DWH&DM Document PDF
Document Details
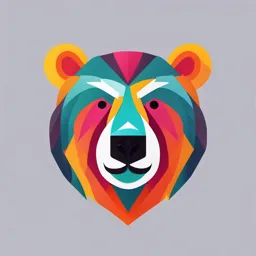
Uploaded by SolicitousPeridot
Tags
Summary
This document is an exam guide or study material, outlining key concepts of data warehousing and data mining. It describes important aspects of the data warehouse environment and introduces concepts like metadata, data transformation, and data reduction.
Full Transcript
**DWH&DM** 1. \_\_\_\_\_\_\_\_\_\_ is a subject-oriented, integrated, time-variant, nonvolatile collection of data in support of management decisions. A. Data Mining. B. [Data Warehousing.] C. Web Mining. D. Text Mining. 2. The data Warehouse is\_\_\_\_\_\_\_\_\_\_. A. [Read on...
**DWH&DM** 1. \_\_\_\_\_\_\_\_\_\_ is a subject-oriented, integrated, time-variant, nonvolatile collection of data in support of management decisions. A. Data Mining. B. [Data Warehousing.] C. Web Mining. D. Text Mining. 2. The data Warehouse is\_\_\_\_\_\_\_\_\_\_. A. [Read only.] B. Write only. C. Read write only. D. None. 3. Expansion for DSS in DW is\_\_\_\_\_\_\_\_\_\_. A. [Decision Support system.] B. Decision Single System. C. Data Storable System. D. Data Support System. 4. The important aspect of the data warehouse environment is that data found within the data warehouse is\_\_\_\_\_\_\_\_\_\_\_. A. Subject-oriented. B. Time-variant. C. Integrated. D. [All of the above.] 5. The time horizon in Data warehouse is usually \_\_\_\_\_\_\_\_\_\_. A. 1-2 years. B. 3-4years. C. 5-6 years. D. [5-10 years.] 6. The data is stored, retrieved & updated in \_\_\_\_\_\_\_\_\_\_\_\_. A. OLAP. B. [OLTP.] C. SMTP. D. FTP. 7. \_\_\_\_\_\_\_\_\_\_describes the data contained in the data warehouse. A. Relational data. B. Operational data. C. [Metadata.] D. Informational data. 8. \_\_\_\_\_\_\_\_\_\_ is the heart of the warehouse. A. Data mining database servers. B. [Data warehouse database servers.] C. Data mart database servers. D. Relational data base servers. 9. \_\_\_\_\_\_\_\_\_\_\_\_\_\_\_\_defines the structure of the data held in operational databases and used by operational applications. A. User-level metadata. B. Data warehouse metadata. C. [Operational metadata.] D. Data mining metadata. 10. \_\_\_\_\_\_\_\_\_\_\_\_\_\_\_\_ is held in the catalog of the warehouse database system. A. Application level metadata. B. [Algorithmic level metadata.] C. Departmental level metadata. D. Core warehouse metadata. 11. \_\_\_\_\_\_\_\_\_maps the core warehouse metadata to business concepts, familiar and useful to end users. A. [Application level metadata.] B. User level metadata. C. Enduser level metadata. D. Core level metadata. 12. \_\_\_\_\_\_\_\_\_\_\_\_\_consists of information in the enterprise that is not in classical form. A. [Mushy metadata.] B. Differential metadata. C. Data warehouse. D. Data mining. 13. \_\_\_\_\_\_\_\_\_\_\_\_\_\_databases are owned by particular departments or business groups. A. Informational. B. [Operational.] C. Both informational and operational. D. Flat. 14. The star schema is composed of \_\_\_\_\_\_\_\_\_\_ fact table. A. [one.] B. two. C. three. D. four. 15. The time horizon in operational environment is \_\_\_\_\_\_\_\_\_\_\_. A. 30-60 days. B. [60-90 days.] C. 90-120 days. D. 120-150 days. 16. The key used in operational environment may not have an element of\_\_\_\_\_\_\_\_\_\_. A. [time.] B. cost. C. frequency. D. quality. 17. Data can be updated in \_\_\_\_\_environment. A. data warehouse. B. data mining. C. [operational.] D. informational. 18. Record cannot be updated in \_\_\_\_\_\_\_\_\_\_\_\_\_. A. OLTP B. files C. RDBMS D. [data warehouse] 19. The source of all data warehouse data is the\_\_\_\_\_\_\_\_\_\_\_\_. A. [operational environment.] B. informal environment. C. formal environment. D. technology environment. 20. Data warehouse contains\_\_\_\_\_\_\_\_\_\_\_\_\_data that is never found in the operational environment. A. normalized. B. informational. C. [summary.] D. denormalized. 21. The modern CASE tools belong to \_\_\_\_\_\_\_ category. A. [Analysis.] B. Development C. Coding D. Delivery 22. Bill Inmon has estimated\_\_\_\_\_\_\_\_\_\_\_of the time required to build a data warehouse, is consumed in the conversion process. A. 10 percent. B. 20 percent. C. 40 percent D. [80 percent.] 23. Detail data in single fact table is otherwise known as\_\_\_\_\_\_\_\_\_\_. A. Monoatomic data. B. Diatomic data. C. [Atomic data.] D. Multiatomic data. 24. \_\_\_\_\_\_\_test is used in an online transactional processing environment. A. MEGA. B. MICRO. C. MACRO. D. [ACID.] 25. \_\_\_\_\_\_\_\_\_\_\_ is a good alternative to the star schema. A. Star schema. B. Snowflake schema. C. [Fact constellation.] D. Star-snowflake schema. 26. The biggest drawback of the level indicator in the classic star-schema is that it limits\_\_\_\_\_\_\_\_\_. A. Quantify. B. Qualify. C. [Flexibility.] D. Ability. 27. A data warehouse is \_\_\_\_\_\_\_\_\_\_\_\_\_. A. Updated by end users. B. Contains numerous naming conventions and formats C. [Organized around important subject areas.] D. Contains only current data. 28. An operational system is \_\_\_\_\_\_\_\_\_\_\_\_\_. A. used to run the business in real time and is based on historical data. B. [used to run the business in real time and is based on current data.] C. used to support decision making and is based on current data. D. used to support decision making and is based on historical data. 29. The generic two-level data warehouse architecture includes \_\_\_\_\_\_\_\_\_\_. A. at least one data mart. B. data that can extracted from numerous internal and external sources. C. [near real-time updates.] D. far real-time updates. 30. Data sets are made up of data objects. a. Sets b. Objects c. Mining d. All the above 31. A **data object** represents an entity. a. View b. Knowledge c. Entity d. None of them 32. Data objects are described by **attributes**. a. Tuples b. Attributes c. Constants d. All of them 33......... Value that occurs most frequently in the data a. Mode b. Median c. Mean d. a & c 34......... is a graphic display of five-number summary. a. Boxplot b. Quantile plot c. Scatter plot d. All of them 35. In........x-axis are values, y-axis represents frequencies a. Boxplot b. Quantile plot c. Scatter plot d. Histogram 36. An ordinal variable can be discrete or continuous. a. Ordinal b. Scaled c. Objected d. All of them 37. A **document** can be represented by thousands of attributes a. Tuples b. Attributes c. Constants d. None of them 38. **Scatter plot**:.....: each pair of values is a pair of coordinates and plotted as points in the plane a. Boxplot b. Quantile plot c. Scatter plot d. Histogram 39............. has only a finite or countably infinite set of values e.g., zip codes, profession etc. e. Continuous attribute f. Both a & b g. None of them 40. The active data warehouse architecture includes \_\_\_\_\_\_\_\_\_\_ A. at least one data mart. B. data that can extracted from numerous internal and external sources. C. near real-time updates. D. [all of the above.] 41. Reconciled data is \_\_\_\_\_\_\_\_\_\_\_. A. data stored in the various operational systems throughout the organization. B. [current data intended to be the single source for all decision support systems.] C. data stored in one operational system in the organization. D. data that has been selected and formatted for end-user support applications. 42. Transient data is \_\_\_\_\_\_\_\_\_\_\_\_\_. A. [data in which changes to existing records cause the previous version of the records to be eliminated.] B. data in which changes to existing records do not cause the previous version of the records to be eliminated. C. data that are never altered or deleted once they have been added. D. data that are never deleted once they have been added. 43. The extract process is \_\_\_\_\_\_. A. capturing all of the data contained in various operational systems. B. [capturing a subset of the data contained in various operational systems.] C. capturing all of the data contained in various decision support systems. D. capturing a subset of the data contained in various decision support systems. 44. Data scrubbing is \_\_\_\_\_\_\_\_\_\_\_\_\_. A. a process to reject data from the data warehouse and to create the necessary indexes. B. a process to load the data in the data warehouse and to create the necessary indexes. C. a process to upgrade the quality of data after it is moved into a data warehouse. D. [a process to upgrade the quality of data before it is moved into a data warehouse] 45. The load and index is \_\_\_\_\_\_\_\_\_\_\_\_\_\_. A. a process to reject data from the data warehouse and to create the necessary indexes. B. [a process to load the data in the data warehouse and to create the necessary indexes.] C. a process to upgrade the quality of data after it is moved into a data warehouse. D. a process to upgrade the quality of data before it is moved into a data warehouse. 46. Data transformation includes \_\_\_\_\_\_\_\_\_\_. A. [a process to change data from a detailed level to a summary level.] B. a process to change data from a summary level to a detailed level. C. joining data from one source into various sources of data. D. separating data from one source into various sources of data. 47. \_\_\_\_\_\_\_\_\_\_\_\_ is called a multifield transformation. A. [Converting data from one field into multiple fields.] B. Converting data from fields into field. C. Converting data from double fields into multiple fields. D. Converting data from one field to one field. 48. The type of relationship in star schema is \_\_\_\_\_\_\_\_\_\_\_\_\_\_\_\_\_\_. A. many-to-many. B. one-to-one. C. [one-to-many.] D. many-to-one. 49. Fact tables are \_\_\_\_\_\_\_\_\_\_\_. A. completely demoralized. B. partially demoralized. C. [completely normalized.] D. partially normalized. 50. Business Intelligence and data warehousing is used for \_\_\_\_\_\_\_\_. A. Forecasting. B. Data Mining. C. Analysis of large volumes of product sales data. D. [All of the above.] 51. The data administration subsystem helps you perform all of the following, except\_\_\_\_\_\_\_\_\_\_. A. backups and recovery. B. query optimization. C. security management. D. [create, change, and delete information.] 52. The most common source of change data in refreshing a data warehouse is \_\_\_\_\_\_\_. A. [queryable change data.] B. cooperative change data. C. logged change data. D. snapshot change data. 53. \_\_\_\_\_\_\_\_ are responsible for running queries and reports against data warehouse tables. A. Hardware. B. Software. C. [End users.] D. Middle ware. 54. Query tool is meant for \_\_\_\_\_\_\_\_\_\_. A. [data acquisition.] B. information delivery. C. information exchange. D. communication. 55. Dimensionality reduction reduces the data set size by removing \_\_\_\_\_\_\_\_\_\_\_\_. A. relevant attributes. B. [irrelevant attributes.] C. derived attributes. D. composite attributes. 56. Effect of one attribute value on a given class is independent of values of other attribute is called \_\_\_\_\_\_\_\_\_. A. [value independence.] B. class conditional independence. C. conditional independence. D. unconditional independence. 57. The main organizational justification for implementing a data warehouse is to provide \_\_\_\_\_\_. A. cheaper ways of handling transportation. B. decision support. C. [storing large volume of data.] D. access to data. 58. Multidimensional database is otherwise known as\_\_\_\_\_\_\_\_\_\_\_\_. A. RDBMS B. [DBMS] C. EXTENDED RDBMS D. EXTENDED DBMS 59. Data warehouse architecture is based on \_\_\_\_\_\_\_\_\_\_\_\_\_\_. A. DBMS. B. [RDBMS.] C. Sybase. D. SQL Server. 60. Source data from the warehouse comes from \_\_\_\_\_\_\_\_\_\_\_\_\_\_\_. A. [ODS.] B. TDS. C. MDDB. D. ORDBMS. 61. \_\_\_\_\_\_\_\_\_\_\_\_\_\_\_\_ is a data transformation process. A. Comparison. B. Projection. C. Selection. D. [Filtering.] 62. \_\_\_\_\_\_\_\_\_\_ are designed to overcome any limitations placed on the warehouse by the nature of the relational data model. A. Operational database. B. Relational database. C. [Multidimensional database.] D. Data repository. 63. MDDB stands for \_\_\_\_\_\_\_\_\_\_\_. A. multiple data doubling. B. [multidimensional databases.] C. multiple double dimension. D. multi-dimension doubling. 64. \_\_\_\_\_\_\_\_\_\_\_\_\_\_ is data about data. A. [Metadata.] B. Microdata. C. Minidata. D. Multidata. 65. \_\_\_\_\_\_\_\_\_\_\_ is an important functional component of the metadata. A. Digital directory. B. Repository. C. [Information directory.] D. Data dictionary. 66. EIS stands for \_\_\_\_\_\_\_\_\_\_\_\_\_\_. A. Extended interface system. B. Executive interface system. C. [Executive information system.] D. Extendable information system. 67. \_\_\_\_\_\_\_\_\_\_\_\_ are some popular OLAP tools. A. [Metacube, Informix.] B. Oracle Express, Essbase. C. HOLAP. D. MOLAP. 68. \_\_\_\_\_\_\_\_\_\_\_\_ proposed the approach for data integration issues. A. Ralph Campbell. B. [Ralph Kimball.] C. John Raphlin. D. James Gosling. 69. The terms equality and roll up are associated with \_\_\_\_\_\_\_\_\_\_\_\_. A. OLAP. B. visualization. C. [data mart.] D. decision tree. 70. Exceptional reporting in data warehousing is otherwise called as \_\_\_\_\_\_\_\_\_\_. A. exception. B. [alerts.] C. errors. D. bugs. 71. Removing duplicate records is a process called \_\_\_\_\_\_\_\_\_\_\_\_\_. A. recovery. B. [data cleaning.] C. data cleansing. D. data pruning. 72. \_\_\_\_\_\_\_\_\_\_\_\_ contains information that gives users an easy-to-understand perspective of the information stored in the data warehouse. A. [Business metadata.] B. Technical metadata. C. Operational metadata. D. Financial metadata. 73. \_\_\_\_\_\_\_\_\_\_\_\_\_\_\_ helps to integrate, maintain and view the contents of the data warehousing system. A. Business directory. B. [Information directory.] C. Data dictionary. D. Database. 74. Data marts that incorporate data mining tools to extract sets of data are called \_\_\_\_\_\_. A. independent data mart. B. [dependent data marts.] C. intra-entry data mart. D. inter-entry data mart. 75. Building the informational database is done with the help of \_\_\_\_\_\_\_. A. [transformation or propagation tools.] B. transformation tools only. C. propagation tools only. D. extraction tools. 76. Which of the following is not a component of a data warehouse? A. Metadata. B. Current detail data. C. Lightly summarized data. D. [Component Key.] 77. \_\_\_\_\_\_\_\_ is data that is distilled from the low level of detail found at the current detailed level. A. Highly summarized data. B. [Lightly summarized data.] C. Metadata. D. Older detail data. 78. Highly summarized data is \_\_\_\_\_\_\_. A. [compact and easily accessible.] B. compact and expensive. C. compact and hardly accessible. D. compact. 79. A directory to help the DSS analyst locate the contents of the data warehouse is seen in \_\_\_\_\_\_. A. Current detail data. B. Lightly summarized data. C. [Metadata.] D. Older detail data. 80. Metadata contains at least \_\_\_\_\_\_\_\_\_. A. the structure of the data. B. the algorithms used for summarization. C. the mapping from the operational environment to the data warehouse. D. [all of the above.] 81. The data from the operational environment enter \_\_\_\_\_\_\_ of data warehouse. A. [Current detail data.] B. Older detail data. C. Lightly summarized data. D. Highly summarized data. 82. The data in current detail level resides till \_\_\_\_\_\_\_\_ event occurs. A. purge. B. summarization. C. archieved. D. ~~all of the above.~~ 83. The dimension tables describe the \_\_\_\_\_\_\_\_\_. A. entities. B. [facts.] C. keys. D. units of measures. 84. The granularity of the fact is the \_\_\_\_\_ of detail at which it is recorded. A. transformation. B. summarization. C. [level.] D. transformation and summarization. 85. Which of the following is not a primary grain in analytical modeling? A. Transaction. B. [Periodic snapshot.] C. Accumulating snapshot. D. All of the above. 86. Granularity is determined by \_\_\_\_\_\_. A. number of parts to a key. B. granularity of those parts. C. [both A and B.] D. none of the above. 87. \_\_\_\_\_\_\_\_\_\_\_ of data means that the attributes within a given entity are fully dependent on the entire primary key of the entity. A. Additivity. B. Granularity. C. [Functional dependency.] D. Dimensionality. 88. A fact is said to be fully additive if \_\_\_\_\_\_\_\_\_\_\_. A. [it is additive over every dimension of its dimensionality.] B. additive over atleast one but not all of the dimensions. C. not additive over any dimension. D. None of the above. 89. A fact is said to be partially additive if \_\_\_\_\_\_\_\_\_\_\_. A. it is additive over every dimension of its dimensionality. B. [additive over at least one but not all of the dimensions.] C. not additive over any dimension. D. None of the above. 90. A fact is said to be non-additive if \_\_\_\_\_\_\_\_\_\_\_. A. it is additive over every dimension of its dimensionality. B. additive over atleast one but not all of the dimensions. C. [not additive over any dimension.] D. None of the above. 91. Non-additive measures can often be combined with additive measures to create new \_\_\_\_\_\_\_\_\_. A. [additive measures.] B. non-additive measures. C. partially additive. D. All of the above. 92. A fact representing cumulative sales units over a day at a store for a product is a \_\_\_\_\_\_\_\_\_. A. additive fact. B. [fully additive fact.] C. partially additive fact. D. non-additive fact. 93. \_\_\_\_\_\_\_\_\_\_\_\_ of data means that the attributes within a given entity are fully dependent on the entire primary key of the entity. A. Additivity. B. Granularity. C. [Functional Dependency.] D. Dependency. 94. SQL stand for \_\_\_\_\_\_\_\_\_. A. Standard Query Language. B. [Structured Query Language.] C. Standard Quick List. D. Structured Query list. 95. OLAP stands for A. [Online analytical processing] B. Online analysis processing C. Online transaction processing D. Online aggregate processing 96. Data that can be modeled as dimension attributes and measure attributes are called \_\_\_\_\_\_\_ data. A. [Multidimensional] B. Singledimensional C. Measured D. Dimensional Answer: a Explanation: Given a relation used for data analysis, we can identify some of its attributes as measure attributes, since they measure some value, and can be aggregated upon. Dimension attribute define the dimensions on which measure attributes, and summaries of measure attributes, are viewed. 97. The generalization of cross-tab which is represented visually is \_\_\_\_\_\_\_\_\_\_\_\_ which is also called as data cube. A. [Two dimensional cube] B. Multidimensional cube C. N-dimensional cube D. Cuboid Explanation: Each cell in the cube is identified for the values for the three dimensional attributes. 98. The process of viewing the cross-tab (Single dimensional) with a fixed value of one attribute is A. [Slicing] B. Dicing C. Pivoting D. Both Slicing and Dicing Answer: a Explanation: The slice operation selects one particular dimension from a given cube and provides a new sub-cube. Dice selects two or more dimensions from a given cube and provides a new sub-cube. 99. The operation of moving from finer-granularity data to a coarser granularity (by means of aggregation) is called a \_\_\_\_\_\_\_\_ A. [Rollup] B. Drill down C. Dicing D. Pivoting Explanation: The opposite operation---that of moving fromcoarser-granularity data to finer-granularity data---is called a drill down. 100. In SQL the cross-tabs are created using A. [Slice] B. Dice C. Pivot D. All of the mentioned Explanation: Pivot (sum(quantity) for color in ('dark','pastel','white')). 101. { (item name, color, clothes size), (item name, color), (item name, clothes size), (color, clothes size), (item name), (color), (clothes size), () } This can be achieved by using which of the following ? A. group by rollup B. group by cubic C. group by D. [none of the mentioned] Explanation: 'Group by cube' is used. 102. What do data warehouses support? A. [OLAP] B. OLTP C. OLAP and OLTP D. Operational databases 103. SELECT item name, color, clothes SIZE, SUM(quantity) FROM sales GROUP BY rollup(item name, color, clothes SIZE); How many grouping is possible in this rollup? A. 8 B. C. 2 D. 1 Explanation: { (item name, color, clothes size), (item name, color), (item name), () }. 104. Which one of the following is the right syntax for DECODE? A. DECODE (search, expression, result \[, search, result\]... \[, default\]) B. DECODE (expression, result \[, search, result\]... \[, default\], search) C. DECODE (search, result \[, search, result\]... \[, default\], expression) D. [DECODE (expression, search, result \[, search, result\]... \[, default\])] 105. Treating incorrect or missing data is called as \_\_\_\_\_\_\_\_\_\_\_. A. selection. B. [preprocessing.] C. transformation. D. interpretation. 106. Converting data from different sources into a common format for processing is called as \_\_\_\_\_\_\_\_. A. selection. B. preprocessing. C. [transformation.] D. interpretation. 107. Various visualization techniques are used in \_\_\_\_\_\_\_\_\_\_\_ step of KDD. A. selection. B. transformation. C. data mining. D. [interpretation.] 108. Using an \_\_\_\_ tool, data is extracted from multiple data sources, transformed, and loaded into a data warehouse after joining fields, calculating, and removing incorrect data fields. A. [ETL] B. TEL C. LET D. LTE 109. After business \_\_\_\_, ETL testing ensures that the data has been loaded accurately from a source to a destination. A. Information B. [Transformation] C. Transfusion D. Transfiction 110. During ETL, various stages of data are verified and used at \_\_\_\_. A. Source B. Destination C. [Both A and B] D. None of the above TRUE FALSE 1. Data mining is also known as knowledge discovery from data. (TRUE) 2. Health care & medical data mining -- often adopted such a view in statistics and machine learning. (TRUE) 3. One can mine tremendous amount of "patterns" and knowledge. (TRUE) 4. Algorithms must be highly scalable to handle such as tera-bytes of data. (TRUE) 5. A natural evolution of database technology, is in great demand, with wide applications. (TRUE) 6. A KDD process includes data cleaning, data integration, data selection, transformation, data mining, pattern evaluation, and knowledge presentation. (TRUE) 7. Mining can be performed in a variety of data. (TRUE) Mining can be performed in less or limited data. (FALSE) 8. Outlier: A data object that does not comply with the general behavior of the data. (TRUE) 9. Data mining plays an essential role in the knowledge discovery process. (TRUE) 10. The evolution of Data collection, database creation, IMS and network DBMS occurred in 1960s. (TRUE) The evolution of Data collection, database creation, IMS and network DBMS occurred in 1980s. (FALSE) 11. Preprocessing of the data including feature extraction and dimension reduction. TRUE 12. Web is a big information network: from PageRank to Google. TRUE 13. Micro-array may have tens of thousands of dimensions. TRUE 14. Clustering detect and remove outliers. TRUE Clustering does not detect and remove outliers. FALSE 15. Data migration tools: allow transformations to be specified. TRUE 16. *In object identification* the same attribute or object may have different names in different databases. TRUE 17. *In derivable data o*ne attribute may be a "derived" attribute in another table, e.g., annual revenue. TRUE 18. Correlation does not imply causality. TRUE Correlation imply causality. FALSE 19. **Data reduction** obtain a reduced representation of the data set that is much smaller in volume but yet produces the same analytical results. TRUE 20. Discrete wavelet transforms for linear signal processing, multi-resolution analysis. TRUE Discrete wavelet doesn't transforms for linear signal processing, multi-resolution analysis. FALSE Descriptive: 1. What is an Outlier? A data object that does not comply with the general behavior of the data. 2. Define data sets? Data sets are made up of data objects. 3. Define data object? 4. What is a boxplot in simple terms? Boxplot is a graph summarising a set of data. 5. What are three types of data attribute? 6\. What are the four major tasks in data preprocessing? Data cleaning, data integration, data reduction and data transformation. 7\. give three examples of data cleaning? Handling missing data, removing duplicates and validating accuracy. 8\. what are the two types of data mart? Dependent data mart & independent data mart & hybrid data mart. 9\. what is a [Star schema]? A fact table in the middle connected to a set of dimension tables. 10\. define **Meta data?** **Meta data** is the data defining warehouse objects. Give the full forms of the following: 1. OLAP:- On-Line Analytical Processing 2. NQM:- Net Query Model 3. OLAM:- On Line Analytical Mining (OLAM) 4. DMQL:- Data Mining Query Language 5. ODBC:- Open Database Connectivity 6. OLEDB :- Object Linking and Embedding Database 7. DW:- Data Warehouse. 8. ROLAP:- Relational OLAP 9. MOLAP:- Multidimensional OLAP 10. SQL:- Structured Query language