Data Analysis Lecture Notes PDF
Document Details
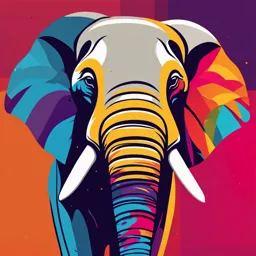
Uploaded by SuperbKazoo2870
2024
Tags
Summary
These lecture notes cover preliminary univariate analyses, describing nominal data, and interval-ratio variables including dispersion measures and detecting outliers. Additional topics include bivariate analysis utilizing Pearson correlation, Spearman's correlation, and Chi-Square. The document also discusses assumptions and interpretation for various statistical tests.
Full Transcript
25-12-2024 Lecture 3 : Preliminary univariate analyses Describing nominal data : Statistics Proficiency Analyze – Descriptive statistics – N Valid 30 Frequencies...
25-12-2024 Lecture 3 : Preliminary univariate analyses Describing nominal data : Statistics Proficiency Analyze – Descriptive statistics – N Valid 30 Frequencies Missing 0 Mean 69.6000 Move item into variable Std. Deviation 16.44342 Charts (Bar chart) – Continue Describing interval-ratio variables Mean : Describe central tendency Dispersion measures Coefficient of variation : Calculation method : (St deviation /Mean) *100 Detect Outliers Create a boxplot Chart Builder Double click on the boxplot Drag the variable 25-12-2024 Another method to detect outliers numrically is to transform all item scores to standardized scores All items with a Z score between 3 and -3 are outliers Descriptive Statistics – Descriptives – Tick Save standardized values as variables Lecture 4 : Bivariate Analysis : Pearson Correlation Correlation means that variation in the scores of one variable corresponds to variation in the scores of a second variable Pearson Product Moment Correlations Age Proficiency Correlation Age Pearson Correlation 1 -.890 ** Sig. (2-tailed).000 Pearson ® measures the N 30 30 ** Proficiency Pearson Correlation -.890 1 strength of a linear Sig. (2-tailed).000 relationship between two N 30 30 **. Correlation is significant at the 0.01 level (2-tailed). variables measured at interval-ratio P Value is considered significant if it is lower than.05 If there is correlation : Interpret : Direction and Strength Strength : Perfect 1 / Strong 0.9-0.7/Moderate 0.6- 0.4/Weak 0.3-0.1 / Zero 0 25-12-2024 Example of an Interpretation : H0 : There is no significant relationship between reflectivity and autonomy among high proficiency learners H1 : There is a significant relationship between reflectivity and autonomy among high proficiency learners The risk of incorrectly rejecting H0 is p=.04. Since the risk is below the.05 threshold , H0 is rejected. There is hence a significant positive moderate relationship r=0.51 between reflectivity and autonomy among high proficiency learners. Pearson ® Assumptions 1- Both variables measured at interval- ratio 2- Linear relationship between the two variables (Scatter plot) 3- No significant outliers (Zscore-Boxplot) 4- Both variables should be approximately normally distributed Normal Distribution : Skewness and Kutosis between -1 and 1 / Histogram/ QQ Plot Linearity : Scatterplot 25-12-2024 Lecture 5 : Bivariate Analysis (Spearman and Chi2) To determine the choice of the test,consider : - Number of variables (Univariate, bivariate, Multivariate) - Test objective (Correlate, Compare, predict) - Level of measurement (Ordinal, Nominal, Interval- ratio) + Test Assumptions Spearman : Non-parametric test Test Assumptions Both variables are measured at least at the ordinal level 1 There should be a monotonic relationship between the two 2 variables Checking monotonicity : Scatterplot 25-12-2024 Interpretation : H0 : There is no significant relationship between English and Maths marks H1 : There is a significant relationship between English and Maths marks The risk of incorrectly rejecting H0 is p=.035. Since the risk is below the.05 threshold , H0 is rejected. There is hence a significant positive moderate relationship r=0.669 between English and Maths marks How to run Spearman Rho on SPSS : Analyze – Correlate – Bivariate – Tick Spearman Spearman and kendall’s tau can be run if the assumptions are not met Chi2 Square Chi2 is a statistical test Correlation : Expected value less than 5 that measures the relationship P value = Continuity correction between two variables (Nominal) Strength : Phi2*2/ Crammer’s V larger than 2*2 25-12-2024 Test Assumptions Sample randomly selected 1 Independance of observation 2 A large Sample 3 No more than 20 percent of cells should have an expected count less than 5 3 Minimum expected count = or more than 1 Solution : Increase the sample size / Merging the categories 4 Interpretation H0 : There is no significant link between the use of haven’t got and don’t have forms of social class H1 : There is a significant link between the use of haven’t got and don’t have forms of social class The risk of incorrectly rejecting H0 is almost null (p=.001, X2 = 11.520, continuity correction) ;Since the risk is below the.05 threshold , H0 is rejected. There is hence a significant link between the use of haven’t got and don’t have forms of social class. 25-12-2024 Lecture 6 : Bivariate Analysis 3 : Comparison tests : Independant Sample t-test + Mann Whitney U Independant Sample t-test is used when we want to compare 2 groups of partcipants in terms of one-interval –ratio variable How to perform this test on SPSS ? Analyze – Compare means – Independant samples t-test 25-12-2024 Calculating effect size : Cohen’s d Effect size d Percentage of overlap small 0.2 85 medium 0.5 67 large 0.8 53 Test Assumptions The dependant variable should be measured at interval-ratio level 1 The independant variable should be measured at the nominal level and 2 should consist of two groups 3 Independance of observations No significant outliers 4 Dependant variable approximately normally distributed 5 Homogeneity of variance between the two groups (Levene's test) 6 In case assumptions are not met – Run Mann Whitney U test 25-12-2024 The dependant variable should be measured at the 1 ordinal level The independant variable should be measured at 2 the nominal level and should consist of 2 groups 3 Independance of observations The distribution of two groups has a similar shape 4 (relaxed) Interpretation :Independant Sample t-test H0 : There is no significant difference between early exposure learners and late exposure learners in terms of their r/l/w test scores H1 : There is a significant difference between early exposure learners and late exposure learners in terms of their r/l/w test scores The risk of incorrectly rejecting the null hypothesis (p=.011,t=2.578). Since the risk is below the threshold.05. H0 is rejected. Hence, there is a significant small difference between early and late exposure learners (d=.387) with early exposed learners producing better scores (X- = 54.7 more than Y-= 49.29) 25-12-2024 2- Paired Sample t-test + Wilcoxon Pretest Intervention Post test A paired samples t-test is used with two groups with data collected from them before and after (or two different conditions) Test Assumptions The dependant variable should be measured at 1 the interval-ratio The independant variable should consist of 2 2 groups with the same subjects 3 No significant outliers in the differences Normal distribution of differences 4 25-12-2024 Interpretation H0 : There is no significant difference between prod vocab in the pre- test and prod vocab in the post test H0 : There is a significant difference between prod vocab in the pre- test and prod vocab in the post test The risk of incorrectly rejecting the null hypothesis (p=.000,t=55.170). Since the risk is below the threshold.05. H0 is rejected. Hence, there is a significant large difference between prod vocab pre-test and prod vocab post-test (d= 2.01) with students performing better in the post- test (X- = 43.11 more than Y-= 30.65) Non parametric equivalent : Wilcoxon Measurement level of DV is at least ordinal 1 Nominal IV consisting of 2 paired groups (1 2 group *2) The distribution of two groups is symmetrical 3 (Relaxed) 25-12-2024 Lecture 8 : Bivariate Analysis (ANOVA + Kruskal Wallis) Multiple comparison A method to compare several groups (2 or more) Two types of variance : Between + within F= Variability between groups / Variability within groups Test Assumptions DV should be measured at interval-ratio 1 2 IV should be measured at the nominal level and consists of 2 or more groups 3 Independance of Observations 4 No significant outliers 5 Dependant variable approximately normally distributed 6 Homogeneity of varainces between the groups 25-12-2024 Running One-Way ANOVA If variances are equal : Levene P is more than.05 = Run ANOVA- Tukey (post hoc) Not equal : Levene P is less than.05 = Run Welsh’s ANOVA- Games’ Howel (post hoc) If assumptions are not met : Kruskall Wallis – Dun Bunforroni (Post hoc) Test of Homogeneity of Variances Words Levene Statistic df1 df2 Sig. ,077 2 39 ,926 ANOVA Words Sum of Squares df Mean Square F Sig. Between Groups 17354,619 2 8677,310 7,654 ,002 Within Groups 44213,857 39 1133,689 Total 61568,476 41 Interpretation 1 H0 : There is no significant difference in the mean scores of syntactic complexity across the three planning groups H1 : There is a significant difference in the mean scores of syntactic complexity across the three planning groups 25-12-2024 The risk of incorrectly rejecting the null hypothesis (p=.057(F=3.085) is above the threshold of.05. Therefore , H0 is accepted and it is possible to conclude that there is no significant difference in syntactic complexity across the three groups. Interpretation 2 H0 : There is no significant difference in the mean scores of syllables across the three planning groups H1 : There is a significant difference in the mean scores of syllables across the three planning groups The risk of incorrectly rejecting the null hypothesis (p=.005(F=6.098) is below the threshold of.05. Therefore , H0 is rejected and it is possible to conclude that there is a significant difference in terms of syllables across the three groups.A tukey HSD test revealed that the pre-task planning group produced largely more syllables than the no planning group (p=.004). Whereas, no significant differences were found between the no planning and the online planning groups (p=.126) and between the pre-task and online groups (p=.296) Lecture 9 : Multivariate Analysis (EFA+PCA) It is used for measuring a concept that cannot be measured directly (Latent variable) The objective is to reduce a large number of items into smaller factors /dimensions for later analysis 25-12-2024 Ex : Questionnaires, tests….. 25-12-2024 Multiple variables measured at scale level 1 Linear relationship between all variables (relaxed) 2 Large enough sample (from 5 to 10 per item+ KMO) 3 The data should be suitable for reduction ; Sufficient correlations 4 exist (Barlett's test of sphericity) No significant outliers 5 Stepof Running / Interpreting EFA Checking multicolinearity Det more than Initial.00001 Minimum Correlations between variables Checks (Barlett's test of sphericity must be significant Sampling adequacy Use KMO Factor Extraction criteria : EIgenvalue more than 1+ Cumulative of variance Main (Merenda) should be at least 50 Percent + Scree plot inflection Analysis Factor rotation methods: Direct oblimin or Varimax Post Reliability analysis Cronbach's a (alpha) Analysis Interpretation The fear of computers, fear of statistics and fear of maths subscales of the SAQ all had high reliabilities, all Cronbach’s a=0.82. However, the fear of negative peer evaluation subscale had low reliability Cronbach’s a=0.57 25-12-2024 Lecture 10 : Multiple Regression Analysis Effect of independant (Predictor) variable on a (response) dependant variable Predict the DV based on a set of predictor variables Correlation vs Regression Correlation Regression Relationship between Effect of IVs on DVs variables Variables are Variables cannot be interchangeable interchanged Variables move together Cause and Effect 25-12-2024 Test Assumptions MLR 1 Sample size 30 per IV The DV and IVs measured at interval-ratio 2 Linear relationship between the DV and each IV 3 IVs should not be highly correlated (No 4 multicollinearity) because it leads to inflation Residuals Assumptions No significant outliers in the residuals (St 1 residuals+ Cook's distance less than 1) Approximate normal distribution of 2 residuals (PP PLot) Homoscedasity (Homogenous 3 distribution of residuals) (Scatterplot) 25-12-2024 Interpretation : 1/ Does the model explain any variation in the DV ? 2/ How much varaibility does the model explain 3/ What IVs significantly predict the DV sig (Adjusted r Squared) 4/ How much variability in the DV does each IV explain( B coefficient )