaller studies are less likely to yield true research findings. o Studies with smaller effect sizes are less likely to produce true results. o Greater flexibility in designs, definitions, outcomes, and analytical modes decreases the likelihood of true research findings. 20 o Financial interests and prejudices can lead to less true research findings. o Scientific fields with high activity are less likely to produce true research findings. Improving Research Practices: o Clearly differentiate between statistical significance and theoretical importance of findings. o Share data, research protocols, and other study information transparently. o Avoid presenting findings as more conclusive than supported by the data. o Disclose any reuse of previously published data. o Avoid unnecessary division of results across multiple publications. o Refrain from formulating or altering hypotheses post-results. o Avoid collecting additional data solely to achieve statistical significance. o Offer authorship to collaborators who have contributed sufficiently. Agreement with Ioannidis: o Acknowledging the potential for false research findings is crucial for maintaining scientific integrity. o While not all research findings may be false, the document highlights the significant impact of various factors on the validity of research outcomes. By adhering to transparent and rigorous research practices, researchers can mitigate the risk of false findings and enhance the credibility and reliability of scientific research in the field of biomedicine. 21 Topic 12; Design your own study What is the hypothesis and why did this particular hypothesis was chosen? The hypothesis of the study is that vitamin C supplementation is expected to reduce the number of sick days in smokers. This hypothesis was chosen based on several key factors explicitly mentioned in the document: 1. Known Role of Vitamin C: Vitamin C is highlighted as essential for the body, acting as an antioxidant and supporting the immune system. Smokers often have lower levels of vitamin C due to increased demand caused by cigarette smoke, which weakens their immune system. Therefore, it is hypothesized that supplementing with vitamin C could strengthen the immune system of smokers, potentially reducing the frequency and duration of illnesses they experience. 2. Cost-Effective Intervention: The study aims to explore effective and cost-effective interventions to improve the health of smokers and reduce associated costs. By focusing on vitamin C supplementation, which is relatively accessible and has low toxicity at the chosen doses, the hypothesis aligns with the goal of finding practical solutions to address the health challenges faced by smokers. 3. Public Health Concern: Smoking is identified as a significant public health problem with implications for individual well-being and healthcare systems. The hypothesis of vitamin C supplementation leading to reduced sick days directly targets the health impact of smoking, making it a relevant and specific hypothesis to investigate in the context of improving the health outcomes of smokers. 4. Scientific Rationale: The hypothesis is grounded in the scientific understanding of vitamin C's role in immune function and disease prevention. By focusing on sick days as a measurable outcome, the hypothesis sets a clear and quantifiable target for assessing the effectiveness of vitamin C supplementation in smokers. In summary, the hypothesis that vitamin C supplementation will reduce the number of sick days in smokers was chosen because it addresses a specific health concern related to smoking, aligns with the known benefits of vitamin C, offers a practical and cost-effective intervention, and provides a clear and measurable outcome for the study. What study design have been chosen and why was this study design chosen over any of the other study designs? The study design chosen for this research is a Randomised Controlled Trial (RCT). Several reasons explicitly mentioned in the document support the selection of this study design over other alternatives: 1. Objective of the Study: The primary objective of the study is to investigate the impact of vitamin C supplementation on the frequency of sick days among smokers. An RCT is well-suited for this objective as it allows for the comparison of different interventions (placebo, mid-subsidy, high-subsidy) in a controlled manner to assess their effects on the outcome of interest. 2. Impartiality and Minimization of Bias: An RCT enables the researchers to track multiple subject groups concurrently while maintaining impartiality in analysis and procedures. By randomly assigning participants to different groups (control, mid-subsidy, high-subsidy), biases are minimized, ensuring a more objective evaluation of the impact of vitamin C supplementation on sick days among smokers. 3. Double-Blind Setup: The study incorporates a double-blind setup where neither participants nor researchers know the group assignments. This design feature further reduces biases that could influence the results, ensuring that the outcomes are more reliable and not influenced by preconceived notions or expectations. 4. Control of Confounding Variables: RCTs are effective in controlling for confounding variables as they distribute these variables equally among the different groups. By ensuring that confounding variables are balanced across the groups, the study can more accurately attribute any differences in outcomes to the interventions being tested. 22 5. Scientific Rigor: RCTs are considered the gold standard in research for establishing cause-and-effect relationships between interventions and outcomes. The rigorous design of an RCT provides a strong basis for drawing conclusions about the effectiveness of interventions, such as vitamin C supplementation in this case. In conclusion, the Randomised Controlled Trial (RCT) study design was chosen for this research over other designs because of its ability to compare interventions, maintain impartiality, minimize biases, control confounding variables, and provide a high level of scientific rigor in evaluating the impact of vitamin C supplementation on sick days among smokers. How will the study participants be select and recruited? What information will be collected and how? What are the inclusion and exclusion criteria and why those? The study participants will be selected and recruited based on specific criteria outlined in the document: 1. Selection and Recruitment: o Participants will be targeted through E-boks messages aimed at individuals aged 18-29 who are working and smoke more than or equal to 15 cigarettes a day. o A questionnaire will be used to gather information on age, smoking status, smoking history, and health status to determine eligibility for the study. o Participants meeting the criteria will be randomly assigned to one of three groups: control group (placebo), mid-subsidy group (125mg of vitamin C daily), or high-subsidy group (500mg of vitamin C daily). 2. Data Collection: o Data will be collected through monthly clinical visits for the first 3 months of the study and then every 3 months thereafter. o Information collected during visits will include the total number of sick days experienced by participants. o Participants will fill out questionnaires during each visit to provide updates on their life and general health status to ensure ongoing eligibility for the study. 3. Inclusion Criteria: o Age group 18-29: Young adults are targeted for the study. o More than or equal to 15 cigarettes a day: Focus on heavy smokers to observe the impact of vitamin C on individuals who are more susceptible to falling sick due to smoking's effects on the immune system. 4. Exclusion Criteria: o Pre-existing health conditions: Participants with chronic diseases are excluded to ensure that differences in sick days are primarily due to the presence or absence of vitamin C supplementation, not pre-existing health conditions that could affect sickness levels. 5. Rationale for Inclusion and Exclusion Criteria: o Inclusion Criteria: By focusing on young heavy smokers, the study aims to observe the effects of vitamin C on a population more likely to experience health issues due to smoking. This targeted approach increases the likelihood of detecting any impact of vitamin C supplementation on sick days. o Exclusion Criteria: Excluding participants with pre-existing health conditions helps isolate the effects of vitamin C on sick days, as the presence of chronic diseases could confound the results. This ensures that any differences observed in sick days can be more confidently attributed to the intervention (vitamin C supplementation). How will exposure be measured? Outcome? Confounders? Is there anything to be aware of in that connection? Self-reporting? If diagnostic tests will be used what will then be the requirements for the test in terms of sensitivity, specificity, positive predictive value and negative predictive value? 23 1. Exposure Measurement: o Exposure in this study refers to the intake of vitamin C. Participants will be assigned to three categories: placebo (control group), mid-subsidy (125mg of vitamin C daily), and high-subsidy (500mg of vitamin C daily). o The intake of vitamin C will be monitored by ensuring participants take the assigned dosage daily as per their group allocation. 2. Outcome Measurement: o The primary outcome of the study is the impact of vitamin C supplementation on the frequency and duration of sick days among smokers. o The outcome will be measured by tracking the total number of sick days experienced by participants at specific time points throughout the study period. 3. Confounders: o Confounding variables are factors that may influence the relationship between the exposure (vitamin C supplementation) and outcome (sick days). In this study, confounders could include factors like age, gender, overall health status, and lifestyle habits. o To address confounders, participants will be randomly assigned to different groups to ensure an equal distribution of potential confounding variables across the groups. 4. Considerations: o Self-Reporting: Participants will self-report the number and length of sick days, which may introduce bias due to misrepresentation or misremembering of sickness. Researchers should be aware of this limitation and consider cross-referencing self-reported data with other objective measures if possible. o Diagnostic Tests: The study does not mention the use of diagnostic tests. If diagnostic tests were to be used, requirements for sensitivity, specificity, positive predictive value, and negative predictive value would need to be established based on the specific tests used and the conditions being assessed. In conclusion, exposure to vitamin C will be measured by monitoring daily intake, the outcome will be assessed by tracking sick days, confounders will be addressed through random group assignment, and researchers should be cautious of potential biases in self-reported data. If diagnostic tests were to be incorporated, appropriate requirements for test performance metrics would need to be defined based on the specific tests utilized. What biases to be aware of? How will these potentially affect results? Which confounders? Effect modifiers? And what about the external validity? 1. Biases to be Aware of: o Self-Reporting Bias: Participants may inaccurately report the number and length of sick days, leading to measurement bias. o Selection Bias: If participants who agree to participate differ systematically from those who decline, it could impact the generalizability of the results. o Detection Bias: Differences in how sick days are identified or reported between the groups could introduce bias. o Performance Bias: Variations in how participants adhere to the assigned vitamin C regimen may affect outcomes. 2. Potential Impact of Biases on Results: o Self-reporting bias could lead to inaccurate data on sick days, affecting the reliability of the results. o Selection bias may limit the generalizability of the findings to the broader population of smokers. o Detection and performance biases could influence the perceived effectiveness of vitamin C supplementation on sick days. 3. Confounders: o Age: Younger participants may respond differently to vitamin C compared to older individuals. 24 o Gender: Gender differences could impact the immune response and susceptibility to illnesses. o Smoking History: Variations in smoking duration or intensity could influence the outcomes. o Overall Health Status: Participants' general health may affect their response to vitamin C supplementation. 4. Effect Modifiers: o Smoking Intensity: The number of cigarettes smoked per day could modify the effect of vitamin C on sick days. o Dietary Habits: Participants' overall diet, including vitamin C intake from food sources, may interact with supplementation effects. o Physical Activity: Levels of physical activity could influence the immune response and health outcomes. 5. External Validity: o Generalizability: The study's findings may be limited to the specific population of young heavy smokers aged 18-29 who smoke more than or equal to 15 cigarettes a day. o Applicability: Results may not be directly applicable to older smokers, light smokers, or individuals with different smoking habits. o Contextual Factors: The study's setting, recruitment methods, and intervention design may impact the external validity of the findings when extrapolating to other populations or settings. In summary, researchers should be vigilant about biases such as self-reporting and selection bias, consider confounders like age and smoking history, explore effect modifiers such as smoking intensity, and acknowledge limitations in external validity when interpreting and applying the study results to broader populations or contexts. How will results be presented, i.e.. which measures of frequency/association will be calculated? How should they be interpreted? 1. Presentation of Results: o The study will calculate measures of frequency and association to analyze the impact of vitamin C supplementation on sick days among smokers. o Key measures will include the average number of sick instances and the average length of sick days per group at different time points throughout the study. 2. Measures of Frequency/Association: o Incidence Rate (IR): IR will be calculated to show the susceptibility of participants to falling sick. It compares the number of sick instances per group over the total number of days not sick. o Average Length of Sick Days: This measure indicates the severity of sickness and will be calculated by dividing the total length of sick days by the total number of participants in each group. o Percentage of Sick Days: This measure shows the time spent being sick and will be calculated by dividing the total length of sick days by the total participant days in each group. 3. Interpretation: o Incidence Rate (IR): A higher IR indicates a higher frequency of falling sick in a particular group compared to others. Lower IR suggests a lower susceptibility to illness. o Average Length of Sick Days: A higher average length of sick days indicates longer durations of sickness within a group, while a lower average length suggests shorter durations of illness. o Percentage of Sick Days: A higher percentage of sick days indicates a larger proportion of time spent being sick, while a lower percentage suggests less time spent sick. In conclusion, the study will present results using measures such as Incidence Rate, Average Length of Sick Days, and Percentage of Sick Days to assess the impact of vitamin C supplementation on sick days among smokers. These measures will help interpret the frequency and severity of sickness within each group and evaluate the effectiveness of the intervention. 25 What statistical test to perform to infer statistical significance (this will depend on the data type of exposure and outcome, if confounders will be included, etc)? The statistical test to infer statistical significance in the study evaluating the impact of vitamin C supplementation on sick days among smokers will depend on various factors such as the data type of exposure and outcome, inclusion of confounders, and the distribution of the data. Here are the considerations for selecting the appropriate statistical test: 1. Data Type: o If the data is normally distributed and continuous, a t-test could be used to compare means between groups. o For non-normally distributed data or small sample sizes, a non-parametric test like the Wilcoxon rank- sum test may be more appropriate. 2. Inclusion of Confounders: o If confounders are included and need to be adjusted for, analysis of covariance (ANCOVA) could be utilized to compare group means while controlling for confounding variables. 3. Multiple Time Points: o For repeated measures data at multiple time points, a repeated measures ANOVA or mixed-effects model could be employed to assess changes over time within and between groups. 4. Effect Modification: o If there are effect modifiers that need to be considered, interaction terms can be included in regression models to assess the impact of these modifiers on the relationship between vitamin C supplementation and sick days. 5. Interpretation: o The statistical test results will provide information on whether there is a significant difference in sick days between the control group and the mid-/high-subsidy groups. o The p-value obtained from the statistical test will indicate whether the observed differences are statistically significant (typically using a significance level of 0.05). In summary, the choice of statistical test will depend on the nature of the data, presence of confounders, consideration of effect modifiers, and the need to analyze changes over multiple time points. The selected test should appropriately account for these factors to draw valid conclusions regarding the impact of vitamin C supplementation on sick days among smokers. What about Type I and Type II errors? How many individuals will be included? Any multiple testing issues? 1. Type I and Type II Errors: o In the study, Type I error (false positive) occurs if the null hypothesis is incorrectly rejected, suggesting a significant effect of vitamin C supplementation on sick days when there is none. o Type II error (false negative) happens if the null hypothesis is not rejected when there is a true effect of vitamin C supplementation on sick days. o The significance level (usually set at 0.05) helps control the Type I error rate. Researchers must be cautious not to interpret results as significant if they are due to chance (Type I error) or overlook true effects (Type II error). 2. Sample Size: o The study aims to recruit at least 400 participants based on the sample size calculation. This sample size is determined to provide adequate statistical power to detect meaningful differences in sick days between the control and subsidy groups. o The participants will be randomly assigned to the control, mid-subsidy, and high-subsidy groups to ensure unbiased comparisons and reliable results. 3. Multiple Testing: 26 o Multiple testing issues may arise if the same data is tested for multiple outcomes or at multiple time points without adjusting the significance level. o To address multiple testing concerns, researchers should consider adjusting the significance level using methods like Bonferroni correction to reduce the risk of false positives. o By adjusting for multiple comparisons, the study can maintain the overall Type I error rate at an acceptable level and minimize the likelihood of incorrectly identifying significant results due to chance. In conclusion, the study will need to carefully consider Type I and Type II errors, recruit a sufficient number of participants to ensure statistical power, and address multiple testing issues to maintain the integrity and validity of the findings regarding the impact of vitamin C supplementation on sick days among smokers. Are there any ethical concerns? Yes, there are ethical considerations that need to be addressed in the study evaluating the effects of vitamin C supplementation on sick days among smokers. Some of the key ethical concerns include: 1. Informed Consent: o Participants must be fully informed about the study objectives, procedures, potential risks, benefits, and their right to withdraw at any time without consequences. o Informed consent should be voluntary, without coercion, and participants should have a clear understanding of what participation entails. 2. Participant Safety: o Ensuring participant safety is paramount. Researchers must monitor participants for any adverse effects related to vitamin C supplementation and take appropriate action if any safety concerns arise. o If significant health improvements or impairments are observed, the study may need to be halted to protect participant well-being. 3. Confidentiality and Data Protection: o Participant data should be kept confidential and protected to maintain privacy. Data should be anonymized and stored securely to prevent unauthorized access. o Researchers must adhere to data protection regulations to safeguard participant information. 4. Beneficence and Non-Maleficence: o Researchers should prioritize the well-being of participants (beneficence) by conducting the study in a manner that maximizes benefits and minimizes harm. o Non-maleficence requires researchers to do no harm, ensuring that the study does not expose participants to unnecessary risks or harm. 5. Fair Participant Selection: o Participants should be selected fairly based on inclusion and exclusion criteria without discrimination. The selection process should be transparent and unbiased. 6. Ethical Review: o The study protocol should undergo ethical review by an Institutional Review Board (IRB) or Ethics Committee to ensure that the study meets ethical standards and participant rights are protected. 7. Communication of Results: o Clear communication of study results to participants is essential. Participants should be informed of the outcomes, whether significant or not, and provided with understandable explanations. By addressing these ethical considerations, researchers can conduct the study in a manner that upholds ethical principles, respects participant rights, and ensures the integrity and validity of the research findings. 27 28 EXTRA Topic 3; Biostatistics An introduction to basic statistics in epidemiology covering hypothesis testing, numerical and categorical data analysis, confidence intervals, and p-values. Hypothesis testing involves making scientific hypotheses and testing them using null hypotheses (Ho). Numerical data includes continuous, discrete, and nominal data types. Prediction intervals describe the range of observations within a sample. Confidence intervals (CI) are used for hypothesis testing and evaluating the precision of estimates. P-values indicate the probability of observing a difference between groups given the null hypothesis. Categorical data is analyzed using the chi2 test for differences between groups. Absolute and relative estimates are used to compare groups in epidemiological studies. Statistical significance is determined by the p-value, with a cutoff of