DRL MCQ 4.pptx
Document Details
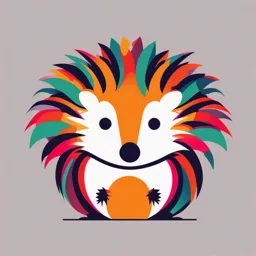
Uploaded by IntuitiveRiver
Yesbud University, School of Excellence
Full Transcript
Data Management in Pharma Manufacturing Pharmaceutical production is a highly complex process requiring extensive data management. This session will cover challenges, trends, best practices and more. Structured data Can be displayed in rows, columns Numbers, and relational dates, databases stri...
Data Management in Pharma Manufacturing Pharmaceutical production is a highly complex process requiring extensive data management. This session will cover challenges, trends, best practices and more. Structured data Can be displayed in rows, columns Numbers, and relational dates, databases strings Estimated 20% of enterprise data (Gartner) Requires less storage Easier to manage and protect with legacy solutions Unstructured data Cannot be displayed in rows, columns and relational databases Images, audio, video, word processing files, emails, spreadsheets Estimated 80% of enterprise data (Gartner) Requires more storage More difficult to manage and protect Data • Internal versus Types external • Structured versus unstructured • Machine generated versu human generated •Static versus dynamic •Raw versus preprocessed •Transactional or not •Sensor data or not s Nano data problems need tender care Medium data problems are playful and most enjoyable Big data problems require engineering brute force Nano, medium and big data problems AI-ML is data hungry & sensitive Challenges 1 Volume 2 Diversity 3 Quality The sheer volume of data Data generated in Data quality is paramount in generated in pharmaceutical pharmaceutical manufacturing is pharmaceutical manufacturing, manufacturing processes is highly diverse, ranging from where errors in data collection, increasing, leading to challenges chemical and biological data to processing or analysis can lead of data storage, sharing and manufacturing and clinical data. to costly quality issues, product analysis. Managing diversity is a major recalls, and loss of reputation. challenge. 4 Compliance Data management is subject to stringent regulatory requirements, both in terms of data privacy and product quality. Compliance with regulations remains a challenge. Trends Smart manufacturing Big data analytics Cloud computing Cybersecurity Pharma manufacturing facilities are Data analytics and machine learning Cloud computing is increasingly being Pharmaceutical manufacturers are increasingly adopting smart algorithms are being extensively used used in pharmaceutical manufacturing focusing more on cybersecurity technologies, including automation, in pharmaceutical manufacturing to to store, share, and analyze data in a measures to protect sensitive data the internet of things (IoT), and analyze complex data sets, identify cost-effective and scalable manner, from sophisticated cyber threats, artificial intelligence (AI), leading to patterns, and predict outcomes, enabling easy access to data from including data breaches, ransomware more data generation and higher leading to more effective data-driven anywhere and anytime. attacks, and other malicious activities. efficiency in data management. decision making. Best Practices 1 Data Governance Framework A data governance framework should be established Master Data Management 2 A master data management system should be to define policies, standards, roles, and responsibilities for data management, ensuring data quality, security and compliance. established to maintain a central repository of master data, harmonizing data across functions and systems, thereby ensuring data integrity and consistency. 3 Data Analytics Data analytics should be integrated with the manufacturing process to facilitate real-time Data Security Data security measures should be established to protect sensitive data from unauthorized access, including encryption, access controls, backup, and disaster recovery. 4 monitoring, predictive analytics and prescriptive analytics for more informed decision making. Real World Examples Sanofi Cipla Pfizer Sanofi deployed a data Cipla established a master Pfizer developed a data integration platform to data management system to analytics platform to automate integrate data from various harmonize data across data analysis, enabling real- sources into a unified view, systems, reducing data time decision making and facilitating decision making redundancy and improving the reducing the risk of errors. and improving operational quality of data. efficiency. The Novartis data lifecycle Data Biases ML carries with it all the biases that data carries Types of Bias in Statistics and the Affect Data Bias Has on Your Business | Mailchimp Fairn ess • What do you see? • Bananas • Stickers • Bananas on shelves What do you see? What do you see? • Green Bananas • Unripe Bananas • Overripe Bananas • Good for Banana Bread Yellow Bananas Yellow is prototypical for bananas Designing for Fairness Consider the problem Ask experts Train the models to account for bias Interpret outcomes Publish with context Fairness: Identifying Bias Missing Feature Values If your data set has one or more features that have missing values for a large number of examples, that could be an indicator that certain key characteristics of your data set are underrepresented. Unexpecte d Feature Values When exploring data, you should also look for examples that contain feature values that stand out as especially uncharacteristic or unusual. These unexpected feature values could indicate problems that occurred during data collection or other inaccuracies that could introduce bias. Data Skew Any sort of skew in your data, where certain groups or characteristics may be underor over-represented relative to their real-world prevalence, can introduce bias into your model. If you can’t measure it, you can’t manage it If it’s easy, it’s probably wrong. A classifier is only as good as the metric used to evaluate it. Types of metrics for evaluating ML models Probabi lity Ranki ng Thresh old Common Metrics That Are Used To Evaluate Predictive Models 1. Confusi on Matrix 5. AUC – ROC 2. F1 Score 3. Gain and Lift Charts 6. Log Loss 7. Gini Coecie nt 9. Root Mean Squared 4. Kolmogoro v Smirnov Chart 8. Concordant – Discor dant Ratio Performance Metrics for Machine Learning Models Performance Metrics for Classification Problems Accura cy Confusi on Matrix RO C Performance Metrics for Regression Problems Log Probabil ity R Square Adjuste dR Square MS E RMS E MA E MAD E MAP E “The unexamined life is not worth living.” Performance MeasurementBasics with examples “The unexamined ML model is not worth-production.” Problems with Accuracy • Assumes equal cost for both kinds of errors – cost(b-type-error) = cost (c-type-error) • is 99% accuracy good? – can be excellent, good, mediocre, poor, terrible – depends on problem • is 10% accuracy bad? – information retrieval • BaseRate = accuracy of predicting predominant class (on most problems obtaining BaseRate accuracy is easy) Percent Reduction in Error • • • • 80% accuracy = 20% error suppose learning increases accuracy from 80% to 90% error reduced from 20% to 10% 50% reduction in error • 99.90% to 99.99% = 90% reduction in error • 50% to 75% = 50% reduction in error • can be applied to many other measures Conclusion Data management is an essential component of pharmaceutical manufacturing. By implementing best practices and adopting emerging trends, manufacturers can effectively manage data, improve operational efficiency, and drive business growth. References Sanofi. “Using Data Integration to Improve Collaboration and Efficiency.” Cipla. “Harmonizing Data Across Systems for Improved Data Quality.” Pfizer. “Automated Data Analysis: A Game Changer for Pharmaceutical Manufacturin