DRL MCQ 6.pptx
Document Details
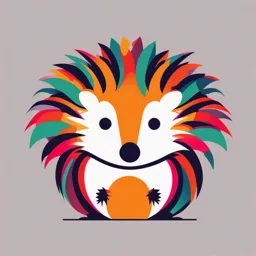
Uploaded by IntuitiveRiver
Yesbud University, School of Excellence
Full Transcript
Data Science Research Process / Life Cycle Learn about the different phases in the data science life cycle and how to create a successful data-driven strategy. The Data Science Research Process Problem Definition Data Governan ce Deployme nt Evaluat n Data Collection 1 Identify Data Sources...
Data Science Research Process / Life Cycle Learn about the different phases in the data science life cycle and how to create a successful data-driven strategy. The Data Science Research Process Problem Definition Data Governan ce Deployme nt Evaluat n Data Collection 1 Identify Data Sources Determine which sources of data are Extract Data 2 relevant for your research project. 3 Validate Data Quality Use APIs and web scraping tools to collect data from various sources. Ensure that the collected data is accurate and free of errors or duplicates. Data Preparation Data Cleaning Data Transformation Data Integration Remove incomplete or irrelevant Convert data into a format that Combine data from multiple data to improve the quality of your can be analyzed and processed sources to build a comprehensive dataset. efficiently. and accurate dataset. Data Analysis Exploratory Data Analysis Descriptive Analytics Predictive Analytics Visualize data distributions Calculate summary statistics Create predictive models and relationships to discover and metrics to describe data based on historical data to patterns and insights. characteristics. forecast future trends. Prescriptive Analytics Recommend optimal decisions or actions based on analytical insights. Data Modeling 1 2 3 Choose Algorithm Train Model Evaluate Model Select an appropriate machine Use a training dataset to build Test your model's ability to learning algorithm for your and refine your model. predict new data accurately. research project. Model Deployment Choose Deployment Method Implement Deployment Decide how to deploy your model: cloud, server, Configure your deployment infrastructure and or on-premises. publish your model as an API or web service. Model Maintenance 1 2 Monitor Model Performance Update Model Track your model's performance and adjust as Retrain your model as new data becomes needed to ensure its accuracy and reliability. available, or as your business requirements evolve. Conclusion The data science life cycle is a continuous process that requires expertise in statistics, programming, and domain knowledge. By following these Best Practices, you can create a data-driven culture and obtain valuable insights from data to drive your business goals forward. Project Management in AI, Machine Learning, and Data Science Effective project management is critical to ensuring the success of AI and data science initiatives. In this presentation, we'll explore the unique challenges of managing these projects and discuss best practices for achieving your goals. What is Project Management? Project Planning Team Management Risk Management Developing a detailed project Ensuring that all team Identifying potential risks and plan that outlines the scope, members understand their developing strategies for timeline, budget, and roles and responsibilities and mitigating them to minimize resources required to complete are working effectively potential impacts on the the project successfully. towards the project goals. project. Project Monitoring and Control Monitoring project progress and adjusting the project plan as needed to ensure successful completion. Why Data Science Initiatives Require Effective Project Management? Data Analysis Complexity Cross-functional Collaboration Data science projects involve analyzing Many data science projects involve Data science projects often require large volumes of complex data, requiring developing complex machine learning collaboration across multiple teams, careful planning and management to algorithms requiring extensive technical making it important to have clear goals, ensure accurate and meaningful insights. expertise, so effective project deadlines, and processes in place to management is essential to ensure the manage these relationships effectively. successful development of these algorithms. How to Project Manage AI Engagements? 1 Define Project Objectives Clearly define and prioritize project goals based on business needs, technological capabilities, and feasibility. 2 Develop a Detailed Project Plan Create a comprehensive project plan that outlines the project scope, timeline, budget, and resources required. 3 Building the Team Assemble a team with the required expertise and skills, including data scientists, developers, business analysts, and project managers. 4 Execution and Monitoring Execute the project plan, monitor progress, and adjust the plan as needed to ensure successful completion of the project. Data Science Project Management Approaches 1 Waterfall 2 Agile 3 Scrum A linear approach to project An iterative approach that A subset of Agile that management that involves emphasizes flexibility, focuses on teamwork, sequential stages, each collaboration, and customer accountability, and iterative completed before moving to feedback to deliver the final progress delivery through the next one. product. sprints. The ML Blueprint Mathema tics Algorith ms Langua ges Librar ies Essential skills and technologies for developers to embrace machine learning Too ls Conclusion and Next Steps Partnership Plan Your Next Project Beyond Project Management Whatever the size of your AI and Take your next step by outlining your AI and Data Science presents Data Science initiatives, effective project scope, deliverables, and limitless opportunities, let's put on project management is essential to timeline. Document intended our thinking hats and brainstorm! their success. Let's work together to resources, develop risk management make your project a success! strategies and explore your approach to project management! Agile Framework for Data Science Agile methodology has revolutionized the way software development works. In this presentation, we will see how Agile principles can be applied in Data Science. What is Agile? 1 A Customer-Centric Approach 🎯 2 Iterative Development 🔄 Agile development is based on In Agile methodology, the the idea of breaking down a focus is on delivering value to project into smaller, more the customer through manageable chunks, and continuous iteration and delivering them incrementally. improvement. 3 Collaboration 💬 4 Continuous Improvement 📈 Agile methodology requires close collaboration between The Agile framework focuses cross-functional teams, on continuous learning and including developers, data improvement, so that the scientists, and stakeholders. team can adapt to changing requirements and improve their processes with each iteration. Agile Data Science Best Practices Start Small Continuously Test and Refine Stakeholder Involvement projects into smaller, more Tests should be conducted every step of the way, from manageable chunks, and deliver continuously to ensure that each project kickoff, standups to them incrementally. iteration or module delivers as project delivery. Break down your data science planned and meets the requirements. Stakeholders should be involved Agile Data Science Principles Embrace Change 🌪 ️ Instead of resisting change, embrace it, and adapt as fast as possible to changing requirements. 1 2 3 Satisfy the Customer 🤝 Collaborate 🎤 Deliver value to the customer through continuous delivery. Cross-functional teams must collaborate closely to improve the development process ongoing. Key Concepts…. Scrum Stand-up Kanban A methodology for project A short daily meeting where A visual workflow management that emphasizes team members discuss their management tool, which teamwork, accountability and progress and any problems allows teams to visualize work, iterative progress toward a they're facing. optimize resources and well-defined goal. improve delivery times. DevOps A set of practices that automates the processes between software development and operations teams, allowing for faster delivery and shorter cycle times. Agile Roles in Data Science 1 2 3 4 Product Owner 🧑💼 Data Scientist 👩💻 Scrum Master 👨✈️ Responsible for the Applies statistical Responsible for Analytics Engineer 👨💻 success of the and machine ensuring that the Responsible for product, working learning techniques Scrum team is building and with the stakeholders to vast quantities of adhering to Scrum maintaining the data to define the product data to extract theory, practices, infrastructure and goals, and insights and and rules throughout data pipeline used by coordinating its generate value for the project lifecycle. the data scientists in development. the customer. their analysis work. Agile Data Science Framework Kickoff 🎬 Data Analysis 📊 Code Development 🖥 ️ Testing & Delivery 🚀 The first step is to define the project Once the data is available, data Once the insights are found, the next The testing phase is crucial to ensure goals, scope, and timeline with scientists use their skills and expertise step is to develop the code or that the software works as intended stakeholders and align everyone's to explore patterns and extract algorithm to achieve the project goals. and delivers the value the customers expectations. insights. are expecting. Benefits of Agile Framework in Data Science Flexibility Collaboration Iterative Process Offers flexibility to adjust the Promotes cross-functional Delivers a working model of the project scope and requirements collaboration, customer product at the end of each based on changing business feedback, and team member iteration, enabling stakeholders needs and market conditions. engagement, improving project to provide feedback and adjust outcomes. project requirements accordingly. Agile Data Science Challenges Data Quality Technical Debt Managing Stakeholders One of the biggest challenges in Technical debt is the accrual of Managing all the stakeholders Agile Data Science is also one of development work that is put off involved can be a significant the most fundamental - the in favor of faster delivery to challenge, as many projects are quality of data itself. maintain the pace of Agile. held up waiting on information from the stakeholders.