Ecology Notes PDF
Document Details
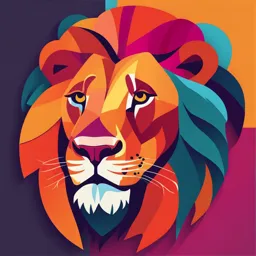
Uploaded by PreEminentPlutonium
University of Mississippi
Tags
Summary
These ecology notes cover a variety of topics, including environmentalism, ecological principles, scientific methods and techniques. Additional topics covered include, biogeography, climate change and how these subjects interact with one another
Full Transcript
\What is Ecology? Derived from greek word Oios, meaning hours and logos meaning reason Ecology- the study of the natural environment and of the relations of organisms to each other and their surroundings ○ Coined by Ersnt Haeckl Interested in external and internal en...
\What is Ecology? Derived from greek word Oios, meaning hours and logos meaning reason Ecology- the study of the natural environment and of the relations of organisms to each other and their surroundings ○ Coined by Ersnt Haeckl Interested in external and internal environment Involves biotic (living) environment such as competition ○ How an animal avoids being attacked Abiotic factors: temperature, how special features have adapted to extreme temp. First real ecologists were Alexander von Humboldt and Charles Darwin Haeckel: Believed in Lamarckian inheritance Proposed theory of ontogeny recapitulates phylogeny Invented the protista kingdom Interested in biogeography- how distributions change over space and time He noticed that as you move away from the poles, vegetation resembles that of a mountain Von Humboldt: Late 1770-1800s Interested in development Studied gradients in Latin America Interested in biogeography- how distributions change over space and time He noticed that as you move away from the poles, vegetation resembles that of a mountain One of the first to recognize similarities between atlantic coast of south america and africa ○ Which were once connected in the supercontinent Gondwana Darwin: Interested in competition and species interactions Interested in population growth of elephants ○ Low population rates would become extremely large if there was no carrying capacity Ecology V Environmentalism: Ecology: ○ A science that observes nature, generates hypothesis, tests them, synthesizes empirically supported hypotheses and theories, and makes inferences about scientific world ○ Is also a political movement aimed at environmental protection Environmentalism: ○ Is a concern Ecologists must be objective however they are influenced by their own prejudices and the world ○ These prejudices are resolved by how well the scientific method is applied Good Science Requirements: Data that are of good quality and are appropriate for the question Appropriate analysis of the data; appropriate experimental design Reasonable interpretation of results Scientific Method: observation -quantitative description Generate and test falsifiable hypotheses Test specific hypothesis Synthesize empirically supported specific hypotheses and parsimonious inferences into theories and scientific laws Not all of these steps need to be found in every experiment Observation and Quantitative description: When you generate a hypothesis, you do a statistical test to see if the hypothesis is reasonable You must gather empirical data to test hypotheses ○ Empirical data- data gathered through observation ○ You can't reject a hypothesis unless you have adequate empirical data You want quantitative data because ○ Counts and amounts can prevent us from being deceived by appearances ○ Quantitative data are more amenable to statistical analysis Statistics allow us to quantify our confidence in the results ○ Error bars quantify confidence Generate a specific; falsifiable hypotheses: Hypothesis: a educated guess that remains largely untested ○ Ex: hypothesis; Japanese honeysuckle reduces plant species richness in forests in North Mississippi Testing the hypothesis requires doing an experiment however, experiments are costly and difficult to do Therefore, Before conducting a experiment, we may see if we have any empirical data to support our hypothesis ○ This exploration uses a statistical technique called regression Regression v Correlation: Both are statistical techniques that quantify the strength of the relationship between continuous variables Mathematically they are same, conceptually they differ Regression: ○ Examines the relationship between a continuous response variable (y axis) and a continuous predictor variable ○ Hypotheses that the predictors variable causes variation in the response variable Correlation: ○ Analysis of 2 continuous variables where cause and effect is not implied ○ Both variables are assumed to be random variables ○ Both variables could be predictor or response variables ○ Just looks at strength of relationship ○ Correlation doesn't prove causation Ex: When looking at where sweetgum is grown and obesity rates in America one may assume that sweetgum causes increased obesity rates, however this is not true because sweetgum is not ingested Regression Analysis: Ecologists are frequently interested in the frequency of one or more species and the values of environmental variables This can be demonstrated using a scatter plot ○ Y axis- response variable ○ X axis- predictor variable Species richness= # of species R2: ranges between 0 and 1 ○ Tells you how much variation in the response variable is explained by the predictor variable ○ If R2=1, the predictor explains 100% of the variation of the response variable P value: ○ Probability of being wrong ○ Lower value, the better R: simple regression coefficient ○ Weighted standardized slope of the best fitting line Do a test to see if the line is different from o ○ To determine if it positive or negative, you must look at whether the relationship is positive or negative ○ Ranges between -1 and 1 Japanese HoneySuckle Example: A study to determine if non-native Japanese honeysuckle potentially had negative effect on the number of native plant species in the forests ○ Before doing an experiment, u want to see if there is a negative correlation between the number of native species and the abundance of japanese honeysuckle ○ Diversity (species richness v. soil compaction r= -.6 ( R2 =.36) Reasons why you don't want to assume cause and effect: 1. Cause confused with effect ○ Variables could be opposite ○ More species rich communities may be more resistant to invasion by honey suckle 2. Confounding variables and lack of independence from other predictors ○ The abundance of japanese honeysuckle is correlated with another predictor variable that is actually causing variation in species richness Multiple Regression: If there is more than one variable that could affect the response variable, you can use a multiple regression to see which has the highest correlation with the response variable ○ Ex: there might be more than one variable that could potentially affect species richness. I examined relationships with Japanese honeysuckle and several environmental variables and wanted to see which one(s) showed the highest correlations with native species richness. These variables might be the focus of additional experimentation. Multiple regression ≠ multiple simple regression ○ If you do multiple simple regression, R2 can add up to more than one; which is impossible Multiple regression shows the independent contribution of each variable to native species richness ○ Multiple simple regressions does NOT do this Japanese HoneySuckle Example Cont: We want to know if plant species richness is affected more by competition from Japanese honeysuckle or by soil compaction or elevation. Multiple Simple Regressions: ○ First calculate simple regressions: Species richness v. soil compaction R = -.73 r2 = 0.53 Species richness v. japanese honeysuckle abundance R = -.60 r2 = 0.36 Species richness v. elevation R = -.39 r2 = 0.15 ○ Add up the three r2; ○ They add up to 1.04, but r2 is the proportion of variation in the response variable (in this case species richness) explained by the predictors Therefore it cannot be more than 1 (100%) ○ These are simple regression coefficients and simple coefficients of determination (r2) They don't take into account (subtract) the common variation explained by the three predictors ○ By not accounting for and removing common variation among the predictors, we overestimate the overall variation explained by the 3 variables and we provide a misleading ranking of the importance of the 3 predictors In the figures below, doing three simple regressions would give A the highest rank and C the lowest In multiple regression would give A the highest rank and B the lowest rank Multiple Regression: combines and compares the independent effects of multiple continuous predictor variables (competition and drainage) on a single response variable (richnesses) Partial Regression: When u do a multiple regression, you are running several partial regressions Partial regression: a regression of residual variation in a response variable against the residual variation in a predictor variable, given one or more covariables ○ Subtract the overlap ○ You want the partial r2 Sum won't be greater than one Covariable: a continuous variable whose variation we want to account for Residual variation: variation in the variable NOT explained by one or more other variables of interest Ex: Partial r = -0.189 Partial r2 = 0.036 Simple regression: simple r = -0.6 simple r2 = 0.36 Note the weaker relationship for the partial regression than for the simple regression (lower r and r2) ○ This indicates a lot of the variation in species richness explained by honeysuckle variation is also explained by soil compaction Getting residuals: 1. To Obtain the partial regression of the response variable (richness) against the predictor variable (japanese honeysuckle), do a simple regression of japanese honeysuckle against soil compaction ○ Locating the line of best fit, explain the total variation, residual variation and model variation ○ Rotate the scatter plot so the line of best fit is horizontal Make r2 = 0 ○ Move the horizontal line and points downward until the horizontal line sits on the X axis ○ The variation in richness that is left and depicted in the model represents the variation in japhoney that is not explained by soil compaction Removes compactions effect on honeysuckle This is residual honeysuckle abundance 2. Then do a second simple regression, except do richness v. compaction and obtain residuals ○ This will give u variation in richness that is not explained by soil compaction ○ This is residual richness 3. Then do a 3red regression with residual richness against residual japhoney abundance ○ Obtain r and r2 ○ r2 gives the proportion of richness explained by japhoney alone This is a partial regression 4. Repeat these procedures to do the partial regression of richness against soil compaction, given Japanese honeysuckle cove ○ Partial regression of species richness vs. Japanese honeysuckle cover: partial r = -0.18 (partial r2 = 0.035) ○ Partial regression of species richness vs. soil compaction: partial r = -0.61 (partial r2 = 0.36) ○ These are partial regression coefficients that subtract the portion of variation in diversity that is attributed to correlation with other predictor variables Doing a partial regression for each of the predictors completes the multiple regression Once you do this u can say the predictors are independent variables Limitations of the correlative/regression approach: correlation doesn't prove causation ○ There could be other variables that were unaccounted for Variables that explain the most variation in species composition may be correlated with other variables that were not measured but affect the distribution of species ○ Ex: is soil compaction reducing plant species richness or is it correlated with past agriculture disturbance, which eliminated the native plant species ○ Richness was negatively correlated with soil compaction, implying that characteristics of the soil eliminated species ○ However compacted soils historically had deep silt loam soils that were conducive to plant growth ○ Many forests were cleared in the mid 1800’s to plant cotton and the soil was subsequently eroded ○ Less compacted soils are less fertile and not conducive to grow agriculture ○ Soil compaction may be an indicator of agriculture in the past, rather than a cause of the species lost Distinguishing between cause and effect: Even if all confounding variables could be accounted for and were found to be unimportant, a significant, negative partial regression it still doesn't prove negative effect because the reverse could be true ○ EX: there is a significant, negative partial regression between species richness and honey suckle cover, but it does prove cause and effect because you do not know which is causing the other Multiplicative effects: Multiple regression assumes additive effects, not multiplicative effects Our multiple regression approach assumes that the effects of soil compaction and honey suckle cover were additive but what if the effects were multiplicative ○ What is the japhoney is a backseat driver What if soil compaction increased the effect of japhoney on native species additive effects: the effects add together when combined ○ Ex: low honeysuckle, high compaction (20) + high honeysuckle, low compaction (5) = high honeysuckle, high compaction (25) Multiplicative effects: the combined effects are increased compared to their individual effects ○ One increases or decreases the other ○ Maybe high soil compaction causes honeysuckle to have greater effects ○ The effect of one depends on the other ○ In this case, one is increasing the effect of the other Ex: low honeysuckle, high compaction (20) + high honeysuckle, low compaction (5) = high honeysuckle, high compaction (50) Manipulative Experiments: There are two major challenges when ecologists perform controlled experiments in the field ○ Designing an appropriate control There is normally not an appropriate control in ecological experiments It is hard to get an appropriate control Treat the controlled and experimental group the same expect the treatment you are testing Treatment may manipulate other things that you don't want to manipulate ○ True and adequate replication of the treatment/controls Getting adequate replication can be hard You need to get adequate replication to do a statistical analysis Example: the problem of control and replication in field experiments Fire stimulated flowering in pine savannas What is the effect of fire on flowering in a given year Naive approach: ○ Find 2 sites ○ One will be burned during peak lightning fire season and the other will not be and has not been burned recently ○ Take multiple samples within each site and then statistically analyze the difference between the no fire site and the burned site In this case, take 40 samples within each site to improve statistical power Statistical power- the ability to detect a statistically significant effect- if one exists ○ Increase # of samples = increased statistical power In an experiment, you want a lot of replication of the treatment If the bars don't overlap, there is significant difference ○ The figure shows that there is a statistically significant difference in % flowering between the “no fire” site and the “lightning fire site” ○ This makes us conclude that fire stimulates flowering However, there are 2 things wrong with this conclusion ○ Pseudoreplication Problem: you did one fire and took samples from it, in a good experiment there is random and individual treatment of samples ○ Inappropriate control Pseudoreplication: Treating multiple correlated observations (samples within replicates) as if they were independent observations Treating a site and taking samples from it Occurs when sampling units within an experimental unit are considered experimental units Experimental unit: ○ An observation that receives a random and independent assignment of the treatment or is randomly assigned to a control ○ Each experimental unit is a true replicate ○ You need multiple experimental units to have true replication Sampling unit ○ An observation sample randomly from within an experimental unit Issues: The lower figure shows that the effect of fire on % flowering is not statistically significant because the error bars overlap slightly Problem: ○ There are too few true replicates (n= 2) and therefore the statistical power is low ○ Dilemma: On one hand, pseudoreplication increases statistical power and therefore the ability to detect differences in sites On the other hand, the difference between the sites might not be due to treatment (fire) because it was not replicated Example of true replicates: If variation btwn replicates that were treated the same way is low relative to sampling variation within the replicates, then you can pool the samples across both replicates of each groups and take advantage of the additional statistical power provided by the pseudoreplicates (samples) As seen in the figure, differences between sites that were treated the same were not significant, but the means of the two pairs of sites (red for burned, blue for not burned) significantly differed from each other There is a significant effect of fire on percent flowering Two approaches to avoiding the problems of pseudoreplication and low statistical power of truly replicated fire experiments: Design the experiment so that it includes both true replicates (multiple experiment units/sites) Achieve replication through multiple pseudo replicated experiments and meta-analysis ○ Meta-analysis: what is the overall consensus (agreement) of multiple experiments Limitations of Manipulative Experiments in Ecology: Ecologists work in large complex systems that may not be able to be manipulated Alternative Approaches to manipulative experiments: Modeling ○ Involves using one or mathematical equations to describe certain physical situations ○ These physical situations often cannot be easily observed directly in a short period of time ○ Therefore, models are used to make predictions of situations that cant be easily observed directly ○ Regression is a type of mathematical model The equation given for the line of best fit is a mathematical model ○ Multiple regression contains predictor variables and associate coefficients (ex: slopes) Modelers refer to these coefficients (slope) as parameters The slope coefficient in the line of best fit equation is an example of a parameter ○ Models can also be based on non-linear equations (ex: exponential growth) Interpolation: Prediction within a range of directly observed measurements of the predictors ○ Known values are used to predict an unknown value Validation of the results of multiple regressions is then achieved through experiments ○ When we discussed regression in relation to ranking hypotheses for the purpose of elimination bad hypotheses for testing, we used this model for interpolation Extrapolation: A prediction beyond the range of directly observed measurements of the predictor ○ A prediction that is beyond the range of known values Modeling can also be used for extrapolation In this case, experiments are not used to validate the predictions of the model Validation must be done in a different way Model Construction involves: Parameterization Application Sensitivity analysis Validation Parameterization: Parameterization involves finding values for the coefficients (aka parameters, the P’s) in the model Each predictor variable (Xi) has an associated coefficient (parameters) Pi ○ Y is the response variable of interest This may involve doing regression analysis or experiments under easily observed conditions ○ You can figure out the relationship between CO2 levels and temperature in a small scale environment or by looking at past relationships ○ Although it is impossible to experimentally manipulate atmospheric is CO2 at the scale of the entire planet, it might be possible to manipulate CO2 concentrations at much smaller scales (ex: in the laboratory) and investigate the effects on plant growth and temperature ○ One might use a correlative approach, using the relationship between atmospheric CO2 and global temperatures in the past ○ Both qualifications could be used to generate a coefficient (parameter) relating to temperature (Y) and CO2 concentration (Xco2) to be used in the model for making predictions for future conditions Application: Manipulate the X’s and solve for Y Involves applying the parameterized model to unobserved, hypothesized conditions (testing hypotheses) ○ Plugging in different values of X for a given predictor, while holding values of X for other predictors constant and solving for Y Manipulating Xs for some predictors and keeping the rest the same Solving the model Manipulate the Xs (or manipulate 1 and keep the rest the same) and solve for Y You can manipulate the predictor of interest by plugging in different values and keeping everything else the same Two categories of Models: Analytical models: ○ Simple models ○ Few parameters ○ Many assumptions ○ A single solution/prediction ○ These are usually wrong but they help with understanding the topic Simulation Models: ○ Complex models ○ Many parameters ○ Few assumptions ○ Range of solutions/predictions ○ Must do sensitivity analysis Sensitivity Analysis: Make small changes to each parameter (P) and predictor (X) to see if there is large or unexpected changes in Y Involves making subtle changes to a single parameter and/or predictor and then seeing how predictions change (testing hypothesis and model stability) This analysis is particularly important for checking the stability of complex simulation models ○ Bc of their complexity, the solutions may not be intuitively obvious ○ If subtle changes to the parameter are made and result in subtle changes in the solution result, then the model is assumed to be stable If subtle changes in X cause subtle changes in Y= model is stable ○ If a dramatic change occurs, the model may not be stable Validation: Does the value of Y predicted by the model (Ŷ) reasonably match what is actually observed Yobs Ŷ= predicted value of response value Yobs =observed value Validations involves verifying that model predictions are supported by empirical observational data ○ See if the models prediction matches what is observed However, it can be hard to observe certain things ○ You can use backcasting- seeing if the model accurately predicts the past ○ Ex: many climate simulation models are forecasting models Potential disadvantages/limitations of modeling compared to manipulative experiments: 1. Have you incorporated all the relevant parameters? 2. Have you accounted for all potential interactions and feedback among variables? 3. Are there threshold (non-linear) responses? In these cases, extrapolation may result in inaccurate predictions 4. Synthesis Negative Feedback: Increase in a response variable (Y) affects the predictor variable (X) and leads into subsequent decrease in the response variable ( Y) Ex: increased atmospheric CO2 result in increased temperature ○ This increase in temperature could result in increased plant growth, which would result in increased uptake and assimilation of CO2 Thereby reducing atmospheric CO2 and temperature Positive Feedback: Increase in a response variable (Y) affects the predictor variable (X) in such a way as to lead an even greater increase in the response (Y) variable Ex: increased CO2 increases temperature, which leads to increased rates of decomposition and soil respiration ○ This liberates more CO2 into the atmosphere- which increases temperature further Positive feedback can make it hard to distinguish between cause and effect Are there threshold/nonlinear responses? In these cases, extrapolation may result in inaccurate predictions Dramatic increase or decrease Synthesis: Generating a general theory Theory- an educated guess that has been tested and has broad general support Law- an educated guess that has been tested for and has not been disproved ○ Not facts, must be observed Two general approaches to generating a theory: ○ Empiricism and rationalism Empiricism: Ecological empiricists focus on experience and repeated experimental tests of specific hypotheses to develop theory They then use techniques such as meta-analysis to see if there is a possible consensus Repeated support elevates the hypothesis to a theory Rationalism: Uses reason and things we already know to limit the number of observations and tests necessary to develop a theory ○ Ex: prior knowledge of how ecosystems work is incorporated into theories Much of the rationalist approach is focused on the development of mathematical theory such as niche theory and energy theory The assumptions of the theory are tested using model systems ○ Aka systems that are simple enough to test assumptions practically Plant strategy: Stress tolerators: ○ Says that plants adapt to nutrient scarcity by storing resources and using them conservatively They may also defend them This results in slow growth and delayed production Ruderals: ○ Plants adapt to frequent disturbances by completing their entire life cycle rapidly Competitors: ○ Dominate areas that are neither nutrient poor nor disturbed Fast growing, big, long lived Delayed reproduction Neither stress-tolerators nor ruderals can persist in undisturbed, nutrient-rich soils ○ They are replaced by competitors Important prediction of plant strategy theory: no viable strategy for dealing with both nutrient scarcity and frequent disturbance Important assumption of plant strategy theory: plants obtain nutrients from the soil in which they are rooted Empiricist approach: ○ Would involve sampling many terrestrial ecosystems, quantifying disturbance and soil nutrients, seeing which plant strategies dominate each of those ecosystems Then sees if the theory correctly predicts which strategy dominates each system Rationalist approach: ○ Looks for exceptions to what the theory predicts and examines weather an important assumption of the theory has been violated Example: Consider the following two ecosystems ○ Logging roads, trails, and stream banks in nutrient poor bods ( aka disturbance combined with nutrient scarcity Theory predicts; no viable strategy ○ Undisturbed bods with nutrient poor soils Theory predicts; stress-tolerators dominance While it is true that undisturbed, poor-nutrient bods are dominated by stress-tolerators, logging roads, ditches, and disturbances by crayfish in these areas are not completely without species and in fact are dominated by short-lived ruderals with a unique adaptation- carnivory ○ The area that the theory predicts has no viable species actually have ruderals that have adapted carnivory Do these results support or refute the predictions of plant strategy theory: ○ They support the theory according to the rationalist approach Botanical carnivory violates the assumption that the plants obtain nutrients from the soil Also violates the assumption that the availability of nutrients to plants are scare in habitats with nutrient-poor soils ○ Therefore, Drosea Capillaris is a ruderal capable of growing rapidly in nutrient poor soils bc it has access to nutrients other than those found in the soil (aka pray) Therefore, they experience less stress than non-carnivorous plants Note that carnivorous plants don't actually occur in the untenable triangle, which supports plant strategy theory ○ This is bc carnivorous plants are not experiencing as much nutrient stress as non-carnivorous plants ○ Carnivorous plants can supplement the supply of nutrients in the soil with nutrients from prey ○ This allows them to exhibit ruderal traits (rapid growth, early reproduction) and therefore take advantage of disturbances in nutrient poor soils Biogeography: A study of distributions of organisms and the factors that contribute to them Temperature: Latitude effect: ○ Temperature decreases with increasing latitude ○ Temperature is higher at the equator and lower at the poles Results from the position of the sun relative to the surface of the earth Sun hits the atmosphere perpendicular and the energy is spread out at the poles and deflected into space When the sun is lower in the sky, a beam of sunlight spreads over a greater area and travels a longer path through the atmosphere- much of this energy is absorbed and radiated back into space Carbon cycle and the Greenhouse effect: ○ CO2 is an important greenhouse gas ○ Co2 results in natural variation in temp. ○ greenhouse effect: natural effect that humans have modified ○ Allows incoming shortwave radiation to penetrate the atmosphere but absorbs outgoing longwave radiation and redirects some of it toward the Earth - traps some of the outgoing radiation (heat) ○ CO2 and other natural greenhouse gases (ex: methane and nitrous oxide) are necessary to sustain life on earth ○ They keep earth's temperature about 33 degrees c (59 F) higher than it would be without them Greenhouse effect: ○ The sun's energy passes through atmosphere and is absorbed into the earth's surface ○ Some is reflected from the surface and then some of it is trapped by greenhouse gases like co2 ○ CO2 is the most abundant greenhouse gas and methane is the most potent greenhouse gas ○ Called greenhouse effect because the sun in the greenhouse is radiated by things and then trapped in the greenhouse Seafloor spreading/Greenhouse effect model hypothesis: A hypothesis of natural variation in greenhouse warming Hypothesis states that atmospheric CO2 may fluctuate naturally in response to the rate of sea-floor spreading associated with the plate tectonics Continents move along a conveyor belt (ocean floor) and there is a subduction that falls down into the ocean floor creating rifts Volcanic activity in rifts on the ocean floor heat up carbon deposits , releasing CO2 to the atmosphere ○ There is a lot of carbon on the ocean floor that is burned by volcanoes, releasing co2 into the water and eventually the atmosphere ○ CO2 dissolves in the clouds containing carbonic acid ○ This precipitates and dissolves carbons that is deposited into the ocean floor At times, volcanic activity increases (incr. The rate of movement of continental plates) The increased combustion of carbon deposits increases the ratio of atmospheric CO2 to carbon deposition ○ this increases the natural greenhouse effect and global warming You can look at fossils to look at the rate of seafloor spreading ○ Greater sea floor spreading= higher temperatures = warmer species dominate ○ This is not always accurate- the last ice age did not correspond with slower sea floor spreading The last ice age could have occurred due to the creation of the himalayas which increase precipitation Temperature effects cont: Topographic effects on temperature: ○ Temperatures are lower on mountaintops and higher in valleys ○ Heat from solar radiation is absorbed by the earth and air near the earth's surface ○ Sensible heat dissipates with increasing elevation as the density of air molecules that store the heat decreases This is associated with a decrease in temperature with increasing elevation Ex: himalayas are cold even though they are located in a warmer area due to elevation ○ Changes in vegetation result from temperature changes ○ As elevation increases, air density decreases, so sensible heat decreases and temperature decreases Earth's orbit, solar activity, ocean currents, volcanos ○ Orbit changes periodically ( on a time scale of tens of thousands of years) ○ Sunspots increase solar irradiance and thus global temperature ○ Sun spot activity goes in cycles More sun spots= more orange area (hot area) Black area is cooler More sunspots= increase in sun's energy ○ Terrestrial volcanoes put lots of particles and aerosols in the atmosphere after erupting which prevents the sun's energy from entering the atmosphere- decreasing temperature Volcanoes are often associated with reduced temperature by increasing the amount of sun-screening aerosol and particles in the atmosphere Precipitation: Affected by many of the same factors as temperature Latitude: Temperature varies with latitude as a result of: ○ Convection: the tendency of warm air to rise Heated air is lighter than cold air, as a result, it rises which cold air sinks ○ Adiabatic lapse: failure of heat transfer from low to high altitudes The tendency of warm air to cool as it rises ○ Condensation: precipitation that occurs as warm air cools Warm air holds more water vapor than cold air ○ When warm air cools, precipitation occurs There is a lot of precipitation at the equator Cool dry air has no moisture ○ The earth’s gravitational pull on the air creates a convection cell Ex: Hadley Cell ○ Earth's gravitational pull keeps warm air from escaping It tends to descend at 30° latitude North and South ○ Equator: low pressure system because air rises - rainforests ○ 30° latitude: high pressure and no rain - deserts Hadley Cell: ○ The sun heating up increases pressure and increases precipitation Polar Cells: ○ Cold dry air descends at the poles (high pressure) ascends at 60 Ferrell Cells: ○ Located between the hadley cells and polar cells in the temperate zone Their movement if driven by the hadley and polar cells Coriolis Effect: ○ The apparent deflection of moving air and water masses relative to a reference point that is moving due to the earth's rotation ○ Earth has a gravitational pull to keep air from leaving because the earth is rotating and it pulls air with it ○ The air gets deflected by gravitation pull and descends which is why the hadley cells don't go to the polls Trade Winds: ○ Pick up the moisture as they move towards the equator ○ Form because air tends to move from an area of high pressure to an area low atmospheric pressure ○ Trade winds originate at 30° latitude, pick up moisture, heat up and rise at the equator Then they cool down and water vapor condenses as rain at the equator Dry, cooler air descends at 30° As a result, deserts form mostly at 30° while tropical rainforests are at lower latitudes (equator) Influences of precipitation vary within and between years: Eastern north america climate: ○ Influenced more by two major air masses- Maritime Tropical and the Continental polar Jet streams moves polar fronts eastward El Nino Southern Oscillation: ○ Pressure gradient that generates as the trade winds weaken ○ Water in the pacific sloshes eastward and piles up against peru northward and southward ○ This brings rain to Baja California and northern chilean desert (30° latitude N and S ) ○ Haldey cell pushes down dry air and trade winds ○ Decreased trade winds and you get warm, moist air completely different than the typical dry weather ○ El nina: mild winter and spring; dry conditions When el nino is followed by el nina, you get wildfires Topography: ○ Rain shadow results from adiabatic lapse and condensation ○ Rain shadow- mountain ranges intercept rain causing deserts to form on the land side of mountains Winds rise over the mountains and cool, loosing their water rapidly so the other side of the mountain receives much less rain and is dry ○ The rain reaches mountain ranges and it rises (convection) because it is warm Then it condenses causing precipitation By the time the air reaches the other side of the mountain there's no moisture and is very dry Patterns of terrestrial vegetation: Biomes: A terrestrial, climatically controlled set of ecosystems characterized by a distinctive vegetation type ○ Vegetation is defined by growth form Whittaker's classification of biomes is based on temperature and precipitation Whittaker's Classification of Biomes: Biome Temperature Precipitation Dominant Comments Growth Forms Tropical Rain Warm; High rainfall year-round Broad-leaf Found primarily in C. and Forest Aseasonal; No evergreen trees, S. America, W. Africa, and freezing epiphytes, lianas S.E. Asia around equator Tropical Warm; Moderately high rainfall; Mix of broad-leaf More common a little Seasonal Forest Aseasonal; No short dry season deciduous and farther away from the freezing evergreen trees equator than tropical rain forests Tropical Warm; Moderately low rainfall; Mix of trees Fire and grazing from Savannas Aseasonal; No long dry season (broad-leaf or megafauna help maintain freezing needle-leaf) and the community grasses, forbs, and thorn-scrub Desert Hot/Temperate; Very low rainfall; wet Succulent Most common around 30d Occasional seasons do not (water-storing) N and S of the equator and Freezing necessarily occur every trees and shrubs, in rain shadows year grasses, forbs, thorn-scrub Temperate Rain Cool/Temperate; Very high rainfall; Needleleaf Mostly around 40 to 60 N Forest Mild summers rivaling but not as high evergreen (mostly and S latitudes; weather and as tropical rainforest; conifers) and some dominated by temperate cool/moderate more precipitation in broadleaf maritime air masses; winters form of drizzle and deciduous trees; Pacific Northwest; snow and fewer epiphytes (primarily Southern Chile; New thunderstorms mosses and Zealand compared to tropical lichens) rain forests Temperate Temperate; Hot Seasonal – wet winters Broad-leaf Mostly between 30 and 50 Deciduous summers; cold and springs; dry deciduous trees; degrees latitude in Forest winters summers and falls spring-ephemeral northern hemisphere – E. forbs; few or no North America, C. Europe, warm-season and E. Asia grasses Temperate Temperate Dry-seasonal; long, dry Warm-season Frequent fires and grazers Grasslands and summers; drier than grasses, forbs, are important; fire/grazing Savannas TDF. shrubs, and suppression alters the needleleaf community; examples evergreen trees include Ponderosa Pine (frequently pines) savannas of the intermountain west and tallgrass prairies of the Great Plains and of the northern Midwest Mediterranean Warm-Temperate Dry-seasonal; long, dry Scrub (low shrubs; Common in southern ; Freezing rare summers; short, wet aromatic herbs) California and southern winters Europe and parts of Australia; Periodic, severe (but not frequent) fires important; Fires occurring at a higher frequency in S. California than historically due to frequent ignitions by humans. Boreal and Cool-Temperate; Wet-seasonal; much Needleleaf Common in Canada, Subalpine Cold Winters; precipitation in the form evergreen trees; Alaska, and moderately Forests; Taiga Mild Summers of snow; occasional dry low diversity; high elevation in the summers spruce, fir, and Rockies; pines with serotinous cones Low frequency of high-intensity, stand-replacing fires associated with dry summers. Serotinous cones [JSB1] allow regeneration of pines following fire Shortgrass Cool-Temperate Dry Short, cool-season Common in the western prairie and and warm-season portion of the Great Plains steppe grasses (E. Colorado) and Siberia; grazers are important Tundra Cold; permafrost Low precipitation but Cool-season Common in northern present soil is generally moist grasses and Alaska, Canada, during the summer due sedges, forbs, Scandanavia, and Siberia; to low including Sensitive to global evapotranspiration rates herbaceous warming willows, lichens (primary food of Caribou), mosses Tropical Rainforests: Wrm, wet Plant growth and precipitation year round Never freezing temps Lots of moisture= plants growing on plants Tropical deciduous forest: Warm; seasonal precipitation Theory that deciduous trees adapted from dry seasons Tropical Seasonal Forests: Long wet season /short dry season Tropical Savanna: Warm,dry Long dry season; short wet season Large herbivores Ecologists have debated if savannas occur due to long dry seasons because places with alot of rainfall can still have savanas ○ Ex: brazilian Cerrado ○ This is due to fires Desert: Extremely dry Cactus spines- leaves adapted for protection Temperate Rainforests: Cool, wet Evergreens High rainfall Temperate deciduous: Temperate; seasonally wet Temperate grasslands: Hot and cold dry -season Frequent low intensity fires Mediterranean: Plants are fire adapted to infrequent but sever fires Plants make seeds, drop them into the soil, and germinate after fire Boreal Forest: Cold, wet ○ Occasionally dry Spruce fir, jackpine ○ Cones dont open and release their seeds except after extreme heat (fires) ○ Major fires occur once every 50 yrs or more but they are extremely intense Controversy: has fire suppression increased severity of wildfires in boreal forest biome? ○ Not clear that it has ○ There is little evidence to show that fire suppression has ever been effective at reducing large fires ○ Large fires are rare in this biome but they may be increasing due to climate change ○ If they become too frequent, the plants wont have enough time to grow and make seeds to reproduce Shortgrass steppe: Cold, dry Often off a mountain TundraL Higher elevation than boreal forest Wet meadow during growing season Permafrost- layer of ice below soul so plants must have shallow roots ○ As a result, no trees Variation in local vegetation types: Soils influence drainage, nutrient availability and toxicity: Soil Type: Soil type varies locally and determines important vegetation Soil impacts moisture and nutrients Low pH often lowers the availability of phosphorus in the soil ○ Low pH of soil makes certain limiting nutrients like phosphorus in a form that cant be taken up by plants ○ Plants need adaptations to this Silt loam soil tends to be the most fertile because they have enough silt and clay to be fertile but not so much clay that they are poorly drained Loam soils have roughly equal parts sand, silt, clay, and organic matter Sandy soil: ○ largest particles ○ typically infertile and excessively drained ○ Nutrient poor and dry Silt soils: ○ medium sized soil are fertile Clay soil: ○ smallest particles ○ fertile but often inadequately drained and can get so hard when they dry out that they prevent root penetration ○ Trees will not grow here because soil is too hard ○ Clay Hardpan: poorly drained soils impede on establishment of trees Nitrogen goes where the water goes w