DRL MCQ 5.pptx
Document Details
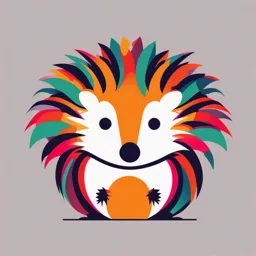
Uploaded by IntuitiveRiver
Yesbud University, School of Excellence
Full Transcript
Advanced Analytics in Pharma An Introduction Advanced Analytics in Pharma Manufacturing Pharma manufacturing is a complex process with a lot of moving parts. Advanced Analytics is a game changer for this industry, solving problems and providing insights that would otherwise be impossible. How...
Advanced Analytics in Pharma An Introduction Advanced Analytics in Pharma Manufacturing Pharma manufacturing is a complex process with a lot of moving parts. Advanced Analytics is a game changer for this industry, solving problems and providing insights that would otherwise be impossible. How Advanced Analytics are benefiting Pharma Manufacturing ? Red PQ Benefit s Pharma Manufacturing Overview What is Pharma Manufacturing? Key Challenges Industry Trends Pharma manufacturing Increased demand for The process of producing involves complex processes, personalized medicine and pharmaceutical products for strict regulations and high new innovative drugs requiring human or animal consumption quality standards. more flexible manufacturing in a safe and cost-effective manner. processes. The Challenges of Pharma Manufacturing Regulatory Compliance Quality Control Resource Allocation Production schedules need to Production runs need to meet Optimizing manufacturing be meticulously tracked and strict quality standards and processes requires juggling the documented to ensure meet pharmaceutical testing competing priorities of time, compliance with FDA requirements. cost, and quality. regulations. Introduction to Advanced Analytics What is it? How does it work? Why is it important? Advanced Analytics is a broad These tools use machine learning This approach allows term that encompasses a variety algorithms to identify patterns and manufacturers to identify problems of tools and techniques used to insights in large amounts of data. and optimize processes in ways analyze complex data sets. that are simply not possible with traditional analysis tools. Benefits of Advanced Analytics • Optimized production processes • Improved product quality • Reduced manufacturing costs • Lower risk of product recalls • Real-time monitoring and feedback • Better use of resources • Increased efficiency • Improved decision making Applications of Advanced Analytics 1 Drug Discovery & Development Advanced Analytics can help manufacturers Manufacturing Process Monitoring 2 Real-time monitoring of manufacturing data, identify new target molecules and predict the effectiveness of drug compounds. This helps them select the most promising collected from various sensors and candidates for clinical trials. processes, provides valuable insights. Manufacturers can continuously monitor processes to ensure consistent quality. 3 Sales & Marketing Analytics Pharmaceutical manufacturers can analyze their sales and marketing data to better Supply Chain Optimization Using analytics to optimize logistics and reduce costs, manufacturers can ensure that products are delivered on time and with fewer interruptions. 4 understand consumer behavior. They can also track market trends and uncover new opportunities. Implementation of Advanced Analytics Data Collection & Management Modeling & Machine Learning Integrated Systems & Partnerships Pharmaceutical manufacturers Manufacturers must develop Finally, the most successful must collect data from multiple and train models to analyze implementations of advanced sources, including sensors, the collected data. This analytics in pharma equipment, and people. requires the use of manufacturing typically Collecting data is only the first sophisticated machine learning involve an integrated suite of step, however; they also need algorithms that can identify systems and partnerships with to manage it in a scalable way. patterns and insights in large vendors. amounts of data. Future Trends in Analytics • Increase in predictive analytics • Growth in real-time monitoring • Increased adoption of cloud-based analytics • Greater use of artificial intelligence and machine learning • More emphasis on data privacy and security Real World Examples GlaxoSmithKline Eli Lilly and Co. Merck GSK is using advanced analytics to Eli Lilly and Co. used advanced Merck used advanced analytics to predict and manage quality issues analytics to optimize waste disposal improve their drug discovery before they occur. This has resulted in their manufacturing processes. process. By analyzing large amounts in improved compliance, lower This resulted in significant cost of data, they were able to accelerate costs, and increased efficiency. savings and a reduced the discovery of new drugs and environmental impact. reduce costs. AI-ML in Pharma Manufacturing Join us on a journey to explore the benefits, challenges and applications of AI-ML technology in the field of pharma manufacturing. Applications of AI-ML in Pharma Manufacturing Manufacturing Processes Drug Discovery Data Analytics Automating various steps of Using machine learning to speed up AI-ML algorithms help pharma manufacturing processes such as the drug discovery process and manufacturers make sense of large assembly, inspection, and develop new treatments with amounts of data and provide packaging to increase efficiency and greater accuracy. insights into how to optimize their quality operations. Benefits of AI-ML in Pharma Manufacturing Predictive Maintenance Using AI-ML can help detect and predict equipment failures and maintenance needs, reducing downtime and costs. 1 2 3 Process Optimization Quality Control AI-ML enables manufacturing processes to be AI-ML can improve detection of defects, quality more efficient, flexible and reduces errors. issues and product failures, allowing for more accurate and timely responses. Successful Implementation of AI-ML 1 GlaxoSmithKline 2 Pfizer Used AI-ML to decrease Implemented predictive drug discovery time from maintenance systems in four years to one in a manufacturing plants to project that looked into decrease breakdowns pharmaceutical lead and increase uptime. optimization. 3 Merck Implemented AI-ML to analyze large amounts of data for the development of both small and large molecules in the manufacturing process. Future Possibilities and Developments 1 Predictive Analytics Using machine learning to predict a variety Smart Manufacturing 2 of outcomes such as demand for drugs and 3 Advanced Analytics supply chain inefficiencies. AI-ML allows companies to achieve smart manufacturing capabilities in the pharma industry, to enable a fully connected workforce and supply chain. Using AI and big data analytics to create a predictive maintenance roadmap that optimizes manufacturing efficiency based on insights obtained from analyses of infrastructure and product performance. Considerations for Companies Regulatory Compliance Data Management and Security Employee Training and Education pharma industry and AI-ML Ensure that robust data Training and education are key systems should be auditable, management systems are put to successful integration of AI- transparent and in compliance in place to protect sensitive ML systems, including with FDA or EMEA regulations. data and ensure data privacy developing necessary skills and security. among employees and Compliance is critical in the encouraing data-driven decision making. Conclusion and Key Takeaways AI-ML is a game changer in Pharma Manufacturing Preparation is Key Future is Bright Companies must properly The future for AI-ML in pharma prepare for the adoption of AI-ML manufacturing is very promising The benefits of AI-ML in pharma solutions to avoid and overcome and it will continue to transform manufacturing are significant, the challenges. the industry in ways we cannot and more and more companies are embracing this technology. yet imagine. Applications of Machine Learning in Pharma Manufacturing Machine learning (ML) is becoming widely implemented in the pharmaceutical manufacturing industry. Here, we will explore some of the ways in which this technology is transforming the field. Applications of ML in Pharma Manufacturing Quality Control Drug Discovery Packaging Optimization Machine learning algorithms can be ML can accelerate the drug By analyzing market trends and used to analyze product quality, discovery process by identifying key consumer preferences, ML can detect defects, and predict quality features in chemical compounds, optimize packaging design and issues on the production line. predicting drug efficacy, and materials to improve efficiency and simulating cellular responses. reduce waste. Benefits of ML in Pharma Manufacturing 1 3 Increased Efficiency 2 Improved Quality ML can automate ML can identify defects routine tasks, reduce and quality issues errors, and optimize earlier in the production production processes, cycle, reducing product resulting in significant recalls and improving cost and time savings. customer satisfaction. Enhanced Innovation ML can help identify new opportunities for drug development, optimize clinical trials, and drive continuous process improvement. Real-World Examples of ML in Pharma Manufacturing 1 Amgen Developed ML models to optimize upstream and downstream processes in bioreactors, resulting in faster and more efficient drug development. 2 Novartis Uses predictive ML algorithms to forecast product demand, optimizing inventory management and reducing waste. 3 Pfizer Implemented a predictive maintenance system for manufacturing equipment, reducing unplanned downtime and maintenance costs. 4 Roche Used ML to analyze patient data and predict which patients would benefit most from cancer immunotherapy drugs, improving patient outcomes. Potential Impact of ML on the Pharma Industry The adoption of ML in pharma has the potential to transform the industry, resulting in faster drug development, improved clinical outcomes, and reduced costs. However, the implementation of this technology also raises ethical and regulatory concerns that need to be addressed. Improved Patient Outcomes Greater Efficiency New Business Models By leveraging ML to optimize ML can streamline The implementation of ML could drug development and clinical manufacturing processes, lead to new and disruptive trials, we could see more reduce waste, and lower overall business models, such as personalized, effective, and costs. outcome-based pricing and targeted treatments. personalized medicine. Conclusion and Future Directions Overall, machine learning has the potential to revolutionize the pharmaceutical industry by improving efficiency, reducing costs, and increasing innovation. As this technology continues to evolve, it is important to carefully consider the ethical and regulatory implications of its use.