Data Ethics PDF
Document Details
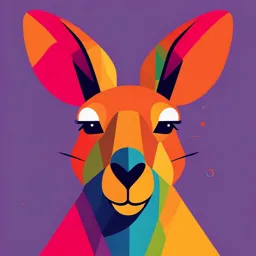
Uploaded by SelfSufficiencyDivisionism4376
MAKAUT
Tags
Summary
This document discusses the principles of data ethics, focusing on data collection, storage, and use practices. It highlights the importance of data ethics in building trust with customers, and considers potential issues such as bias in algorithms and the need for transparency.
Full Transcript
**Data Ethics** **Introduction** Data can be used to drive decisions and make an impact at scale. Yet, this powerful resource comes with challenges. How can organizations ethically collect, store, and use data? What rights must be upheld? The field of data ethics explores these questions and offer...
**Data Ethics** **Introduction** Data can be used to drive decisions and make an impact at scale. Yet, this powerful resource comes with challenges. How can organizations ethically collect, store, and use data? What rights must be upheld? The field of data ethics explores these questions and offers guiding principles for business professionals who handle data. Data ethics encompasses the moral obligations of gathering, protecting, and using personally identifiable information and how it affects individuals. "Data ethics asks, 'Is this the right thing to do?' and 'Can we do better?'" **What is data ethics?** Data ethics is a branch of ethics that evaluates data practices---collecting, generating, analyzing and disseminating data, both structured and unstructured---that have the potential to adversely impact people and society. It includes addressing and recommending concepts of right and wrong conduct, with transparency in and defensibility of actions and decisions driven by automated/artificial intelligence (AI) in relation to data in general and personal data in particular. Data ethics is built upon and extends the limits of computer and information ethics. Instead of being information focused, they are being shifted to data-focused. For instance, data ethics wants to focus on individuals' data based on third party practices rather than media and journalism ethics that information ethics focuses on. Data that companies collect from regular individuals raises quite a few ethical issues. Since this type of collection from people and data ethics is pretty new, there is still a lot to figure out in regard to this new territory. This is becoming especially important as companies begin to use the data, they have collected from people to monetize it for purposes that are different from what the data was originally intended for. **Why is Data Ethics Important?** --------------------------------- Data ethics is important because there must be a universal framework for what companies can and cannot do with the data they collect from people. While there is still a lot of grey area in this territory and there is nothing clearly black and white, there are five things that all experts generally agree should be put into place. Private customer data and the identity of the person associated with that data needs to remain private and confidential. If data has been collected from a person with their knowledge that is ok, but it should never be exposed or given to other businesses that has their personal identity attached to the data in some way. Customers who give out their data should also have a complete, open, and transparent view of how they data is being used, if it is being sold for other purposes they initially intended, and they should be able to have control in the flow of their private information. It is also agreed that data should never interfere with human will. This data should never be used to determine who a person is before another person is able to make up their owns minds in regard to said person. Perhaps one of the biggest, most complex, and controversial issues regarding data ethics is how individual's data may create unfair biased. Sexism and racisms are the biggest concerns. This bias can come from actual humans, but it can also come from machine learning and algorithms. They can learn to make unconscious biases based on certain things. These are just a few of the main focuses and principles that are being focused on most heavily at the moment. Although there is still much more to learn, discuss, and develop when it comes to the basis of and formation of data ethics. ##### **What are the business benefits of data ethics?** ##### With AI algorithms becoming more commonplace, and without regulated codes of ethics, businesses need to create and maintain a structured and transparent data ethics strategy, which can yield three important business benefits: - **Trust.** Businesses that apply the key ethics principles of fairness, privacy, transparency and accountability to their AI models and output can retain trust in how they use their data---and thus build greater goodwill and loyalty, which enhances their reputation and brand value. - **Fair practices. **Unintended bias can creep in from anywhere and negatively impact business decisions. Companies that adhere to data ethics principles and standards can demonstrate their fairness in decision-making. - **Data privacy compliance.** Existing data privacy regulations like the General Data Protection Regulation (GDPR) and the California Consumer Privacy Act (CCPA) do not directly address ethics, but there is a significant overlap between key privacy requirements, such as lawfulness and accountability, and the principles of AI ethics. Thus, ensuring ethical AI helps ensure data privacy compliance. ##### ##### **How can data ethics engender trust in customers?** ##### The biggest threat to companies today comes not from the competition, but rather from their own inability to win and keep consumer trust. The increasing importance of data has changed not only how companies deliver products and services, but also how consumers make decisions. To build trust at every touchpoint throughout the customer journey, senior executives must ensure their companies have the right leadership, culture, organizational design, operating model, skills, technology and processes. ##### Here are a few guidelines to win customers' trust in the new digital world: - **Ensure "gives" and "gets."** Where there are manageable "gives" and positive "gets" for consumers, sharing information is a no-brainer. However, if the "get" factor is wrongly imposed---such as asking consumers to accept terms without explaining the ramifications---trust can be instantly and badly damaged. Therefore, clear and open communication regarding give-to-get trade-offs are the foundation for creating the needed transparency that makes it worthwhile for both the company and its customers. - **Give customers a delete button.** Customers should have a complete 360-degree view of their information and full control over it. A good example is an e-monitor that enables cardiac patients to control how much data goes to whom, using a browser and an app. Patients can set up networks of healthcare providers, family members, friends, fellow users and patients, and share their desired data with each. - **Be quick to respond to failures.** In spite of world-class technology infrastructure, history shows that organizations can't promise customers that nothing bad will happen to their digital information. Winning organizations need to recognize, understand and proactively manage potential issues. For instance, after a recent cyber-attack, a communications provider was quick to notify customers and banks of the incident, which helped maintain consumer trust and confidence. Data ethics are of the utmost concern to analysts, data scientists, and information technology professionals. Anyone who handles data, however, must be well-versed in its applications. For instance, your company may collect and store data about customers' journeys from the first time they submit their email address on your website to the fifth time they purchase your product. If you're a digital marketer, you likely interact with this data daily. While you may not be the person responsible for implementing tracking code, managing a database, or writing and training a machine-learning algorithm, understanding data ethics can allow you to catch any instances of unethical data collection, storage, or use. By doing so, you can protect your customers\' safety and save your organization from legal issues. Here are five principles of data ethics to apply at your organization. **5 PRINCIPLES OF DATA ETHICS FOR BUSINESS PROFESSIONALS** ---------------------------------------------------------- ### **1. Ownership** The first principle of data ethics is that an individual has ownership over their personal information. Just as it's considered stealing to take an item that doesn't belong to you, it's unlawful and unethical to collect someone's personal data without their consent. Some common ways you can obtain consent are through signed written agreements, digital privacy policies that ask users to agree to a company's terms and conditions, and pop-ups with checkboxes that permit websites to track users' online behavior with cookies. Never assume a customer is OK with you collecting their data; always ask for permission to avoid ethical and legal dilemmas. ### **2. Transparency** In addition to owning their personal information, data subjects have a right to know how you plan to collect, store, and use it. When gathering data, exercise transparency. For instance, imagine your company has decided to implement an algorithm to personalize the website experience based on individuals' buying habits and site behavior. You should write a policy explaining that cookies are used to track users' behavior and that the data collected will be stored in a secure database and train an algorithm that provides a personalized website experience. It's a user's right to have access to this information so they can decide to accept your site's cookies or decline them. Withholding or lying about your company's methods or intentions is deception and both unlawful and unfair to your data subjects. ### **3. Privacy** Another ethical responsibility that comes with handling data is ensuring data subjects' privacy. Even if a customer gives your company consent to collect, store, and analyze their personally identifiable information (PII), that doesn't mean they want it publicly available. PII is any information linked to an individual's identity. Some examples of PII include: - - - - - - - - To protect individuals' privacy, ensure you're storing data in a secure database so it doesn't end up in the wrong hands. Data security methods that help protect privacy include dual-authentication password protection and file encryption. For professionals who regularly handle and analyze sensitive data, mistakes can still be made. One way to prevent slip-ups is by de-identifying a dataset. A dataset is de-identified when all pieces of PII are removed, leaving only anonymous data. This enables analysts to find relationships between variables of interest without attaching specific data points to individual identities. ### **4. Intention** When discussing any branch of ethics, intentions matter. Before collecting data, ask yourself why you need it, what you'll gain from it, and what changes you'll be able to make after analysis. If your intention is to hurt others, profit from your subjects' weaknesses, or any other malicious goal, it's not ethical to collect their data. When your intentions are good---for instance, collecting data to gain an understanding of women's healthcare experiences so you can create an app to address a pressing need---you should still assess your intention behind the collection of each piece of data. Are there certain data points that don't apply to the problem at hand? For instance, is it necessary to ask if the participants struggle with their mental health? This data could be sensitive, so collecting it when it's unnecessary isn't ethical. Strive to collect the minimum viable amount of data, so you're taking as little as possible from your subjects while making a difference. ### **5. Outcomes** Even when intentions are good, the outcome of data analysis can cause inadvertent harm to individuals or groups of people. This is called a disparate impact, which is outlined in the Civil Rights Act as unlawful. In Data Science Principles, Harvard Professor Latanya Sweeney provides an example of disparate impact. When Sweeney searched for her name online, an advertisement came up that read, "Latanya Sweeney, Arrested?" She had not been arrested, so this was strange. "What names, if you search them, come up with arrest ads?" Sweeney asks in the course. "What I found was that if your name was given more often to a Black baby than to a white baby, your name was 80 percent more likely get an ad saying you had been arrested." It's not clear from this example whether the disparate impact was intentional or a result of unintentional bias in an algorithm. Either way, it has the potential to do real damage that disproportionately impacts a specific group of people. Unfortunately, you can't know for certain the impact your data analysis will have until it's complete. By considering this question beforehand, you can catch any potential occurrences of disparate impact. **ETHICAL USE OF ALGORITHM** ---------------------------- Algorithm ethics is becoming a critical issue across businesses of every shape, size, and type as artificial intelligence and machine learning systems are being integrated into standard business workflows. These machines, originally thought to perform only menial tasks, have sophisticated rapidly with [technological advances](https://www.brookings.edu/research/protecting-privacy-in-an-ai-driven-world/). Now, AI and ML are being adopted heavily. Though it is exciting that these machines are rapidly being added to many industries, the approach to algorithm ethics and regulations must keep up with that pace. In research and in practice, people have found many ethical problems. Companies should focus on understanding what these problems are, why they exist, and come up with safeguards to prevent any trouble with algorithms. The [algorithm ethics problems](https://www.forbes.com/sites/cognitiveworld/2020/12/29/ethical-concerns-of-ai/?sh=516cb90e23a8) in AI and ML and their impacts can differ based on industry. Since these technologies are so versatile, it makes it hard to pinpoint exactly what a company should expect. However, there are some overarching patterns of which companies should be aware. **Bias and discrimination: **These algorithms are capable of comprehending much more information, at a much faster rate, than humans. However, this does not mean the conclusions are any more [neutral or just](https://clarkstonconsulting.com/insights/mitigating-bias-in-analytics/). For example, AI being used to predict future criminals showed a bias and e[levated the potential for racial discrimination](https://www.brookings.edu/research/understanding-risk-assessment-instruments-in-criminal-justice/). This bias can also be translated into the workplace, especially through new [HR recruiting software](https://clarkstonconsulting.com/insights/dei-in-recruiting-processes/). Though these may help eliminate human bias in the recruiting process, AI can still make unfair judgements. Remember, humans are behind the codes and systems which can create an inherent bias. If we want to make the algorithms fairer, we must consciously check our biases and make sure they do not infiltrate our algorithms. **Privacy concerns: **[Privacy](https://www.brookings.edu/research/how-to-address-ai-ethical-dilemmas/) is a major concern already, as data on you can be collected from seemingly anywhere. The emergence of new algorithms means these existing concerns will just be amplified. AI enables companies to track what people are doing and predict what they'll do next. For example, [social media sites](https://clarkstonconsulting.com/insights/social-media-data-use-cases/) and streaming services are able to push content you are likely to engage with based on previous clicks and plays even you may have forgotten. Having your every action tracked and having profiles built about you can be concerning. The pervasiveness of AI-enabled knowledge will continue to grow. The question is now: How will companies use this data? While some regulators are trying to implement and enforce legislation to protect user privacy, this is a continuously evolving space with implications for most businesses. **Transparency in decision making: **The goal of advanced algorithms is to have them make decisions based on information and calculations that humans cannot compute themselves. However, as these intelligent machines get smarter, humans may no longer understand how they make their final conclusions. Therefore, it will be hard to identify what steps the algorithm need to be fixed or amended to get better results. Ideally, AI and ML progress will be [traceable and explainable](https://www.itproportal.com/features/the-top-three-risks-posed-by-ai-and-how-to-safeguard-against-them/) so human oversight can be implemented. This allows for human judgement to correct for wrong assumptions or faulty conclusions. Different applications have different trade-offs on interpretability vs. accuracy. some complex models may give very accurate results in testing but are considered 'black box' models that can't be explained at the most detailed level. While in some applications that's acceptable for others the algorithm inputs need to be explainable, particularly for the safety of children and teens as in the case of [Facebook](https://www.technologyreview.com/2021/10/05/1036519/facebook-whistleblower-frances-haugen-algorithms/). **Setting up Safeguards to Prevent Potential Problems** ------------------------------------------------------- ** **The use of AI and ML are still relatively new in business, so it may be hard to predict what the best practices are in using these technologies. But we can set up safeguards that can track how we use the algorithms and set up a process for dealing with potential problems. The main goal for safeguarding against advanced algorithms is to maintain human oversight at all stages of implementation. Unless we are confident that machines can work with human sensitivity and empathy, it is important that companies are allowing people to make the final call. **AI Review Boards: **Businesses who are looking to implement AI in their workflow should set up AI review boards. These board members will be responsible for making sure integration of AI and ML is seamless in the company's decision-making processes. They will also be responsible for the procedures used within the company when developing, adopting, or deploying and AI-related products. An important aspect of these review boards is diversity. The board members should not only be diverse in background, but also in terms of their stakeholder position within the company. Companies can set up these review boards in a process similar to how DevOps reviews code. Just as software engineering best practice is to have code reviews and thorough testing before code makes it to production, the same is true of AI and ML models. the work should be verified and thoroughly tested.  ML Ops actually requires further validation and ongoing model maintenance. DevOps you build a solution then it works. Model Ops you would build a solution and then have to monitor it over time because the data is always new and changing so the model may not work as expected a year from now **Code of Ethics: **As with any ethical decision-making strategy, companies should construct a formal code of ethics with which to abide. This code should be thorough in laying out core principles and values of the company, any processes, and [how to address algorithm ethics issues](https://www.coe.int/en/web/commissioner/-/safeguarding-human-rights-in-the-era-of-artificial-intelligence) in AI development. Once developed this code should be made accessible to all employees and other company stakeholders. External parties should be able to access the code as well so they can see how the company is being proactive in their adoption of AI. **AI Audit Trails**: Finally, to deal with future problems, companies should have AI audit trails. These [audit trails](https://www.infoworld.com/article/3054055/3-safeguards-for-intelligent-machines.html) are necessary in boosting explainability and transparency in how the algorithms are being developed.  Not only will this help outside parties understand how AI is being used within the firm, but also internal employees to track progress and potential missteps within the process. These audit trails may be especially relevant if there are any consumer harm or product liability cases that arise because of AI use.