Deep Learning 1 Edited transcript.pdf
Document Details
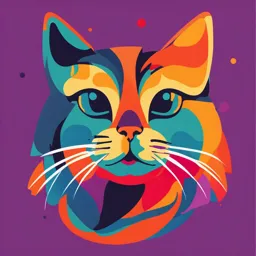
Uploaded by PalatialRelativity
Tags
Full Transcript
00:24 - 00:34 Dr Anand Jayaraman: Hello everyone. Hello everyone. This is Professor Anand Jayaraman. Am I audible? Yes, Professor. 00:34 - 00:36 Speaker 2: Yes, Professor. Good evening. 00:36 - 00:36 Speaker 3: Good evening. 00:38 - 01:00 Dr Anand Jayaraman: I will be leading this particular module...
00:24 - 00:34 Dr Anand Jayaraman: Hello everyone. Hello everyone. This is Professor Anand Jayaraman. Am I audible? Yes, Professor. 00:34 - 00:36 Speaker 2: Yes, Professor. Good evening. 00:36 - 00:36 Speaker 3: Good evening. 00:38 - 01:00 Dr Anand Jayaraman: I will be leading this particular module on deep learning and its variants. Good to see you all. How many people join this session normally? Any idea? 01:03 - 01:11 Speaker 3: The class size is 40 plus. So normally I've seen at least 31. Yeah, 35 sometimes, 35 for sure. 01:11 - 01:19 Dr Anand Jayaraman: Okay, good, good. We already have 31, which is just fantastic. I think we can just01:19 - 01:22 Speaker 3: Professor, can you be a little louder? 01:22 - 01:25 Dr Anand Jayaraman: Oh, my voice is not audible. Clearly, 01:25 - 01:28 Speaker 3: it's not clear. You're quite far to the mic. 01:28 - 01:36 Dr Anand Jayaraman: I see. I see. You know, let me see if I can use my headset. Hopefully that'll be better. 01:38 - 01:38 Speaker 3: 1 01:41 - 01:41 Dr Anand Jayaraman: second. 01:43 - 01:47 Speaker 3: I'll be 01:49 - 01:49 Dr Anand Jayaraman: on 01:52 - 01:52 Speaker 3: mute 01:54 - 01:55 Dr Anand Jayaraman: yourself. 01:57 - 02:00 Speaker 3: Okay, sorry. 02:02 - 02:04 Dr Anand Jayaraman: Yeah. Is this better? 02:06 - 02:08 Speaker 3: Yes, this is better. Yes. 02:08 - 02:36 Dr Anand Jayaraman: Okay. Good. I think we have 32 folks right now. I think that's a good enough number for us to get started. So I know I've seen some of you when I did some of those makeup classes for people who joined this cohort a little bit late. 02:37 - 03:29 Dr Anand Jayaraman: But for others, good seeing you all. As I said, my name is Anand Jayaraman. Let me just get my slides up. So I have been teaching with, teaching machine learning and AI statistics and these types of courses for now about, I don't know, late 9 years, but I'm not a person with computer science or a machine learning background. Hi, I have a physics background and I got a PhD in physics, I don't know, half a century ago. 03:32 - 04:13 Dr Anand Jayaraman: So I'm a science guy, pure science guy, and I was in academia for a while and doing different postdocs. I was there at Penn State University. I was at Duke for a while, you know, all the while teaching, either being in either physics departments or mathematics departments. I also held, you know, did some work on neuroscience at that time. You know, but After 6 years in academia, I realized that people, some of my classmates who were not in academia were actually getting a paycheck. 04:13 - 04:58 Dr Anand Jayaraman: And I decided that perhaps it's time to explore something else. And I left academia and I joined a hedge fund company, a Seattle based hedge fund company as a quant, where we were using mathematics, differential equations and advanced statistics to understand the financial markets. I was there in the hedge fund for about 10 years or so. And by the time I left the firm, I was a portfolio manager, was managing a fund close to $80 million. And we were building algorithmic trading strategies in variety of sectors. 05:02 - 05:46 Dr Anand Jayaraman: It was a fun ride when I was there, but I thought it's time to try something else in career and I thought I'll move to India and figure out what I want to do. Moved to India about 10 years ago and I've been sort of exploring, I mean, trading in financial markets, that's what I do, so I do that. But about close to 7, 8 years back, I started teaching data sciences at the institution called INSOFI, International School of Engineering. That institution got acquired by UpGrad. And so here I am teaching on behalf of UpGrad these set of topics. 05:47 - 06:27 Dr Anand Jayaraman: I have a couple of different positions in addition to my current position in Upgrad. I am a chief scientist at this company, Sujt Sair Analytics, a Detroitbased company. I reside in Hyderabad, India, but you know we do projects in the US, in the Middle East, and in India. Most of these are analytics projects. In addition, I have my own consulting company as well, and where, again, I do projects for, but the projects that I do are mostly related to financial markets. 06:27 - 07:13 Dr Anand Jayaraman: Pretty much all the time, I'm using some ideas from data scientists to make some useful contributions towards financial markets, right? To be able to be investing in financial markets. So I build, I don't know, risk prediction algorithms or algorithms that try to decrease, actively decrease or increase leverage based on the market's volatility, predicted volatility of the markets and so on. So pretty much my bread and butter is analytics. So either standard machine learning or neural networks, this is what I do. 07:14 - 07:53 Dr Anand Jayaraman: So I'm actually more of a practitioner rather than an academician. Majority of my time I am building solutions for companies. You know, the interface that I have here, where I'm projecting the screen and talking, unfortunately does not allow me to see the browser. So in case of any of you are typing any questions or anything, I won't be able to actually see it. Right. 07:53 - 08:08 Dr Anand Jayaraman: So here's my request to you. If please, if there are any questions that you have, please do unmute yourself and ask. Yep. I'm hoping I'm still audible. Right? 08:08 - 08:10 Speaker 3: Yes, Professor. Yes, Professor. 08:10 - 08:28 Dr Anand Jayaraman: Wonderful. Thank you. I like it when you unmute yourself and ask. The session can get quite boring if it is 1 way, where I'm the only person who's talking. I know when I'm the only person who's talking, generally people tend to sleep. 08:29 - 08:51 Dr Anand Jayaraman: I've seen that plenty of times whenever I try to teach my daughters. I mean I'm sure it happens other times too during my lectures. I do get paid anyways but it'll be it's always fun when people are awake and interact and ask questions. So that's about me. I don't know your background yet. 08:51 - 09:18 Dr Anand Jayaraman: I had requested a grad to share with me your backgrounds. I haven't done so yet. I'm hoping by the end before the beginning of next session they will share some your backgrounds. That will be very helpful for me to pace the course, to pace the, you know, decide on how fast to teach and what to cover, what not to cover and so on. But today is the first session. 09:19 - 10:11 Dr Anand Jayaraman: We have, you know, long, 11 long lectures, so we'll be able to adjust the pace accordingly starting from next lecture. With so many students here, you know, around 30-35 students, it's hard to do a round of introductions with everyone, which is what I normally tend to do in my other DBA program. Typically, you know, this DBA programs are typically a little bit smaller in size, but this particular program has had fantastic reception, which is great, which is great for a teacher, right? I mean, normally, when I'm teaching these DBA programs, it is like, you know, 7 students there, right? And it's very hard to stay awake when you have just 7 students, and there's not that many interactions. 10:11 - 10:35 Dr Anand Jayaraman: But I'm told that, you know, that's not the problem with this batch. Everyone interacts, which is fantastic. So I'm looking forward that you will keep up the tradition and interact during the session. As always, please do stop me and ask any questions at any point of time. Don't wait until the end of the session to ask any questions. 10:36 - 10:39 Dr Anand Jayaraman: Yeah? Any comments before we get started? 10:42 - 10:43 Speaker 3: OK, good. 10:45 - 11:37 Dr Anand Jayaraman: OK, so what is the agenda today? The agenda today is, you know, we are starting our journey in understanding neural networks. And so today we'll be talking about a very simple model of, we'll introduce neural networks, we'll talk about a simple perceptron model, and we'll talk about the relationship between this model and some of these other models that you've already learned, and we'll talk about what are the limitations of these models, And hopefully by the end of today's session, we'll also cover how to overcome this limitations. So that's basically what is in plan today. And it doesn't matter if you don't get all the way there, or as I said, we have 11 long sessions. 11:37 - 12:20 Dr Anand Jayaraman: We'll be able to cover everything that we have planned for this particular module. Okay, enough of long-winded introductions. Let's just get started, yeah? So I bet all of you signed up for this particular program because you've heard about AI, right? I mean, clearly, 1 of the reasons why this particular DBA program has been so successful and so many people joining, is mostly, I'd say, because of the sudden excitement about chat GPT, right? 12:20 - 12:58 Dr Anand Jayaraman: Chat GPT, which was introduced now about a year and a half ago, completely took the world by surprise, right? Of course, AI researchers have been working on this and have been building this a lot of fun things for a while. But, you know, the common public rarely gets, I don't think a majority of the public would have ever even heard of this term LLM before chat GPT came. However, most people had heard about automation. Automation has been there everywhere. 12:58 - 13:48 Dr Anand Jayaraman: You see in a factory floor where on the assembly line, you have robots that are helping with assembly, right? And you have heard about, Obviously you've seen this, the Defense Department has been actively working on trying to build robots for its particularly risky mission. And we also know that when We have the Mars Rover that went up. It's operating without explicit human guidance in doing its, in the research, when it's helping out, when it's collecting samples and helping in the research. There's a whole bunch of automation over there. 13:49 - 14:32 Dr Anand Jayaraman: And of course, we all know about self-driving cars. And we have seen how some of us have even ridden in the self-driving cars. And There's been plenty of examples, even before Chadjee Piddy came, of what we all recognize is AI. And this is the main reason why we have a program like this, and where many of you who are business leaders, know that AI is going to have big impact in industry, in business, and in life in general. And so I'm sure you're very excited to learn about what makes AI tick. 14:33 - 15:01 Dr Anand Jayaraman: Perhaps some of you have even heard about, you know, algorithms such as, you know, we have heard the term deep learning, right? Deep learning sounds fancy and deep and philosophical. And you might have heard people say that, deep learning is what makes AI really tick, right? And it sort of makes sense. Artificial intelligence sounds profound. 15:02 - 15:45 Dr Anand Jayaraman: Clearly there must be some deep learning happening there to make AI work. Then instead of teaching this, we have been teaching you lots of other stuff. I know we have had fantastic set of lecturers leading the other sessions, but we have been teaching about SVM and linear regression and logistic regression and random for us and so on. I won't blame yourself if you're wondering, did I even register for the right course? I mean, where exactly is AI in all of that and where is this mysterious thing called deep learning? 15:46 - 16:31 Dr Anand Jayaraman: You know, the wait is done, here we are. And this is the module where we are going to be talking about all of this. Hopefully, while you looked at the previous module where they were discussing different machine learning models, you were paying attention, you understand how general machine learning works because what we're gonna see is that a lot of the concepts that we learned there is going to be applicable over here. Most of examples that we've talked about in the previous modules were all about structured data. What is structured data? 16:31 - 17:07 Dr Anand Jayaraman: Structured data is data that can be put in the form of an Excel sheet. That is what is structured data, rows and columns. I would venture to say that 90% of businesses, day-to-day businesses, when they're talking about data and they're dealing with data, they are pretty much dealing with structured data, which is why databases are so common. It doesn't matter which industry you're in. There's a database admin there, and they're collecting data. 17:07 - 17:51 Dr Anand Jayaraman: So structured data has been extremely important in business analysis. Now, what we will do, but you do know that when people talk about AI, any of these, robots or chat GPT or self-driving car and so on, it's not dealing with structured data. It necessarily is interacting with the real world, the raw data that's coming from the real world. So images, videos, audio, sound, and text, all of these are natural human interactions. These natural human interactions do not easily fit into this particular form of structured data, right? 17:51 - 18:32 Dr Anand Jayaraman: Which is why we call all of this as unstructured data. The machine learning models that you've learned till now are not really suited to handle unstructured data, right? Which is where, you know, this other set of methodologies called deep learning come in and they will help us understand unstructured data. But even though this particular module is deep learning, for majority of this module, we are going to continue dealing with only structured data. We're gonna be dealing with structured data because We have been seeing structured data still now. 18:32 - 19:09 Dr Anand Jayaraman: We understand the models, machine learning models that have been taught to us with structured data. And so what we'll be doing today is we'll introduce neural networks, which is the first step before we get to deep learning, using structured data. And once we understand what is neural networks, how does it work, and so on, then we'll slowly go towards deep learning, and we'll discuss deep learning. But most of the time, our crutch is structured data. We use structured data to understand these new set of models, right? 19:09 - 19:33 Dr Anand Jayaraman: And once we are comfortable with that, then we'll move on to unstructured data, and then we'll see how I can take the same models and start using, applying it on unstructured data, right? So that's the way we are able to handle this particular module. Questions, comments? Everyone awake? Even 1 volunteer is good enough for 19:36 - 19:37 Speaker 3: me. Yes, absolutely. Even 1 volunteer has to be 19:37 - 19:37 Dr Anand Jayaraman: in our movie. Yes. Yes, we're awake. Yes, absolutely. Yes. 19:38 - 19:40 Dr Anand Jayaraman: We're waiting. We're gladly waiting. Fantastic. 19:41 - 19:41 Speaker 3: Very exciting. 19:41 - 19:46 Dr Anand Jayaraman: This is like giving a super long introduction without actually introducing the hero of the movie. 19:46 - 19:49 Speaker 3: Actually, when you asked, we woke up. 19:50 - 19:50 Dr Anand Jayaraman: Ah. Good. 19:51 - 19:53 Speaker 3: Good. Good. Good. Good. Good. 19:53 - 19:54 Speaker 3: Yeah. Yeah. 19:54 - 20:00 Dr Anand Jayaraman: Good. Good. Good. Good. I got to remember the frequency and the tone and the volume with which I asked. 20:01 - 20:12 Dr Anand Jayaraman: So it continues working. Good. OK. Let's start. So what is the, we've learned a bunch of different machine learning models. 20:16 - 20:20 Dr Anand Jayaraman: What is the best learning system that we know? Applying common sense. 20:21 - 20:23 Speaker 3: Sorry? Applying common sense. 20:26 - 21:03 Dr Anand Jayaraman: Applying common sense, absolutely. Well, The best learning system that we know is humans. We magically are able to learn stuff from things around us. What we've been trying to do is trying to see how an expert human being is able to make some predictions, right? And we want to train an algorithm to be able to do the exact same thing. 21:04 - 21:57 Dr Anand Jayaraman: An expert human being might be able to look at data, credit card data, and identify what data might be likely to be fraud. Or a person sitting at the bank, I'll be looking at loan applications and seeing the person who's come for the loan, and from then be able to make a guess as to whether this person is a good bet or a bad bet. This is what an expert human being do. And our goal so far has been trying to develop algorithms which in a systematic way can bring about the similar kind of predictive accuracy or better, hopefully, compared to a human being, an expert human being. So we know the best learning system is the human brain, the biological learning system. 21:58 - 22:24 Dr Anand Jayaraman: The thing is, If I want to replicate a human brain, I got to sort of understand how it works and sort of understand its complexity. Now the thing is, Do you know what is the most basic computational unit in the human brain? What it's called? Neuron. 22:24 - 22:25 Speaker 3: Neurocell. 22:25 - 22:46 Dr Anand Jayaraman: Neuron, right? So neuron, the single cell is the neuron. Our brain, right, has billions of neurons, billions of neurons, right? And not single neuronal cell, but billions of neurons that are acting together. And these billions of neurons are interconnected in extremely complex ways. 22:46 - 23:08 Dr Anand Jayaraman: There are trillions of connections that are there. Now if you want to create an artificial brain. That's just hopeless. Absolutely hopeless for us to create billions of neurons with trillions of connections. So right away you'd want to give up. 23:08 - 23:34 Dr Anand Jayaraman: So that meant I can't start that big. There's no way I can start that big. So then we start to ask this question, you know, do I really need billions of neurons to be able to learn something, right? Does the size of this learning system, does the size of the brain really important? Very valid question to ask, right? 23:36 - 23:51 Dr Anand Jayaraman: So the question that I'm effectively asking is that, is intelligence possible even with a small brain? What do you think? Is intelligence possible? Does the size of the brain matter? Yes. 23:52 - 24:01 Dr Anand Jayaraman: Yes, it's possible. Okay. What do you think of chimpanzees, which might probably have a bit smaller than us? 24:01 - 24:09 Speaker 2: I think it's not the size, it is the density of the neurons and the maturity of the proliferation that matters more even in a biological system. 24:10 - 24:11 Dr Anand Jayaraman: Okay, good. 24:11 - 24:17 Speaker 3: But 1 neuron also can learn right? 1 neuron can also play a role and learn. 24:18 - 24:28 Dr Anand Jayaraman: So I don't know yet. Right? It's not clear to me yet that a single neuron can learn. We know certainly millions of neurons can learn. 24:29 - 24:31 Speaker 3: I believe it will learn. 24:32 - 24:39 Speaker 2: 1 neuron can learn and carry information but I think it is a series of neuron that makes a biological system. 24:40 - 24:46 Dr Anand Jayaraman: Okay, we know that the series of neurons are needed. I don't know yet about 1 neuron. We'll come there in a second. 24:46 - 24:52 Speaker 4: Even a single cell organism has intelligence, right? So that is effectively a single neuron. 24:52 - 25:03 Dr Anand Jayaraman: OK, are we sure it has got intelligence? So that's the question, right? So let's get there 1 step at a time, right? Let's not get right away to a single solution. And we have to define intelligence. 25:04 - 25:18 Dr Anand Jayaraman: Yes, exactly. Exactly. And that's where we are going with this. We agree that humans are intelligent. Although there are cynics among us who question that, looking at the damage that we cause around us. 25:19 - 25:32 Dr Anand Jayaraman: But agreed if you agree that humans have intelligence. The next question is does that's the size of the brain matter. That's a smaller brain. Can it think does it have intelligence. That's a question. 25:32 - 26:06 Dr Anand Jayaraman: And the answer is, as you all have given, is most definitely yes. What kind of examples are there around us to show that? There's been like fantastic, huge set of examples can be given as to how even learning or thinking is possible even at a small break. Right? Now here's a set of experiments that with pigeons, which is amazing, where it was shown that pigeons can be trained to recognize art. 26:08 - 26:33 Dr Anand Jayaraman: I'm showing you here a bunch of pictures, some from Van Gogh, some from Chagall. And if you ask me, if you show me a painting and ask, do you think it's Van Gogh or Chagall? Or was it a sixth grader who's splashing paint around? I wouldn't know. I've been diagnosed as aesthetically blind by my wife. 26:34 - 26:52 Dr Anand Jayaraman: So I don't know most of art. I can't recognize numbers. I see beauty in numbers, but art, no, that's far off from for me. But pigeons can be trained to recognize art, which is amazing. The thing is, how do they train it? 26:53 - 27:22 Dr Anand Jayaraman: So they put this pigeon in a cage. Let's keep aside the animal cruelty and experiments with animals and all of that, the moral dilemmas and all that aside for a bit. So how do they train pigeons to recognize art? So they have this pigeon in the cage and they put 2 buttons in front of it. And the pigeon is randomly pecking at this button. 27:23 - 27:52 Dr Anand Jayaraman: And they start showing images in front of it. And let's say this 1 button is a blue button, another 1 is a red button, right? And a picture, a painting by Van Gogh shows in front of it. And this pigeon, let's say out of randomness, pure randomness, presses the red button. The moment it presses the red button, you know, a pellet, a food pellet drops in front of it. 27:52 - 28:16 Dr Anand Jayaraman: It's happy, it eats, right? Now, if it presses the wrong button, nothing comes, right? Next time some other painting is shown, And if it again presses the right button, according to the painter, it gets a food pellet. Eventually, the pigeon learns to make correction that when it sees this particular painting, I need to press this button. When I see the other painting, I need to press the other button. 28:16 - 28:52 Dr Anand Jayaraman: And that's the way they train. I mean, the remarkable thing is that they are able to get 95% accuracy. The patients are able to discriminate van Gogh and Chagall with 95% accuracy. I can challenge you, most regular folks will have much lower accuracy than that. What is even more remarkable is that when you show paintings that it has never seen before by the same painters, it's still able to discriminate with 85% accuracy. 28:53 - 29:17 Dr Anand Jayaraman: So it has actually learned the fundamental style of painting by these artists, which is just amazing. Clearly, you have to admit that the pigeons are displaying intelligence. Right. There are plenty of examples. I mean you can pick an animal species and there are people have done experiments there which shows intelligence. 29:17 - 29:38 Dr Anand Jayaraman: Mice for example mice have been trained to figure its way around mazes. So it has this strong spatial memory, and it can find its way around mazes. I have these links over there. You should check it out when you've got time. It's just really remarkable what it can do. 29:38 - 30:17 Dr Anand Jayaraman: Mice can also be trained to recognize drugs or explosives and things like that, right? We know that dogs do a good job of recognizing explosives. But when you have some particular bomb hidden in some narrow corridor or a narrow path, there's only a narrow pathway there, Having a trained mice might be helpful. So they have shown that mice can be used to do this detection, which is just quite amazing. So again, it goes to show that thinking is possible even with a small brain. 30:18 - 30:55 Dr Anand Jayaraman: Now that gives us hope, right? It gives us hope because if we are trying to build an artificial intelligence system, we thought of the human brain, But then we know that the size of the human brain is too big and it's very complex and there is no way we can build an artificial human brain. So we went to this next question like can I you know can I build something smaller? Is it possible to have learning with a smaller brain? And clearly we have sort of hopefully have convinced you that it is possible to have your thinking process even with a smaller brain. 30:56 - 31:33 Dr Anand Jayaraman: Now, let's go back and do some biology. The fundamental unit of thought, the biological unit in our brain, the thinking cell, is called as a neuron. And a human brain has about 100 billion neurons and about 1,000 trillion connections, complicated connections, synaptic connections between these neurons, right? And together, this is what forms this intelligent system. Now, we have sort of agreed that perhaps you don't need that many. 31:33 - 31:56 Dr Anand Jayaraman: We might be able to do learning with a smaller number of neurons. So then the question becomes, how small a set of neurons do I need in order to have intelligence? Is 1 really enough? And that's where we are going with this discussion. So before we go there, let's first look at a single neuron and look at the structure of a single neuron. 31:57 - 32:23 Dr Anand Jayaraman: Now, I see, I can hear some jingling sound. I suspect it's when somebody is typing a question or answering or making a comment that this tingling sound is coming. But unfortunately I have to stop the presentation each time to go back and see what is the comment that's being made. I would very much appreciate if you can just speak up. It's very helpful. 32:23 - 32:49 Dr Anand Jayaraman: Or if you are not able to unmute yourself and speak up, because you're in some public setting or something, if 1 of others who are part of the session, if you are reading a comment by your colleague, please speak up on their behalf. It'll really help me and allow us to keep pace of the class. Yeah. Are there any questions that I haven't addressed? 32:49 - 33:16 Speaker 3: No, there is no open question at this point. Thank you. Sir, human brain has 2 logical brain and creative brain. Many of us we use our logical brain because of the learning. The creative brain is mostly used by people who question, why should I accept, why not I create something better, which is called as creative. 33:17 - 33:25 Speaker 3: Does the animals, even the mice, or the animals, and have similar classification of logical and creative? 33:27 - 33:58 Dr Anand Jayaraman: So this separation of logical versus creative, that is a human construct and it's a functional separation. The biology itself does not separate it. You have a single unit over there. The biology itself does not separate it. And this is exactly what our quest towards AGI is all about. 33:58 - 34:44 Dr Anand Jayaraman: Can I build 1 system which can do everything, which can do multiple functions? And the separation per se is our human construct as this class, this way we solve some things, some set of problems is different than this other class of problems and we handle it differently, right? We are going to take a very pragmatic approach with respect to structure, right? We are going to say, I don't care what the structure is. If it can do the task in front of me that I've given, I will accept that it's intelligence. 34:48 - 34:55 Dr Anand Jayaraman: Perhaps I should step back a little bit. Was this discussed before? How do I think about AI? 34:59 - 35:00 Speaker 3: What is AI? 35:01 - 35:03 Dr Anand Jayaraman: What is artificial intelligence? 35:04 - 35:07 Speaker 3: Trying to mimic the human brain in an artificial way. 35:08 - 35:14 Dr Anand Jayaraman: Trying to mimic the human brain in an artificial way. Good definition. Any other definitions? 35:15 - 35:23 Speaker 4: Responding so that it cannot be distinguished whether it is a human or a machine. The ability of a system to respond so that you cannot distinguish. 35:24 - 35:26 Dr Anand Jayaraman: Very nice. Very nice. Yeah. Yeah. 35:26 - 35:36 Speaker 3: It has 2 components to that and the way it is, it is mimicking the way human beings learn and automating the repeatable tasks. Okay. Mimicking the way human beings learn and 35:36 - 35:44 Dr Anand Jayaraman: automating the repeatable tasks. OK. Mimicking the way human beings learn and automating repeatable tasks. OK. Any other thoughts? 35:45 - 35:45 Dr Anand Jayaraman: Any other? 35:50 - 35:54 Speaker 3: I think the intelligence have the 60 cents, 7 cents and 8 cents. 35:55 - 36:27 Dr Anand Jayaraman: Okay, good, this is fun. Let me start by asking these questions. When I say that I'm creating an intelligent system, I'm going to create an intelligent program. What do you expect from it? I see Srinivasan has raised his hand, please go ahead. 36:35 - 36:43 Dr Anand Jayaraman: So, I like it. So, we have decision making. Okay. Oh, man. Decision making. 36:43 - 37:01 Dr Anand Jayaraman: Okay, that's an important component of intelligence. Anything else that comes to mind? Also, I think all the problems, all the problems, we're able to take. Sorry, Can you repeat it again? 37:01 - 37:03 Speaker 3: It should be able to solve problems. 37:04 - 37:16 Dr Anand Jayaraman: So problem solving, OK? Problem solving, decision making. So they're all related, OK? But let's definitely write it out separately. Problem solving, decision making. 37:16 - 37:18 Dr Anand Jayaraman: Anything else? Come to mind? 37:18 - 37:24 Speaker 3: How it responds to a scenario where it is not being trained. 37:26 - 37:58 Dr Anand Jayaraman: Scenario not being trained, okay, that is a hard 1. That's a very, very, very hard 1. We as human beings will fail most of the time if that's the threshold you place. If I show you, for example, the Schrodinger equation, if you haven't been trained there, you would find it challenging. 37:59 - 38:08 Speaker 3: Yeah, What I was trying to mention is like it's a similar situation, that similar function like it has been trained on, there's a prediction. 38:09 - 38:30 Dr Anand Jayaraman: Right. So definitely training is needed. So some similarity of training is needed. The problem sets, when after training on 1 set of problems, we are able to solve it again, that would be intelligence. Regions where we haven't been trained to be able to expect to solve, that's hard even for human beings. 38:31 - 38:36 Dr Anand Jayaraman: That's correct. So decision making, problem solving. 38:37 - 38:41 Speaker 3: The main problem of intelligence is thinking ability, right? 38:42 - 38:59 Dr Anand Jayaraman: Okay, so thinking, okay, I like that except that word is very hard to define. I know what it is to make decisions. I know what it is to solve problem. Okay. 38:59 - 39:09 Speaker 3: We know how to process. Cognitive thinking as the human does it read, learn, remember, and then. 39:10 - 39:23 Dr Anand Jayaraman: Okay, so learn is there, remember is there. Remember is that basic recollection of facts. We have some facts was there. Now I'm telling something. The same facts are coming up. 39:24 - 39:28 Dr Anand Jayaraman: Remember, these are all good ones. 39:28 - 39:32 Speaker 3: And then you can start to think that intelligence systems 39:35 - 39:45 Dr Anand Jayaraman: should be able to basically take decisions itself, right? Not with the help of any tool. Okay, so it should be a decision-makers, yes. We have written that. So here is 1 big thing. 39:45 - 39:53 Dr Anand Jayaraman: I'm gonna strike and write a program. And, what is the other objective? 39:54 - 39:56 Speaker 4: Yeah, input discrimination and output control. 39:58 - 40:21 Dr Anand Jayaraman: OK, there should be. So discrimination decision making and problem solving is what you're talking, but these should be made based on an input or interaction with the real world. Right. And that's what you're effectively saying. So let's start. 40:21 - 40:23 Dr Anand Jayaraman: We have a couple of points to 40:23 - 40:25 Speaker 3: work with. What about cognition? 40:26 - 40:43 Dr Anand Jayaraman: See, cognition is a very difficult word. I don't know how you measure it, how you measure thinking. See, these are all actions that can be measured and observed. Cognitive ability. But how do you define cognitive ability? 40:43 - 40:44 Dr Anand Jayaraman: Based on actions, right? 40:45 - 40:58 Speaker 3: So just 3 things here, doctor. 1 is the perception where you have the 5 senses, right? And then you do the cognition where you understand and then do some action based on it. And learning part comes over there. If these are the right 1, the AI. 40:59 - 41:11 Dr Anand Jayaraman: Right, so yeah. I want to avoid using the word cognition or thinking. I want to define things that I can observe. Actions are something I can observe. 41:12 - 41:15 Speaker 3: Professor, can we include a parameter like... 41:18 - 41:19 Dr Anand Jayaraman: Go ahead, Sunila. 41:19 - 41:28 Speaker 3: Yeah, Professor, I was thinking about working within constraints, let's say, to be able to understand the problem statement. 41:29 - 41:44 Dr Anand Jayaraman: Absolutely. Yeah. So clearly, I have tried to abstract out things. There is some input. Based on the input, I am making decisions and taking actions. 41:44 - 41:51 Dr Anand Jayaraman: That is what Intelligence. And that's what we expect out of an intelligence system. What 41:52 - 41:54 Speaker 3: are self-monitoring capabilities? 41:57 - 42:11 Dr Anand Jayaraman: We will come there. All of that is clearly related to this, perhaps trying to improve over time and so on, right? We'll get there in a second, right? I'm just trying to understand, just to have a bare bones definition of intelligence. 42:11 - 42:12 Speaker 3: It's not 42:12 - 42:13 Dr Anand Jayaraman: going to be comprehensive. 42:15 - 42:34 Speaker 3: It is only programmed into this as an input. It does not think. It does not create anything other than what is being programmed inside? Am I right, Mr. Rawaluddin? 42:35 - 43:01 Dr Anand Jayaraman: So, again, Srinivasan, you're using the word think. That is something that we cannot measure. We want to define something based on action. Just 1 second, we are coming there. We do have a reasonable amount of discussion to start working with and we are going to get there. 43:04 - 43:32 Dr Anand Jayaraman: I am going to start by writing a program. And I'm going to start writing, I know this is not a hands-on coding session or anything, but still you will be able to understand this particular program very easily. Hello. Is this AI? Is this an AI program? 43:33 - 43:34 Speaker 3: No. 43:34 - 43:39 Dr Anand Jayaraman: No. Okay, we're all very clear on that. No. Why is it not an AI program? 43:41 - 43:42 Speaker 2: Instruction and command. 43:44 - 43:55 Dr Anand Jayaraman: What is it missing from our definition, from our expectation of AI program? We basically said talked about input and essentially decision making or problem solving. 43:55 - 44:00 Speaker 2: There is no decision part involved over here because it's just giving an instruction to print hello. 44:00 - 44:11 Dr Anand Jayaraman: Very nice And how will it even take a decision? There's no input. It's not taking any input from the world at all. So everyone agrees this is not an AI program? Yes. 44:11 - 44:20 Dr Anand Jayaraman: Good. So now let me make a modification. OK, print. What is your name? Print what is your name. 44:24 - 44:48 Dr Anand Jayaraman: And now I'm going to read from the screen, read name. So when the user types the name, we are going to read it. And now I'm going to say, hello name. Now, when I run this program, it's going to show up on your screen, what is your name? And then when you type your name, it's going to read it. 44:49 - 44:51 Dr Anand Jayaraman: And then now it's going to print hello name. 44:51 - 44:52 Speaker 3: It's been instructional. 44:53 - 44:59 Dr Anand Jayaraman: It's listening to the instructions. Yes. Would you call it as AI? No. No. 44:59 - 45:03 Dr Anand Jayaraman: What is it missing? It's interacting with the environment, right? It's getting input. 45:04 - 45:05 Speaker 4: No decision, no problem. 45:06 - 45:19 Dr Anand Jayaraman: Very nice. No decision-making. Good. Let's fix that. Now, I'm going to insert over here, print, are you a male? 45:21 - 45:40 Dr Anand Jayaraman: Then I'm going to read, And then instead of this, I'm going to say, if male print. Hello mr name else print hello miss name. 45:41 - 45:42 Speaker 2: This has got 45:42 - 45:46 Dr Anand Jayaraman: decision making, interactions and decision making. There is decision component. 45:46 - 45:58 Speaker 3: But still this is a rule-based, right? It's not a... This is more of an expert system where it relies on symbolic logic. So it's not an AI. 45:58 - 45:59 Speaker 4: Learning, there's no learning. 46:01 - 46:14 Dr Anand Jayaraman: So this is the thing, right? As much as it is, today we don't consider it as AI, all of this rule-based decision making comes under the purview of AI. 46:15 - 46:20 Speaker 3: Yes. It is a smart system, so it is able to do something. 46:20 - 46:38 Dr Anand Jayaraman: So let's talk about now what exactly is AI. AI is this large field of study. You have physics is a field of study. Chemistry is a field of study. AI is a field of study. 46:39 - 46:55 Dr Anand Jayaraman: This field of study is a very old field of study, started from the 1940s on, perhaps even before. What is the goal of the field of study? Physics has a goal. You are trying to understand the physical systems. And chemistry has a goal. 46:55 - 47:18 Dr Anand Jayaraman: Biology has a goal. Roughly, what is this goal of AI? To understand the set of rules that will enable us to replicate human decision making. If you are able to replicate human decision making, then you have AI. This is what the field actually was trying to do. 47:19 - 47:40 Dr Anand Jayaraman: And the earliest AI were all these rule-based methods. Rule-based methods. Your old chess algorithm, right? Where's that? In fact, early on, if you ask someone in the 1950s, how do you recognize whether something is AI or not? 47:40 - 47:51 Dr Anand Jayaraman: They would say 1 of the examples is chess. If computers can play chess, then I would say it's intelligent. Now, exactly. Heuristic systems are also AI. All of these are AI. 47:52 - 48:18 Dr Anand Jayaraman: Today, we don't call them as AI. Today, we don't call them as AI. The thing is that AI is actually a field of study. When common press is talking about AI, this is an AI enabled phone or something like that, it's actually a meaningless statement. Because it's like saying this is a physics-enabled phone. 48:18 - 48:35 Dr Anand Jayaraman: Physics is a field of study. Of course, physics was used to make the phone. It's not physics-enabled phone. AI is a field of study. So what do we mean when we start talking about AI-enabled technologies and AI-enabled systems or whatever? 48:35 - 49:05 Dr Anand Jayaraman: The thing is that usage has started to change, mostly because of popular press. Now in 1970s, as a sub area of AI that got started, this is called as machine learning. Now, what is machine learning, per se? Machine learning is when, and again, I know you have all read this, you have done this, you have had an entire module covering it, I don't plan on recovering the model. I'm just trying to give you the bigger picture of where it stands. 49:06 - 49:35 Dr Anand Jayaraman: So what is machine learning? In machine learning, you want to do things differently than this rules-based method. What is rules-based method? Rules-based method is that an expert human being has given the set of rules on how do you approve a loan. A person, when the person who's coming to a loan officer, if their salary is greater than 2 times the loan requested. 49:36 - 49:52 Dr Anand Jayaraman: And if the number of dependents is less than this, and their credit card balance is less than this, then go ahead and approve the loan. The set of rules is written out. Who has created those rules? A human being has created the rules. An expert has created the set of rules. 49:54 - 50:39 Dr Anand Jayaraman: Now, for the way these rules-based systems work is that you give it important data, the data regarding the loan applicant, what is their salary, what is their credit card balance, how many dependents they have, you're giving important data. And that important data is passed through this set of rules and if else then conditions, and outcomes the decision. This was your basic AI before. These are all rules-based systems or expert systems. But then in 1970s, before 1970s itself, people realized that it's very hard to articulate rules for every single thing that we are doing. 50:41 - 51:16 Dr Anand Jayaraman: For example, I am going to, I had that video on sometime back, I turned the video off and I turned the video back on again. Hopefully you can see my image. And hopefully you recognize that I am the same person who started the lecture. It wasn't a new person. How exactly did you know that You didn't use a set of rules like, the distance between his eyes is 3 centimeters and the length of the nose is 2 centimeter and he's the most handsome person you've ever seen. 51:16 - 51:40 Dr Anand Jayaraman: You haven't used those kinds of rules to recognize me. You are able to recognize, but you are not able to articulate what set of rules you're able to, you use to recognize me. This is why a new way of solving problems started, which is what is machine learning. In machine learning, the way we approach things was different. We just said that, you know, I don't know what the rules are. 51:41 - 52:18 Dr Anand Jayaraman: I am going to figure out, give you examples of important data, past decisions, and all the past decisions. So decisions that were made and the data using those decisions were made. I'm going to send all this data, provide this data to you. And we are going to rely on an algorithm to look at all of this past data, past examples of this thing, and figure out the set of rules. Automatically figure out the set of rules. 52:19 - 52:53 Dr Anand Jayaraman: This is machine learning. In machine learning, examples are given and the past outcomes are given and you're figuring out rules based on your past experience. So you don't have to articulate them. When you have to start articulating them, then it becomes, you can handle some set of examples, some set of human tasks can be handled by explicitly specifying rules, but many of these other human tasks, you're not able to explicitly state the rules. And that is why this machine learning really helped us. 52:53 - 53:23 Dr Anand Jayaraman: This set of methods became extremely popular in 1970s. But then this set of methods were mostly able to handle only structured data. Structured data, this set of methods worked very well with structured data. But to handle unstructured data, we were really struggling. New innovations had to come in in order to start handling unstructured data. 53:24 - 54:08 Dr Anand Jayaraman: That happened just 10 years ago, 10 to 12, maybe 14 years ago, a new set of methods that came up as subset of machine learning called deep learning. This set of methods, which is now a subset of machine learning all of this is another big umbrella of AI. This deep learning is a new set of methods that came up. Now what this set of methods allow you to do is in a sense a little bit of magic. Here you do not even have to explicitly type out your important data. 54:09 - 54:52 Dr Anand Jayaraman: Instead, you just give raw data from the world, a picture, a speech, an email, or whatever. Internally, it is able to, from the raw data, extract out the important data, and then go through this and figure out the rules automatically. And that is what deep learning does. But then as you might imagine, if machine learning required a lot of past data to be able to figure out rules, we are now not only, we are not directly giving the important data points, We are actually asking the algorithm to figure it out automatically. Is the distance between the eyes important? 54:52 - 55:11 Dr Anand Jayaraman: Is the shape of the nose important in recognizing a person? Is the fact that someone has a balding hairline, a receding hairline, is important? You don't know what set of features are important. Instead, you're giving examples and asking the algorithm to automatically learn. So you're providing raw data. 55:11 - 55:34 Dr Anand Jayaraman: From the raw data, it automatically figures out important data. So this deep learning method is much more data hungry than this machine learning methods. So deep learning is extremely data hungry set of methods that have come up. And this has required a lot more computational power than what was required for machine learning. So this is the big picture. 55:35 - 56:00 Dr Anand Jayaraman: Now, this deep learning is basically what modern press calls us AI. Modern press does not really know that AI was a field from 1940s. When people are talking about AI-enabled phones, they are specifically talking about deep learning. And this is what we are going to be learning in this model. We are going to be talking about what is deep learning and so on. 56:00 - 56:22 Dr Anand Jayaraman: But before we get to deep learning, we need to understand the little bit of the history process of how we evolved there, right? How do we, what kind of wrong pathways the researchers took before they arrived at this, right? We will sort of talk about that. We'll give a hint of that. Questions? 56:28 - 56:32 Dr Anand Jayaraman: Professor, could we think in this way? Heuristic rules, 56:33 - 56:34 Speaker 2: over a period of time, 56:34 - 56:57 Dr Anand Jayaraman: it has got more structured and evolved with the ML system? So the thing is this, heuristic rules were still given by a human being. Yes. Right? It is like saying, if you want to win a one-day cricket match, you should think about that. 56:57 - 56:59 Dr Anand Jayaraman: There's a lot of noise. You have some target. 57:00 - 57:05 Speaker 3: There's a lot of noise going on. Sorry, Professor, I think it's, yeah, there is a lot of noise. Yeah, oh, 57:05 - 57:07 Dr Anand Jayaraman: it's OK. Srinivasan, can you go on mute, please? 57:07 - 57:09 Speaker 3: Yeah, it seems to be Srinivasan's idea. 57:10 - 57:19 Dr Anand Jayaraman: Yeah, that's fine. I mean, sometimes when you're outside, there's a lot more noise. And I know particularly when the fan is running fast, it creates a lot of noise as well. 57:19 - 57:29 Speaker 3: No, not from your end, sir. Not from your end. I think this was not turned on, so we got a lot of echo. Yeah, when they're not speaking, if they can go on mute, it'll be great. Thank you. 57:29 - 57:48 Dr Anand Jayaraman: Yeah, that'll help. Yeah. Good, yeah. So Shrini, the thing is, in heuristics, you are using your past experience to explicitly state some rules. Those are all what came under the old rules-based decision making. 57:48 - 58:07 Dr Anand Jayaraman: Expert systems were all about that. In machine learning, I'm just going to say that, you know what, I'm not going to give any of my biases. I'm just going to present to you some data. I'm going to say that here were different loan candidates. Loan candidate 1, loan candidate 2, loan candidate 3, so on. 58:07 - 58:41 Dr Anand Jayaraman: Their salary was so much, the loan amount requested was so much, their number of dependents were so many, and so on and so forth. And I'm going to say that this person returned the loan, this person did not return the loan, and so on. I'm just going to put the past data. And I'm going to ask the algorithm to automatically figure out What set of combinations of numbers is predictive of someone not retiring the loan? And what set of combination of numbers are likely to tell comfortably that this person will pay back? 58:43 - 59:04 Dr Anand Jayaraman: We are asking the algorithm to automatically figure out the rules. So in a sense, the user can be, pardon me for using this term, can be dumb. I don't need to even think. The algorithm will automatically figure out the rules and tell me whether I should approve a loan or not. That is what ML does. 59:06 - 59:37 Dr Anand Jayaraman: So heuristics require some expertise. But ML is taking out that expertise and therefore enabling you in terms of business function, enabling you to quickly replicate expertise. Even when experts are not available in some branch, you're able to replicate expertise because the algorithm has figured out the set of rules that perhaps should be used to give a loan or not give a loan. Is that clear? So it is different than heuristics. 59:39 - 59:53 Speaker 2: Thanks a lot, Professor, because in my mind, I had that even many times in office also I have explained in similar way and in evolution way, but I can understand the expertise angle what you have talked about. Definitely next time I will include it in my thoughts. Thanks a lot. 59:53 - 59:58 Dr Anand Jayaraman: Thank you. Thanks for letting me know. Any other questions? 01:00:00 - 01:00:07 Speaker 1: Of the ability to create new knowledge would that be falling within the ambit of AI now is that still too far ahead? 01:00:08 - 01:00:52 Dr Anand Jayaraman: Creating new knowledge right now that is exactly what we are actually using AI right now and we'll see we can we can come back we'll when we start discussing this more I'll start giving examples right what we think of as what human intelligence is capable of things which we thought were exclusive to human intelligence we are now finding out that perhaps it's not all that exclusive, and it can be replicated. And, you know, in a sense, it is possible for us to play God, in a sense that we are creating new intelligence, which is again able to do things that human previously we thought only human beings can do. 01:00:53 - 01:00:53 Speaker 3: Some examples? 01:00:55 - 01:01:12 Dr Anand Jayaraman: We'll talk about that. I don't want to take away the punchline in the first 1 hour of the movie. This is not 1 hour of the serial, right? This is a long 12 episode serial. We will use the punchline appropriately over time. 01:01:14 - 01:01:23 Dr Anand Jayaraman: Any other questions? Good. Let us get back to the slides. 01:01:24 - 01:01:39 Speaker 4: 1 question, Professor. So in case of deep learning, you're saying raw data, the algorithm is able to extract the important data out of the raw data and then the rules but how would algorithm find out what are the past decisions. 01:01:42 - 01:01:56 Dr Anand Jayaraman: So that is also done it's just that both raw data and the past decisions are fed in. Okay. Okay. Right. And it figures out along with that, right? 01:01:56 - 01:02:19 Dr Anand Jayaraman: The same structure as machine learning, which is why it's actually within a subset of machine learning. So subset of machine learning, right deep learning, it's just that the data can be, you know, in a raw unprocessed format. And it's still able to actually make useful decisions based on that. 01:02:22 - 01:02:26 Speaker 5: So the data cleaning is part of the deep learning thing then? 01:02:28 - 01:03:05 Dr Anand Jayaraman: For now, I don't want to get into that level of details data cleaning all the time it's best if it's done separately but can deep learning handle some of this data cleaning yes absolutely right in that sense we are seeing that right I mean charge EPT you're sending in your statements with the errors, with not full statements and spelling mistakes and so on. And it's still able to figure it out from there. But any time you make the provide cleaner and cleaner data all of these systems will benefit from clean data. 01:03:08 - 01:03:13 Speaker 4: Professor 1 quick question you said deep learning is a subset of machine learning right? 01:03:13 - 01:03:14 Dr Anand Jayaraman: Correct. 01:03:15 - 01:03:21 Speaker 4: Why is that professor? Why can't they keep it as just machine learning why the subset was created what's the necessity if you can 01:03:21 - 01:04:13 Dr Anand Jayaraman: because yeah the subset became essential because the general methods of machine learning that you have learned till now can handle only structured data okay right Now we like the principle of that, where that you're figuring out rules automatically. We like the principle of that. I want to use the same principle now for speech recognition, for email understanding, for understanding images, drawing information from images or videos. I want the algorithm to be able to view a YouTube video and quickly summarize for me what it does, right, I want it to be able to do that, but I don't know how to train it. I know how to train structured data and unstructured data I don't know and deep learning basically allows us to take that additional step. 01:04:13 - 01:04:16 Speaker 4: Thanks professor absolutely for it. Thank you. 01:04:17 - 01:04:27 Speaker 6: So Professor out of curiosity, you know, Dr. Srimam, Srimam Murthy had actually toiled blood and sweat in teaching us over the last 3 months. So are we faring well in your Q&A? 01:04:28 - 01:04:57 Dr Anand Jayaraman: Yeah, yeah. I mean, this is great. I love the fact that everyone is interacting and this is wonderful but so let me tell you this right the my lecturing style is such that you know I changes take slightly different pathways based on questions and it's lovely that we are having questions and it allows me to spend more time here less time there and so on. 01:04:57 - 01:05:02 Speaker 6: But whiteboard is good professor because you're causing us to think which is good. Thanks. 01:05:02 - 01:05:13 Dr Anand Jayaraman: Yeah, because we have plenty of time right now. As I said, 12 episodes are there. We're towards the end. We might have have speed up a little bit, but this is first class. We are meant to chill. 01:05:13 - 01:05:15 Dr Anand Jayaraman: That was the main agenda today. 01:05:15 - 01:05:17 Speaker 3: We love the style and pace. 01:05:17 - 01:05:28 Dr Anand Jayaraman: Okay, good. Yeah, we are yet to see the hero of the movie. The hero is going to show up soon. Other than me, of course. Yeah. 01:05:28 - 01:05:29 Dr Anand Jayaraman: Sorry. Go ahead. 01:05:29 - 01:05:36 Speaker 5: Yeah. This is my first class with Abhijit and this course. Are these recorded and shared with us, the sessions? 01:05:41 - 01:05:46 Dr Anand Jayaraman: Sorry, there was also someone else saying to the 2, Mona, is it? 01:05:46 - 01:06:01 Speaker 7: Yes, yes. Hi, Professor, and today is my first class and I'm from a human resource background. So I have no clue, no idea about AI, but this has been excellent class and thank you so much for Thank you. 01:06:02 - 01:06:58 Dr Anand Jayaraman: So 1 of the things that I'm going to do, I know I'm supposed to stay away from code. I, however, believe that your understanding gets better when you see code, right? And I'm going to be every time during this class, my former lectures, I'm going to start showing you code snippets. I want you to feel comfortable about it. I'm not going to ask you to look at right code right that's not needed I am not a programmer myself but seeing code right in your mind clarifies how are someone else going to write this right your managers right you sometimes are going to be overseeing projects and you will be able to look at the code and understand what's actually happening. 01:06:58 - 01:06:59 Dr Anand Jayaraman: Yeah, 01:07:00 - 01:07:01 Speaker 7: sure. 01:07:01 - 01:07:23 Dr Anand Jayaraman: Yeah, so I will show you code too. So but you don't need to know programming that I'll explain the code syntax and so on. And soon you will find yourself and I asked questions, you know, how exactly that you wonder what is the code piece you'll write, you will be able to articulate it easy. Yes, the lectures are recorded. Yeah, sorry. 01:07:23 - 01:07:42 Dr Anand Jayaraman: Okay, good. Now, let's talk about the neuron. So let me quickly remind you different things that we have talked about. We talked about the opinion that human brain is wonderful to replicate. Except that it's too big, too complicated for us to replicate. 01:07:42 - 01:08:04 Dr Anand Jayaraman: Then we asked the question, is it possible for thinking to happen with smaller brain and we all agree that it is possible. Intelligence can exist even in smaller brain. So then we said that, okay, how small a brain can it be for it to have intelligence? Is a brain with single neuronal cell. Would you call that as intelligent? 01:08:04 - 01:08:25 Dr Anand Jayaraman: That is the question that we are heading towards. And for that, we need to actually start talking about some biology. I talked about general information about human brain. I mentioned how many neurons are there and so on. And I talked about I got to this particular slide which gives you the structure of a single neuron. 01:08:25 - 01:08:54 Dr Anand Jayaraman: Right. OK. So here is a single neuron. And this is a picture that you have probably seen from your biology textbooks whenever you did, you know, middle school biology. If you don't remember this and if you were 1 of those fortunate people who didn't have to do biology at all, and now you're looking at it, you probably think it looks like an alien creature, right? 01:08:54 - 01:09:30 Dr Anand Jayaraman: You know, with all these tentacles and so on, right? Here is this, but this is the way a neuron looks. You have, you know, in our textbooks, when you study biology, they would have said that a cell, they would have given a schematic of cell, they said, this is a cell. And there is a portion of it called the nucleus and then there is mitochondria, there is cytoplasm and these other things that are there in the cell. But the neuronal cell looks, you know, as I said, bizarre, like positively alien-like. 01:09:32 - 01:09:53 Dr Anand Jayaraman: The elements of cell that you learned still are there. Here is the nucleus over there. So this is the main cell body. But these octopus that these tentacles like structures are called as dendrites. These are called as dendrites. 01:09:54 - 01:10:23 Dr Anand Jayaraman: And there are multiple dendrites that are there for a particular cell. In addition, there is this 1 particularly long dendrite like structure that is there. This is called as an axon. And again towards the end of the cell there are again those arms you know or tentacle like structures that are over there. Now these dendrites serve an important function and I'll talk about that. 01:10:23 - 01:10:54 Dr Anand Jayaraman: Now what neurons do, every single part of this neurons have a very important function. The way direction, what does neurons do? Neurons basically carry information in our body. It's carrying information and we know that neurons are key to decision making ability. So somewhere decision making is also happening. 01:10:55 - 01:11:35 Dr Anand Jayaraman: But clearly it's carrying signals. For example you've seen something images formed on your eye at the back of the your eye and from there that information is taken to your brain right neurons are actually playing a role over there right so neurons carry information the direction of the thing in which the signals are carried is like this, right? Basically, information is coming in from these, these dendrites over here are really your input sensors, so to speak, right? Information is coming in from there. All of this information is going inside the cell body. 01:11:36 - 01:12:01 Dr Anand Jayaraman: The cell body from there, this information is going there and the cell body is sending the output over there through this axon. And this in turn, this output is again sent through these other connections. Each of these connections in turn connects to other neurons. Right? This is part of a complicated circuit diagram. 01:12:01 - 01:12:39 Dr Anand Jayaraman: This is 1 component in that right. So these dendrites are your inputs. This axon is taking the combined output signal and these over here are outputs that are being sent for further processing into other neurons. So this is the structure of a single neuron. Now, when clearly this neuron we know is involved in decision making. 01:12:42 - 01:13:10 Dr Anand Jayaraman: Let me ask you a question. What is 5 times 6? 30. Clearly you did not do the computation now information was stored and you are retrieving the stored information, right? Where do you think this intelligence is? 01:13:10 - 01:13:15 Speaker 4: Stored in neurons. All the tables, whatever we learned are stored in some neurons. 01:13:16 - 01:13:18 Dr Anand Jayaraman: Here I'm showing you a single neuron. Neuroplastic. 01:13:19 - 01:13:19 Speaker 4: Nucleus. 01:13:19 - 01:13:20 Dr Anand Jayaraman: Sorry? 01:13:20 - 01:13:21 Speaker 4: Nucleus. 01:13:21 - 01:13:34 Dr Anand Jayaraman: Nucleus, right? Good guess. Nucleus. Turns out, this is our current understanding, right? The intelligence is really not in the nucleus. 01:13:36 - 01:14:05 Dr Anand Jayaraman: We believe that intelligence is in the connections between 2 neurons, right? There is other neuron from which that's coming here and that is what is the signal is coming from there and it's connecting over here. These connections where 1 neuron connects to another, these are called as synaptic connections. Right? The strength of the synaptic connections indicates intelligence. 01:14:06 - 01:14:46 Dr Anand Jayaraman: Why do we believe that? We believe that and this comes, this is something that comes from some set of experiments that were done in 1949. Donald had done this experiments on frog neurons from frog legs, right? What he found was that when a particular neuronal pathway is used again and again, is excited again and again, right? The signal traverses through that much more easily, faster and faster, right? 01:14:46 - 01:14:51 Dr Anand Jayaraman: First, let's take a step back. I'm talking about signal. What 01:14:56 - 01:14:56 Speaker 4: signal am I referring to? Input signal. 01:14:58 - 01:15:00 Dr Anand Jayaraman: What is the nature of this input signal? Can 01:15:01 - 01:15:05 Speaker 4: be a language here as you ask 5 into 6 right so 01:15:05 - 01:15:06 Speaker 8: when you're talking about 01:15:07 - 01:15:14 Dr Anand Jayaraman: language now you're talking about some conceptual level I'm talking about physical signal what physical signal is that electrical 01:15:17 - 01:15:17 Speaker 8: chemical 01:15:18 - 01:16:27 Dr Anand Jayaraman: correct right so in our body these are electrochemical signals right now when we are talking about when you did a basic physics, right, you're talking about a copper wire through which electrical signal travels, right, except here, it's not in when over there, when you're talking about electrical signal traveling through a copper wire, what is actually traveling electrons, right, electrons are actually moving. But when you're talking about signals in a human body these are electrochemical signals and these are mostly done through ions. Sodium calcium and potassium ions are responsible for connections or for transport of signals. This is why when for example you are doing a long distance run or cycling or you're working out a lot and your body starts to sweat, right? When you're sweating, your sweat has salts, right? 01:16:27 - 01:16:46 Dr Anand Jayaraman: What salts are these? Your body is leaving out potassium and sodium. The salts are coming out of your body. So there is a lack of salts in your body. When your body, the concentration of potassium and sodium ions drop, suddenly your signaling mechanism no longer works right. 01:16:47 - 01:17:30 Dr Anand Jayaraman: And hence you start to cramp up right which is why you start to drink electrolytes right so you're trying to maintain the balance of sodium and potassium and so then my aunt she was you know when she was getting old and she was like 85 approaching 90. Once in a while what happens is that you see somebody starts she stops recognizing people and starts saying something nonsensical. Most of the time she's fine. But once in a while this happens and people panic and then they take her to the hospital, to the doctor, like what's happening? And the doctor says, oh, well, it's electrolyte imbalance. 01:17:30 - 01:17:53 Dr Anand Jayaraman: And then they give her a saline drip and you know what an hour later she's fine right. These sodium and potassium ion concentrations are so key in our comprehension the way we think right. I don't know if you guys any of you have done the Grand Canyon hike. I remember when we went down the Grand Canyon. 1 of the things that was saying was this. 01:17:54 - 01:18:17 Dr Anand Jayaraman: Remember to hydrate a lot. And because people crazy things happen and you start losing your salts. Suddenly people start becoming delusional and they have been instances of people jumping off the cliff because they believe they can fly, right? All because lack of salts not being there, right? And 1 of the cures for it is very easy. 01:18:17 - 01:18:37 Dr Anand Jayaraman: This is a carry junk food, right? Carry chips and this thing because chips typically have a lot of salts. This is 1 time where chips are actually healthy, right? Make sure that you have enough salts to go through the client. So the sodium is how signals are actually traversing through these neurons. 01:18:38 - 01:18:39 Dr Anand Jayaraman: Yeah. Sorry, there was a question. 01:18:40 - 01:18:54 Speaker 8: I would like to understand where exactly is the neurotransmitter because it is, is it at the end of axon or is it a synaptical is it at the synaptical terminal where it gets where it converts the electrical signal into chemical signal right 01:18:54 - 01:19:14 Dr Anand Jayaraman: here here the the neurotransmitters that they are talking about are basically enabling the transport of the signals through these connections. Right. Now the way it works is this. So here is 1 neuron you are getting. I want you to imagine this like this 1 particular neuron. 01:19:14 - 01:19:30 Dr Anand Jayaraman: Yeah this is coming from the eye. So some signal is coming there. And some other portion of the eye, another signal is coming from there. And perhaps in the ears, some signal is coming there. All of these signals are coming in here and it's being attached over there from this 1 of these neurons. 01:19:31 - 01:19:59 Dr Anand Jayaraman: Now this connection is not a perfect electrical connection. It's an imperfect connection. Parts of the signal that's coming in it's modulated when it's coming in here. It gets modulated when it comes here. All of the signals reach over there into the middle of the neuron and when the signal threshold reaches some number, suddenly this neuron fires an output. 01:20:00 - 01:20:44 Dr Anand Jayaraman: The behavior of the neuron is non-linear it's not like when the signal increases by 2 percent the output is going to increase by 2 percent that's not the way it works right it's non-linear and so when it reaches a threshold the neuron fires and this new signal is like this signal now gets to this next set of neurons. Right? What we are going to do is we are going to try and create a mathematical model of a neuron to further, you know, better understand how a single neuron works. And then we use our understanding of a single neuron to actually try and build an artificial brain. Right? 01:20:44 - 01:20:51 Dr Anand Jayaraman: That's our plan. All good? Yes, sir. Yes. 01:20:51 - 01:20:59 Speaker 4: Professor, 1 quick question. Yes. So the neuron body you said about nucleus right. Is it just a storage storage media? 01:21:00 - 01:21:17 Dr Anand Jayaraman: Ah, it's not storing anything. So right now, think of it as you know, some electrical device that is that complicated electrical device. There is once enough amount of ions flow into it now it's going to fire and it's going to open up the dam and it's going to fire out. 01:21:17 - 01:21:35 Speaker 4: The reason why I ask the question is, sorry to interrupt, you said deep learning, if I'm going by the definition called deep learning, right? You said about input data, a large amount of input data and decisions, therefore it can build the rules on its own. So if I'm applying the principle I'm trying to get to. 01:21:35 - 01:21:49 Dr Anand Jayaraman: Yeah, not yet. Let's just keep that on hold for a bit. We are going to get there to deep learning, you know, in the third episode or fourth episode. We are in the first episode, right? We are still waiting for the hero to arrive. 01:21:49 - 01:21:52 Dr Anand Jayaraman: So, we'll come there. I'll hold on. 01:21:53 - 01:22:09 Speaker 9: A quick question. I mean, very related to what Shetty asked, although it is there. This data what coming up, right? Or which passes through, does it also have things like for instance the emotions and other things, right? I'm sure there would be a separate signal for that. 01:22:10 - 01:22:15 Speaker 9: Does it also get, I don't know, I mean how does the emotions or anything around with that gets stored? Very, 01:22:16 - 01:22:50 Dr Anand Jayaraman: very important question, right? So this is why there is so my daughter is in 11th grade and she's taking psychology, right? And it's interesting, right? I mean, I have looking at a textbook, it's fascinating. There is 1, the entire book is divided into multiple sections and the very first section was the biological approach to psychology. 01:22:51 - 01:23:06 Dr Anand Jayaraman: Right. And which all it's talking about is this hormone is there. When this hormone goes up, you're happy. When this hormone goes up, you're stressed and so on and so forth. Completely biological approach to understanding psychology. 01:23:06 - 01:23:58 Dr Anand Jayaraman: And then there is this next section which is cognitive approach to psychology, which is talking about more of thought process. Again, there is this particular way you are viewing the world and because of that you are having this reaction and so on, right? Basically, what we are trying to do is we are trying to understand, this is like the blind man and the elephant problem, right? We are trying to approach and understand this elephant in multiple ways right and so how exactly does emotions are triggered is the biological and you are the question that you're asking is essentially a biological approach to trying and understanding emotions right And people try to explain it through other ways too, right? Our goal here is much more narrow, right? 01:23:58 - 01:24:14 Dr Anand Jayaraman: All I want to do, right? I'm a business guy, I'm a capitalist, right? And for me, it's like, the most important outcome is like, you know, show me the money, right? Tell me here is a business problem, show me how to solve this business problem. Right? 01:24:14 - 01:24:39 Dr Anand Jayaraman: And that is where we are going, right? That's where we started off, right? Can I make better loan decisions? Can I make better fraud detection? That is all I care about right now right we haven't come to the emotions part at all in order to do that I've said I want to replicate a human brain then I came to smaller brain and now I'm trying to replicate a single neuron and I want to see can a single neuron help me in making my loan approval decisions better. 01:24:40 - 01:24:48 Dr Anand Jayaraman: Right. I'm not going to approach emotions right now. But we'll talk about that a little bit later. 01:24:49 - 01:24:50 Speaker 6: Can we have a break. 01:24:52 - 01:25:05 Dr Anand Jayaraman: Yes, absolutely. Thank you for that. They reminded me we have it's a 5 minutes to 8 and Indian time zone. I know you're most of you are not here. So let's take a 10 minute break. 01:25:05 - 01:25:09 Dr Anand Jayaraman: So please look at your watch. It's 10 minutes good enough. 01:25:09 - 01:25:10 Speaker 6: Yeah, I think it's good. Yeah. 01:25:12 - 01:25:15 Dr Anand Jayaraman: Fantastic. I think we're at the right place. So let's take a 10 minute break. 01:34:45 - 01:35:20 Speaker 10: Thank you for calling CVS Pharmacy located at 1950 Baghdad Road, corner of West New Hope Drive, Cedar Park, Texas. If you're a healthcare provider or calling from a doctor's office, press 2 now. Just so you know, our pharmacy is currently closed. If you are having a medical emergency, hang up and dial 911. For help locating a 24-hour pharmacy location please visit the CVS.com store locater while the store is closed there is something I can help you with here. 01:35:21 - 01:35:22 Dr Anand Jayaraman: Are we ready to get started? 01:35:22 - 01:35:23 Speaker 7: Yes sir. 01:35:30 - 01:35:48 Speaker 6: A syllabus in our last module where which was our Google map right we knew where we were so if you could give that right what is the content going to be covered in the 12 sessions would be great. Second thing is that if you can provide the slides earlier before the class I think that can help a lot of us looking at it and coming it will be attractive. 01:35:50 - 01:36:48 Dr Anand Jayaraman: So the first request regarding the schedule I definitely will provide that I thought it was already shared my apologies it wasn't shared now I had shared with the organizers and I assumed it would have reached you, but I apologize if it hasn't. The second request, however, is about slides is challenging and I prefer not doing it because usually I have lots of slides and they're all unorganized and depending on the questions, depending on the pace, I take slightly different paths. I cover everything, but slightly different paths over there. Right. And so I, you might be seeing my screen and are you seeing that I have 2 PowerPoints that are open over here. 01:36:50 - 01:37:14 Dr Anand Jayaraman: Depending on the questions, depending on this, I take slightly different paths to get there. So I prefer not doing that. And I want to, when the session is done, I'll clean up the, put together the slides that was presented to you and send that and and I'll move the slides other slides which I will go to the next lecture. So that's why I prefer not doing that. 01:37:14 - 01:37:21 Speaker 3: Professor I have a suggestion. Is it possible to include your speaker notes of the transcripts along the slides? 01:37:25 - 01:37:29 Dr Anand Jayaraman: Transcripts are I don't know whether it's even possible in this. 01:37:30 - 01:37:34 Speaker 3: We do have speech to text converters and 01:37:34 - 01:37:47 Dr Anand Jayaraman: kind of correct. So zoom would do that. So but right now we're using. Yeah. Right now we are doing the upgrade interface and I don't really know whether it has that or not. 01:37:49 - 01:37:51 Dr Anand Jayaraman: So I will need to check 01:37:51 - 01:38:06 Speaker 3: this challenge because I was late starter is trying to go through the lecture notes recorded mode and you have to spend 3 hours to get this. If you have the speaker notes for a specific slide, you can get that specific information. 01:38:07 - 01:38:26 Dr Anand Jayaraman: I understand. I can see why it's useful. But unfortunately I can't do anything about it. I apologize. I'll find out whether there's a way to do the transcript but unlikely is what I would think given that it's subgrads proprietary interface. 01:38:27 - 01:38:30 Speaker 6: No issue so we are like algorithms we give immediate feedback. 01:38:30 - 01:38:35 Speaker 3: Can we use some AI over there from AI interface for our AI class? 01:38:38 - 01:39:05 Dr Anand Jayaraman: It's actually quite amazing, right? I mean, how I completely understand your request. Thank you. Right. So, but this is a more of a philosophical and cultural thing the the request that you made right I mean like where voice to be able to get to a transcript and to be able to search, right? 01:39:05 - 01:39:40 Dr Anand Jayaraman: This was even 2 years ago, was realm of science fiction, right? To be able to do effectively. Products promised, but I've seen how bad it was. But today we do products, the consulting company that I consult with, we build products which do this. Our expectations now, suddenly what was considered science fiction 2 years back, now it's possible, right? 01:39:40 - 01:40:20 Dr Anand Jayaraman: So you can not just expect it to create a transcript, but also be able to allow you to search using concepts rather than exact words. And all of that, certainly now, our expectations have also increased because of what we have seen in the past year. I mean, we are truly witnessing magic around us. Anyways, let's go ahead and start with the class. I still haven't introduced the hero to you, a mathematical model of a neuron. 01:40:20 - 01:40:24 Dr Anand Jayaraman: And the hero is finally ready to come the last hour of the class. 01:40:24 - 01:40:28 Speaker 8: Professor, we expected the hero to come in the interval block, but it looks like not. 01:40:29 - 01:41:11 Dr Anand Jayaraman: Yes, yeah, exactly. An appropriate music, I have to play an appropriate music and I was searching for 1 and but yeah but finally the hero is ready to come and it will be sad if the entire first episode goes without the hero but no need to despair the hero is here finally. So what we are trying to do now is we have this rough understanding of how a biological neuron works. Clearly a cartoonish understanding of how a biological neuron works. This is the biological neuron. 01:41:12 - 01:41:23 Dr Anand Jayaraman: You have a cell body. Let me go ahead and enable my pen. OK. So this is your main cell body. And here are these dendrites. 01:41:23 - 01:42:02 Dr Anand Jayaraman: So these dendrites are where signals are coming in from other neurons, right? From different parts of the body or different parts of the brain. The signals are coming in and I already mentioned that the connection between the neuron that's connecting here and this our main hero, this particular neuron. That connection is an imperfect connection, right? Whatever signal is coming in from this neuron gets modulated and then it flows in over here, right? 01:42:02 - 01:42:19 Dr Anand Jayaraman: So the signals are flowing in over here. They get modulated and they go in here. And so imagine these ions are flowing in. And this ions are sort of accumulating over here. And this nucleus has a nonlinear response to these ions. 01:42:22 - 01:43:08 Dr Anand Jayaraman: So imagine it's like a dam, a mini dam that's there where water is flowing in and nothing happens, everything is in control. But once it reaches some threshold, suddenly the dam bursts and it releases everything. So this is a non-linear function and then the output once the neuron fires this output goes there this output is in turn again goes to other neurons over there. Now I mentioned to you that we believe the learning that happens when we are practicing some task, multiplication table or learning to drive a car or so on, right? You know how it is when you're trying to drive a car. 01:43:08 - 01:43:57 Dr Anand Jayaraman: Initially when you're trying to drive a car you often wonder like you know how do I take care of all of these things and drive, right? I need to look left. I need to look right. I need to keep an eye on the on the rear view mirror and I need to you know signal whether I'm going to turn or not and I excuse me I have the clutch. I have the brake and I have the accelerator and then you have music running as well like how do I pay attention to all of that right but then once you've learned driving it's suddenly it's all easy right you don't even need to think right this comes because of practice right you practice over and over again you have learned how to drive right now I mentioned to you learning is all about strengthening of the synopsis. 01:43:59 - 01:44:27 Dr Anand Jayaraman: When we learn a particular skill over and over again, that's equivalent to 1 of the synaptic strengths becoming stronger and stronger, allowing more easy propagation of signal, or stronger, more amplified propagation of signal. When some things are not being used, then those synapses get weaker. Right? So learning is all in this synaptic strength. Right? 01:44:27 - 01:44:53 Dr Anand Jayaraman: That's our, that's understanding comes from Donald Hemp. Right? Now, what I'm going to do is I'm going to try and mimic this neuron, this biological neuron. I'm going to try and make a computational model of a neuron. And for that, we want to abstract away unnecessary details and put together what is the bare essential. 01:44:54 - 01:45:33 Dr Anand Jayaraman: So here is our abstraction of this particular neuron, the cell body, you know, I'm going to remove away all of these alien looking structures and I'm going to represent the cell body with this circuit that's a cell body and it's got multiple connections that's coming in and I have those connections representing over here. So here is this 1 particular neuron which sent in the signal. Let's say it's sending some current through this ionic current that's flowing in. I'm going to represent that amount of current that's coming in from there as X naught. Amount of current coming from this neuron, I'm going to call it as X1. 01:45:33 - 01:45:52 Dr Anand Jayaraman: And this neuron, I'm going to call it as X1 and this neuron I'm going to call it as X2. These are all amount of current that's coming in. This is the signal that's coming in from different neurons. So here is the signal that's coming in and this signal is going to get attached. It's going to be sent in through this particular dendrite right and here is this connection. 01:45:54 - 01:46:28 Dr Anand Jayaraman: Now, I mentioned that this is not a perfect connection and so this signal is going to get modulated as it goes in here. Right? And that modulation and the synaptic strength I'm going to represent by this weight W not. So the original signal X not was coming in that gets transformed into W not times X not. So I wanted to imagine like maybe some current is coming yet and only half of the current flows in which case W not is 1 half. 01:46:29 - 01:47:27 Dr Anand Jayaraman: 1 half times x not is what is going in or 1 third it might be coming in. So the strength of the connection I'm representing with this W not. There are multiple inputs that are coming in. Each 1 of these inputs I'm representing as W not X not W 1 X 1 W 2 X 2 right these are all the modulated inputs that are coming in right now all of this current is flowing into the cell body right and here is my cell body so the all these currents are adding up and that is what I'm representing here by this symbol right summation of wi xi so w not x not plus w1 x1 plus w2 x2 that's the amount of current that's flowing into the cell body. So far clear? 01:47:27 - 01:47:35 Dr Anand Jayaraman: Yeah, I know I'm introducing mathematical symbols now slowly. I want you to feel comfortable about that. Right? I nothing complex happening yet. I want you to feel comfortable about that. 01:47:35 - 01:47:46 Dr Anand Jayaraman: Right? I nothing complex happening yet. I want you to feel comfortable about the mathematical symbols, right? Not be scared of those symbols. So that's the amount of current that's flowing in. 01:47:46 - 01:48:17 Dr Anand Jayaraman: Now, this cell already might have some base level of chemicals, right? That I'm going to call it as B, right? That's the previous existing base level of chemicals. So now I have the overall amount of chemicals that are there in the body ions that are there in the body is summation of WXI plus B. That's the amount of current that's going to be signal ions that are there inside the cell body. 01:48:18 - 01:48:44 Dr Anand Jayaraman: Right. Now, this is the output of this neuron. The output is going to depend on how much current is currently there, how much ions are currently there, right? So the output is a function of whatever was the total amount of chemicals inside. And that's what I'm representing here. 01:48:44 - 01:49:10 Dr Anand Jayaraman: F of the total amount of chemicals here that is the output. This function we call it as activation function. The exact details of if I increase to this level, the output is going to be this level. If I have if the level of ions are so much, that neuron won't trigger at all. All of that detail, right? 01:49:10 - 01:49:30 Dr Anand Jayaraman: That is the functional form. That's what I call this activation function, right? The function that describes how the neuron gets activated okay that's called as the activation function. So here what I have here is a mathematical model of the neuron any questions? 01:49:35 - 01:49:42 Speaker 4: This activation function is nothing but you said imagine a dam you open it flushing out the right is that similar. 01:49:43 - 01:49:59 Dr Anand Jayaraman: So it is exactly that so for a dam the activation function will look something like this. And in fact, that's what we're going to start off for the neuron too. The output of the dam. Okay, imagine the axis. This is the output level of output. 01:50:00 - 01:50:40 Dr Anand Jayaraman: Okay, and this is the on the x-axis I'm going to put the level of water okay as the level of then let me perhaps use a pen different colored pen okay so if the level of water is very 0 right The output from the dam is also going to continue remaining 0. But as the level of water increases, there is still going to be no output from the dam, right? The output of the dam will continue remaining 0. But beyond some threshold, suddenly the dam is going to burst. And that's going to be a huge amount of output that's going to go. 01:50:41 - 01:50:59 Dr Anand Jayaraman: Right? This is the threshold at which suddenly the dam bursts and the output from the bad dam has suddenly increased. This would respond, this would be an output activation function for a particular dam. Is that clear? What I'm doing? 01:50:59 - 01:51:00 Speaker 4: Clear, Professor. 01:51:01 - 01:51:20 Dr Anand Jayaraman: Right? This function, this description, real question, how much level of water and how much output is what is called as activation function. Yes. So is it acting like a binary function that activates and deactivates? So, yes, that is 1 option for the activation function. 01:51:21 - 01:52:10 Dr Anand Jayaraman: And in fact, when, yeah, when people design the first computational neuron, that is exactly the activation function they chose. They said if the level of current is less than some threshold, the output will be 0. When the level of current, summation of current inside is greater than some threshold, the output will be 1. Right? This is basically like a breaking down is how they thought of the activation function right this is how the early AI researchers and early AI researchers and early neuroscientists tried to model a neuron. 01:52:11 - 01:52:37 Dr Anand Jayaraman: This model of neuron, The AI researchers nicely called it as perceptron. You've got to give it to the AI researchers. The AI researchers are excellent marketers. They know how to name a product and what kind of product gets you funding. So they didn't call it as mathematical model of a neuron. 01:52:38 - 01:52:56 Dr Anand Jayaraman: They right away called it as perceptron. Why? Because this is a mathematical model of how we perceive things, right? I, with this model, have basically figured out how God designed humans, right? So to speak, I don't mean, I'm not trying to get religious here, right? 01:52:56 - 01:53:06 Dr Anand Jayaraman: So they, these guys were like phenomenal in coming up with an appropriate name for this mathematical model. They called it as perceptron. Sorry, there was a question. Go ahead. 01:53:08 - 01:53:21 Speaker 5: I have a question. So the data keeps coming in all the time, right? And then we got a threshold where the data will be sent out. But when the data got reduced, then will that be again back to 0? 01:53:21 - 01:53:33 Dr Anand Jayaraman: Correct. So if the summation of all of these inputs, this modulated inputs, WIXI is less than some threshold, the neuron will not do anything. If it's greater than some pressure, it will fire. 01:53:35 - 01:53:40 Speaker 5: So, it does calculation based on the point of time the data comes in. 01:53:40 - 01:54:11 Dr Anand Jayaraman: For yeah. So, let's keep that that that's the initial cartoon picture I started off with and then quickly change that in a bit but you're right so far right I don't we won't think of it as current constantly flowing instead that's fine that's fine that's a perfect model to think of perfect model to think of we'll make a modification slightly in a bit. So professor how do 01:54:11 - 01:54:15 Speaker 9: we get the threshold because threshold is again cannot be a constant there right? 01:54:17 - 01:55:03 Dr Anand Jayaraman: The threshold right now is a constant. But I'm making a model, right? I'm trying to understand this and keeping the simplest model I can think of for understanding a neuron. Right? And so this is the simple perceptron model our model is going to be this step function when the summation of inputs is below some value the value of that output is 0 and the summation of inputs is above some value the value of this function is going to be 1 right it's a binary output this neuron is either firing or not firing when it fires the value of the current is 1 when it's not firing the value of the current is 0. 01:55:04 - 01:55:15 Dr Anand Jayaraman: Right? This is the binary output. This is the perceptron. This is what is called as perceptron. Fine so far? 01:55:16 - 01:55:40 Dr Anand Jayaraman: I know this is a new concept, new way of thinking about it. We're very different than the machine learning models that you guys learned. But we'll just keep at it and things will become clear. We'll connect in the right places. So now this is perceptron. 01:55:41 - 01:56:20 Dr Anand Jayaraman: Now this kind of whenever we want to make mathematical models, right? Now 1 very, very important tool that we have in mathematics is calculus, right? We rely on this tool a lot. Calculus, what does it do? It talks about, I'm sure you remember that you had your curves and functions were all discussed when we learned calculus in our high school. 01:56:21 - 01:56:48 Dr Anand Jayaraman: And what calculus is all about is talking about when a curve is given, the rate of change, the rate of increase or the slope. The slope is what a derivative is, right? Dy by dx gives you the value of slope. It describes how steep a curve is or how flat a curve is, right? That is what the derivative function actually does, right? 01:56:48 - 01:57:34 Dr Anand Jayaraman: Being able to calculate derivatives allow us to understand the function and the rate of change of that particular function very well. And that is very important in lots and lots of actual business use cases, right? When I, for example, if I want to understand the, you know, what level of investment I should make in a particular business in order for my profit to be maximum. And I have this notion of a maximum. I know that as if too little investment, the business does not do well, right? 01:57:35 - 01:58:08 Dr Anand Jayaraman: And too much of an investment actually takes really long time for me to actually make back the money. I probably have to take a lot of loans for it. So eventually my business end up being unprofitable if the amount of investment is way too much more than what is actually needed. There is this notion of there is an optical optimal level of investment to in order to maximize my profit. Right to understand and figure out what is the right level of investment to maximize my profit, the tool that becomes extremely important is calculus. 01:58:09 - 01:58:40 Dr Anand Jayaraman: Calculus is a wonderful tool which allows us to do all kinds of magical things in business. I'm not talking about just pure math. So we, whenever we are dealing with a problem, we like to be able to use this tool set that we have developed, the calculus tool set. Why am I saying all of this? I'm saying all of this because the function that I have decided, the activation function that I've decided, right? 01:58:41 - 01:59:12 Dr Anand Jayaraman: This is a step function, right? This is a step function. This function has 0 value. And then suddenly at 1 point it jumps to value 1 this is a function that is a nondifferentiable function right Because at the value of the slope of this function is 0 here, slope of the function is 0 here. And at this 1 particular point, the slope is undefined. 01:59:16 - 01:59:53 Dr Anand Jayaraman: This is a non-differentiable function. We generally don't like to deal with non-differentiable functions right calculus is an extremely important tool and we want to be able to continue using this tool calculus So what we decide to do is we decide to modify this function and we say that you know we won't keep a strict step function instead we'll keep a function that is slowly transitioning from 0 to 1. Right. That kind of function is called a sigmoid function. Okay. 01:59:53 - 01:59:54 Dr Anand Jayaraman: That kind of function is called as a sigmoid function. 02:00:00 - 02:00:33 Dr Anand Jayaraman: So what we will be doing for our mathematical neuron, so the mathematical neuron that we had, I am going to say that the output of that, instead of it being a step function, I'm going to assume that it's a sigmoid function. So this is called as a sigmoid neuron, sigmoid neuron. All I've done is change the activation function, right? And the functional form of this activation function is this 1 over some complicated thing, don't worry about it. But this is the sigmoid neuron. 02:00:33 - 02:00:39 Dr Anand Jayaraman: This is the way we are going to model a mathematical neuron. Questions? 02:00:42 - 02:00:48 Speaker 2: Professor, what is the policy of step function over sigmoid function? Because you started with step function, 02:00:49 - 02:00:49 Dr Anand Jayaraman: and you said it's a 02:00:49 - 02:00:59 Speaker 2: steel, right? Straight line. And therefore, you want to gradually increase. Is this basically for learning purpose for the machine to learn. Correct 02:00:59 - 02:01:37 Dr Anand Jayaraman: yeah the thing is what happens is and again in real world nothing is that sudden There is a gradual change from 0 to 1. So that's what we are doing. This is, in a sense, introducing some amount of reality that the transition is going to be a little bit slow. And what it actually helps us do is the machine learning, when it learns, the learning actually becomes feasible if you have a differentiable function. I know suddenly the class has gotten heavy. 02:01:37 - 02:01:50 Dr Anand Jayaraman: I get it, right? You know, the price to pay for this, you know, and to be able to completely understand the complexities of the hero, I need to introduce a little bit of math. 02:01:50 - 02:01:51 Speaker 3: Wondering when. 02:01:53 - 02:01:54 Dr Anand Jayaraman: Sorry, go ahead. 02:01:58 - 02:02:01 Speaker 3: So when he was asking why you're calling him a hero, he should 02:02:01 - 02:02:19 Dr Anand Jayaraman: be aware. Yes, I mean, he was reading. You are going to see all the good things that's going to come out of it in a second. Sorry, I missed that 1 part. So what would be the key advantage of moving from a step function to the sigmoid? 02:02:19 - 02:02:31 Dr Anand Jayaraman: I mean, essentially, we are changing the weight slightly and moving it into a slightly smoother curve than a steep curve. Correct. So what would you do with that? I mean, I didn't. Good. 02:02:31 - 02:02:52 Dr Anand Jayaraman: Yeah. So 1 I'm going to say, let me rephrase it as this way. In real world, nothing is step function. If you're talking about a neuron, the neuron does not change from this state to that state suddenly, there is always a finite transition period. So that is what the sigmoid function is trying to do. 02:02:52 - 02:03:24 Dr Anand Jayaraman: It's showing you a finite transition. So that's what it's there. But the real reason why we do it is when you're training a machine learning algorithm, the way we train an algorithm is using calculus. We use a method called gradient descent. Was this discussed at all? 02:03:26 - 02:03:36 Dr Anand Jayaraman: Till now? Yes. Yeah. So the idea of gradient descent relies on calculus. Right. 02:03:36 - 02:04:14 Dr Anand Jayaraman: And so we want to be able to deal with functions that are differentiable. I will also introduce gradient descent again, I know that, you know, for many of us, math might not have been done in the recent past. It might have been a while before you did math. So when it comes to that, please, I will remind you again what is gradient descent and convey to you my way of understanding gradient descent. But for now, all I'm saying is I prefer dealing with smooth functions. 02:04:14 - 02:04:17 Dr Anand Jayaraman: That's all I'm saying. OK? 02:04:17 - 02:04:35 Speaker 2: Yeah, Professor, along with that, you said calculus. If you can give a real example and explain more in detail about calculus, like as you said, sales prediction. If I invest this much amount, how much I get profit, right? So with the calculus, right? So if you can give some examples and explain it, that will be really helpful. 02:04:36 - 02:04:39 Dr Anand Jayaraman: So you mean how do I solve that particular example? 02:04:40 - 02:04:44 Speaker 2: Any example where you apply calculus and show how to. 02:04:45 - 02:04:58 Dr Anand Jayaraman: Oh, I mean, the number of examples are, I have to say, infinite. I'm having time really struggling right now to think about which 1 to pick. 02:04:58 - 02:04:58 Speaker 3: OK, you 02:04:58 - 02:05:03 Dr Anand Jayaraman: know what, let me Pick 1 from my feed. OK, I have 02:05:03 - 02:05:12 Speaker 4: 1 example. The example you gave about the amount of investment, how will that relate to this curve compared to the step function and the sigma function? 02:05:13 - 02:06:05 Dr Anand Jayaraman: Oh, so here is the thing. So let's talk about this. So the way I am going to represent, let's say the x-axis is total amount of investment that I need to make, and this y-axis is profit. Now, you're starting a business, and you are looking at how much investment to actually make. Let us say, in order to actually, you're buying, I don't know, some, you're importing iPhones, and you're selling iPhones here for a profit. 02:06:06 - 02:06:46 Dr Anand Jayaraman: Now, the person from whom you're going to be importing iPhones, they expect you to pay up the money before they send you the product. Now, there is an option for you, you can actually take a loan, right? Not make any investment at all. If you don't make any investment at all, you're taking the whole amount in loan, and you need to pay interest on that particular loan. And it turns out that the interest is so high that if you, by the time the phones come, you pay for the shipping and everything, and then you sell, you still are not able to pay the investment, the loan amount back, and you haven't actually made any profit at all. 02:06:47 - 02:07:10 Dr Anand Jayaraman: Like if you take too much of a loan, the loan interest itself completely overwhelms you. So your profit is actually negative. If you don't put in any of your own cash, your profit is actually negative. But I suppose you start using some of your own cash over there, right? Then what happens is that as the level of investment increases, you have to pay less and less interest. 02:07:10 - 02:07:43 Dr Anand Jayaraman: And so the level of your loss actually reduces. At some level, there is a transition. It goes from being lossy, as a level of investment that you're able to afford increases, it starts to become profitable. But as you start putting more and more investment in it, they're effectively, essentially I'm saying buying more and more phones, you might end up actually crossing the market threshold of how many phones it can actuall