Decision Making in Management (MGMT 4023) PDF
Document Details
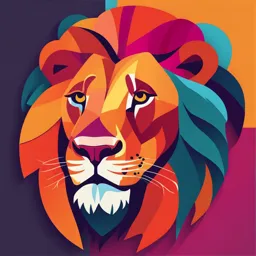
Uploaded by Unibeltol
University of Belize
Dr. Romaldo Isaac Lewis (DBA)
Tags
Summary
This University of Belize document provides an overview of decision-making models, including various data forecasting techniques. It covers topics such as rational models, qualitative methods, and data analysis.
Full Transcript
UNIVERSITY OF BELIZE FACULTY OF MANAGEMENT & SOCIAL SCIENCE COURSE: Decision Making in Management (MGMT 4023) LECTURER: Dr. Romaldo Isaac Lewis (DBA) Chapter No.2; Effective Management Decision Making: OBJECTIVES After studying this chapter, you should be able to understand: 1. Conclude the final po...
UNIVERSITY OF BELIZE FACULTY OF MANAGEMENT & SOCIAL SCIENCE COURSE: Decision Making in Management (MGMT 4023) LECTURER: Dr. Romaldo Isaac Lewis (DBA) Chapter No.2; Effective Management Decision Making: OBJECTIVES After studying this chapter, you should be able to understand: 1. Conclude the final portion of the RAT module that encompasses the end of decision making. 2. Learn other model of decision making spectrum via data forecasting. 3. Understand why analyst should be subjective in choosing how to model with that data. 2.1) Developing rational models with qualitative methods and analysis: Data forecasting? When you come across the phrase – data forecasting – from a decision making perspective, it usually refers to time series data (or other sequentially presented and gathered data). Within this data may also be other influences (such as a recurring trend or a seasonality influence). Clearly, the conditions which generated that data (whether as sales by an organisation over a 2-year timeframe or the rate of change of innovations in a given product for example), are important in our confidence that whatever model we develop, will be robust and a reliable guide to future data from that context. Within our analyses therefore we must also be concerned with the reliability, validity and verifiability of our forecasts, which requires a consideration of the stability and longevity of the assumptions we made about that context. Hyndman (2009:1) describes the role and function of forecasting as. “Forecasting should be an integral part of the decision-making activities of management, as it can play an important role in many areas of a company. Modern organisations require short-medium- and long-term forecasts, depending on the specific application. Short-term forecasts are needed for scheduling of personnel, production and transportation. As part of the scheduling process, forecasts of demand are often also required. Medium-term forecasts are needed to determine future resource requirements in order to purchase raw materials, hire personnel, or buy machinery and equipment. Long-term forecasts are used in strategic planning. Such decisions must take account of market opportunities, environmental factors and internal resources”. In general, the methods presented in this chapter are focused upon short and medium term forecasting for managers and moreover this text adopts the view of using projective forecasting for short term analyses and causal forecasting for medium term analyses (these terms are discussed shortly). Longer horizon forecasting is outlined in chapter 7. However, data forecasting is not just restricted to developing quantitative models (see chapter 3 for a further narrative on modelling), which might naturally be assumed. Data forecasting can be both qualitative and quantitative. In the case of the former, it can be interpretivist and subjectivist. This includes decision making methods such as the study of heuristics (strategic, biological and behavioural decision making), variations on Delphi Decision Making and other futures analyses (such as FAR (Field Anomaly Relaxation) from studies of strategy, market research methods, cross impact analyses and historical analogy (to name a few)). 1 Returning now though to the quantitative focus on data forecasting, we can differentiate between projective methods of data forecasting (which are concerned with short term forecasts of the order of a few days or a couple of weeks (for example, the restocking decisions of independent small grocery / convenience stores)) and causal forecasting (or explanatory forecasting) which is concerned with longer future forecasting and which rather than rely upon the absolute data to guide future decisions, is focused upon the relationships between the absolute data, which can be argued to more robust and stable. In this chapter, we explore varying data forecasting methods, from simple averaging, through data smoothing methods, linear and non-linear regression and data decomposition. Multiple regression (with multiple (non related) independent variables) will be presented in outline, although effective solutions to such problems are more easily undertaken by using appropriate software. 2.2) Simple Averaging Forecasting? Time series data is typically sourced and presented in a chronological order. If the units of the progression are unclear, then they may have to be transformed into a more appropriate format. In using such data to predict future trends and future data, key questions to consider in their interpretation are whether such data would be representative of all trends in that data, whether the model chosen to forecast future data will be also be able to reflect short term preferences and whether the environment is stable (and to what extent) to support future forecasts. Forecasting methods are generally understood as comprising three generic types of modelling: 1) Smoothing – projective forecasting based upon the most recent historical data, to predict short term future data. Typical methods of decision making include simple averaging, moving averages and one variable exponential smoothing. 2) Trend analysis – can be projective and/or causal forecasting which considers both the recent historic data and immediate future forecast, to generate the next future forecasts and modelling. Typical methods of decision making include two variable exponential smoothing and trend smoothing. 3) Trend analysis with seasonal and/or cyclical influence – is usually focused upon classical data decomposition and can encompass both linear changes in data and non-linear changes in change, to generate complex forecasting models. 2 The first smoothing method of simple averaging allows a manager or analyst to use historic time series data to determine the next data in that time sequence. For example, consider the two sequences below: Series 1 98 100 98 104 100 Series 2 140 166 118 32 44 Both series 1 and series 2, have the same average (of 100) – but clearly from the range of data presented, you would have more confidence with this forecast for series 1 data – why? The variance of series 1 is small compared with Series 2 and hence the environment which generated this data is seemingly more stable and hence, predictable. We therefore have more confidence in our future forecast for Series 1. Consider for example – averaging is simply described as: ∑ (x1+x2+x3...xn) F(t+1) = n Where F(t+1)= future forecast in time period (t+1) t= time (assumed to be current) xn= data for ith period (where i=1 to n) n= number of data points in the averaging calculation The variance in series 1 and series 2 is defined as the average of the squared differences from the mean, or: ∑ (xi-xm)2 Variance = n Where xm = mean of time series data sampled xi= ith data point in the time series data. 3 Working through series 1 and series 2 – the variance for series 1 is 4.8 whilst that for series 2 is 2808! Aside from this problem with both this method and these two data series, other concerns focus upon the response of this method to changes in data (i.e. averaging over a large number of data values (a large n) will mean that the next forecast at (t+1) will be slow to respond to changes in that historic data). Also there are potential trends arising from other factors shaping the data (as well as how rapid those trends change) and noise in the data (which is hard to eliminate). 2.3) Moving Averages Clearly using simple averaging and including ALL the data in that sampling can generate significant problems in terms of forecasting responsiveness and accuracy (with large variances). One immediate improvement is to sample some, but not all the available data in the time series dataset. The choice of how many historic data points are considered in the moving average forecast (N) can be chosen depending upon the stability of the environment of the data and sometimes, reflect a regular period in the data (i.e. the data may evidence a cyclical trend in the data and an effective choice of N can help ‘depersonalize’ that data). The ‘moving average’ has the simple formula of: F (t+1) = [D(t) + D(t-1) +… D(110) + D(109) + D(108)…]/N Or (say for N=3) – F(t+1) =(D(t)+D(t-1)+D(t-2))/N Where F(t) = forecast of a data value at time t Where D(t) = data actually observed at time t Clearly a moving average projective forecast for time period (t+1) is more responsive to changes in the historic data (and for example, a smaller value of N increases the sensitivity of the response to changes in that data). This method also allows the manager / analyst to also ignore some data. Conventions diverge on how to represent moving averages – within datasets. One approach is to recognise that as an average, this forecast should be placed at the mid-point of those data points sampled. Alternatively, the moving average forecast should be placed at the next point in time (i.e. t+1). For example: MS Excel offers additional tools and statistical functions to aid the analysis of data. These functions are accessed through the ‘Data Analysis’ Excel Add In. For MS Office 2007 for example, this is added through the clicking the Office Icon (top LH corner of the screen), selecting excel ‘options’, then highlighting the radio button for ‘Analysis Toolpak’, followed by selecting ‘Go’. Next select ‘Analysis Toolpak’ and click OK again. You will then find ‘Data Analysis’ under the Data menu tab. Under this Data Analysis tool, there are a range of additional statistical functions available for use. This includes ‘moving averages’. 4 Populating the relevant cell entries in the Moving average dialog box (below) is straightforward. Where the input range is the original historic time series data, the interval represents the number of data points over which you wish to average, and the output range is the cells into which you wish the moving average calculations to be placed. You can also choose to plot a chart of the moving average output and calculate the standard error (which is the difference between the forecast moving average and the actual data observed for that time period). 2.4) Exponential Smoothing Data Forecasting Exponential Smoothing refers to a forecasting method that considers a different weighting given to both the most recent forecast and the most recent historic data. It is a form of moving average forecasting but offers greater responsiveness and noise reduction. In this sense, it reflects the ‘exponential curve’ although the exponential function itself is not part of this analytical model. Figure 2.1: Representation of varying weighting of data Much fewer data points are needed to support the next period forecasting compared with simple averaging or moving averages. The calculation used is: New Forecast = (a fraction of the most recent actual data) +(1-the fraction chosen) x most recent old forecast made This can be written as: F t = α A t-1+ (1- α) Ft-1 F t+1 = α A t+ (1- α) Ft Where: 5 α = weighted smoothing constant ( 0< α 16, we simply extend the x column (Quarters), to determine the trend forecast and then modify it by the appropriate seasonal percentage value. So – it is clear that the decomposition method, utilizing an additive or multiplicative approach to manage seasonality, provides significant scope and flexibility to model a variety of univariate data (with one dependent variable). However, there are two questions that then arise which need further consideration. 1. Can we develop greater understanding about the errors in our models – in particular how good is our model? Or in other words, how much variance have we been able to explain by our simplifying assumptions used to generate our assumed mathematical relationships? 2. And what can we do, if these approaches only seem to generate poor or inappropriate modelling interpretations of the data we have gathered? We can take these questions in turn and explore another statistical relationship – namely Pearson’s Coefficient (of correlation) r and the Coefficient of Determination (Pearson’s Value) or R2. We will examine this mechanistically first, before introducing some excel shorthand to determine its value more efficiently. Pearson’s Coefficient (r) is a measure of the linear association between two variables (and by implication how accurately you can predict one from knowing the other). As we are at this time considering only linear (assumed) relationships, we would expect r to have the range of: -10.5 difference, then we have a poor fitting chosen model and need to review our methodology. We can either determine R2 by a simple calculation (using the formula given), or use Excel’s functionality to determine this value and aid our understanding of what we might have missed in setting up our forecasting model. We introduced earlier, the Data Analysis add-in for Excel when discussing moving averages and exponential smoothing. The Add-in also has a regression function which can be used to determine both linear and non linear best fit equations, to aid your modelling. Let’s take a simple problem first and then develop it further. Question: Q) A specialist has advised that the number of FTEs( Full time employees) in a hospital can be estimated by counting the number of beds in the hospital ( a common measure of hospital size). A researcher decided to develop a regression model in an attempt to predict the number of FTEs of a hospital by the number of beds. 12 hospitals were surveyed and the following data obtained. These are presented in sequence (by number of beds): 22 Figure 2.11 mber of beds FTEs 23 69 29 95 29 102 35 118 42 126 46 125 50 138 54 178 64 156 66 184 76 176 78 225 We need to find the appropriate regression equation to test the state hypothesis and consider the errors in that resulting equation. From Data – Analysis – we select ‘Regression’ – 23 We populate the relevant cell entries in the dialog box (follow the numbered arrows above) as: 1) X range – is your independent variable data (here the number of beds). Place the cursor in the dialog box and highlight ALL the cells (i.e. the cell range) you wish to include as your X data (this would be here the values from 23 through to 78). 2) Y range – is your dependent variable data (here the number of FTEs). Place the cursor in the dialog box and highlight ALL the cells (i.e. the cell range) you wish to include as your X data (this would be here the values from 69 through to 225). 3) Select ‘output range’ and then select a blank cell on the worksheet from which Excel will put the statistical calculations 4) You can also check the residual and residual plots boxes. Residual calculations are the Errors (i.e. E(t)). This is the difference between the observed data and the forecast data using the model structure you have told excel to use. In this example we have assumed a simple linear relationship as the plot of beds vs FTEs is shown below (and as we have been asked to test the hypothesis that there is a linear relationship between the two sets of data). A residual plot will be therefore, a plot of the errors of the forecast. It is helpful for a manager (and modeller) as it will identify if there are any trends in the errors. 24 82.23706148 -13.2371 95.62608545 -0.62609 95.62608545 6.373915 109.0151094 8.984891 124.6356374 1.364363 133.5616534 -8.56165 142.4876693 -4.48767 151.4136853 26.58631 173.7287253 -17.7287 178.1917332 5.808267 200.5067732 -24.5068 204.9697812 20.03022 In the output from excel is a range of data, some of which has been highlighted. For completeness, Cameron (2009) offers insight on the remaining information as follows: Adjusted R2 – is the coefficient of determination when multiple independent variables have been used in the forecast (hence you’ll only need to refer to this if for example you have y, and variables for x1, x2, x3 etc). Standard Error – is the sample estimate of the standard deviation of the error Observations – number of data points (observations) used in the forecasting For the ANOVA table (the analysis of variance in the data), breaks down the sum of squares into its components, so that: Total sums of squares = Residual (or error) sum of squares + Regression (or explained) sum of squares. Thus Σ i (yi – mean of y)2 = Σ i (yi – forecast of yi)2 + Σ i (forecast of yi – mean of y)2 25 The F column- overall F-test of H0 (this is the so called null hypothesis where there are no expected other independent variables affecting the observed data (i.e. variables for x2 = 0 and variables for x3 = 0)): variable for x2 = 0 and variable for x3 = 0 versus Ha: at least one of variables of x2 and x3 does not equal zero (these are the possible independent variables). An F-test is a statistical measurement of the extent to which a data set exhibits key expected relationships. Hence in this analysis, an F test is the ratio between explained variance and unexplained variance. The next column labelled significance F has the associated P-value (which is a statistical measurement of the confidence you have in a given analysis (i.e. in this example that there is a simple linear relationship apparently determining the dependent data)). As per statistical convention as this value is