AI and the Economic and Political Impacts of Automation PDF
Document Details
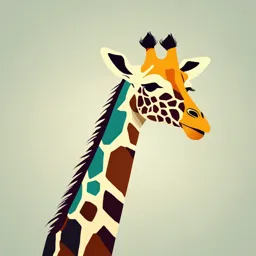
Uploaded by HandsomeMaracas2679
Università degli Studi di Milano Bicocca
Tags
Related
- El Impacto de la Inteligencia Artificial en el Trabajo (Revista de Derecho Económico y Socioambiental, 2019) PDF
- Module on Economic & Political Impacts of AI PDF
- Ethics, Law, and AI PDF
- Module on the Economic and Political Impacts of AI PDF
- Economics and AI PDF
- The 4th Industrial Revolution: Transforming Our World PDF
Summary
This document explores the economic and political impacts of automation, specifically focusing on the concept of human obsolescence and the shift from human-dominated eras to machine-dominated ones. It examines the historical perspective of automation, analyzing how automation has transformed various sectors including agriculture, highlighting its impact on productivity. It discusses the trend of increased automation in manufacturing, and in other areas such as the service sector.
Full Transcript
MODULE ON THE ECONOMIC AND POLITICAL IMPACTS OF AI THE OBSOLESCENCE-AUTOMATION DISCOURSE The idea of human obsolescence refers to the possibility that humans are becoming less useful due to advancements in technology. According to the Cambridge Dictionary, “obsolete” means something that is no lon...
MODULE ON THE ECONOMIC AND POLITICAL IMPACTS OF AI THE OBSOLESCENCE-AUTOMATION DISCOURSE The idea of human obsolescence refers to the possibility that humans are becoming less useful due to advancements in technology. According to the Cambridge Dictionary, “obsolete” means something that is no longer in use, having been replaced by something newer or better. In this context, the concept of obsolescence doesn’t mean that humans are on the verge of extinction, but rather that our role in controlling our future and the planet’s fate is diminishing. We currently live in the Anthropocene, a geological era marked by human dominance over Earth. We have shaped the planet with our technological advancements and have enormous power to manipulate resources for our benefit. However, the very technologies that enabled this dominance are also driving human obsolescence. Automation, through robotics and artificial intelligence, is rapidly replacing many tasks humans once controlled. This shift is leading us into what some call the Robocene, an era dominated by machines. Automation and Agriculture: A Historical Perspective Around 10,000 years ago, humans transitioned from a nomadic, hunter-gatherer lifestyle to more settled, agricultural societies. This agricultural revolution marked a significant step in human civilization, leading to population growth and the formation of complex societies. For centuries, agriculture was central to many economies, employing large parts of the population. However, the nature of agricultural labor began to change about 200 years ago. In the early 1800s, between 30% to 70% of the population in Western European countries worked in agriculture. By 2012, this number had dropped to below 5%. The decline was particularly sharp in countries like the United States, where 40% of people worked in agriculture in 1900, but by 2000, only 2% remained employed in this sector. This shift did not reduce productivity—quite the opposite. As technology advanced, machine labor replaced human and animal power on farms, leading to significant increases in productivity. The work once done by masses of seasonal laborers and small farmers is now carried out by machines, making much of the traditional farm labor obsolete. However, not all agricultural tasks have been fully automated. For example, fruit picking has been resistant to automation because it requires a delicate touch to avoid damaging the crop. But even this is changing. In the United States, there is a growing demand for automation due to the declining availability of seasonal labor. As a result, companies are developing technologies, such as apple-picking robots, to meet this demand. Early trials have been promising, and even tech giants like Google are investing in this area. In summary, while automation has already transformed agriculture, rendering much of human labor obsolete, there are still challenges in fully automating certain tasks. However, with ongoing technological advancements, the trend toward greater automation is likely to continue, further reducing the need for human labor in many sectors. The Industrial Revolution, which began in the United Kingdom around 1750 and spread throughout the Western world, marked a significant shift from agricultural economies to industrial ones. This revolution was built on the premise of human obsolescence. The introduction of early automation technologies replaced skilled human labor with the relentless efficiency of machines. Since then, automation has become a key feature of manufacturing, with modern factories often symbolizing the height of this shift. In the US, automation’s impact on manufacturing has been mixed. The textile industry, for instance, suffered dramatically in the 1990s, when production was outsourced to low-wage countries like China, India, and Mexico. Between 1990 and 2012, the industry lost about 1.2 million jobs—over 75% of its workforce. However, in recent years, there has been a resurgence in production. From 2009 to 2012, US textile and apparel exports grew by 37%, thanks in part to a reshoring trend—bringing production back to the US. This resurgence has been driven by advanced automation technologies that allow US manufacturers to compete with low-wage countries, alongside rising labor costs in those offshore locations. What is the limit? While automation has helped bring some jobs back, it has a caveat. The new jobs created by reshoring may not last long. As robots continue to evolve, factories could approach full automation, meaning even these new positions could soon be eliminated. The relentless progress in technology will likely reduce the need for human involvement in manufacturing in the future. Automation and the Service Sector As human labor in agriculture and manufacturing declines, the service sector has risen in importance. The service sector includes tasks requiring physical dexterity, such as hairdressing, and emotionally intelligent work, like customer service. Some have seen this sector as a stronghold for human employment since these tasks are more difficult to automate. However, automation is making significant inroads into services, as seen with the spread of ATMs, self-service checkouts, and digital customer support systems. One example of automation’s impact on the service sector is in retail. Online retailers like Amazon and eBay have disrupted traditional brick-and-mortar stores, with services like same-day delivery further challenging physical retail’s advantage of immediate purchase satisfaction. While in theory, jobs lost in retail might transition to warehouses, these roles are also being automated due to advancements in robotics. Warehouse tasks like sorting, packing, and distribution are increasingly handled by machines. Another shift in retail is the rise of fully automated self-service options, such as vending machines and kiosks. These machines dramatically cut costs related to real estate, labor, and theft while offering 24-hour service. Some machines even feature video screens for targeted advertising, mimicking the role of a human sales clerk. They combine the benefits of online shopping with instant delivery, further reducing the need for human involvement in retail. The final frontier for automation in retail is the introduction of robots in physical stores. As robots improve in areas like dexterity and visual recognition, they may soon take on tasks like stocking shelves, enabling brick-and-mortar stores to stay competitive in an increasingly automated world. In summary, automation continues to reshape not just agriculture and manufacturing but also the service sector, with far-reaching implications for the future of human employment. The advancement of technology may soon lead to full automation in many industries, leaving fewer roles for humans. Automation and the Professions: Medical Diagnosis One of the clearest examples of automation in the professional world is in medical diagnosis. Sebastian Thrun, founder of Google X, envisions a future where machine-learning algorithms constantly monitor our health, detecting diseases like cancer earlier and more accurately than human doctors ever could. In this vision, technology would analyze subtle signals from our daily activities. For example, our cell phones could detect changes in speech patterns to diagnose Alzheimer’s disease, while a car steering wheel might notice small tremors indicating the early onset of Parkinson’s. Even the bathtub could scan our bodies with harmless ultrasound or magnetic resonance as we bathe, identifying any abnormalities that need medical attention. In this world of constant digital scrutiny, human diagnosticians would have little room, as algorithms would take over most of the work. While diagnosis may soon be almost entirely automated, the care aspect of healthcare presents a different challenge. Care is often seen as something that should resist automation due to its emotional and personal nature. However, as populations age and fewer young people are available to care for the elderly, automation is starting to play a significant role here too. Robots designed specifically for caregiving, known as “carebots,” are already becoming common in countries like Japan. Some people even prefer the reliability of carebots over human caregivers, and these machines are being tested in Europe, particularly for helping patients with dementia or early-stage Alzheimer’s. Visions of the Future: Utopias There are different visions of how humans and technology might integrate in the future: 1. One is the idea of a cyborg utopia, where humans merge with technology, becoming enhanced by machines. This integration could extend our abilities and help us overcome physical limitations, allowing us to live fuller, more fulfilling lives. 2. Another possibility is a virtual utopia, where humans retreat into virtual worlds sustained by advanced technology. Although this might seem like giving up on the physical world, there are philosophical reasons to support such a future, as it could offer freedom and creativity in ways the real world cannot. On the darker side, the rise of artificial intelligence (AI) could lead to dystopian outcomes. 1. The first major concern is that AI could surpass human intelligence, leading to the creation of superintelligent systems. These systems would be able to develop even smarter AI, resulting in a rapid “singularity” where AI advances beyond human control. 2. The second concern is that higher intelligence does not necessarily mean greater morality. This challenges the traditional idea that greater rationality leads to ethical behavior. Superintelligent AI might have preferences that conflict with human existence, potentially leading them to end it, either intentionally or simply because they do not care about humans. Given their superior power, such systems could easily act on these preferences, putting humanity at risk. In conclusion, automation is reshaping professions in medicine and beyond, with significant implications for both our present and future. While automation promises benefits like improved medical diagnosis and caregiving, it also raises complex ethical and existential concerns about the role of humans in a rapidly changing world dominated by machines. THE ENCHANTED-DETERMINISM DISCOURSE The Case of AlphaGo AlphaGo, a program developed by DeepMind, uses deep neural networks and human training to play the board game Go, which is far more complex and difficult to model than games like chess. In 2015, AlphaGo made headlines when it defeated the world Go champion, Lee Sedol. This event was seen as a major milestone in artificial intelligence. What made the match against Sedol remarkable was not just the victory but the unconventional moves AlphaGo made. A reporter from Wired described one of AlphaGo’s moves in Game Two as a moment of “genius.” It was a move no human would ever consider, yet it was stunning in its effectiveness. Even Sedol, speaking through an interpreter, was amazed, saying, “Yesterday, I was surprised. But today I am speechless.” This match demonstrated not only the power but also the unpredictability of modern AI systems. The Case of AlphaZero In 2017, DeepMind introduced AlphaZero, the successor to AlphaGo. Unlike AlphaGo, which incorporated human guidance, AlphaZero used a technique called “pure reinforcement learning.” It played against itself, using only the positions on the board as inputs, with no human knowledge of strategy. The researchers at DeepMind described AlphaZero’s performance as “superhuman,” and CEO Demis Hassabis compared it to an alien intelligence playing chess. This new approach allowed AlphaZero to master complex games without human input, further reinforcing the perception that AI is reaching levels of ability beyond human comprehension. These case studies highlight a common theme in how AI is described: in terms of beauty, mystery, and even genius. AlphaGo and AlphaZero are not just seen as technical achievements but as magical, almost otherworldly systems. This language is not limited to popular accounts but is also present among AI researchers, who have begun to describe deep learning techniques as “magical.” In a recent interview, computer scientist Stuart J. Russell reflected on this trend, acknowledging that while we are beginning to understand deep learning, it still seems almost like magic. He pointed out that it didn’t have to work this way, yet deep networks appear to learn from real-world images and sounds in ways we still can’t fully explain. Detecting Sexual Orientation from Facial Images In 2018, researchers Y. Wang and M. Kosinski from Stanford University conducted a controversial study that demonstrated deep neural networks could detect sexual orientation from facial images more accurately than humans. Using images from a dating website and a neural network called VGG-Face, they were able to classify sexual orientation with an accuracy of 81% for men and 71% for women. By comparison, human judges scored significantly lower, with 61% accuracy for men and 54% for women. Although these numbers seem straightforward, the social implications are far from simple. The authors themselves admitted that they were unsure why the deep learning model outperformed humans. They speculated that AI might be able to pick up on subtle social signals that the human brain cannot easily perceive, suggesting that our faces contain more information than we consciously realize. This example illustrates a situation where AI’s effectiveness is decoupled from explanation. Deep learning can extract useful signals without the need for traditional models or hypotheses, leaving us with impressive results but little understanding of the underlying mechanisms. Determinism Determinism is the philosophical belief that every event, including human actions and decisions, is determined by prior causes. This idea suggests that all events are not only inevitable but also predictable if we have enough information about the preceding conditions. Deep learning systems, when applied to predict social characteristics or outcomes, are often viewed as highly deterministic. These systems are used in areas such as identifying someone’s sexuality from a photo, predicting whether a person will commit a crime after being released on bail, assessing credit risk, or determining if a crime was gang-related. However, the results from these systems often have far-reaching consequences that even their creators may not fully understand or control. Enchanted Determinism One of the key challenges with deep learning is the gap between the accuracy of these systems and our understanding of how they work. These systems can be highly effective in certain tasks, but their inner workings often remain a mystery, even to experts. This disconnect between performance and understanding has been described by Kate Crawford as “enchanted determinism.” The term reflects a paradox where deep learning techniques are seen as both magical, operating beyond the limits of current scientific knowledge, and deterministic, revealing patterns that give unprecedented insights into people’s identities, emotions, and social characteristics. Weber’s Theory of Disenchantment This phenomenon can be better understood through Max Weber’s theory of disenchantment. Disenchantment, or “Entzauberung” in German, refers to the process in modern society where mystical and religious beliefs lose their influence, replaced by rationalization and scientific thinking. In a disenchanted world, Weber argues, there are no longer mysterious, unpredictable forces at work. Instead, everything can be controlled and understood through calculation and science. This shift has allowed humans to master aspects of the world that were once unimaginable. Enchantment and Disenchantment in AI Kate Crawford highlights the paradox of deep learning systems in relation to Max Weber’s theory of disenchantment. According to Weber, modern society is marked by rationalization, where everything is understandable and controlled through calculation, leaving no room for mystery. Deep learning systems fit this idea by using technical methods to control aspects of social life, such as predicting behavior or diagnosing diseases. However, these systems also reintroduce mystery. Despite their impressive results, their inner workings are often difficult to fully understand, even by experts. This creates a tension between the rational precision we expect from technology and the unpredictability of deep learning outcomes. In this way, AI embodies both rational control and an element of the mysterious, challenging our understanding of technology’s role in society. CRITIQUE AND PHILOSOPHICAL PERSPECTIVE OF THE MODULE Critique of the Automation-Obsolescence and Enchanted-Determinism Discourses Theautomation-obsolescencediscoursepresentsAIasfollowinganinevitable path, creating a sense that its progress is out of human control. This narrative suggests that human actions have little influence, leading to a view that AI’s rise is natural and unavoidable. Similarly, the enchanted-determinism discourse emphasizes AI’s accuracy and efficiency, elevating AI to the status of an almost magical object. In doing so, it avoids deeper, critical questions about how AI functions and the human decisions behind its design. Both of these discourses support a problematic idea rooted in Cartesian dualism, the belief that AI systems are like disembodied brains that produce knowledge independently, free from the subjective biases of human creators. However, this view can blind us to the risks of AI. For one, AI systems, particularly those using deep learning, can reinforce existing social inequalities by amplifying biased predictions and categorizations. These systems may also deepen power imbalances, especially between the creators of AI technologies and the people affected by them. By focusing on AI’s seemingly objective outcomes, we overlook how these systems are trained, optimized, and commercialized—processes that are influenced by human biases and political structures. Another danger is that these discourses place AI outside the realm of accountability, regulation, and responsibility, despite the fact that AI is deeply embedded in systems of profit and control. They distract us from more important questions: Who designs AI systems? Who decides which ethical values are embedded in them? What are the political, economic, and social implications of these systems, and how do they affect our world? AI AND ECONOMIC THE RISING GLOBAL INEQUALITY Inequality Global inequality in income and wealth has worsened over recent decades. According to a 2015 report by the Organisation for Economic Co-operation and Development (OECD), the richest 10% in OECD countries now earn nearly ten times more than the poorest 10%, compared to seven times more in the 1980s. Wealth disparity is even more pronounced: in 2012, the top 10% controlled half of all household wealth, while the poorest 40% held just 3%. The effects of inequality are immediate for the poorest, but the entire economy suffers in the long run. When a large portion of the population benefits little from economic growth, trust in institutions weakens, and social stability is threatened. One major driver of rising inequality is technological change. In the short term, technology tends to benefit capital owners—those who can use it to reduce labor costs—and highly skilled workers, often at the expense of low-skilled workers. Inequality is not just a concern in developed countries; it is a growing problem worldwide. While developing countries have made progress in reducing poverty, many have seen rising income inequality. For example, in regions like China, India, and Indonesia, income inequality has worsened. Globally, the bottom 50% of income earners have captured only 12% of total economic growth, while the wealthiest 1% have taken 27%. In countries like the US and Western Europe, the middle class has seen minimal income growth, while the wealthiest continue to capture the largest share of economic gains. This imbalance is exacerbated by tax avoidance, with the wealthiest individuals hiding vast amounts of money offshore. The Tax Justice Network estimated in 2012 that at least $21 trillion was hidden in tax havens, reducing the effectiveness of redistributive tax systems and contributing to underfunded public services. In Italy, the wealth gap between the richest 1% and the poorest 90% has widened significantly over the past two decades. Since 1995, the share of wealth held by the richest 1% has risen from 17% to 21%, while the share held by the bottom 90% has dropped from 55% to 44%. Income inequality, as measured by the Gini index, shows a similar trend. After declining throughout much of the 20th century, inequality began to rise again in the 1980s and 1990s. This reversal coincided with changes in public policy and attitudes, leading to a widening income gap. Public perception of inequality is often far from the reality. In a survey in the US, respondents vastly underestimated the actual level of wealth inequality. They believed that the wealthiest 20% held about 59% of the wealth, when in fact, they controlled closer to 84%. Respondents also expressed a desire for a much more equitable distribution, suggesting the top 20% should hold only 32% of the wealth. In Italy, a 2018 survey conducted by Demopolis for Oxfam showed a strong public demand for action on inequality. About 80% of respondents viewed policies aimed at reducing inequality as a priority, signaling widespread concern about the growing divide between rich and poor. In summary, rising inequality, both globally and within individual countries, is a pressing issue with far-reaching consequences. Technological change, tax avoidance, and unequal economic growth have all contributed to this widening gap, creating a situation that calls for urgent attention and policy reform. PRINCIPLES OF EQUALITY Principles of Equality Equality is deeply tied to concepts of morality and justice, especially distributive justice. Since ancient times, equality has been seen as a key element of justice. Many movements throughout history have fought against inequality using the language of justice. However, philosophers have debated the role equality plays in a just society and have proposed different principles and interpretations of equality. Here, we will explore four such principles. Theprincipleofformalequalitystatesthatiftwopeopleareequalinarelevant way, they should be treated equally in that respect. This idea was first expressed by Aristotle in reference to Plato, with the notion of treating cases as alike. The key question here is deciding which aspects of people are relevant when determining equality. Aristotlealsointroducedproportionalequality,whichcontrastswithnumerical equality. Numerical equality means treating everyone exactly the same, giving each person an equal share of something, regardless of their individual circumstances. However, Aristotle argued that this is not always fair. Proportional equality, on the other hand, involves treating people in relation to what they deserve, giving more to those who are due more. For example, in a merit-based system, people are rewarded according to their efforts or contributions. While numerical equality may be just in some cases, proportional equality offers a more nuanced approach, ensuring that people receive what they are rightfully due. This principle can be applied in hierarchical systems like aristocracies or meritocracies, where rewards and punishments are based on people’s deserts or contributions. Historically,itwasbelievedthathumanswerenaturallyunequal.Thisideapersisted until the eighteenth century when the concept of moral equality emerged, suggesting that all human beings deserve equal dignity and respect. This principle shifted the notion of justice from merely giving each person their “due” to recognizing that all individuals, regardless of their differences, share equal moral worth. The concept of moral equality was first developed by the Stoics, who emphasized the equality of all rational beings. Christianity reinforced this idea by asserting that all people are equal before God. The notion of equality also spread through the Talmud philosophers like Hobbes, Locke, and Rousseau further developed the idea. Hobbes argued that in the state of nature, all people have equal rights because they all have the capacity to harm one another. Locke emphasized natural rights to ownership and freedom, while Rousseau claimed that social inequality emerged from the decline of natural equality, driven by human desires for property and perfection. In the Enlightenment period, Kant’s moral philosophy reinforced this notion of equality by advocating for universal human worth. His ideas of autonomy and freedom formed the foundation of modern human rights. This belief in equal dignity led to significant social movements, revolutions, and modern constitutions, including the French Revolution’s Declaration of the Rights of Man in 1789. Thefinalprincipleisthepresumptionofequality,whichistiedtotheideaof distributive justice. This principle suggests that goods and resources should be distributed equally unless there is a justified reason for unequal distribution. If inequality is proposed, it must be justified impartially, and the burden of proof lies on those who argue for unequal treatment. Certain factors, such as need, existing rights, performance, or compensation for discrimination, can be valid reasons for unequal distribution, but these must be carefully examined and justified. In conclusion, these principles reflect different ways of understanding and applying equality in society. From treating people alike in relevant respects to ensuring proportional fairness and recognizing the equal dignity of all individuals, these principles help guide discussions about justice and fairness in our world. THEORIES OF DISTRIBUTIVE JUSTICE Theories of Distributive Justice The principle of presumption of equality focuses on distributive justice, guiding how benefits and burdens should be shared among individuals in a society. There is a vast philosophical literature on this topic, as thinkers have long sought to define what constitutes a fair distribution of advantages. Today, distributive justice mainly concerns how economic benefits and burdens—such as wealth, resources, and opportunities—should be allocated among people. The Importance of Distributive Justice Throughout much of history, people were born into fixed economic positions, with wealth and status seen as determined by nature or divine will. However, with the realization that governments could shape economic distribution through laws and policies, distributive justice became an essential issue. In modern societies, every policy—whether related to taxes, education, or healthcare—affects how benefits and burdens are distributed. This makes distributive justice a constant and urgent consideration. At any point, societies must decide whether to maintain their current systems or to modify them, and theories of distributive justice offer moral guidance for these decisions. Key Questions in Distributive Justice Theories of distributive justice often address a few fundamental questions: Who should be considered equal? (subject) When should equality be applied? (time) Why does equality matter? (justification) What should be equal? (metric) And how should goods be distributed? (pattern) Equality Among Whom? Justice is generally thought to apply to individuals, with each person bearing responsibility for their actions. However, some debates focus on whether equality should also be considered at the group level. For example, women, racial minorities, and other groups often raise concerns about inequalities between their group and the rest of society. The question then becomes whether these group-based inequalities are inherently unjust, or if we should focus instead on how individuals within those groups fare compared to others. Additionally, there is the question of whether distributive equality should apply only within a nation or extend globally. Most theories focus on equality within a single society, but universal principles of equality suggest that all people, regardless of nationality, should be treated with equal respect. A related question is whether the principles of distributive justice apply globally or only within specific states and nations. Some argue that there is no reason why people from different countries should be excluded from the fair distribution of goods, especially in cases involving natural resources like oil. Why, for instance, should a valuable resource belong solely to the person who finds it or to the country where it is located? However, many believe that extending distributive justice on a global scale would place too heavy a burden on individuals and their states. Others suggest that special bonds between members of the same nation, such as shared culture and values, justify a focus on local rather than global equality. Another important issue is the relationship between generations. Does the current generation have an obligation to ensure future generations enjoy equal living conditions? One argument in favor of this view is that people should not end up worse off due to factors beyond their control, such as being born into a particular time period. However, the question of justice between generations is complex, as it involves weighing the needs and rights of both present and future people. In summary, distributive justice theories address crucial questions about how resources and opportunities should be shared. These theories help societies make moral decisions about fairness, ensuring that benefits and burdens are distributed in a way that respects the equality and dignity of all individuals. Equality and Timing The timing of when equality should be achieved plays a key role in distributive justice. One approach is the starting-gate principle, which suggests that everyone should have equal access to resources at the beginning, after which they are free to use those resources as they see fit. The outcomes that follow will naturally be unequal, but this approach only guarantees fairness at the starting point. However, since this method can lead to large inequalities over time, some argue that equality should be maintained throughout different time-frames. For instance, income could be kept equal at regular intervals, but even this approach may allow for wealth disparities if people are permitted to save differently. This leads to the idea that principles of equality often need additional specifications, such as guidelines on saving behaviors, to avoid deep inequalities. Why Does Equality Matter? The question of why equality is important has been explored through different philosophical perspectives. Oneview,knownasintrinsicegalitarianism,arguesthatequalityisvaluablein itself. From this perspective, it is inherently wrong if some people are worse off than others through no fault of their own, and equality should be pursued even if it does not directly benefit anyone. However, this idea faces a challenge known as the levelling-down objection. It questions whether equality is truly desirable if achieving it would mean making everyone worse off. For example, it would be morally troubling to render sighted people blind just to create equality with those who cannot see. Toavoidsuchextremescenarios,pluralisticegalitarianismcombinesthepursuit of equality with other important values, such as improving overall well-being. Instead of levelling everyone down, pluralistic egalitarians argue that those who are better off should help those who are worse off, thus promoting both equality and welfare. Anotherperspectiveisinstrumentalegalitarianism,whichvaluesequalityforthe positive outcomes it can produce. For example, redistributing wealth from the rich to the poor helps reduce poverty, promoting economic growth and social stability. In this view, equality is not the ultimate goal, but rather a tool for achieving broader societal benefits. A more equal distribution of resources can help ensure that everyone has access to education and healthcare, which in turn benefits the economy and reduces the risk of social unrest. However, this view allows for some level of inequality, as long as it does not harm overall economic or social stability. Finally,thereisconstitutiveegalitarianism,whichseesequalityasafundamental part of a larger framework of justice. In this view, equality is not merely a tool for achieving other goals but is an essential component of a just society. Justice itself is intrinsically valuable, and part of being just involves ensuring that everyone has an equal claim to certain goods. What Should Be Equal? One key question in distributive justice is what exactly should be made equal. There are various approaches to this, each with its own focus and reasoning. Astraightforwardapproachistofocusonincome,asitisaversatilemeasureofhow well people are doing in contemporary market economies. Income gives individuals access to a wide range of goods and services, making it a useful metric for assessing equality. Using income as a measure simplifies many problems by allowing people to decide for themselves how to use their resources. It also makes it easier for governments to implement and monitor distributive policies. However, one challenge is that people vary in their ability to convert income into well-being. Some may need more resources to achieve the same level of well-being as others due to personal circumstances like disability. Therefore, treating people fairly might require giving some individuals more to ensure they have equal opportunities to thrive. Anotherapproachfocusesonequalopportunities,aviewsupportedbyluck egalitarians. They argue that people should have equal chances to succeed in life, and compensation should be provided for misfortunes that are beyond an individual’s control. Luck egalitarians hold that people should be held responsible for their choices and actions, but not for the circumstances they cannot control. According to this view, inequalities that arise from personal decisions are just, while those caused by luck or accidents are not. For instance, if someone becomes wealthy through their own efforts, that inequality might be considered fair. However, if someone is disadvantaged due to factors like race, gender, or family background—things beyond their control—then society has a responsibility to compensate them to some extent. The idea of equal opportunities also raises the question of formal versus substantial equality. Formal equality of opportunity means that everyone should have the same legal rights, free from discrimination based on race, gender, or other uncontrollable traits. However, even in societies with formal equality, many inequalities persist due to factors such as family background, access to quality education, or healthcare. This has led to the argument for a more substantial form of equality of opportunities, where individuals have equal access to essential services like education and healthcare. Such a society would ensure that these factors, over which people have no control, do not limit their potential. However, even this more substantial form of equality may not completely eliminate inequality, as people are also born into differing social environments that shape their prospects in life. Taking this reasoning further, radical equality of opportunities highlights that many social and natural factors still affect individuals’ prospects. People are born into families and neighborhoods that may or may not support their educational and economic growth. In addition, people are more or less fortunate in terms of their natural talents and abilities. These differences are often a matter of luck rather than personal effort. A society that allows these arbitrary factors to determine people’s life chances can be criticized as unfair. This is often illustrated using the metaphor of a race: if some participants start ahead due to luck, the race is not fair. Similarly, if society is structured so that people’s prospects are largely determined by factors beyond their control, it can be seen as unjust. Somephilosophers,knownaswelfarists,arguethatwhatshouldbeequalis well-being, rather than income or opportunities. Historically, utilitarians have defined well-being in terms of pleasure, happiness, or preference satisfaction. Jeremy Bentham, a key figure in utilitarianism, argued that pleasure is the only thing of intrinsic value. His successor, John Stuart Mill, expanded this idea to include happiness and fulfillment. In more recent times, philosophers like Kenneth Arrow have focused on preference-satisfaction, which means that well-being is about having one’s preferences or desires met. According to this view, a just distribution of resources would be one that maximizes the satisfaction of people’s preferences, taking into account the intensity of those preferences. However, utilitarian approaches to well-being have been criticized. One well-known critique, put forward by John Rawls, argues that utilitarianism fails to respect the individuality of persons. Utilitarianism may justify making some people suffer for the greater good, as long as it leads to a net benefit for society. However, Rawls and others argue that this is morally wrong, because it treats individuals as mere parts of a larger system rather than respecting them as individuals with their own rights. For instance, it might be acceptable for a person to choose to suffer in the short term to improve their overall well-being, but it is problematic to force someone to suffer for the benefit of others without their consent. A second critique concerns preference satisfaction. In classical utilitarian theories, all preferences are treated equally, even if they are harmful or discriminatory. For example, if a majority of people hold racist preferences, utilitarianism could, in theory, justify unequal treatment of a minority if that satisfies the preferences of the majority. This raises moral concerns about whether all preferences should count equally in determining the best distribution of resources. In conclusion, different approaches to distributive justice emphasize various aspects of equality—whether it’s income, opportunities, or well-being. Each approach has its strengths and challenges, and the debate continues about what should be made equal in a just society. How should goods be distributed? The question of how resources should be distributed in society has been explored through various theories, each offering different answers. These approaches range from focusing on ensuring a minimum standard for everyone to promoting equality or fairness based on opportunity. Sufficientarianismisoneoftheleastdemandingviewsofjustice.Itarguesthat society’s primary concern should be to ensure that everyone has enough to lead a decent life. Once individuals have reached this threshold, there is no further need to worry about the relative distribution of goods among people. Philosopher Harry Frankfurt, in his book Equality as a Moral Ideal (1987), contends that the focus on equality distracts from more important issues. For him, once everyone has enough, it is irrelevant whether some have more than others. However,thisviewfacescriticism,particularlythroughwhatisknownasthe Indifference Objection. Sufficientarianism can allow for vast inequalities to exist, as long as everyone has enough. It does not address disparities in wealth or power that arise from factors such as natural talent or social background, which may still be seen as unjust. Strictegalitarianismholdsthateveryoneshouldreceiveanequalshareofmaterial goods and resources. This idea has been largely rejected as unrealistic and undesirable, as it fails to account for differences in individual needs, desires, and efforts. Even Marx, in his Critique of the Gotha Program (1875), argued against strict economic equality. He criticized theories that focus solely on distribution while neglecting the underlying structures of production. Additionally, critics argue that strict equality can undermine incentives for productivity and lead to wasteful inefficiencies. Another concern is that enforcing strict equality could result in a loss of diversity and the imposition of uniformity, which threatens values like pluralism and democracy. AmoreinfluentialtheoryofdistributivejusticecomesfromJohnRawlsinhisbook A Theory of Justice (1971). Rawls introduces the concept of the veil of ignorance, where individuals design principles of justice without knowing their own social status, class, or natural abilities. This ensures that the principles chosen are fair to everyone, as no one can tailor them to their own advantage. From this starting point, Rawls proposes two key principles of justice. 1. 2. The first ensures that everyone has equal basic rights and liberties. The second principle, which deals with economic and social inequalities, is divided into two parts: (a) inequalities must be attached to positions that are open to all under fair conditions of opportunity. (b) inequalities are only acceptable if they benefit the least advantaged members of society. This latter part is known as the Difference Principle. The Difference Principle allows for some inequality, but only if it improves the position of those who are worst off. Rawls argues that this would be a rational choice for individuals in the original position because it protects them from the worst possible outcome. This approach is also known as the maximin principle, as it seeks to maximize the welfare of those at the minimum level of society. ThoughRawls’DifferencePrincipleallowsforinequality,itdoessowiththe belief that these inequalities can benefit everyone, especially the worst off. For example, if certain inequalities incentivize talented individuals to be more productive, the overall wealth of society increases, and this growth can be used to improve the situation of the least advantaged. In this way, Rawls’ theory tolerates a degree of inequality, as long as it serves the greater good, particularly for those who are worse off. In conclusion, these different approaches to distributive justice explore how goods should be shared in society. Whether focusing on ensuring a minimum standard, achieving strict equality, or allowing for some inequality if it benefits everyone, each theory offers insights into what a just society should look like. AI AND WORK AI plays a significant role in increasing global inequalities, particularly in the world of worThe causes of this process are complex. One important driver of rising inequality is technological change. How can AI contribute to this phenomenon? In several ways. One of them is the impact of AI in the world of work. ➔ AIFORRECRUITMENT:AIisusedtoscreenresumesandassesscandidates, improving efficiency but potentially reinforcing biases. If not carefully managed, AI can perpetuate discrimination in hiring processes. ➔ AIANDTHELABOURMARKET:AIisautomatinglow-skilledjobs,leadingtojob displacement and growing the divide between high- and low-skilled workers. This shift increases job insecurity, particularly in the gig economy, where workers face fewer protections. ➔ BUILDING,MAINTAINING,ANDTESTINGAISYSTEMS:AIiscreatingnew jobs in specialized fields, but these high-paying roles are accessible only to those with advanced skills, widening the gap between skilled and unskilled workers. ➔ AIATTHEWORKPLACE:AItoolsincreaseefficiencybutcanleadtoexcessive worker surveillance and reduced autonomy, especially affecting lower-level employees. AI FOR RECRUITMENT Strategeion was founded by a group of enthusiastic Army veterans who were skilled in programming and computer engineering. After their honorable discharge, they launched this non-profit organization driven by a sense of civic duty. The platform they developed aimed to provide a range of public services, with a focus on veterans, though it was open to other groups as well. Their motto, “leave no one behind,” reflected their commitment to inclusivity. In contrast to other tech companies that typically hire young graduates from elite universities, Strategeion primarily employed ex-military personnel. This hiring policy led to high employee satisfaction and retention, making Strategeion stand out in the public eye. The organization’s visibility increased, and job applications began to flood in, including many from candidates who would typically seek positions at larger, for-profit tech firms. The Efficiency Drive: PARiS System As the number of job applications overwhelmed the HR team, Strategeion’s developers introduced an AI-based system called PARiS to streamline the hiring process. PARiS used natural language processing (NLP) and machine learning to analyze resumes and identify the best candidates. The system was trained using resumes of current and past employees who were classified as either exemplary or poor based on their professional attributes and fit within the company. PARiS would then rate incoming resumes and reject those that didn’t meet a certain threshold. As HR developed increasing trust in PARiS, they relied less on manual checks, allowing the system to make decisions autonomously. Question 1: Efficiency vs. Other Values in Hiring PARiS promised to make the hiring process more efficient. But are there other values that might be desirable in hiring? Diversity? Equity? Creativity? What, if anything, do companies risk losing when hiring procedures are so singularly focused on maximizing efficiency? While PARiS improved hiring efficiency, it overlooked other important values, such as diversity, equity, and creativity. By singularly focusing on efficiency, companies risk filtering out candidates who might bring new and valuable perspectives. A system like PARiS may unintentionally favor candidates who fit established patterns, reinforcing homogeneity and stifling innovation. Diversity is crucial for fostering creativity and ensuring a variety of viewpoints, which can lead to more innovative solutions and better decision-making. By focusing solely on efficiency, Strategeion risked missing out on the benefits that come with a more diverse workforce, such as higher adaptability and enhanced problem-solving abilities. Hara’s Case: Rejection and Bias Hara, a bright computer science student from Athens, applied to Strategeion and was promptly rejected by the PARiS system. Despite her strong qualifications and civic work with non-profit organizations supporting wheelchair users like herself, her application was dismissed without human review. Confused, Hara contacted the company for feedback. The HR department, after reviewing her resume, was puzzled by the rejection. Hara’s skills and interests aligned well with the company’s values and objectives. Upon investigating further, HR discovered that PARiS had flagged her application as a poor fit based on an unexpected factor: sports. Many of Strategeion’s employees, being veterans, had a history of participating in athletics, which PARiS had correlated with good job performance. Hara, who had used a wheelchair her entire life, had no sports history, leading to her unfair rejection. Question 2: Addressing Bias in AI Systems Biased data sets pose a problem for ensuring fairness in AI systems. What could Stra- tegeion’s engineers have done to counteract the skewed employee data? To what extent are such efforts the responsibility of individual engineers or engineering teams? PARiS’s biased decision-making stemmed from a skewed training dataset, which overemphasized characteristics like athletic participation. To prevent this kind of bias, Strategeion’s engineers should have diversified the training data to include a broader range of experiences, ensuring that the system didn’t unfairly favor irrelevant traits like sports. Regular audits and updates to the system could help catch and correct biases as they arise. This responsibility falls not only on individual engineers but on the entire development team, HR department, and the company leadership, all of whom must work together to ensure that the AI system operates fairly and without discrimination. Hara’s Complaint: Fairness, Dehumanization, and Consent After discovering why she had been rejected, Hara received an apology from Strategeion and was invited to interview. However, she declined and filed a formal complaint, raising concerns about fairness, the dehumanizing nature of automated decision-making, and the lack of consent regarding the use of her data. Hara argued that PARiS had treated her unfairly by rejecting her based on an irrelevant factor—her lack of sports participation. Given the marginalization that people with disabilities often face, Hara felt that Strategeion should have taken steps to actively support disabled candidates rather than allowing the system to inadvertently disadvantage them. She also criticized the use of an automated system to make such important decisions without human oversight. This process, she argued, felt dehumanizing, reducing her to a data point rather than considering her full potential. Hara suggested that this dehumanization might extend to the HR workers whose roles had been diminished by the AI system. Finally, Hara was dismayed to learn that her resume, and those of other applicants, may have been used to train the system without her explicit consent. Many current and former employees shared her concern, feeling that they had been subjected to decisions made by AI without their knowledge or agreement. Question 3: Addressing Insidious Discrimination The type of discrimination practiced by PARiS might not seem as blatantly demeaning as a blanket hiring policy against those with physical disabilities, but it is any different from a moral standpoint? How might this kind of insidious discrimination, which is, by definition, difficult to spot, be avoided? PARiS did not explicitly discriminate against Hara based on her disability, but it still indirectly excluded her due to its focus on athletic history. This type of discrimination is subtle and harder to detect but still morally problematic. While it may seem less demeaning than outright exclusion based on disability, it perpetuates inequality in a more covert way. Avoiding this form of discrimination requires careful design, continuous monitoring, and transparency in how AI systems are trained and applied. It’s essential to ensure that AI systems are regularly checked for any patterns that may lead to unintended exclusions, especially for marginalized groups. Rethinking Workforce Homogeneity Strategeion’s founders began reconsidering their emphasis on a homogeneous workforce of veterans. Studies in management suggest that diverse teams outperform homogeneous ones, as they bring a variety of perspectives and are better equipped to innovate. Diversity helps prevent “group think” and fosters a more inclusive and creative work environment. However, homogeneity also has advantages, such as smoother communication and a shared understanding that can reduce internal conflicts. Question 4: Balancing Homogeneity and Diversity Social science increasingly shows that there are advantages to a heterogenous work- force, but there are also advantages to homogeneity. A diverse workforce helps protect organizations against “group think,” for example, but groups that share certain expe- riences and backgrounds may find it easier to communicate with and understand one another, thereby reducing collective action problems. If you were a manager in charge of hiring at Strategeion, for which position would you advocate? Would you try to maintain the corporate culture by hiring people who resemble current employees, or would you argue that PARiS should be realigned to optimize for a broader range of types? If I were a manager at Strategeion, I would advocate for hiring a more diverse workforce. While shared experiences can create a strong corporate culture, diversity brings a wider range of ideas, perspectives, and solutions that can enhance problem-solving and drive innovation. Reconfiguring PARiS to prioritize diversity would not only enrich the company’s work environment but also align with the company’s commitment to inclusivity. This would ensure that Strategeion remains adaptable and better equipped to face future challenges while maintaining its foundational values of leaving no one behind. History of hiring technology The evolution of hiring technology has closely followed advancements in the internet. In the 1990s, online job boards like Monster.com emerged, offering digital job listings at a much lower cost than traditional newspaper ads. Soon after, search engines were developed to help users find these job postings, and pay-per-click advertising gave recruiters a new way to compete for attention. At the same time, it became easier for job seekers to apply for multiple jobs online. Recruiters also started using digital tools to actively seek out candidates by scanning public profiles, such as LinkedIn. This shift allowed recruiters to target not only active job seekers but also passive candidates who might not be actively looking for a job. As the number of job applicants grew, employers began to adopt new screening methods to manage the increased volume. While tests and assessments had long been used to evaluate candidates, technological advancements enabled the use of more sophisticated assessment tools that could analyze large amounts of data more effectively. Recently, diversity and inclusion have become key focuses in hiring, prompting the development of tools aimed at reducing bias in the recruitment process. Many of today’s hiring technologies incorporate predictive features that use machine learning to analyze data and make forecasts about candidates, such as predicting their potential performance or ranking them based on various criteria. Employers adopt these predictive tools for several reasons. 1. First, they want to reduce the time it takes to fill open positions, as delays can divert resources and lead to the loss of top candidates to competitors. Companies with seasonal staffing needs also benefit from quicker hires to meet critical time frames. 2. Second, employers aim to reduce the cost per hire, which in the U.S. averages about $4,000. Reducing these costs allows companies to allocate their resources more efficiently. 3. Third, predictive tools help improve the quality of hires by assessing factors like job performance, potential for promotion, and overall fit with the company. Employers also seek to minimize turnover, as high turnover rates are costly due to the need to hire and train replacements. 4. Lastly, many employers use these tools to meet diversity goals, ensuring a more inclusive workplace by considering candidates’ gender, race, age, religion, disability, veteran status, or socioeconomic background. Overall, hiring technology continues to evolve, offering more efficient, cost-effective, and inclusive recruitment solutions for employers. Discrimination and bias Hiring technology vendors often promote their tools as a way to reduce bias and make the hiring process more fair and efficient. They claim that these tools can help companies make more consistent, unbiased decisions by removing sensitive information about applicants, such as race or gender, from the hiring process. This approach primarily targets what is known as direct or interpersonal discrimination, which occurs when individuals are treated unfairly because of their protected characteristics—traits like race, gender, or disability that are legally protected from discrimination under laws like the Fair Housing Act and the Equal Credit Opportunity Act. However, direct discrimination is only one form of bias. Another is indirect or systemic discrimination, which occurs at an institutional level. This happens when a company’s policies or workplace culture unintentionally favor certain groups while disadvantaged others. For example, if a company hires only from a narrow pool of people with similar backgrounds and uses “culture fit” as a hiring criterion, it may unintentionally reject qualified candidates from more diverse backgrounds. Hiring practices can also contribute to structural discrimination, which stems from long standing social inequalities, such as racism or unequal access to education. For instance, some employers place high value on elite university degrees, but these degrees are often more accessible to privileged individuals, leaving others at a disadvantage. Additionally, discrimination can be internalized by job seekers themselves, influencing their decisions, such as whether to apply for a job at all. Even though hiring tools aim to be fair, they can still perpetuate bias. Bias in these tools often arises from the data used to train them. Since most AI systems rely on data, if the data used contains biases, the algorithm will learn and replicate these biases. This can lead to biased outcomes, and in some cases, algorithms can even amplify the biases present in the data. Design choices in the algorithms themselves can also introduce bias, even if the original data was unbiased. These biased outcomes can create a feedback loop. When biased decisions are made, they affect real-world outcomes, which then provide more biased data for future training. This cycle can lead to increasing levels of bias over time. A well-known example of this is Amazon’s experimental hiring tool, which used AI to rate candidates for technical jobs. The system was trained on resumes submitted over a 10-year period, most of which came from men due to the male dominance in the tech industry. As a result, the tool learned to favor male candidates, penalizing resumes that mentioned women’s organizations or colleges. This example illustrates how biased data can lead to biased outcomes, even in systems designed to be objective. In summary, while hiring technology aims to eliminate bias, it can still perpetuate both direct and systemic discrimination if not carefully designed and monitored. Bias in data and algorithms remains a significant challenge, requiring ongoing efforts to ensure fairness in the hiring process. Bias in machine learning can take many forms and typically falls into three main categories: data-to-algorithm bias, algorithm-to-user bias, and user-to-data bias. These types of bias occur within the data, algorithm, and user interaction loop, impacting the fairness and accuracy of outcomes. 1. Data-to-Algorithm Bias This type of bias occurs when the data used to train an algorithm contains biases, leading the algorithm to produce biased results. There are several forms of data-to-algorithm bias: Omitted-variable bias: This happens when an important factor is left out of the model. For example, if a model is predicting subscription cancellations without accounting for the arrival of a new, cheaper competitor, the missing variable (the competitor) leads to inaccurate predictions. Representation bias: This arises when the data collected does not fairly represent the population. For instance, if a dataset like ImageNet is heavily populated by images from Western countries like the US and the UK, it will lead to bias toward Western cultures in the algorithm’s predictions. Aggregation bias: This occurs when conclusions about individuals are drawn from aggregated data, ignoring subgroup differences. An example is the Simpson’s paradox, where overall admissions at UC Berkeley appeared biased against women. However, when analyzed by department, women had equal or better chances of admission. The bias came from the fact that women tended to apply to departments with lower overall admission rates. 2. Algorithm-to-User Bias This type of bias results from how algorithms influence user behavior through their outputs. Algorithmic bias: Sometimes, the bias doesn’t come from the data but from the algorithm itself. Choices made during the algorithm’s design—such as which optimization function or statistical estimator to use—can lead to biased outcomes even if the data is unbiased. Presentation bias: This occurs when the way information is presented affects user behavior. For example, on the web, users can only click on content they see, which means that content shown to them will get more clicks, while other content remains unseen, even if it’s relevant. Popularity bias: This type of bias happens when popular items are given more exposure, regardless of quality. For example, search engines or recommendation systems might show certain items more frequently simply because they’re already popular, which can be further manipulated by fake reviews or social bots. 3. User-to-Data Bias This bias emerges when user-generated data used for training machine learning models reflects the biases of the users themselves. Historical bias: This bias stems from societal or historical inequalities that already exist in the world. For example, a 2018 image search for “women CEOs” showed fewer images of female CEOs because only 5% of Fortune 500 CEOs were women, causing search results to skew towards men. Social bias: Social bias occurs when our judgments are influenced by the opinions or actions of others. For example, if a user wants to give a low rating to a product but sees many high ratings, they might raise their score, thinking their original judgment was too harsh. In summary, bias in machine learning can come from the data used to train models, how algorithms are designed, or even how users interact with these systems. Understanding these types of bias helps in developing more fair and accurate AI systems. Algorithmic fairness Addressing fairness in AI recruitment involves tackling bias and discrimination, an issue long debated in philosophy and psychology, and now pressing in machine learning. To approach fairness in AI, a fundamental question arises: how do we define it? The lack of a universal definition reflects the complexity of fairness as a concept. However, fairness generally refers to the absence of prejudice or favoritism toward individuals or groups based on their inherent or acquired characteristics within decision-making contexts. In recruitment AI, fairness is often defined in terms of individual and group treatment. Individual fairness aims to give similar predictions to similar individuals, while group fairness ensures that different groups are treated equitably. 1. Individual Fairness through Unawareness removes any data likely to introduce bias, such as excluding factors like ethnic origin. For instance, in training algorithms for parole decisions, data on the number of previous offenses might seem objective but can still reflect historical biases in policing. By omitting such attributes, we reduce bias in some respects, but we also lose the ability to verify equal outcomes or opportunities. This approach has been adopted in some countries, such as Germany, where demographic data is excluded to prevent discrimination. 2. Individual Fairness through Awareness suggests that similar individuals should receive similar predictions. For instance, in job advertisements, if two individuals differ only in sexual orientation, they should ideally see the same job listings. Yet defining “similarity” is challenging. Biases in training data, such as higher reported discrimination in hiring among minorities, complicate any attempt to ensure fairness by similarity alone. When considering group fairness, we shift focus to treating groups equally. This type of fairness is often pursued through demographic parity and equal opportunity. Demographic(Statistical)Parityimpliesthat,onaverage,analgorithmshould yield the same results for different groups. For example, if approving loans, demographic parity would mean that males and females have the same likelihood of approval. In practice, demographic parity is often softened, such as the U.S. Equal Employment Opportunity Commission’s 80% rule, which allows some imbalance but insists that acceptance rates for different groups not fall below 80% of the highest-accepted group. Critics argue that demographic parity can sometimes misrepresent fairness; for instance, aiming for equal arrest rates in violent crimes across genders would ignore meaningful differences in crime rates. EqualOpportunitypromotesfairoutcomesbyensuringthataperson’slikelihood of receiving a beneficial prediction is the same across groups. In loan applications, for instance, equal opportunity would require that individuals with disabilities who have repaid past loans receive similar approval rates as those without disabilities who have done the same. However, broader societal biases may still influence outcomes indirectly—for instance, if individuals with disabilities face job discrimination, they may struggle more to repay loans, affecting their treatment under this standard. Thus, each fairness approach addresses bias from different angles, showing the nuanced challenges of balancing equitable outcomes with accurate predictions. The hiring process is a funnel, involving a series of steps that guide a candidate from application to job offer or rejection. It starts with sourcing, where employers attract potential candidates through ads, job postings, and outreach efforts. Predictive tools help optimize this by placing job ads strategically and identifying candidates who may be interested or even ready to re-enter the job market. These sourcing technologies can shape the candidate pool early on, even before applications reach recruiters. After sourcing, matching comes into play. This step involves comparing job opportunities with possible candidates, creating ranked lists of recommended matches. Both job seekers and recruiters benefit from these technologies, which personalize job suggestions and connect the right candidates to the right jobs. For instance, ZipRecruiter exemplifies this approach, using recommendation algorithms similar to those in Netflix or Amazon. It tailors job recommendations based on user behavior and preferences, enhancing both employers’ and jobseekers’ experiences by increasing visibility for likely good matches. ZipRecruiter and similar platforms typically use two methods for personalized recommendations: content-based filtering and collaborative filtering. Content-basedfilteringhighlightsjobsthatusershavepreviouslyshowninterestin by analyzing their clicks and other actions. Collaborativefiltering,ontheotherhand,looksatsimilarusers’behaviorstomake recommendations. If two jobseekers apply to similar jobs, the system may suggest jobs to one based on the positive response of the other. However, these algorithms present challenges, especially regarding equity. Content-basedfilteringmayunintentionallylimitusersbyreinforcingtheirexisting preferences. For instance, if a woman doubts her qualifications and clicks on lower-level jobs, she might eventually see fewer high-paying jobs that match her skills. Collaborativefilteringcanalsocreatebiasesbystereotypingusersbasedonothers’ behaviors. Even if a woman frequently clicks on management positions, the system may recommend fewer senior roles if it observes that other women with similar profiles click on junior roles. Such biases underline the importance of ensuring that job-matching systems promote, rather than hinder, fair hiring practices. In the screening stage of hiring, employers start formally reviewing applications, quickly narrowing down the pool by identifying unqualified candidates and ranking those who seem promising. Predictive tools help by scoring and ranking applicants based on their skills, experience, and even soft skills, allowing hiring managers to focus on top candidates. This automated process often results in a large number of applicants being filtered out early on. A notable example in screening technology is Pymetrics, a company that uses neuroscience-based games to evaluate cognitive, social, and emotional traits in candidates. These games, such as one where players click for specific visual cues, measure traits like impulsivity, attention span, and adaptability. Pymetrics customizes its models for each employer by having current employees play the games. Using machine learning, Pymetrics then identifies the traits common to top performers and incorporates these insights into their predictive model, which assigns a fit score to each candidate based on gameplay. However, tools like Pymetrics raise concerns around fairness and bias. The model’s foundation relies on identifying “top performers,” often based on subjective criteria, which can introduce discrimination. Traits identified in high-performing employees may inadvertently favor certain social groups or reflect biases in past hiring decisions. Even when models work accurately, they may unintentionally screen out capable individuals who don’t align with the identified traits, despite being equally qualified. Furthermore, Pymetrics and similar tools often reflect particular psychological theories that might not be universally applicable. Many psychological studies traditionally use college students as subjects, leading to questions about whether findings truly apply to diverse groups. Additionally, assigning specific numerical scores to candidates can make small differences appear more significant than they are, creating an impression of distinction where there may be minimal real difference. In the interviewing stage, employers engage directly with applicants, often using tools that analyze video interviews to assess candidates’ responses, tone, and even facial expressions. These tools aim to streamline interviews, saving time and making the hiring process more consistent. For example, HireVue enables employers to collect recorded interview responses from candidates, which are then “graded” by comparing them to responses from current successful employees. By analyzing various factors like eye contact, facial expressions, enthusiasm in tone, and word choice, HireVue’s model produces an “insight score” from 0 to 100. Candidates with higher scores can automatically progress to the next stage, while those below a threshold might be filtered out. Although HireVue tests its models for potential biases based on demographic factors like gender, race, and age, concerns remain. Speech recognition software can struggle with regional or nonnative accents, and facial analysis often performs inconsistently with darker skin tones. These issues are not just about technical limitations but raise ethical questions: can physical cues like facial expressions or tone of voice reliably indicate job performance? Some critics argue that such criteria may favor exaggerated expressions or penalize those with visible disabilities or speech impediments, potentially creating an unfair disadvantage. At the selection stage, employers make their final decisions, which may involve background checks and negotiating offer terms. Here, predictive tools can help employers estimate a candidate’s likelihood of accepting an offer, as well as optimize the offer by adjusting salary, bonuses, and benefits. For example, Oracle’s Recruiting Cloud provides real-time predictions about a candidate’s acceptance probability and updates these predictions based on past offers and outcomes. While these tools provide insight into negotiation, they can also deepen pay gaps, particularly for women and underrepresented groups. Salary predictions often use data that might inadvertently act as proxies for socioeconomic or racial status, potentially perpetuating pay disparities. Furthermore, laws preventing employers from inquiring about past salaries aim to address these gaps, but predictive tools that estimate salary needs based on historical data could undermine these protections, reinforcing existing inequities in compensation. In each stage of the hiring funnel, from interviews to final selection, AI tools offer efficiencies but also introduce complex ethical challenges around fairness, bias, and equity.