Data Science Overview | Applications, Life Cycle | PDF Guide
Document Details
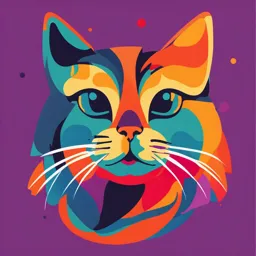
Uploaded by LargeCapacityMaxwell5730
Tags
Summary
This document provides an overview of data science, covering essential concepts, roles, and applications across various industries such as healthcare and finance. The document defines key terms and explores the data science life cycle, ethics, and emerging trends. It serves as an introduction to the field including machine learning and data analysis techniques.
Full Transcript
**UNIT I** Data Science Overview, Evolution of Data Science, Data Science Roles, Tools for Data Science, Applications of Data Science Data Science Process Overview, Defining Goals, Retrieving Data, Data Preparation, Data Exploration, Data Modeling, Presentation Data Science Ethics, Doing good Dat...
**UNIT I** Data Science Overview, Evolution of Data Science, Data Science Roles, Tools for Data Science, Applications of Data Science Data Science Process Overview, Defining Goals, Retrieving Data, Data Preparation, Data Exploration, Data Modeling, Presentation Data Science Ethics, Doing good Data Science, Owners of the Data, Valuing different aspects of Privacy, Getting Informed Consent, The Five Cs of Data Science, Diversity, Inclusion, Future Trends in Data Science. **DATA SCIENCE OVERVIEW** **What is Data Science?** ***Data science is the science of analyzing raw data using statistics and machine learning techniques with the purpose of drawing conclusions about that information*** [Data science](https://www.geeksforgeeks.org/what-is-data-science/) is an interdisciplinary field that focuses on extracting knowledge and insights from structured and unstructured data using various scientific methods, processes, algorithms, and systems. Simply put, it\'s the process of turning raw data into valuable information. It involves using statistics, computer science, and knowledge of the specific area you\'re working in. Think of it as detective work where you use data to uncover patterns, make predictions, and inform decision-making. **Key Concepts and Terminologies** 1. **Big Data:** [Big data](https://www.geeksforgeeks.org/what-is-big-data/) refers to extremely large data setsthata cannot be managed or processed using traditional data processing techniques, It encompasses the three ***VS: Volume, Velocity, and Varuiety***. 1. **Machine Learning :**[ Machine Learning](https://www.geeksforgeeks.org/ml-machine-learning/) is a subset of artificial intelligence that enables systems to learn from data and improve performance without explicit programming. It involves algorithms such as regression, classification and clustering. 1. **Artificial Intelligence:** [Artificial intelligence](https://www.geeksforgeeks.org/artificial-intelligence-an-introduction/) (AI) is the broader concept of machines being able to carry out tasks in a way that we would consider \"smart.\" AI includes machine learning, natural language processing, and robotics. 1. **Data Mining:** [Data mining](https://www.geeksforgeeks.org/data-mining/) involves discovering patterns and knowledge from large amounts of data. It uses methods at the intersection of machine learning, statistics, and database systems. 1. **Predictive Analytics**: Predictive analytics uses historical data to predict future outcomes. It involves statistical techniques, machine learning algorithms, and data mining. - **Statistics**: The use of statistics for data analysis dates back to 800 AD with the work of Iraqi mathematician Al Kindi. - **Relational databases**: In the 1970s and 1980s, relational databases and SQL (Structured Query Language) allowed for more efficient data storage and retrieval. - **Business intelligence**: Companies began using data to inform their decision-making processes. - **Machine learning** - **Deep learning** - **Cloud computing** - **Data visualization** - **Open-source tools** - **Data analyst**: Collects, cleans, and aggregates data. They design reports, data models, and visualizations. - **Business intelligence analyst**: Builds and updates reports and dashboards. - **AI engineer**: Creates algorithms and models that integrate machine learning and artificial intelligence. - **Data scientist**: Uses visualization to detect outliers, validate model assumptions, and identify correlations. - **Data scientist**: Writes computer programs and analyzes large datasets. They use programming languages like Java, R, Python, and SQL. - **Database administrator**: Manages an organization\'s database to ensure data security, user access, and efficient functioning. - **Data scientist and software engineer**: Collaborate to create new capabilities for analyzing and processing data. - **Data scientist**: Uses statistical techniques and data visualization tools to identify patterns and gain insights from data. **APPLICATIONS OF DATA SCIENCE** Data Science plays a crucial role in transforming raw data into actionable insights. Its importance lies in its ability to help organizations make informed decisions, predict trends and improve operational efficiency. - **Healthcare:** Improving patient care, predicting disease outbreaks, and optimizing treatment plans. - **Finance:** Fraud detection, risk management, and algorithmic trading. - **Marketing:** Personalized marketing strategies, customer segmentation, and sentiment analysis. - **E-commerce:** Recommendation systems, inventory management, and sales forecasting. - **Transportation:** Route optimization, predictive maintenance, and autonomous driving. **DATA SCIENCE LIFE-CYCLE** 1. **Data Collection:** The first step in the data science process involves gathering data from various sources, such as databases, APIs, web scraping, and sensors. The quality and quantity of data collected significantly impact the subsequent stages of the process. 1. **Data Cleaning: **Data cleaning, or data preprocessing, involves identifying and correcting errors, handling missing values, and transforming data into a suitable format for analysis. This step ensures the reliability and accuracy of the data. 1. **Data Analysis: **[Data analysis](https://www.geeksforgeeks.org/what-is-data-analysis/) involves applying statistical and computational techniques to explore and understand the data. This step may include descriptive statistics, correlation analysis, and hypothesis testing to uncover patterns and relationships. 1. **Data Visualization: **[Data visualization](https://www.geeksforgeeks.org/data-visualization-and-its-importance/) is the graphical representation of data, making it easier to identify trends and insights. Tools like Matplotlib and Seaborn are commonly used to create visualizations such as bar charts, histograms, and scatter plots. 1. **Data Interpretation: **[Data interpretation](https://www.geeksforgeeks.org/data-interpretation-questions-aptitude/) involves deriving meaningful conclusions from the analysis and visualization results. It requires domain knowledge. **ESSENTIAL TOOLS AND TECHNOLOGIES:** **Programming Languages:** - [**Python**:](https://www.geeksforgeeks.org/history-of-python/) Widely used for its simplicity and extensive libraries for data science. - **[R](https://www.geeksforgeeks.org/r-programming-language-introduction/) : **Popular for statistical analysis and visulaization. **Data Analysis Tools:** - **[Pandas](https://www.geeksforgeeks.org/python-pandas-series/):** A Python library for data manipulation and analysis. - **[NumPy](https://www.geeksforgeeks.org/python-numpy/):** A Python library for numerical computations. **Machine Learning Libraries:** - **Scikit-Learn:** A Python library for machine learning, providing simple and efficient tools for data mining and data analysis. - **[TensorFlow](https://www.geeksforgeeks.org/introduction-to-tensorflow/):** An open-source library for numerical computation and machine learning. **Visulaization Tools:** - **[Matplotlib](https://www.geeksforgeeks.org/python-introduction-matplotlib/):** A plotting library for creating static, interactive, and animated visualizations. - [**Seaborn:**](https://www.geeksforgeeks.org/introduction-to-seaborn-python/) A Python visualization library based on Matplotlib, providing a high-level interface for drawing attractive statistical graphics. **Database Mangement Systems:** - **[SQL](https://www.geeksforgeeks.org/sql-tutorial/): **A language for managing and querying relational databases. - **[NoSQL](https://www.geeksforgeeks.org/introduction-to-nosql/):** Non-relational databases like MongoDB, designed for large-scale data storage and flexible data models. 1. **Data Privacy and Security**: Ensuring data is protected from unauthorized access and misuse. 1. **Handling Big Data:** Managing and processing large volumes of data effectively. 1. **Model Interpretability:** Making complex models understandable to non-experts. 1. **Keeping Up with Evolving Technologies: **Continuously learning and adapting to new tools and methods. 1. **AI and Machine Learning Advancements: **Expect more advanced algorithms and greater computing power. 1. **Increased Automation: **Tools that automate data science workflows, making it easier for everyone to use. 1. **Ethical Considerations and Regulations:** Developing guidelines to ensure data is used responsibly and fairly. 1. **Integration with IoT and Edge Computing: **Analyzing data from IoT devices in real-time, enabling smart cities and industrial automation. - **Privacy: **It means respecting an individual\'s data with confidentiality and consent. - **Transparency: **Communicating how data is collected, processed, and used, So it will maintain transparency. - **Fairness and Bias: **Ensuring fairness in data-driven processes and addressing biases that may arise in algorithms, preventing discrimination against certain groups. - **Accountability: **Holding individuals and organizations accountable for their actions and decisions based on data. - **Security: **Implementing robust security measures sensitive data and protects them from unauthorized access and breaches. - **Data Quality: **Ensures the accuracy of the data , completeness and the reliability of the data to prevent any misinformation. - **Protects privacy**: Informed consent protects participants\' privacy rights by ensuring they\'re aware of how their data will be used. - **Builds trust**: Informed consent helps build trust between researchers and participants. - **Ensures ethical research**: Informed consent is a requirement for ethical research and helps ensure that research is conducted responsibly. - The purpose of the research - What data will be collected - How the data will be used - How the data will be stored and shared - How the participant\'s anonymity will be protected - The participant\'s right to withdraw from the research - Informed consent is required for all research that involves human participants. - It\'s especially important for research that involves sensitive personal data. - State privacy laws in the USA in states including Montana Consumer Data Privacy Act, Florida Digital Bill of Rights, Texas Data Privacy and Security Act, Oregon Consumer Privacy Act, and Delaware Personal Data Privacy Act. - In 2024, Canada introduced the Consumer Privacy Protection Act (CPPA), the Personal Information and Data Protection Tribunal Act, and the Artificial Intelligence and Data Act (AIDA). You can expect enhanced individual control over personal data and more substantial penalties for non-compliance from these acts. -  In the EU, an ePrivacy Regulation (ePR) finalized in 2024 establishes regulations on cookie usage and apps like WhatsApp and Facebook Messenger. -  AI regulation is entering a pivotal phase in 2025, with the long-awaited AI Act, which is a general EU legislation that brings a category-based approach to different types of artificial intelligence. - Digital Services Act (DSA) is an upcoming EU regulation that defines legal and harmful content that can be removed from digital platforms. - **Data democratization** - **Explainable artificial intelligence** - **Data unification** - **Graph analytics** - **Large language models** - **Data-driven consumer experience** - **Adversarial machine learning** - **Data fabric** **Real-world Applications of Data Science** **1. In Search Engines** The most useful application of Data Science is Search Engines. As we know when we want to search for something on the internet, we mostly use Search engines like Google, Yahoo, DuckDuckGo and Bing, etc. So Data Science is used to get Searches faster. **For Example, **When we search for something suppose "Data Structure and algorithm courses " then at that time on Internet Explorer we get the first link of GeeksforGeeks Courses. This happens because the GeeksforGeeks website is visited most in order to get information regarding Data Structure courses and Computer related subjects. So this analysis is done using Data Science, and we get the Topmost visited Web Links. **2. In Transport** Data Science is also entered in real-time such as the Transport field like Driverless Cars. With the help of Driverless Cars, it is easy to reduce the number of Accidents. **For Example, **In Driverless Cars the training data is fed into the algorithm and with the help of Data Science techniques, the Data is analyzed like what as the speed limit in highways, Busy Streets, Narrow Roads, etc. And how to handle different situations while driving etc. **3. In Finance** Data Science plays a key role in Financial Industries. Financial Industries always have an issue of fraud and risk of losses. Thus, Financial Industries needs to automate risk of loss analysis in order to carry out strategic decisions for the company. Also, Financial Industries uses Data Science Analytics tools in order to predict the future. It allows the companies to predict customer lifetime value and their stock market moves. **For Example, **In Stock Market, Data Science is the main part. In the Stock Market, Data Science is used to examine past behavior with past data and their goal is to examine the future outcome. Data is analyzed in such a way that it makes it possible to predict future stock prices over a set timetable. **4. In E-Commerce** E-Commerce Websites like Amazon, Flipkart, etc. uses data Science to make a better user experience with personalized recommendations. **For Example, **When we search for something on the E-commerce websites we get suggestions similar to choices according to our past data and also we get recommendations according to most buy the product, most rated, most searched, etc. This is all done with the help of Data Science. **5. In Health Care** In the Healthcare Industry data science act as a boon. Data Science is used for: - Detecting Tumor. - Drug discoveries. - Medical Image Analysis. - Virtual Medical Bots. - Genetics and Genomics. - Predictive Modeling for Diagnosis etc. **6. Image Recognition** Currently, Data Science is also used in Image Recognition. **For Example, **When we upload our image with our friend on Facebook, Facebook gives suggestions Tagging who is in the picture. This is done with the help of machine learning and Data Science. When an Image is Recognized, the data analysis is done on one's Facebook friends and after analysis, if the faces which are present in the picture matched with someone else profile then Facebook suggests us auto-tagging.  **7. Targeting Recommendation** Targeting Recommendation is the most important application of Data Science. Whatever the user searches on the Internet, he/she will see numerous posts everywhere. This can be explained properly with an example: Suppose I want a mobile phone, so I just Google search it and after that, I changed my mind to buy offline. In Real -World Data Science helps those companies who are paying for Advertisements for their mobile. So everywhere on the internet in the social media, in the websites, in the apps everywhere I will see the recommendation of that mobile phone which I searched for. So this will force me to buy online. **8. Airline Routing Planning** With the help of Data Science, Airline Sector is also growing like with the help of it, it becomes easy to predict flight delays. It also helps to decide whether to directly land into the destination or take a halt in between like a flight can have a direct route from Delhi to the U.S.A or it can halt in between after that reach at the destination. **9. Data Science in Gaming** In most of the games where a user will play with an opponent i.e. a Computer Opponent, data science concepts are used with machine learning where with the help of past data the Computer will improve its performance. There are many games like Chess, EA Sports, etc. will use Data Science concepts. **10. Medicine and Drug Development** The process of creating medicine is very difficult and time-consuming and has to be done with full disciplined because it is a matter of Someone's life. Without Data Science, it takes lots of time, resources, and finance or developing new Medicine or drug but with the help of Data Science, it becomes easy because the prediction of success rate can be easily determined based on biological data or factors. The algorithms based on data science will forecast how this will react to the human body without lab experiments. **11. In Delivery Logistics** Various Logistics companies like DHL, FedEx, etc. make use of Data Science. Data Science helps these companies to find the best route for the Shipment of their Products, the best time suited for delivery, the best mode of transport to reach the destination, etc. **12. Autocomplete** AutoComplete feature is an important part of Data Science where the user will get the facility to just type a few letters or words, and he will get the feature of auto-completing the line. In Google Mail, when we are writing formal mail to someone so at that time data science concept of Autocomplete feature is used where he/she is an efficient choice to auto-complete the whole line.  Also in Search Engines in social media, in various apps, AutoComplete feature is widely used.