Data Preparation for Exploration 1 PDF
Document Details
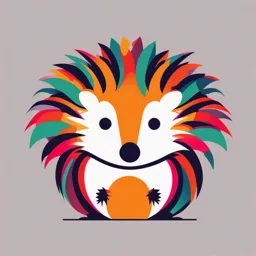
Uploaded by ConstructiveVenus3071
Tags
Summary
This is a presentation on data preparation for exploration, covering topics such as data collection factors for making decisions, types of bias, methods for assessing reliability, and data organization and cleaning best practices, including considerations of sample size and how databases function.
Full Transcript
Data Preparation for Exploration Session 1 Agenda Recap Data Collection Factors for Making Decisions Differentiate Between Biased and Unbiased Data Database Types, Functions, and Components Data Organization and Cleaning Best Practices...
Data Preparation for Exploration Session 1 Agenda Recap Data Collection Factors for Making Decisions Differentiate Between Biased and Unbiased Data Database Types, Functions, and Components Data Organization and Cleaning Best Practices 2 Data Collection Factors for Making Decisions Data collection is a critical step in the decision-making process. It enables organizations to gather information, analyze trends, and make informed choices. Proper data collection ensures the accuracy, relevance, and completeness of the data used for analysis. 3 Data Collection Factors for Making Decisions 1- Data Source Reliability: The reliability of data sources significantly impacts decision-making. Reliable sources ensure the accuracy and validity of collected data. Real-World Example: Decisions based on reliable patient data from well-established medical institutions in the healthcare industry have led to successful treatment outcomes. In contrast, reliance on unverified online sources has resulted in misinformation and patient harm. Methods for Assessing Reliability: Check the credibility of the source, perform peer reviews, and cross-reference data with other reliable sources. 4 Data Collection Factors for Making Decisions Data Relevance: Collecting relevant data is crucial for making informed decisions. Irrelevant data can lead to biased or inaccurate conclusions. Real-World Example: In marketing, using customer data from the relevant demographic has led to effective ad campaigns, while using irrelevant data from unrelated demographics resulted in wasted resources. Criteria for Determining Relevance: Alignment with research objectives, context-specific relevance, and up-to-date information. 5 Data Collection Factors for Making Decisions Sample Size: The size of the sample directly influences the reliability of insights drawn from data. Larger sample sizes tend to provide more accurate representations of populations. Real-World Example: In pharmaceutical trials, a larger sample size ensures that the drug's effects are accurately captured, minimizing the risk of incorrect conclusions. Implications for Decision-Making: Trade-offs between large and small sample sizes, including cost, time, 6 and accuracy. Large Sample Size Larger samples tend to yield more precise estimates of the population parameters. Larger samples reduce the effect of random fluctuations in the data, narrowing the margin of error around the estimated values. Data Collection Factors Case Studies Example 1: Market Research Scenario: A company conducts market research using reputable sources versus unreliable sources. Outcome: The company's decision-making process and market strategy differ significantly based on the reliability of the data. 8 Data Collection Factors Case Studies Example 2: Healthcare Analysis Scenario: Hospital A collects patient data from a small sample size, while Hospital B uses a larger sample size. Outcome: Hospital B's decisions regarding patient care and resource allocation are more informed due to the larger sample size. 9 Differentiating Between Biased and Unbiased Data Bias in data refers to systematic errors or distortions in the collection, analysis, interpretation, and presentation of data. These biases can significantly impact decision-making processes, leading to inaccurate conclusions and flawed strategies. 10 Types of Bias Selection Bias: Selection bias occurs when certain individuals or groups are systematically excluded or overrepresented in the data. It affects the representativeness of the sample and can lead to misleading results. Example: In a survey about consumer preferences, if only urban residents are included, the data may not accurately represent rural consumers. Mitigation Strategies: Random sampling, stratified sampling, and ensuring representative sample selection. 11 Selection Bias 12 Types of Bias Measurement Bias: Measurement bias arises from errors or inaccuracies in the measurement process. It can result from faulty instruments, biased observers, or inconsistent measurement techniques. Example: In clinical trials, if a faulty thermometer is used, it might consistently record incorrect temperatures, skewing the results. Mitigation Strategies: Calibration of instruments, training observers, and using standardized measurement techniques. 13 Types of Bias Reporting Bias: Reporting bias occurs when there is a tendency to selectively report certain information while omitting others, skewing the perception of reality. Example: Media reports may selectively focus on negative aspects of a political event, ignoring positive outcomes. 14 Types of Bias Confirmation Bias: Confirmation bias refers to the tendency to search for, interpret, and favor information that confirms pre- existing beliefs or hypotheses, leading to a biased interpretation of data. Example: A researcher who believes a new drug is effective may unconsciously emphasize positive results while downplaying negative 15 Hands-On Exercises on Bias Types Exercise 1: Identify Biases in Sample Datasets: Objective: Analyze provided datasets to identify different types of biases, such as selection bias, measurement bias, and other biases (e.g., reporting bias, confirmation bias). 16 Hands-On Exercises on Bias Types Exercise 1: Identify Biases in Sample Datasets: Survey Data on Consumer Preferences (Dataset 1): Data collected from 500 participants across different regions. Participants include 400 urban residents and 100 rural residents. Questions cover product preferences, purchase Purchase_Frequenc Region Preference_A Preference_B Brand_Loyalty frequency, and brand loyalty. y Urban 1 0 High 1 Urban 0 1 Low 0 Rural 1 0 Medium 1 Urban 1 0 High 1 Urban 0 1 Low 0 Rural 0 1 Medium 0 17 Hands-On Exercises on Bias Types Exercise 1: Identify Biases in Sample Datasets: Clinical Trial Data (Dataset 2): Data from a trial involving 200 patients testing a new drug. Measurements include blood pressure readings taken using different devices across different centers. Some data points show significant variance due to Patient_ID equipment malfunction. Device_Used BP_Reading Center 1 Device_A 120/80 Center_1 2 Device_B 130/90 Center_2 3 Device_C 140/95 Center_1 4 Device_A 125/85 Center_2 5 Device_C 145/100 Center_3 18 Hands-On Exercises on Bias Types Exercise 1: Identify Biases in Sample Datasets: Employee Performance Review Data (Dataset 3): Performance scores for 150 employees across different departments. Data includes ratings from two managers, with notable differences in ratings. Some managers only rated employees they directly Employee_IDsupervised, leaving othersManager_Rating_1 Department unrated. Manager_Rating_2 1 Sales 4 2 HR 3 3 IT 5 4 4 Marketing 3 5 Sales 5 19 Hands-On Exercises on Bias Types Exercise 1: Identify Biases in Sample Datasets – Solution: Dataset 1 (Consumer Preferences): Selection bias due to the overrepresentation of urban residents, which could skew the results in favor of urban preferences. 20 Hands-On Exercises on Bias Types Exercise 1: Identify Biases in Sample Datasets – Solution: Dataset 2 (Clinical Trial): Measurement bias due to inconsistent readings across different devices, possibly leading to unreliable conclusions. 21 Hands-On Exercises on Bias Types Exercise 1: Identify Biases in Sample Datasets – Solution: Dataset 3 (Employee Performance Review): Both selection and reporting biases. Selection bias is evident where some employees are not rated, and reporting bias where ratings may be influenced by the direct relationship between the manager and the employee. 22 Hands-On Exercises on Bias Types Exercise 2: Mitigating Biases: Objective: Using the biases identified in Exercise 1 propose methods to mitigate these biases. 23 Hands-On Exercises on Bias Types 1- Mitigating Selection Bias: Dataset 1 (Consumer Preferences): Proposed Solution: Ensure a balanced sample by increasing the number of rural participants or using stratified sampling to equally represent different regions. Impact: A more balanced representation of the population will lead to more generalizable results and insights. 24 Hands-On Exercises on Bias Types 1- Mitigating Selection Bias: Dataset 3 (Employee Performance Review): Proposed Solution: Ensure all employees are rated by implementing a system where each employee is evaluated by at least one manager. Alternatively, use peer reviews to complement managerial assessments. Impact: More comprehensive and fair performance reviews across all employees, reducing bias in performance evaluation. 25 Hands-On Exercises on Bias Types 2- Mitigating Measurement Bias: Dataset 2 (Clinical Trial): Proposed Solution: Standardize the equipment used across all trial centers or calibrate the existing devices to ensure consistency in measurements. Training staff on proper measurement techniques could also help reduce errors. Impact: More reliable and accurate data, leading to valid conclusions about the drug's effectiveness. 26 Hands-On Exercises on Bias Types 2- Mitigating Measurement Bias: Dataset 3 (Employee Performance Review): Proposed Solution: Implement a review system that encourages managers to rate all employees, possibly through anonymized evaluations or rotating managers to reduce personal bias. Impact: A more objective and comprehensive view of employee performance, which is crucial for fair promotions and feedback. 27 Database Types, Functions, and Components Databases serve as critical infrastructure for storing, managing, and accessing data across various applications. They play a pivotal role in modern information systems, enabling efficient data organization and retrieval. 28 Database Types, Functions, and Components Databases serve as critical infrastructure for storing, managing, and accessing data across various applications. They play a pivotal role in modern information systems, enabling efficient data organization and retrieval. 29 Database Types Relational Databases: Relational databases organize data into tables with rows and columns, linked by common attributes. Common Use Cases: Transaction processing, business applications, and data warehousing. Non-Relational Databases: Non-relational databases, also known as NoSQL databases, offer flexible data models beyond the traditional tabular structure. Advantages: Scalability, flexibility, and support for unstructured or semi-structured data. Use Cases: Big data analytics, real-time applications, and content management systems. Distributed Databases: Distributed databases distribute data across multiple nodes in a network, enhancing scalability and fault tolerance. 30 Data Organization and Cleaning Best Practices Introduction Effective data organization and cleaning are critical for ensuring the quality, integrity, and reliability of data used in analysis and decision-making processes. By implementing best practices, organizations can optimize data usability and minimize errors. 31 Data Organization and Cleaning Best Practices Data Structuring: Discuss methods for structuring data to facilitate efficient analysis and retrieval. Examples include organizing data into tables, matrices, or hierarchical structures based on the nature of the data and the analysis requirements. Emphasize the importance of consistent data formats and naming conventions for ease of interpretation and compatibility across systems. Indexing: Explain the concept of indexing and its role in optimizing data retrieval performance. Indexes are data structures that allow for faster data retrieval by providing quick access to specific data points. Discuss techniques such as B-trees and hash indexes and their application in databases to enhance query performance. 32 Data Organization and Cleaning Best Practices Handling Missing Values: Strategies for dealing with missing data to prevent bias and ensure completeness include imputation, where missing values are replaced with estimated values based on statistical techniques or domain knowledge. Emphasize the importance of understanding the reasons for missing data and selecting appropriate imputation methods accordingly. 33 Data Organization and Cleaning Best Practices Outliers: Techniques for identifying and handling outliers in datasets. Outliers can skew statistical analysis and model results, leading to erroneous conclusions. Discuss approaches such as visual inspection, statistical tests, and methods like trimming or winsorizing to mitigate the impact of outliers on data analysis. 34 Data Organization and Cleaning Best Practices Duplicate Data Best practices for detecting and removing duplicate entries to maintain data accuracy. Duplicates can arise from data entry errors, system bugs, or data integration processes. Highlight methods such as deduplication algorithms, fuzzy matching, and record linkage to identify and resolve duplicate records efficiently. 35 Any Questions ? 36 Thank You 37