EFFAS_MR.pdf PDF
Document Details
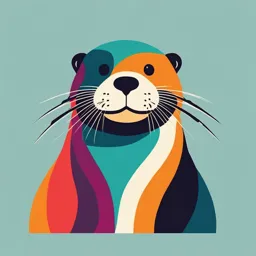
Uploaded by InspirationalMoose
Tags
Summary
This document provides an overview of financial risk management, focusing on market risk. It delves into approaches for quantifying potential losses in financial markets and discusses various market risk metrics.
Full Transcript
14 PRINCIPLES OF RISK MANAGEMENT Credit risk is segmentable into 4 major groups: 1.1.2.2 Market Market risk is the exposure implicitly assumed in positions adopted in the financial markets inherent to changes in the values of four major groups of financial assets: Issuer Settlement Delivery Counterp...
14 PRINCIPLES OF RISK MANAGEMENT Credit risk is segmentable into 4 major groups: 1.1.2.2 Market Market risk is the exposure implicitly assumed in positions adopted in the financial markets inherent to changes in the values of four major groups of financial assets: Issuer Settlement Delivery Counterparty The metrics used for the quantification of credit risk are usually of a structural nature and will aim to measure the potential losses that may occur throughout the life of the contracted operations. It arises from the eventuality that losses are generated due to non-compliance with the payment obligations of the borrowers, as well as losses of value due to the simple deterioration of the economic situation and the solvency of their assets. Concentration risk is also considered, understood as exposures with a loss potential large enough to affect the Entity’s solvency, derived from both the individual concentration of borrowers and sectoral concentration. The establishment of limits in line with the entity’s risk appetite is one of the most important binding frameworks in the risk function. Limits should be easily implementable and measurable, and should be adapted to the type of risk to which they are associated. The main drivers to be considered are: -- The process of requesting approval of limits should be one of the initial steps in business activity. -- The proposal must come from the unit that will adopt them (with the support of the risk area) to facilitate their technical evaluation, before being submitted to higher levels for approval. The follow-up returns to the risk area and may coexist with a middle office within the business unit. -- Equities: stocks: indexes, derivatives on these, etc. -- Interest rates: deposits, bonds, derivatives on these, etc. -- Exchange rates: purchase/sale of currencies, derivatives on them, etc. -- Commodities: purchase/sale of commodities, gold, oil, etc. Market risk is induced by the positioning acquired in the different areas described above. It is fundamentally induced by the volatility that the prices of all variables quoted in these markets usually present when they are subject to the forces of demand and supply that concur in the free markets. The roles of each of these financial assets listed on the markets cause a great diversity in the levels of volatility, as well as relative causal behaviors that generate uncertainty about the future values of the positions adopted. We can say that the future evolution of the prices of these financial variables have a stochastic component that generates a potential risk of losses that depend on the levels of market volatility that, in turn, are a function of aspects as diverse as monetary policies, the macroeconomic situation, political events, market perception, expectations, etc. Market risk has a more conjunctural character because it depends fundamentally on the positions that are adopted at any given time in the various financial assets; it is for this reason that the metrics intended for the quantification of market risk usually have a shorter horizon than credit risk. 15 The market risk measurement methods are divided into two main groups: a) One in which we quantify the potential changes in the value of our instruments as a consequence of unit variations in market variables. An example will be presented for the case of fixed-income assets. b) The estimation of the probability of occurrence of certain losses in a given future time horizon through measures that will be framed within the scope of the value at risk and scenario analysis by virtue, to a large extent, of historical information. This analysis would be incomplete if we do not answer the other great unknown: the probability of occurrence of these variations. Knowing this and combining it with the estimated magnitude of loss, we will have a measure of risk that will give us a little more information. The answer to this second question will be answered with the metrics that we will see later on, in the area of what we will call value at risk and/or stress scenarios. In both cases, we will estimate potential losses that may occur over certain time horizons and with certain confidence levels. In some cases, we will refer to losses in extreme market situations while others will be in normal market conditions. The objectives to be achieved are: To identify the valuation criteria in the different types of financial instruments. To exemplify the sensitivity metrics for the case of fixed income assets. To outline additional metrics that will provide “probabilistic” market risk information. To answer the second question on the measurement of potential market risks (What is the probability or risk of occurrence of such variations?) we must resort to different metrics. In the previous points, the first fundamental aspect in the analysis of market risks has been identified: the one that allows estimating the impact of a variation in exogenous variables on the instrument held in a portfolio. It should be noted that, in the metrics previously discussed, only the valuation model and its derivatives were used to measure the price elasticity with respect to exogenous market variables. At no time was historical information on these variables used and only what we have come to call the sensitivity of the instrument was evaluated. It should be noted that, in order to achieve these objectives, the metrics we are going to use require, in one way or another, historical information on the evolution of the prices that determine the instruments whose risk we wish to quantify. This is a differential aspect of the metrics that will be discussed in the next topic. Having dealt with those market risk metrics that allow us to quantify sensitivities through the calculation of the first derivatives of their corresponding valuation functions, we will now deal with the metrics that will quantify losses and gains for certain confidence levels and for specified time horizons. If the previous metrics were independent of the behavior of market variables in the past and only dealt with the valuation models and variables that determined them, in these metrics, historical information will become especially relevant. Their treatment will take different forms and their application will respond to different methodological criteria that must be outlined by the analyst. 16 PRINCIPLES OF RISK MANAGEMENT The metrics around what we will call Value at Risk date back to the early 1990s. At that time, one of the world’s leading investment banks, JP Morgan, made a methodological proposal for calculating market risk under the name of Riskmetrics. Since then, this methodology, implemented by the investment bank, has been a benchmark in the field of market risk. It has also given rise to methodological developments that have been evolving the proposal and have brought with them at least three different methodological currents that will be studied throughout the course. 1.1.2.3 Balance sheet (structural) Balance sheet risk or Asset-Liabilities Management (ALM) is the strategic management of the balance sheet of financial institutions from the perspective of market and liquidity risks. The ALM function is complemented by the management or monitoring of trading positions, funding and equity planning. It affects all financial institutions that have a balance sheet subject to business risks as a result of credit operations, regardless of their trading activities. The fundamental objectives pursued are the measurement, analysis and management of the aforementioned risk factors by: Maximizing the profit between assets and liabilities. Modulating the exposure of these factors according to the future vision of: -- Market variables. -- New business opportunities. -- Customer behavior that could lead to transfers from one instrument to another. Also complying with banking regulations in terms of control, internal supervision, reporting and good capital management practices is part of ALM. 1.1.3 Fundamental Methodologies In order to address the measurement of the different risk factors that exist in the financial field, an approxima- tion must be made that covers at least 4 perspectives. The first aims to quantify the exposure to the risk being measured. That is, it is a question of estimating the basis on which losses can occur. As these apply to the present value as to the base future values of the exposure, the determination is not always immediate, especially when it comes to estimating potential exposures that may occur at future points in time. The second has to respond to sensitivity exercises, that is, metrics that allow us to know what would be the impact on the value of a portfolio in the face of certain movements of the exogenous market variables. The third approach will take into account a probabilistic component that, in the first instance, will quantify losses under normal market conditions. That will be covered by Value At Risk measures implemented through 3 main streams of methodologies: Historical simulation. The Monte Carlo Simulation. Parametric techniques. Finally, the estimation of losses that may occur under extreme conditions will be treated by what is called stress testing. 1.1.3.1 Exposure The starting point for measuring market risk is the proper valuation of the different types of instruments. The exposure is defined by the current and potential value of the instruments. In this topic, we will address and identify the main criteria that must govern the valuation processes of instruments in the field of equities, fixed income, currencies, money markets and commodities. Each of them will have their own specificities, although the observation of market prices will be a common factor that will provide the necessary inputs for the valuation of the different types of finan- 17 cial instruments. The demand and supply of the various financial assets in the markets will determine the prices used for the valuation processes of the different instruments. As we have already seen, there are different activities that involve the assumption of market risks. The ways of measuring market risks fall into two large groups: -- One in which we quantify the potential changes in the value of our instruments as a consequence of unit variations in market variables. An example will be presented for the case of fixed income assets. -- The estimation of the probability of occurrence of certain losses in a given future time horizon through measures that will be framed within the scope of the value at risk and scenario analysis by virtue, to a large extent, of historical information. The objectives to be achieved are: -- To identify valuation criteria for different types of financial instruments. -- To exemplify the sensitivity metrics for the case of fixed income assets. -- To outline additional metrics that will provide “probabilistic” market risk information. Each financial instrument in a portfolio will have its own more or less simple or complex valuation model. The possible variations in the market prices of the variables (which serve as input for determining the value of each financial instrument) will ultimately determine the level of market risk of each one and of a portfolio as a whole. It is therefore essential to identify these models, since the determination of the potential risk will be given by the difference between the value of the instruments according to these models under market conditions and under adverse conditions for certain confidence levels. These activities are basically classified as follows: Equities. Foreign exchange. Fixed income. Money markets. Commodities. And, in all cases, there were different derivative instruments that could be traded. We will mainly identify futures, options and swaps. 1.1.3.2 Sensitivity One of the first questions we have to answer in risk measurement is: “What would be the impact on a portfolio if there were specific variations in the market variables (exogenous)? In order to answer this question (i.e., to quantify the impact that a portfolio would have if specific variations in the [exogenous] market variables were to occur), elasticity measures must be used. Those that quantify the variation in the value of the instrument, in the face of unit variations in the explanatory variables, will provide an answer to this question. For example, in fixed-income financial assets, risk measurement in terms of elasticity is immediate, given the dependence of the value of the asset on interest rate levels. In the following, we will approach the subject both from a mathematical point of view and from financial aspects that are useful for the understanding of risk management. We will develop this type of market risk analysis for fixed income assets, although its application can be extended to any other 18 PRINCIPLES OF RISK MANAGEMENT type of financial instrument. We will mainly deal with the calculation of sensitivities of the instrument’s value for a given variation of the exogenous variable. The price of a bond is the present value of the flows discounted at the market interest rate for that term. FIGURE 1 Bond valuation. Given the function relating the price and YTM (yield to maturity) of a bond, it is essential to quantify the impact on the value of a fixed-income asset of a unit change in interest rates (the fundamental variable of these instruments). To do this, we will derive the above function in first order and obtain: -- Absolute Sensitivity. -- Modified Duration. -- Duration. These measures refer to aspects related to: Risk: degree of exposure of the value of a bond to variations in the YTM. Time: average residual maturity weighted by the present value of the flows. 19 For example, for a security with a duration of 5 years, we can say of that bond that: Its price will fall by approximately 5% in the face of a percentage increase in the YTM of 1%. This is the definition from the risk side. The existence of three elasticity measures is justified simply by the different possibilities in which the variations of both price and YTM can be expressed. We will discuss the following: Price variations: Its weighted average remaining life is 5 years. This is the definition from the time side. The term “approximately” appears, since the three measures of price-YTM elasticity are an analytical approximation to the real function. In fact, the absolute sensitivity is the first derivative of the function, approximating it by a straight line even though it is a convex function. If we wanted a better approximation, we should perform the second derivative of the bond value with respect to the YTM, thus obtaining the second order elasticity measures, which are: -- Convexity. -- Modified convexity. -- Absolute convexity. Relative: Absolute: P1 - P0 YTM variations: Relative: Absolute: Y1 - Y0 Under the above assumptions, we have that: Duration represents the percentage or relative variation of the bond price in the face of a unit percentage variation of the YTM. Modified duration represents the percentage or relative change in the price of the bond in the face of a unit absolute change in the YTM. Sensitivity represents the absolute change in the bond price to a unit absolute change in the YTM. FIGURE 2 Price-YTM relationship of a bond. Financially, duration is very intuitive if we turn to that definition based on the time aspect of it, so that we have: 20 PRINCIPLES OF RISK MANAGEMENT FIGURE 3 Calculation of duration. Mathematically: Therefore, between the three expressions there is the following relationship: Duration: Convexity Modified duration: Sensitivity: where: P: price F: flow t: time in years N: number of flows D: duration MD: modified duration AS: absolute sensitivity The function that defines the YTM-price relationship in a fixed income asset with intermediate flows is not a straight line. It is a convex curve. This implies that the approximation obtained by the first family of elasticities (duration, modified duration or sensitivity) does not perfectly fit the real function. For further approximation, we will find the second derivative of the function: being the modified convexity: 21 Mathematically and generally: to know the effect of convexity for variations other than 1 %: Convexity has a number of properties that are worth mentioning: if we divide all by P: Convexity is asymmetric: price rises of an asset as a consequence of decreases in profitability by a given magnitude are usually greater than decreases in these in the face of an increase in YTM of a similar amount. This is due to the increasing, though not linear, relationship between duration and convexity. Convexity is positive: higher durations imply higher convexities. In general: Convexity is maximal at low coupons: in extreme cases, zero coupon bonds define higher convexity than securities with similar maturity. However, they are less convex than bonds of the same duration and with intermediate flows. Convexity is “desirable”: for the bondholder. It is always in his favor. This implies that we must now consider, for example, in terms of absolute sensitivity at first and second order: So it is practical to establish a correction coefficient for convexity: To the extent that duration and convexity are positively related, the causal relationships (which were between term, coupon, YTM with duration) are the same for convexity. While we have illustrated the sensitivity metrics in the field of fixed income assets, this risk quantification is applicable to any type of financial instrument. For example, within the field of derivative instruments we will find similar metrics for quantifying the risks of financial options. The main metrics that we have that quantify the elasticity of the value of the options to changes in the different market variables that affect their value are: 22 PRINCIPLES OF RISK MANAGEMENT Delta: measure of the elasticity of the value of an option against changes in the underlying asset in the first order. Gamma: measure of the elasticity of the value of an option in the face of changes in the underlying asset in the second order. Vega: measure of the elasticity of the value of an option in the face of changes in implied volatility. Theta: measure of the elasticity of the value of an option in the face of variations in time. Rho: measure of the elasticity of the value of an option in the face of changes in interest rates. Phi: measure of the elasticity of the value of an option against variations in dividends in the case of equity options. These are just other examples of measures of activity used in the financial field to quantify potential changes in value in the face of unit variations in exogenous variables. The analysis must be complemented with metrics that incorporate probability of occurrence of the shuffled variations because they alternately project probable variations based on different estimation criteria. 1.1.3.3 Value At Risk The Value at Risk (VaR) is the maximum likely loss of a portfolio for a given confidence level over a specified time horizon. The term “maximum probable quantity” implies normal market movements (excludes large financial crises). It does not calculate the maximum loss of the portfolio in absolute terms. Therefore, we are facing a measure that will quantify the potential loss of the portfolio but associated with a statistically defined level of confidence and within a specific time horizon. While the VaR reflects a potential loss, it is expressed as a positive figure in the same base currency (currency units) and summarizes all the risk of the portfo- lio, however complex it may be. Afterwards, it will be convenient to check the results of the portfolio at the end of the time horizon with the Figures of the VaR. In this sense, it should not be surprising that some of the losses that occur are higher than this VaR figure. In fact, statistically it must occur on a certain number of occasions. The important thing is that this number of occasions is within the confidence interval expected from a statistical point of view. Loss estimation can be conceived indistinctly in historical distributions or, for example, in normal distributions. 1.1.3.4 Stress testing VaR is a useful risk control tool in “normal” market environments. Using the VaR movements of the variables under control can be anticipated with a certain level of confidence. Because it is an estimate, a series of scenarios are left out, which are characterized by having a low probability of occurrence and a great impact if they occur. The VaR does not contribute anything about this type of situation, so it is necessary to complement it with a measure that fills this gap (Knop, Ordovás and Vidal, 2004). Stress testing is not a substitute for VaR, but a risk management tool. Its functions are as follows: 1. Identifying potential sources of risk. 2. Evaluating the impact of the risk factor on the portfolio. 3. Managing risk through limits. 4. Applying tools to mitigate risk. 23 FIGURE 4 Value At Risk Basel’s capital agreements require stress testing to be applied in the case of the implementation of internal models (Internal Model Approach) or in the case of advanced credit risk models (Internal Ratings-Based Approach). Stress testing is more intuitive and simple (a priori) for the analyst/risk manager. Work on possible scenarios and their impact on the income statement. The application of VaR methodologies involves the prior study of statistical distributions (movement analysis, estimation and backtesting) and their subsequent effect on the income statement. One of the weaknesses of stress testing is: not being able to predict structural impacts (e.g. shift from a fixed to a floating currency). Its impact goes beyond exchange rate products, as it affects interest rates and equities. Scenario analysis can be interpreted as a coherent measure of risk. Scenarios that remain above the VaR level can be considered extreme risk situations. In addition, under the distribution assumption on which the estimate has been made, they are associated with probabilities of occurrence. The mean of all extreme scenarios (Expected Shortfall) is a coherent measure of risk. Scenario analysis It was initially used as a tool for risk analysis before the development and implementation of VaR methodologies. It consists of selecting a group of 24 PRINCIPLES OF RISK MANAGEMENT scenarios and evaluating their impact on the portfolio (measured as loss in a given time horizon). There are no defined rules for the choice of scenarios: the judgment and experience of the risk analyst intervenes a lot. In general, you have to choose those that do not contain contradictory or impossible assumptions and that preferably include the relationships between variables. We must try to cover, in an ideal way, all the situations covered. A priori, a probability of occurrence is not associated with each scenario that, in many cases, is in the opinion of the analyst: thus, subjectivity appears. The generation of scenarios can be: 1. Event-driven: The impact on profit and loss comes from financial situations that could significantly affect. Examples: 11-Sep-2001 effect, Lehman’s Sept.-Dec. effect, 2008, etc. 2. Portfolio-driven, in which portfolio weaknesses are identified, and ad hoc scenarios are created to compute the impact. Example: portfolio of long positions in senior issues in which interest rates increase and credit spreads increase. According to the effects analyzed, the following can be distinguished: 1. Analysis of univariate sensitivity scenarios. 2. Analysis of multivariate sensitivity scenarios. First, in the analysis of univariate sensitivity scenarios, the impact on the total portfolio of extreme movements in a variable is analyzed. It does not take into account possible correlations between variables. These univariate sensitivity scenarios are: 1. Parallel movements of the curve. 2. Changes in the slope of the curve. 3. Combination of previous ones. 4. Changes in interest rate volatility. 5. Changes in stock market index values. 6. Changes in stock index volatilities. 7. Changes in the levels of exchange rates of quoted currencies. 8. Changes in exchange rate volatility. On the other hand, in the analysis of multivariate sensitivity scenarios, the impact on the total portfolio of extreme movements in several variables is simultaneously studied. It extends to other areas of risk management (balance sheet risk, etc.). These multivariate sensitivity scenarios are: 1. Historical: based on past crises. Not all crises affect all assets equally. Extreme movements occur over different time horizons. You have to find the one that really affects the portfolio under study (trading books, investment portfolios, liquidity buffers, etc.). 2. Prospective: based on possible future crises (e.g. an earthquake in New York, a drastic reduction in fuel production, armed conflicts, etc.). Factor push method: it is based on moving all the underlying risk factors of the portfolio. The movement is performed in a manner proportionate to your average jump: 3, 4, 5 standard deviations. The direction of the movements can be (1) the same for all or (2) the one in which it generates the greatest loss for each variable. Applying the above involves ignoring the correlations between the underlying variables (even in some cases, the greatest loss occurs if the underlying does not move). 45 FIGURE 14 Internal ratings Monographic reports with indicators of the evolution of provisions: distribution by stages, transition matrixes, analysis of the causes of entry into stages, etc., aimed at defining management measures with a direct impact on provisions. Specific indicators of policy compliance: overdue revisions, out-of-policy concessions, etc. 1.4 Market risk Market risk is induced by exposure to price variations in financial variables that may adversely affect the value of our portfolios. Its estimate aims to obtain potential losses under normal market conditions largely based on historical information or, in some cases, scenarios with a stochastic component. Achievement of objectives 1.4.1 Definition It is essential to conceptualize the portfolio monitoring process as an analysis exercise against target values of metrics and KRIs. This drives management decision-making in the face of quantitative triggers in the event of deterioration. As already mentioned, the Value at Risk is the maximum likely loss of a portfolio for a given confidence level over a specified time horizon. The term “maximum probable quantity” implies normal market movements (excludes large financial crises). 46 PRINCIPLES OF RISK MANAGEMENT There are certain concepts that we must specify: correlations and assuming a perfectly positive correlation structure (100%). Confidence levels Historical data The level of probability that the actual losses of the portfolio will exceed the estimated VaR figure for a pre-defined time horizon depends on the confidence level selected. Virtually all market risk measurement methodologies use historical data (prices, interest rates, volatilities, etc.). The final result will depend on the size and historical period (time window) chosen. Time horizon A time horizon must be established to calculate the VaR and be able to contrast the result. It is the period for which the maximum likely loss is estimated, from the present moment to the future. It is usually associated with the period necessary to be able to undo the position or cover the portfolio if it is not possible to close it. This means acting in the market without the variables, which affect the value of the portfolio, being altered and harmfully affecting the value of the portfolio itself as a result of the action. By virtue of this, short time horizons should be assigned to shortterm and long-term trading or speculative portfolios to more structural portfolios whose philosophy and dimension are not consistent with their liquidation in short periods of time. The VaR for a portfolio of assets The correlated net VaR is that VaR figure of a portfolio of financial instruments that reflects their risk considering the correlation structure between them. The un-correlated gross VaR is that figure of VaR obtained as the sum of the individual VaRs of the financial instruments that compose it and that reflects their risk considering a correlation structure of 100% between them. Therefore, it overestimates the real risk. The correlation effect is the difference between the correlated net VaR and the uncorrelated gross VaR. It reflects the difference in risks inherent in considering The use of historical data far removed in time may not reflect the current situation of market risk. Therefore, in certain measurement techniques, the choice of the time window is crucial, since it can, depending on its length, incorporate particularly binding historical facts. The following suggestions may be used for guidance: -- Minimum dimension: 252 days. -- Optimal dimension: around 504 days. -- Dimension in time: constant. The size of historical samples should not be arbitrarily altered over time. The entry and exit of events that are relevant in terms of price variation must occur naturally and according to the size of the sale that has been chosen at a given time. Example Suppose a portfolio of 5,000,000 shares of a company listed at 4.83 EUR (24,155,000 EUR market value) whose daily VaR is 932,890 EUR, considering a confidence level of 95%. Interpretation: It is estimated that the losses should be higher than the VaR figure of 932,890 EUR in only 5% of the next sessions. In the others, losses below that figure, zero results or profits will take place. 47 VaR Properties The VaR is proportional to the market value of the position. An excess of VaR consumed over the limit can be normalized by reducing the position proportionally. VaR varies by market value, by changes in asset volatility, and by substitutions in the vector of historical price data. VaR varies daily, even while keeping the positions stable, due to changes in revaluation prices and in the vector of historical data. The VaR of a portfolio of assets is not the sum of its VaRs because of the correlation that exists between them. VaRs from different business units are also not summable due to their relationship. Earnings At Risk (EaR): It is the maximum likely benefit of a portfolio for a given confidence level over a specified time horizon. It is the antonym of VaR, insofar as it expresses the likely profit rather than the corresponding loss. Under assumptions of normality, in the variations of the value of the portfolio whose average is zero, the EaR will be the same as the VaR. Under any other premise, this will not happen. FIGURE 15 EaR and VaR. The EaR will relate the current market value of a portfolio of financial instruments and the potential gain generated by it for a given confidence level. 48 PRINCIPLES OF RISK MANAGEMENT The EaR/VaR ratio can be of great help in portfolio management to the extent that it will be an indicator of symmetry of results for a given level of confidence, especially when calculating the VaR with simulation techniques. Instruments or portfolios that show the highest possible EaR/VaR ratio will be preferred, as they will potentially generate positive results greater than negative ones in absolute value for the specified confidence level. Example Suppose a portfolio of 5,000,000 EUR shares of a company listed at €4.83 (24,155,000 EUR market value) and the daily EaR is 992,637 EUR, considering a confidence level of 95%. Interpretation: it is estimated that the benefits should be higher than the EaR figure of 992,637 EUR in only 5% of the next sessions. In the others, profits below this figure, zero results or losses will take place. Given the portfolio of shares in this example, whose VaR is €932,890 and whose EaR is 992,637 EUR, the EaR/VaR ratio is 106.40%. This means that, for the same level of confidence (in this case 95%), the expected profits are greater than the losses. Therefore, it is recommended that the portfolio only caters for this indicator. However, in the measurement of risk and management, this indicator is one more factor to take into account within a wide range of decision tools. Profit estimation can be conceived indistinctly in historical distributions or, for example, normal distributions (i.e. Gauss bell-shaped). FIGURE 16 Earnings at risk 49 Obviously, the selection of the distribution with which we have projected the profit and loss account will have implications for the results obtained, both for the calculation of the VaR and for the calculation of the EaR. The methodologies that we will address later differ fundamentally in the type of distribution they assume. It is one of the main methodological hypotheses that will condition the VaR figure. However, while there will be differences in the results obtained, these should not be materially relevant in relative terms. In any case, we will use that hypothesis about the distribution of the income statement that is best able to predict the behavior of these in the future. This is the big goal of market risk metrics in the context of Value at Risk. The Expected Shortfall (ES) If with the VaR we try to predict, under a certain probability, the risk that is assumed, the average excess (Expected Shortfall) predicts, for that probability, the average loss once the VaR has been overcome. This analysis is what is known as Expected Shortfall and should be included in the backtesting analysis. Profit and loss estimation can be conceived indistinctly in historical distributions or, for example, in normal distributions, i.e. in the shape of a Gauss bell. FIGURE 17 Expected Shortfall 50 PRINCIPLES OF RISK MANAGEMENT VaR and ESF are two risk measures Both are useful for measuring capital requirements, for quantifying and for regulating the risk taken by market participants. In a bank, the first utility is to ensure that the risk incurred remains within the forecast. In addition, for the management of that risk to be acceptable over time, any corrective action must be possible. The VaR does not tell us the magnitude of the potential losses that exceed the level of VaR; on the other hand, the Shortfall does. Not in vain, the guidelines outlined from Basel have reinforced this measure as a reference of growing prominence, especially after noting in the financial crisis of 2008 or in 2020, the limitations of the VaR despite all its virtues. In addition, it is necessary to know that the ES (Expected Shortfall) is always higher in absolute value than the VaR. 1.4.2 VaR metholologies The calculation of the VaR can be carried out through various methodologies. The starting assumptions in the various parameters that are used will mark the differences between methodologies. The most relevant differential element between them is the form of the distribution of the expected profit and loss account. There are three different methodological currents for the calculation of VaR: 1. Parametric VaR. 2. VaR for historical simulation. 3. VaR by Monte Carlo simulation. As we will see, the parametric VaR is characterized by assuming a predefined functional form of the profit and loss account to be projected. Quite often, this assumed functional form is a normal distribution, as long as the variable or variables that we are going to project are within the financial field. The two simulation methodologies, that is, the historical and MonteCarlo, will differ in the genesis of the simulation scenarios. 51 In the case of historical simulation, the scenarios will come from the distribution historically defined by changes in the prices of the variables that determine the value of the portfolio. In the case of MonteCarlo simulation, the scenarios will come from a stochastic simulation process. In any case, a predefined functional form will be attributed (in the financial field, it will most often be a normal distribution). In an introductory way, let’s define the three methodologies that will be developed later: 1.4.2.1 Parametric VaR It applies to the current value of the portfolio volatilities and correlations of market variables considering a calculation model and assuming the form of the function of distribution of the rates of variation (results) of the assets. αVaR = -μP&L + σP&Lzα zα = normal distribution of the variable α μP&L = P&Laverage σP&L = P&L standard deviation FIGURE 18 Normal VaR 1.4.2.2 Historical simulation VaR It applies to the current portfolio historical price variations of the variables to generate possible verifiable scenarios against that current position, generating simulated results from which the VaR will be obtained. It does not assume any form of the function of distribution of the rates of variation (results) of the assets. αVaR = -μP&L + σP&L hα μP&L = P&L average σP&L = P&L standard deviation hα = historical distribution of the α 52 PRINCIPLES OF RISK MANAGEMENT FIGURE 20 FIGURE 19 MonteCarlo Simulation VaR Historical Simulation VaR 1.4.2.3 MonteCarlo simulation VaR It applies to the current portfolio random price variations of the variables to generate possible verifiable scenarios against that current position, generating simulated results from which the VaR will be obtained. αVaR =-μP&L+σP&L εα μP&L = P&L average Case study; equity portfolio risk measurement with different methodologies Calculate the Value At Risk of a portfolio of shares of TELEFÓNICA, BBVA, worth 100,000 Euros distributed in equal proportions, using: Historical simulation methodology MonteCarlo simulation methodology Parametric normal methodology σP&L = P&L standard deviation A dataset is provided in Excel containing the historical 1000 prices for both shares εα = random distribution of the variable α Historical Simulation With the calculations made in Excel file: -- Returns: for each of the two stocks held in the portfolio and for each pair of consecutive price observations, the rates of relative variation are calculated. 53 -- Simulated values: from the last available prices of both shares, the simulated values are calculated by applying on them the variation rates obtained in the previous step. -- Value of the portfolio: once the prices of the simulated securities of each of the shares have been obtained, they are multiplied by the number of securities that each of them has in such a way that in this way the value in monetary terms of the portfolio is obtained for each of the simulations generated. -- Profit and loss: simply comparing each of the simulations generated both at the individual level of the two shares and for the total portfolio proceeds to calculate the difference with respect to the securities originally invested on the day on which the risk is being calculated, thus obtaining a profit and loss vector on which the VaR will be extracted. -- Finally, on the vector of total losses and gains obtained in the previous step, the percentile corresponding to the confidence level is calculated: 100%-95%, thus obtaining a Value At Risk of 2,776.48 Euros. Montecarlo Simulation With the calculations made in Excel file: -- Returns: for each of the two stocks held in the portfolio and for each pair of consecutive price observations, the rates of relative variation are calculated. -- Volatility and correlations: volatility for each series is calculated and the correlation matrix as well. -- Cholesky matrix: the Choslesky matrix is obtained from volatilities and correlations in order to generate random correlated scenarios. -- Simulated values (5000 simulations): from the last available prices of both shares, the simulated values are calculated by applying on them the random variation rates obtained in the previous step. 54 PRINCIPLES OF RISK MANAGEMENT -- Value of the portfolio: once the prices of the simulated securities of each of the shares have been obtained, they are multiplied by the number of securities that each of them has in such a way that the value in monetary terms of the portfolio is obtained for each of the simulations generated. -- Profit and loss: simply comparing each of the simulations generated both at the individual level of the two shares and for the total portfolio proceeds to calculate the difference with respect to the securities originally invested on the day on which the risk is being calculated, thus obtaining a profit and loss vector on which the VaR will be extracted. -- Finally, on the vector of total losses and gains obtained in the previous step, the percentile corresponding to the confidence level is calculated: 100%-95%, thus obtaining a Value At Risk of 3,090 Euros. 55 Parametric Normal With the calculations made in the Excel file: -- Returns: for each of the two stocks held in the portfolio and for each pair of consecutive price observations, the rates of relative variation are calculated. -- Volatility (σ) and correlations: volatility for each series is calculated and for the correlation matrix as well. -- Application of these volatilities to the individual nominal values of the positions, considering the time horizon for which the VaR estimate will be made. Thus, individually, the VaR has to be: 56 PRINCIPLES OF RISK MANAGEMENT If we consider together all the j assets of a portfolio, given the matrix of correlations, we have: 1. Maximizing the profit between assets and liabilities. 2. Modulating the exposure of these factors according to the future vision of: We obtain a correlated VaR of 3179 Euros. -- Market variables. -- New business opportunities. -- Customer behavior that could lead to transfers from one instrument to another. In summary, we have: Volatility Multiplier (95%) 1.91% 2.34% 1.64485363 1.64485363 1.593 € 1.963 € 100.00 % 59.30% 59.30% 100.00% VaR Correlation Correlated VaR -3,179 EUR 1 day VaR, 95% confidence level Historical Simulation -2.776 EUR MonteCarlo Simulation -3.042 EUR (5000 simulations) Parametric Normal -3.179 EUR 1.5 Asset liability risk 1.5.1 Definition Asset-Liabilities Management (ALM) is the strategic management of the balance sheet of financial institutions from the perspective of market and liquidity risks. The ALM function is complemented by the management or monitoring of trading positions, funding and equity planning. It affects all financial institutions that have a balance sheet subject to business risks as a result of credit operations, regardless of their trading activities. The fundamental objectives pursued are the measurement, analysis and management of the aforementioned risk factors by seeking: Also complying with banking regulations in terms of control, internal supervision, reporting and good capital management practices is part of ALM. 1.5.2 Types The banking business is based on three fundamental pillars, which are: Clients Margins Risk management. This last aspect interrelates numerous levers of the banking business, since in its field traditional banking activities are interrelated, with the most innovative in both commercial and wholesale banking. Depending on the type of banking business of each entity, the quantitative weight of these pillars may be different in the final income statement. In general, the financial risks that the balance sheet of the financial institution will face will be fundamentally: Credit risk. Interest rate risk. Liquidity risk. Exchange rate risk, if it has activity in various currencies. Operational risk. Legal risk. 60 PRINCIPLES OF RISK MANAGEMENT In addition, we can differentiate between static and dynamic indicators. FIGURE 22 Interest rate risk metrics Metrics have evolved over time and have been complemented to cover all the needs of quantification and capital endowment FIGURE 23 Methodologies