CS-Sem-5-Artificial-Intelligence.pdf
Document Details
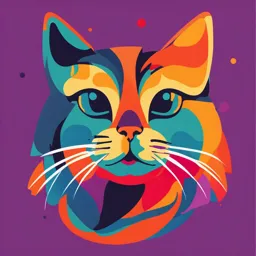
Uploaded by IngenuousIntegral
University of Mumbai
2023
University of Mumbai
Tags
Related
- Artificial Intelligence with Machine Learning (Unit 1) PDF
- Artificial Intelligence PDF
- Artificial Intelligence (AI) PDF
- COMCTN4 Second Grading Exam - Artificial Intelligence and Machine Learning (PDF)
- DST301 Artificial Intelligence Applications Lecture 02 - Machine Learning PDF
- CPCS-335 Introduction to Artificial Intelligence Lecture 8 PDF
Full Transcript
T.Y.B.Sc. (C. S.) SEMESTER - V (CBCS) ARTIFICIAL INTELLIGENCE SUBJECT CODE: USCS501 © UNIVERSITY OF MUMBAI Prof. (Dr.) D. T. Shirke Offg. Vice Chancellor...
T.Y.B.Sc. (C. S.) SEMESTER - V (CBCS) ARTIFICIAL INTELLIGENCE SUBJECT CODE: USCS501 © UNIVERSITY OF MUMBAI Prof. (Dr.) D. T. Shirke Offg. Vice Chancellor University of Mumbai, Mumbai Prin. Dr. Ajay Bhamare Prof. Prakash Mahanwar Offg. Pro Vice-Chancellor, Director, University of Mumbai IDOL, University of Mumbai Programme Co-ordinator : Shri Mandar Bhanushe Head, Faculty of Science and Technology IDOL, Univeristy of Mumbai - 400098 Course Co-ordinator : Ms. Mitali Vijay Shewale Doctoral Research, Veermata Jijabai Technological Institute Matunga, Mumbai. Editor : Mr. Anish Raut Assistant Manager, Dahua Technology India Pvt.Ltd., Mumbai. Course Writers : Dr. Rajeshri Pravin Shinkar Assistant Professor, SIES (Nerul) College of Arts, Science & Commerce.Navi Mumbai. : Dr. Rajendra Patil Principal, Bunts Sangha Anna Leela College of Commerce and Economics, Mumbai. : Ms. Mitali Vijay Shewale Doctoral Research, Veermata Jijabai Technological Institute Matunga, Mumbai. August 2023, Print - 1 Published by : Director, Institute of Distance and Open Learning, University of Mumbai, Vidyanagari,Mumbai - 400 098. DTP composed and Printed by: Mumbai University Press CONTENTS Unit No. Title Page No. 1 Artificial Intelligence...............................................................................................1 2. Intelligent Agent.....................................................................................................19 3. Problem Solving By Searching..............................................................................30 4. Learning From Examples.......................................................................................79 5. Learning Probabilistic Models.............................................................................106 6. Reinforcement Learning.....................................................................................130 T.Y.B.Sc. (C. S.) SEMESTER - V (CBCS) ARTIFICIAL INTELLIGENCE SEMESTER V SYLLABUS THEORY Course: TOPICS (Credits : 03 Lectures/Week:03) USCS501 Artificial Intelligence Objectives: Artificial Intelligence (AI) and accompanying tools and techniques bring transformational changes in the world. Machines capability to match, and sometimes even surpass human capability, make AI a hot topic in Computer Science. This course aims to introduce the learner to this interesting area. Expected Learning Outcomes: After completion of this course, learner should get a clear understanding of AI and different search algorithms used for solving problems. The learner should also get acquainted with different learning algorithms and models used in machine learning. What Is AI: Foundations, History and State of the Art of AI. Intelligent Agents: Agents and Environments, Nature of Environments, Structure of Agents. Unit I 15L Problem Solving by searching: Problem-Solving Agents, Example Problems, Searching for Solutions, Uninformed Search Strategies, Informed (Heuristic) Search Strategies, Heuristic Functions. Learning from Examples: Forms of Learning, Supervised Learning, Learning Decision Trees, Evaluating and Choosing the Best Hypothesis, Theory of Unit II Learning, Regression and Classification with Linear Models, Artificial Neural 15L Networks, Nonparametric Models, Support Vector Machines, Ensemble Learning, Practical Machine Learning Learning probabilistic models: Statistical Learning, Learning with Complete Data, Learning with Hidden Variables: The EM Algorithm. Reinforcement Unit III learning: Passive Reinforcement Learning, Active Reinforcement Learning, 15L Generalization in Reinforcement Learning, Policy Search, Applications of Reinforcement Learning. Textbook(s): 1) Artificial Intelligence: A Modern Approach, Stuart Russell and Peter Norvig,3rd Edition, Pearson, 2010. Additional Reference(s): 1) Artificial Intelligence: Foundations of Computational Agents, David L Poole,Alan K. Mackworth, 2nd Edition, Cambridge University Press ,2017. 2) Artificial Intelligence, Kevin Knight and Elaine Rich, 3rd Edition, 2017 3) The Elements of Statistical Learning, Trevor Hastie, Robert Tibshirani and Jerome Friedman, Springer, 2013 Course: TOPICS (Credits : 03 Lectures/Week:03) USCS502 Linux Server Administration Objectives: Demonstrate proficiency with the Linux command line interface, directory & file management techniques, file system organization, and tools commonly found on most Linux distributions. Effectively operate a Linux system inside of a network environment to integrate with existing service solutions. Demonstrate the ability to troubleshoot challenging technical problems typically encountered when operating and administering Linux systems. Expected Learning Outcomes: Learner will be able to develop Linux based systems and maintain. Learner will be able to install appropriate service on Linux server as per requirement. Learner will have proficiency in Linux server administration. 1 ARTIFICIAL INTELLIGENCE Unit Structure : 1.0 Objectives 1.1 What is AI? 1.2 Foundations of AI 1.2.1 Acting Humanlay: The Turing Test Arrpoach 1.2.2 Thinking Rationally: The “Laws of Thought” Approach 1.2.3 Thinking Rationally: The “Laws of Thought” Approach 1.2.4 Acting Rationally: The Rational Agent Approach 1.2.5 Categorization of Intelligent Systems 1.2.6 Components of AI 1.2.7 Computational Intelligence (CI) Vs Artificial Intelligence (AI) 1.3 History of Artificial Intelligene 1.3.1 Applications of AI 1.3.2 Domains or sub areas of AI 1.4 State of Art of AI 1.5 Problem Solving with Artificial Intelligence 1.5.1 Problems Summary Questions 1.0 OBJECTIVES After completing this chapter learner will able to: Understand What is Artificial Intelligence? Foundations of Artificial Intelligence Categories of Intelligent System Components of Artificial Intelligence. History of Artificial Intelligence. Applications of AI Problems of AI 1 Artificial Intelligence 1.1 WHAT IS AI? It is a branch of Computer Science that pursues creating the computers or machines as intelligent as human beings. It is the science and engineering of making intelligent machines, especially intelligent computer programs. It is related to the similar task of using computers to understand human intelligence, but AI does not have to confine itself to methods that are biological observable. 1.1.1 Introduction Artificial intelligence (AI) is a relatively recent branch of science and engineering. Soon after World War II, work began in earnest, and the term was coined in 1956. In addition to molecular biology, AI is frequently mentioned by scientists from other fields as the "field I'd most like to be in." A physics student may fairly believe that all of the good ideas have already been taken. Galileo, Newton, and Einstein are three of the most famous scientists of all time. Definition: Artificial Intelligence is the study of how to make computers do things, which, at the moment, people do better. According to the father of Artificial Intelligence, John McCarthy, it is “The science and engineering of making intelligent machines, especially intelligent computer programs”. Artificial Intelligence is a way of making a computer, a computer-controlled robot, or a software thinks intelligently, in the similar manner the intelligent humans think. AI is accomplished by studying how human brain thinks and how humans learn, decide, and work while trying to solve a problem, and then using the outcomes of this study as a basis of developing intelligent software and systems. It has gained prominence recently due, in part, to big data, or the increase in speed, size and variety of data businesses are now collecting. AI can perform tasks such as identifying patterns in the data more efficiently than humans, enabling businesses to gain more insight out of their data. From a business perspective AI is a set of very powerful tools, and methodologies for using those tools to solve business problems. Intelligence Because our intelligence is so vital to us, we call ourselves Homosapiens- 2 man the wise. For thousands of years, scientists have attempted to comprehend how we think: how a small amount of matter can see, Artificial Intelligence comprehend, predict, and manage a world considerably larger and more sophisticated than itself. Artificial intelligence, or Al, is a field that goes even further. 1.2 FOUNDATIONS OF AI Now we discuss the various disciplines that contribute ideas, viewpoints and techniques to AI. Philosophy provide base to AI by providing theories of relationship between physical brain and mental mind, rules for drawing valid conclusions. It also provides information about knowledge origins and the knowledge needs to actions. Mathematics gives strong base to AI to develop concrete and formal rules for drawing valid conclusions, various methods for date computation and techniques to deal with uncertain information. Economics support AI to make decisions so as to maximum payoff and make decisions under certain circumstances. Neuroscience gives information which is related to brain processing which helps AI to developed date processing theories. Psychology provides strong concepts of how humans and animals act which helps AI for developing process of thinking and actions. Historically there are four approaches to AI have been followed, each by different people with different methods. A rationalist approach involves a combination of mathematics and engineering. The various group have both disparaged and helped each other. Intelligent Systems In order to design intelligent systems, it is important to categorize them into four categories (Luger and Stubberfield 1993), (Russell and Norvig, 2003) 1. Systems that think like humans 2. Systems that think rationally 3. Systems that behave like humans 4. Systems that behave rationally Human-Like Rationally Cognitive Science Approach Laws of thought Approach Think : “Machines that think like “Machines that think humans” Rationally” Turing Test Approach Rational Agent Approach Act : “Machines that behave like “Machines that behave humans” Rationally” 3 Artificial Intelligence 1.2.1 Acting Humanlay: The Turing Test Arrpoach Turing test: a method of determining intellect. Turing Test was conceived by Alan Turing in 1950. He proposed a test based on common characteristics that can be matched to the most intelligent entity on the planet – humans. Computer would need to process the following capabilities: I) Natural language processing - In order for it to be able to communicate effectively in English. II) Knowledge representation to store what it knows, what it hears. III) Automated reasoning to make use of stored information to answer questions being asked and to draw conclusions. IV) Machine learning to adapt to new circumstances and to detect and make new predictions by finding patterns. V) Turing also proposed that the interrogator and the computers engage physically. The Turing test avoids this, but the Total Turing Assess includes a video signal to allow the interrogator to test the subject's perceptual abilities, as well as the ability to pass physical things "through the hatch." VI) To pass total Turing test in addition, computer will need following capabilities. VII) Computer vision to perceive objects. VIII) Robotics to manipulate objects. 1.2.2 Thinking Rationally: The “Laws of Thought” Approach Because we're claiming that the given software thinks like a human, we need to understand how humans think. The theory of human minds must be investigated in order to achieve this. There are two methods for doing so: introspection (trying to catch our own thoughts as they pass us by) and psychological experiments. We can argue that some of the program's mechanisms are also operating in human mode if computer programmers’, input, output, and timing behaviors’ mirror similar human behaviors. Cognitive science is an interdisciplinary study that draws together computer models from AI and experimental approaches from psychology to try to build accurate and testable explanations of how the human mind works. 1.2.3 Thinking Rationally: The “Laws of Thought” Approach “Right thinking” concept was introduced by Aristotle. Patterns for argument structures that always gives correct decisions when the premises are correct. It is known as the laws of thought approach. 4 "The study on mental faculties through the use of computational models. Artificial Intelligence (Charmiakand McDemott, 1985) "The study of the computations that make it possible to perceive, reason, and act." (Winston, 1992) Law of thought were supposed to govern the operation in the mind; their study initiated the field called Logic which can be implemented to create the system which is known as intelligent system. 1.2.4 Acting Rationally: The Rational Agent Approach Something that acts is called an agent (Latin agre-to-do). Computer agents, on the other hand, are intended to have additional characteristics that separate them from "programmes," such as independent control, time perception, adaptability to change, and the ability to take on new goals. When there is uncertainty, a rational agent is required to act in such a way that the best possible outcome is achieved. The laws of thought emphasis on correct inference which should be incorporated in rational agent. “Computational Intelligence is the study of the design of intelligent agents.” By Poole et at, 1998 1.2.5 Categorization of Intelligent Systems There are various types and forms of AI. The various categories of AI can be based on the capacity of intelligent program or what the program is able to do. Consideration of the above factors there are three main categories: 1) Weak AI (Artificial Narrow Intelligence) 2) Strong AI (Artificial General Intelligence) 3) Artificial Super Intelligence 1) Weak AI : Weak AI is AI that focuses on a single task. It isn't an intellect that can be used in a variety of situations. Narrow intelligence or weak AI refers to an intelligent agent that is designed to solve a specific problem or perform a certain task. For example, it took years of AI research to beat the chess grandmaster, and humans still haven't beaten the machines at chess since then. But that's all it can do, and it does it exceptionally well. 2) Strong AI : Strong AI, often known as general AI, refers to machine intelligence proven in the performance of any cognitive task that a person can execute. It is far more difficult to construct powerful AI than it is to develop weak AI. Artificial general intelligence machines can display human qualities such as reasoning, planning, problem solving, grasping complicated ideas, learning from personal experiences, and so on by using artificial general intelligence. Many corporations and companies are working on developing general intelligence, but they have yet to finish it. 5 Artificial Intelligence 3) Artificial Super-Intelligence : AI thinker Nick Bostrom defined “Super intelligence is an intellect that is much smarter than the best human brains in practically every field, including scientific creativity, general wisdom and social skills.” Super intelligence ranges from a machine which is just a little smarter than a human to a machine that is trillion times smarter. Artificial super intelligence is the ultimate power of AI. Weak AI Strong AI It is a narrow application with a It is a wider application with a more limited scope. vast scope. This application is good at specific This application has an incredible tasks. human-level intelligence. It uses supervised and It uses clustering and association to unsupervised learning to process process data. data. Example Example Siri, Alexa Advanced Robotics 1.2.6 Components of AI The intelligence is intangible. It is composed of − Reasoning Learning Problem Solving Perception Linguistic Intelligence Let us go through all the components briefly − Reasoning − It is the set of processes that enables us to provide basis for judgement, making decisions, and prediction. There are broadly two types − 6 Artificial Intelligence Inductive Reasoning Deductive Reasoning It conducts specific observations to It starts with a general statement makes broad general statements. and examines the possibilities to reach a specific, logical conclusion. Even if all of the premises are true If something is true of a class of in a statement, inductive reasoning things in general, it is also true for allows for the conclusion to be all members of that class. false. Example − "Nita is a teacher. Nita Example − "All women of age is studious. Therefore, All teachers above 60 years are grandmothers. are studious." Shalini is 65 years. Therefore, Shalini is a grandmother." Learning − It is the activity of gaining knowledge or skill by studying, practising, being taught, or experiencing something. Learning enhances the awareness of the subjects of the study. The ability of learning is possessed by humans, some animals, and AI-enabled systems. Learning is categorized as − o Auditory Learning − It is learning by listening and hearing. For example, students listening to recorded audio lectures. o Episodic Learning − To learn by remembering sequences of events that one has witnessed or experienced. This is linear and orderly. o Motor Learning − It is learning by precise movement of muscles. For example, picking objects, Writing, etc. o Observational Learning − To learn by watching and imitating others. For example, child tries to learn by mimicking her parent. o Perceptual Learning − It is learning to recognize stimuli that one has seen before. For example, identifying and classifying objects and situations. o Relational Learning − It involves learning to differentiate among various stimuli on the basis of relational properties, rather than absolute properties. For Example, Adding ‘little less’ salt at the time of cooking potatoes that came up salty last time, when cooked with adding say a tablespoon of salt. o Spatial Learning − It is learning through visual stimuli such as images, colors, maps, etc. For Example, A person can create roadmap in mind before actually following the road. 7 Artificial Intelligence o Stimulus-Response Learning − It is learning to perform a particular behaviour when a certain stimulus is present. For example, a dog raises its ear on hearing doorbell. Problem Solving − It is the process in which one perceives and tries to arrive at a desired solution from a present situation by taking some path, which is blocked by known or unknown hurdles. Problem solving also includes decision making, which is the process of selecting the best suitable alternative out of multiple alternatives to reach the desired goal are available. Perception − It is the process of acquiring, interpreting, selecting, and organizing sensory information. Perception presumes sensing. In humans, perception is aided by sensory organs. In the domain of AI, perception mechanism puts the data acquired by the sensors together in a meaningful manner. Linguistic Intelligence − It is one’s ability to use, comprehend, speak, and write the verbal and written language. It is important in interpersonal communication. 1.2.7 Computational Intelligence (CI) Vs Artificial Intelligence (AI) Computational Intelligence (CI) Artificial Intelligence (AI) Computational Intelligence is the Artificial Intelligence is the study study of the design of intelligent of making machines which can do agents. things which at presents human do better. Involvement of numbers and Involvement of designs and computations. symbolic knowledge representations. CI constructs the system starting AI analyses the overall structure of from the bottom level an intelligent system by following computations, hence follows top down approach. bottom-up approach. CI concentrates on low level AI concentrates on high level cognitive function implementation. cognitive structure design. 1.3 HISTORY OF ARTIFICIAL INTELLIGENE John McCarthy in 1955 introduced the term Artificial Intelligence. The early work of Artificial Intelligence was done in the period 1943 to 1955. The first AI thoughts were formally put by McCulloch & Walter Pitts in the year 1943. They introduced with the concept of AI was based on different three theories. First theory is based on phycology i.e. Neuron 8 functions in the brain. Second theory is based on formal analysis of propositional logic and third theory is based on Turing’s theory of Artificial Intelligence computations. 1956-61 The first year of this period gave rise to the terminology ‘Artificial Intelligence’ proposed by McCarthy & supposed by the participants in the conference. In the same year Samuel developed a program for chess playing which performed better than its creator. Around 1956-57, Chomsky’s grammar in NLP i.e. linguistic model processing was a remarkable event. In 1958, McCarthy made a very significant contribution, development of LISP, an AI programming language and advice taker which combined the method of knowledge representation and resoning. Herbert Gelerriter at IBM in 1959 designed the first written AI program for geometry theorem proving in quick succession of time. In 1960, Window alone & then with Hoff developed networks called ‘Adaline’, based on the concepts of Hebbian learning. In 1956-57, logic theorist (LT), a program for automatic theorem proving was developed. 1962-67 At the beginning of this period Frank Rosen blatt proposed the concept of ‘perception’ in the line of Window’s concept for artificial neural networks (ANN), a biological model to incorporate computational rationality. In 1963. McCarthy developed a general purpose logical reasoning method and it was enhanced by the Robinson’s ‘Resolution principle’ (Robinson, 1965). The logical neural model of McCulloch and Pitts was enhanced by Winograd & Cowan in 1963. James Slage’s program was developed for the interpretation of calculus in 1963. In 1965, Hearsay was developed at CMU for natural language interpretation of subset language. 1968-73 In this period, some AI program for practical use were developed. In 1967, David Bobrow developed ‘STUDENT’ to solve algebra story problems. The first knowledge-based expert system DENDRAL was developed by J. Lederber, Edward Feigenbaum and Carl Djerassi in 1968, although the work had started in 1965. The program discovered the molecular structure of an organic compound based on the mass spectral data. Simon stated that within 10 years a computer would be chess champion, & a significant mathematical theorem would be proved by machine. These predictions came true or approximately true within 40 years rather than 10. The new back-propagation learning algorithms for multilayer networks that were to cause an enormous resurgence in neural-net research in the late 1980’s were actually discovered first in 1969. 1974- 1980 In 1969 Minsky and Papert’s book Perceptron’s proved that perceptrons could represent vary little. Although their result did not apply to more 9 Artificial Intelligence complex, multilayer networks, research funding for neural-net research soon dwindled to almost nothing. In 1973 Professor S ir James Lightill mentioned the problem of combinatorial explosion or intractability which implied that many of AI’s most successful algorithms would grind to a halt on real world problems and were only suitable for solving “toy” versions. 1981-1985 In this period many expert system shells, expert system tools and expert system programs were developed. During 1984-85 the expert system shells came into picture was EMYCIN by Buchanan, a rule-based diagnostic consultant based on LISP, EXPERT by Weiss and KAS by DUDE are the rule-based model for classification using FORTRAN; a semantic network- based system using LISP, others are knowledge crafts by GILMORE using object-oriented programming (OGP), KL-ONE by Brakeman using LISP for automatic inheritance. The most important development was PROLOG as AI programming language by Clockcin 1984. 1986-91 In this period significant developments occurred in ANN model in particular, the appearance of error back propagation algorithm formulated by Rumelhart and Hinton in parallel distributed processing. The probabilistic reasoning method in intelligent system appeared in 1988 by the work of Pearl. The distributed artificial intelligence concepts were formally incorporated in the multi-agent systems. The complete agent- based architecture was first implemented in a model SOAR, designed by Newel, Laired and Rosenbloom. Hidden Markov Model (HMM) was also conceptualized for speech processing and natural language processing during this period. 1992-97 In this period full swing of rise to the agent-based technology and multi- agent system (MAS). In 1992, Nawana brought the concept of autonomous agent, capable of acting independently with rationality. Different kinds of agent were defined. In 1994, Jennings Yoam introduced social and responsible agents, Yoam Shoham in 1993, described the concept of agent- oriented programming with different components and modalities. Belief, desire and intention (BDI) theory was introduced by Cohen (1995) during this period. The concept of cooperation, coordination and conflict resolution in MAS was introduced in this period. In the NLP, a mean X-project was developed at Zurich by Nobel laureate Gerd Binning, who emphasized the use of word ‘knowledge’ to achieve comprehension. Gordon made a model language for representing strategies on standard AI planning techniques. The ABLE (Agent Building & Learning Environment) was developed by Joe Bigns, which focused on building hybrid intelligent agents for both reasoning and learning. 10 1998-2003 Artificial Intelligence In introduction, incorporation and integration of AI concepts, theories and algorithms in and with web technology for information retrieval, extraction and categorization, document summarization, machine translation (single or multilingual) discourse analysis were performed in this period. Courteous logic program in which users specify the scope of potential conflict by pairwise mutual exclusion is implemented in common rules, a Java library used for e-commerce, business and web intelligence emerged as the front of AI. Formula Augmented Network (FAN) was developed by Morgenstern and Singh, is a knowledge structure, which enabled efficient reasoning and about potentially conflicting business rules. Heuristic search methods were devised for game playing such as chess, checkers, Rubik cube with the concept of MPC. Blue Deep was a chess- playing computer developed by IBM on May 11, 1997. Robotics in game playing and surgery marks the splendid achievements in AI based robotics. The Seoul Robotic Football Game from 2001 onwards regularly updated is a good example of the development and incorporation of AI search methodology in game playing. 2004- Future directions and dimensions Communications of the ACM 2003, a certain direction and dimension of AI have been envisaged for the future. Shannon has given the frame qualification and ramification and learning from books, i.e. reading the text and extracting relevant information. Three-dimensional robot surrounding in distilling from the www, a huge knowledge base, the developed of semantic scrapes and computational engines such as human mind and brain. Knowledge discovery and vision system for biometric and automated object regulated in supermarkets are imported milestones. Intelligent interface design should be intuitive for the novice, efficient perception for expert and robust undermines which would facilitate recovery from cognitive and manipulative mistakes. Programs that are helpful for diagnosis of errors and suggestions for corrective actions are to be developed. 1.3.1 Applications of AI Artificial Intelligent Systems 1. Medical : AI has applications in cardiology (CRG), neurology (MRI), Embryology (Sonography), and difficult internal organ procedures, among other fields. 2. Education : Training simulators can be built using artificial intelligence techniques. Software for pre-school children are developed to enable learning with fun games. Automated grading, Interactive tutoring, instructional theory are the current areas of application. 11 Artificial Intelligence 3. Military : When decisions have to be made quickly taking into account an enormous amount of information, and when lives are at stake, artificial intelligence can provide crucial assistance. Training simulators can be used in military applications for the purpose of difficult task which human can not do easily, Robots are also used in many situations. AI plays important role in modern military. 4. Entertainment : Playing different AI based games, where one side human and other side the player (machine) which works on AI technology. Many film industries use Robots to play a role for critical situations like fire, jump etc. 5. Business and Manufacturing: Robots are well equipped with the various task in business and manufacturing. Vehicle workshops Robots are useful for jack purpose, car painting etc. 1.3.2 Domains or sub areas of AI AI applications can be roughly classified based on the type of tools used for inoculating intelligence in the system. Various sub domains and areas in intelligent systems can be given as follows: Expert Systems Natural Language Processing Neural Networks Robotics Fuzzy Logic Sr.No. Research Areas Example 1 Expert Systems Examples − Flight-tracking systems, Clinical systems. 2 Natural Language Processing Examples: Google Now feature, speech recognition, Automatic voice output. 12 Artificial Intelligence Sr.No. Research Areas Example 3 Neural Networks Examples − Pattern recognition systems such as face recognition, character recognition, handwriting recognition. 4 Robotics Examples − Industrial robots for moving, spraying, painting, precision checking, drilling, cleaning, coating, carving, etc. 5 Fuzzy Logic Systems Examples − Consumer electronics, automobiles, etc. 1.4 STATE OF ART OF AI Artificial Intelligence has infiltrated every part of our daily lives. Everywhere, from washing machines to air conditioners to smart phones. AI is assisting us in making our lives easier. AI is also doing fantastic things in industries. In factories, sound work is done by robots. Self-driving cars are now a reality. Barbie, who is WiFi-enabled, uses speech recognition to converse with and listen to children. AI is being used by businesses to improve their products and increase sales. Machine learning has made considerable progress in AI. Areas in which AI is showing significant advancements as follows: 1. Deep Learning 2. Machine Learning 3. AI replacing Workers 4. Internet of Things (IoT) 5. Emotional AI 6. AI in shopping and customer service 7. Ethical AI 13 Artificial Intelligence 1. Deep Learning : Deep learning has been successfully used to a variety of text analysis and understanding challenges in recent years. Document categorization, sentiment analysis, machine translation, and other similar techniques are used, and the results are frequently dramatic. Top Applications of Deep Learning Across Industries Self Driving Cars. News Aggregation and Fraud News Detection. Natural Language Processing. Virtual Assistants. Entertainment. Visual Recognition. Fraud Detection. Healthcare. 2. Machine Learning : Machine Learning is an artificial intelligence application in which a computer/machine learns from past experiences (input data) and predicts the future. The system's performance should be at least human-level. The system learns from the data set provided in order to complete task T. Top 10 real-life examples of Machine Learning Image Recognition. Image recognition is one of the most common uses of machine learning. Speech Recognition. Speech recognition is the translation of spoken words into the text. Medical diagnosis. Statistical Arbitrage. Learning associations. Classification. Prediction. Extraction. 3. AI Replacing Workers : Machines are already better than humans in physical jobs; they can move quicker, more precisely, and lift heavier loads. There will be almost nothing these machines can't accomplished or learn to do quickly. Once they are as sophisticated as we are. 4. Internet of Things (IoT) : AI-assisted The Internet of Things (IoT) develops intelligent machines that mimic smart behaviour and assist in decision-making with little or no human intervention. While IoT is concerned with devices connecting with each other over the internet, AI is concerned with devices learning from their data and experience. 14 5. Emotional AI : Emotion AI, often known as affective computing, is Artificial Intelligence all about utilising artificial intelligence to identify emotions. Machines with this level of emotional intelligence can comprehend both cognitive and emotional channels of human communication. 6. AI in shopping and customer service : Voice detection technology powered by AI may enable customers to converse with digital assistants in order to get the most out of the products they purchase. Most consumers will benefit greatly from this virtual link. Artificial intelligence can help retailers decrease operational costs by automating in-store processes. It can assist customers in the store without the use of salespeople, reduce lines with cashier-less payments, refill stock with real-time stock monitoring, and digitise store displays and trial rooms. 7. Ethical AI : The notion of building artificially intelligent systems utilising norms of behaviour that ensure an automated system can respond to situations in an ethical manner is known as Roboethics, or robot ethics. To do so, we turn to machine ethics, which is concerned with the process of imbuing AI robots with moral characteristics. 1.5 PROBLEM SOLVING WITH ARTIFICIAL INTELLIGENCE 1.5.1 Problems To identify desirable answers, problem-solving relates to artificial intelligence techniques such as building efficient algorithms, heuristics, and doing root cause analysis. The problem-solving agent can decide what to do by reviewing various possible sequences of actions that lead to states of known value and then selecting the best sequence. Search is the term for the process of looking for such a sequence. 1.5.1.1 Classic examples of Artificial Intelligence Search Problems 1. 3*3*3 Rubik’s cube problem 2. 8/15/24 -puzzle problem 3. N-queen problem 4. Water Jug problem 1. 3*3*3 Rubik’s cube problem : A Rubik's cube is a three- dimensional puzzle with six faces, each of which has nine stickers in a three-dimensional (3x3) pattern. The goal of the puzzle is to solve it such that each face has just one colour. 15 Artificial Intelligence Rubic’s cube Problem 2. 8/15/24 -Puzzle Problem : The 8 puzzle problem by the name of N puzzle problem or sliding puzzle problem. N-puzzle that consists of N tiles (N+1 titles with an empty tile) where N can be 8, 15, 24 and so on. In our example N = 8. (that is square root of (8+1) = 3 rows and 3 columns). The 8-puzzle is a sliding puzzle that is played on a 3-by-3 grid with 8 square tiles labelled from 1 through 8, plus a blank square. The goal is to rearrange the tiles so that they are in row-major order, using as few moves as possible. You are permitted to slide tiles either horizontally or vertically into the blank square. 8 – Puzzle Problem 16 3. N – Queen Problems : In N-Queen, the queens need to be placed on Artificial Intelligence the n*n board, in such a way that no queen can dash the other queen, in any direction i.e. horizontally, vertically as well as diagonally. N – Queen Problem N=8 4. Water Jug Problem : In the Artificial Intelligence water jug problem, we are given two jugs, one of which can contain 3 gallons of water and the other of which can store 4 gallons of water. There is no additional measuring equipment accessible, and the jugs themselves are not marked in any way. The agent's job is to fill the 4- gallon jug with 2 gallons of water using only these two jugs and no additional materials. Both of our jugs are initially empty. SUMMARY This chapter gives the details about Artificial Intelligence, History of Artificial Intelligence with its applications, state of art of AI, Various problem related to Artificial Intelligence, Applications, Domains or sub Areas in which AI is showing significant advancements. QUESTIONS Q.1) What is Artificial Intelligence? Q.2) What are the components of Artificial Intelligence? Q.3) Explain various applications of Artificial Intelligence. Q.4) Define Artificial Intelligence Q.5) Discuss examples of problem solving with AI. Q.6) Give the difference between Computational Intelligence (CI) and Artificial Intelligence (AI). 17 Artificial Intelligence TEXT BOOK 1. Artificial Intelligence A Modern Approach, Third Edition, Stuart Russell and Peter Norvig, Pearson Education 2. Elaine Rich, Kevin Knight, & Shivashankar B Nair, Artificial Intelligence, McGraw Hill, 3rd ed.,2009 REFERENCES 1) Introduction to Artificial Intelligence & Expert Systems, Dan W Patterson, PHI.,2010 2) S Kaushik, Artificial Intelligence, Cengage Learning, 1st ed.2011 3) Artificial Intelligence, 3rd Edn., E. Rich and K. Knight (TMH) 4) Artificial Intelligence, 3rd Edn., Patrick Henny Winston, Pearson Education. 18 2 INTELLIGENT AGENT Unit Structure : 2.0 Objectives : 2.1 Introduction 2.1.1 What Is Agent? 2.1.2 Actuators 2.2 Agent Function 2.3 Rationality 2.3.1 Rational Agent 2.4 Intelligent Agent 2.4.1 Structure of Intelligent Agents 2.5 Types of Agents 2.5.1 Simple Reflex Agents 2.5.2 Model Based Reflex Agents 2.5.3 Goal Based Agents 2.5.4 Utility Based Agents 2.5.5 Learning Agents 2.6 Nature of Environments 2.6.1 Natures of Environment 2.0 OBJECTIVES In this chapter we are going to learn agent, intelligent agent, actuators, agent function, what is rationality, rational agent, structure of intelligent agent, various natures of environments, PEAS properties of agent as well as different types of agents. 2.1 INTRODUCTION Agent is something that perceives its environment or surroundings with the help of sensors and act upon that environment with the help of actuators. 2.1.1 What Is Agent? An agent is anything that can be thought of as sensing its surroundings through sensors and acting on them through actuators. 2.1.2 Actuators A human agent has eyes, ears, and other organs for sensors and hands, legs, vocal tract, and so on for actuators. A robotic agent might have cameras and 19 Artificial Intelligence infrared range finders for sensors and various motors for actuators. A software agent receives keystrokes, file contents, and network packets as sensory inputs and acts on the environment by displaying on the screen, writing files, and sending network packets. We use the term percept to refer to the agent's perceptual inputs at any given instant. An agent's percept sequence is the complete history of everything the agent has ever perceived. In general, an agent's choice of action at any given instant can depend on the entire percept sequence observed to date, but not on anything it hasn't perceived. By specifying the agent's choice of action for every possible percept sequence, Fig: Environment and Agent Fig: Generic Robotic Agent Architecture As sensors, the robotic agent uses cameras, infrared range finders, scanners, and other devices, while actuators include various types of motors, screens, printing devices, and other devices. Agent Human Robotic Human Actions Cameras and Human sens organs like Infrared range Various motors organs like eyes, hands, legs, finders for for actuators ears ang others mouth and others sensors Fig : Sensors and Actuators in Human & Robotic Agent 20 2.2 AGENT FUNCTION Intelligent Agent The agent function is the description of what all functionalities the agent is supposed to do. The agent function provides mapping between percept sequences to the desired actions. Figure : Agent Function An agent is anything that can perceive its environment through sensors and acts upon that environment through effectors. A human agent has sensory organs such as eyes, ears, nose, tongue and skin parallel to the sensors, and other organs such as hands, legs, mouth, for effectors. A robotic agent replaces cameras and infrared range finders for the sensors, and various motors and actuators for effectors. Agent Terminology Performance Measure of Agent − It is the criteria, which determines how successful an agent is. Behavior of Agent − It is the action that agent performs after any given sequence of percepts. Percept − It is agent’s perceptual inputs at a given instance. Percept Sequence − It is the history of all that an agent has perceived till date. Agent Function − It is a map from the precept sequence to an action. 21 Artificial Intelligence 2.3 RATIONALITY Rationality is nothing but status of being reasonable, sensible, and having good sense of judgment. Rationality is concerned with expected actions and results depending upon what the agent has perceived. Performing actions with the aim of obtaining useful information is an important part of rationality. What is Ideal Rational Agent? An ideal rational agent is the one, which is capable of doing expected actions to maximize its performance measure, on the basis of − Its percept sequence Its built-in knowledge base Rationality of an agent depends on the following four factors − The performance measures, which determine the degree of success. Agent’s Percept Sequence till now. The agent’s prior knowledge about the environment. The actions that the agent can carry out. 2.3.1 Rational Agent A rational agent always performs right action, where the right action means the action that causes the agent to be most successful in the given percept sequence. The problem the agent solves is characterized by Performance Measure, Environment, Actuators, and Sensors (PEAS). Figure : Rational Agent Work 22 2.4 INTELLIGENT AGENT Intelligent Agent An intelligent agent is anything that perceives the environment through it’s sensors and acts upon it through it’s actuators (effectors). The actions of the agent are always directed towards the goal. 2.4.1 Structure of Intelligent Agents Figure : Intelligent Agent The few types of agents are Human Agent : Sensors : Nose, Ears, Eyes, Tongue, Skin. Actuators : Hands, Legs, Mouth. Robotic Agent : Sensors : Camera, Infrared range finders. Actuators : Motors. Software agent : They have encoded bit strings as sensors and actuators. Agent’s structure can be viewed as − Agent = Architecture + Agent Program Architecture = the machinery that an agent executes on. Agent Program = an implementation of an agent function. 2.5 TYPES OF AGENTS TYPES OF AGENTS 1. SIMPLE REFLEX AGENTS 2.MODEL-BASED RELEX AGENTS 3. GOAL BASED AGENTS 4. UTILITY BASED AGENTS 5. LEARNING AGENTS 23 Artificial Intelligence 2.5.1 Simple Reflex Agents They choose actions only based on the current percept. They are rational only if a correct decision is made only on the basis of current precept. Their environment is completely observable. Condition-Action Rule − It is a rule that maps a state (condition) to an action. 2.5.2 Model Based Reflex Agents They use a model of the world to choose their actions. They maintain an internal state. Model − The knowledge about “how the things happen in the world”. Internal State − It is a representation of unobserved aspects of current state depending on percept history. Updating the state requires the information about − How the world evolves. How the agent’s actions affect the world. 24 2.5.3 Goal Based Agents Intelligent Agent They choose their actions in order to achieve goals. Goal-based approach is more flexible than reflex agent since the knowledge supporting a decision is explicitly modeled, thereby allowing for modifications. Goal − It is the description of desirable situations. 2.5.4 Utility Based Agents They choose actions based on a preference (utility) for each state. Goals are inadequate when − There are conflicting goals, out of which only few can be achieved. Goals have some uncertainty of being achieved and you need to weigh likelihood of success against the importance of a goal. 2.5.5 Learning Agents We've gone over different approaches for selecting actions in agent programmes. So far, we haven't detailed how the agent programmes are created. Turing (1950) examines the possibility of programming his intelligent machines by hand in his famous early article. 25 Artificial Intelligence It calculates the amount of time this will take and concludes, "Some more expeditious method appears desired." Building learning machines and then teaching them is the way he advocates. This is now the favoured strategy for developing cutting-edge AI systems in many fields. Another advantage of learning is that, as previously said, it allows the agent to operate in previously unknown contexts and to grow more proficient than its starting understanding would allow. The core concepts of learning agents are briefly introduced in this section. Throughout the book, we discuss learning possibilities and strategies for various types of agents. Part V delves deeper into the learning algorithm. As indicated in Figure, a learning agent can be separated into four conceptual components. The most crucial contrast is between the learning element, which is in charge of improving, and the performance element, which is in charge of deciding on external activities. The performance element is what we previously thought of as the full agent: it processes information and makes decisions. The learning element takes the critic's comments on how the agent is performing and decides how the performance aspect should be tweaked in order for the agent to perform better in the future. The learning element's design is heavily influenced by the performance element's design. When trying to create an agent that learns a specific capacity, the first thing to ask is "What type of performance factor will my agent need to do this once it has learned how?" rather than "How am I going to get it to teamthis?" Learning methods can be built to improve any aspect of an agent given its design. The critic informs the learning aspect of the agent's performance against a predetermined benchmark. Because the percepts do not provide any evidence of the agent's success, the critic is required. A chess programme, for example, might receive a percept indicating that it has checkmated its opponent, but it would need a performance standard to know that this is a good thing because the percept does 26 not state so. The learning agent's final component is the problem generator. It is in Intelligent Agent charge of recommending measures that will lead to new and educational experiences. The idea is that, given what it knows, if the performance factor had its way, it would continue to do the best activities. However, if the agent is prepared to experiment a little and take some potentially poor behaviours in the short term, it may uncover much better long-term activities. It is the role of the problem generator to suggest these exploratory actions. When scientists conduct experiments, they do them in this manner. Galileo did not consider dropping rocks from the top of a Pisa tower to be valuable in and of itself. He wasn't attempting to breach the law. 2.6 NATURE OF ENVIRONMENTS Some programs operate in the entirely artificial environment confined to keyboard input, database, computer file systems and character output on a screen. In contrast, some software agents (software robots or softbots) exist in rich, unlimited softbots domains. The simulator has a very detailed, complex environment. The software agent needs to choose from a long array of actions in real time. A softbot designed to scan the online preferences of the customer and show interesting items to the customer works in the real as well as an artificial environment. The most famous artificial environment is the Turing Test environment, in which one real and other artificial agents are tested on equal ground. This is a very challenging environment as it is highly difficult for a software agent to perform as well as a human. Turing Test The success of an intelligent behavior of a system can be measured with Turing Test. Two persons and a machine to be evaluated participate in the test. Out of the two persons, one plays the role of the tester. Each of them sits in different rooms. The tester is unaware of who is machine and who is a human. He interrogates the questions by typing and sending them to both intelligences, to which he receives typed responses. This test aims at fooling the tester. If the tester fails to determine machine’s response from the human response, then the machine is said to be intelligent. Properties of Environment 2.6.1 Natures of Environment The environment has multifold properties − Discrete / Continuous − If there are a limited number of distinct, clearly defined, states of the environment, the environment is discrete 27 Artificial Intelligence (For example, chess); otherwise it is continuous (For example, driving). Observable / Partially Observable − If it is possible to determine the complete state of the environment at each time point from the percepts it is observable; otherwise it is only partially observable. Static / Dynamic − If the environment does not change while an agent is acting, then it is static; otherwise it is dynamic. Single agent / Multiple agents − The environment may contain other agents which may be of the same or different kind as that of the agent. Accessible / Inaccessible − If the agent’s sensory apparatus can have access to the complete state of the environment, then the environment is accessible to that agent. Deterministic / Non-deterministic − If the next state of the environment is completely determined by the current state and the actions of the agent, then the environment is deterministic; otherwise it is non-deterministic. Episodic / Non-episodic − In an episodic environment, each episode consists of the agent perceiving and then acting. The quality of its action depends just on the episode itself. Subsequent episodes do not depend on the actions in the previous episodes. Episodic environments are much simpler because the agent does not need to think ahead. 2.6.2 PEAS Properties of Agent PEAS: Performance Measure, Environment, Actuators, & Sensors. Agent Performance Environment Actuators Sensors Type Measure Taxi driver Safe, fast, Roads, other Steering, Cameras, legal, traffic, accelerator, sonar, comfortable pedestrians, brake, signal, speedometer, trip, maximize customers horn, display GPS, profits odometer, accelerometer, engine sensors, keyboard Medical Healthy Patient, Display of Keyboard diagnosis patient, hospital, staff questions, entry of system reduced costs tests, symptoms, diagnoses, findings, treatments, patient's referrals answers 28 Intelligent Agent Agent Performance Environment Actuators Sensors Type Measure Satellite Correct image Downlink Display of Color pixel image categorization from orbiting scene arrays analysis satellite categorization system Part- Percentage of Conveyor belt Jointed arm Camera, joint picking parts in correct with parts: and hand angle sensors robot bins bins Refinery Purity, yield, Refinery, Valves, Temperature, controller safety operators pumps, pressure, beaters, chemical displays sensors Interactive Student's score Set of Display of Keyboard English on test students, exercises. entry tutor testing agency suggestions, corrections Examples of agent types and their PEAS descriptions SUMMARY This chapter gives the details about Artificial Intelligence agent and how it works, agent types, use of agent function, structure of intelligent agents, nature of environments and PEAS properties, various types of agent. QUESTIONS Q.1) What Is Intelligent Agent? Q.2) Define Agent Q.3) Explain Different Types of Agents. Q.4) Explain Peas Q.5) Discuss Different Natures of Environments in Detail. 29 Artificial Intelligence 3 PROBLEM SOLVING BY SEARCHING Unit Structure : 3.0 Objectives: 3.1 Introduction (Problem Solving) 3.1.1 Search 3.1.2 Importance of Search in AI 3.1.3 Problem Solving Agent 3.1.3.1 Steps in Problem Solving 3.1.3.2 Algorithm 3.1.3.3 Solutions to The Problem Solving 3.2 Uninformed Search Strategies: 3.2.1 Introduction (Uninformed Search) 3.2.2 Breadth First Search (BFS) 3.2.2.1 Concept 3.2.2.2 Implementation 3.2.2.3 Algorithm 3.2.2.4 Performance Evaluation 3.2.3 Depth First Search (DFS) 3.2.3.1 Concept 3.2.3.2 Implementation 3.2.3.3 Algorithm 3.2.3.4 Performance Evaluation 3.2.4 Uniform cost search (UCS) 3.2.4.1 Concept 3.2.4.2 Implementation 3.2.4.3 Algorithm 3.2.4.4 Performance Evaluation 3.2.5 Depth Limited Search (DLS) 3.2.5.1 Concept 3.2.5.2 Implementation 3.2.5.3 Algorithm 3.2.5.4 Performance Evaluation 3.2.6 Iterative Deepening DFS (IDDFS) 3.2.6.1 Concept 30 3.2.6.2 Implementation Problem Solving by Searching 3.2.6.3 Algorithm 3.2.6.4 Performance Evaluation 3.2.7 Bidirectional Search 3.2.7.1 Concept 3.2.7.2 Implementation 3.2.7.3 Algorithm 3.2.7.4 Performance Evaluation 3.2.7.5 Advantages of Bidirectional Search 3.2.7.6 Disadvantages of Bidirectional Search 3.2.8 Comparision of Searching Methods 3.2.8.1 Unidirecional and Bidirectional Search 3.2.8.2 Difference between BFS & DFS 3.2.8.3 Comparison of tree search strategies basis on performance evaluation 3.3 Informed Search Techniques 3.3.1 Heuristic 3.3.1.1 Introduction 3.3.1.2 Heuristic Search 3.3.1.3 Heuristic Search Techniques 3.3.1.4 Characteristics of Heuristic Search 3.3.1.5 Comparison of Blind Search and Heuristic Search 3.3.1.6 Heuristic Function 3.3.2 Best First Search : 3.3.2.2 Implementation 3.3.2.3 Algorithm 3.3.2.4 Performance Evaluation 3.3.3 Greedy Best First Search 3.3.3.1 Concept 3.3.3.2 Implementation 3.3.3.3 Algorithm 3.3.3.4 Performance Evaluation 3.3.4 A* SEARCH 3.3.4.1 Concept 3.3.4.2 Implementation 3.3.4.3Algorithm 3.3.4.4 Flow chart of A* search algorithm 31 Artificial Intelligence 3.3.4.5 Performance Evaluation 3.3.5 Memory Bounded Heuristic Search 3.3.5.1 Concept 3.3.5.2 SMA* : Simplified Memory Bounded A* 3.3.6 Local Search Algorithm and Optimization Problems 3.3.6.1 Hill Climbing 3.3.6.2 Local Beam Search 3.0 OBJECTIVES In this chapter we are going to learn about: what is problem, problem solving methods, problem solving agents. Study of Various searching techniques and types, comparison of searching techniques, Heuristic function etc. AI and related fields Logical AI : What a program knows about the world in general the facts of the specific situation in which it must act, and its goals are all represented by sentences of some mathematical logical language. The program decides what to do by inferring that certain actions are appropriate for achieving its goals. Search: AI programs often examine large numbers of possibilities, e.g. moves in a chess game or inferences by a theorem proving program. Discoveries are continually made about how to do this more efficiently in various domains. Pattern Recognition : When a program makes observations of some kind, it is often programmed to compare what it sees with a pattern. For example, a vision program may try to match a pattern of eyes and a nose in a scene in order to find a face. More complex patterns, e.g. in a natural language text, in a chess position, or in the history of some event are also studied. Representation : Facts about the world have to be represented in some way. Usually, languages of mathematical logic are used. Inference : From some facts, others can be inferred. Mathematical logical deduction is adequate for some purposes, but new methods of non-monotonic inference have been added to logic since the 1970s. The simplest kind of non-monotonic reasoning is default reasoning in which a conclusion is to be inferred by default, but the conclusion can be withdrawn if there is evidence to the contrary. For example, when we hear of a bird, we man infer that it can fly, but this conclusion can be reversed when we hear that it is a penguin. It is the possibility that a conclusion may have to be withdrawn that constitutes the non- monotonic character of the reasoning. Ordinary logical reasoning is 32 monotonic in that the set of conclusions that can the drawn from a set Problem Solving by Searching of premises is a monotonic increasing function of the premises. Common sense knowledge and reasoning : This is the area in which AI is farthest from human-level, in spite of the fact that it has been an active research area since the 1950s. While there has been considerable progress, e.g. in developing systems of non-monotonic reasoning and theories of action, yet more new ideas are needed. Learning from experience : Programs do that. The approaches to AI based on connectionism and neural nets specialize in that. There is also learning of laws expressed in logic. Programs can only learn what facts or behaviors their formalisms can represent, and unfortunately learning systems are almost all based on very limited abilities to represent information. Planning : Planning programs start with general facts about the world (especially facts about the effects of actions), facts about the particular situation and a statement of a goal. From these, they generate a strategy for achieving the goal. In the most common cases, the strategy is just a sequence of actions. Epistemology : This is a study of the kinds of knowledge that are required for solving problems in the world. Ontology : Ontology is the study of the kinds of things that exist. In AI, the programs and sentences deal with various kinds of objects, and we study what these kinds are and what their basic properties are. Emphasis on ontology begins in the 1990s. Heuristics : A heuristic is a way of trying to discover something or an idea imbedded in a program. The term is used variously in AI. Heuristic functions are used in some approaches to search to measure how far a node in a search tree seems to be from a goal. Heuristic predicates that compare two nodes in a search tree to see if one is better than the other, i.e. constitutes an advance toward the goal, may be more useful. Genetic Programming : Genetic programming is a technique for getting programs to solve a task by mating random Lisp programs and selecting fittest in millions of generations. 3.1 INTRODUCTION (PROBLEM SOLVING) Search and Control Strategies Problem Solving Problem solving is an important aspect of Artificial Intelligence. A problem can be considered to consist of a goal and a set of actions that can be taken to lead to the goal. At any given time, we consider the state of the search space to represent where we have reached as a result of the actions we have applied so far. For example, consider the problem of looking for a contact lens on a football field. The initial state is how we start out, which is to say 33 Artificial Intelligence we know that the lens is somewhere on the field, but we don’t know where. If we use the representation where we examine the field in units of one square foot, then our first action might be to examine the square in the top- left corner of the field. If we do not find the lens there, we could consider the state now to be that we have examined the top-left square and have not found the lens. After a number of actions, the state might be that we have examined 500 squares, and we have now just found the lens in the last square we examined. This is a goal state because it satisfies the goal that we had of finding a contact lens. 3.1.1 Search Search is a method that can be used by computers to examine a problem space like this in order to find a goal. Often, we want to find the goal as quickly as possible or without using too many resources. A problem space can also be considered to be a search space because in order to solve the problem, we will search the space for a goal state. We will continue to use the term search space to describe this concept. In this chapter, we will look at a number of methods for examining a search space. These methods are called search methods. 3.1.2 Importance of Search in AI It has already become clear that many of the tasks underlying AI can be phrased in terms of a search for the solution to the problem at hand. Many goal based agents are essentially problem solving agents which must decide what to do by searching for a sequence of actions that lead to their solutions. For production systems, we have seen the need to search for a sequence of rule applications that lead to the required fact or action. For neural network systems, we need to search for the set of connection weights that will result in the required input to output mapping. 3.1.3 Problem Solving Agent Problem formulation is the basic step of problem solving agent where problems defined by using five components. 3.1.3.1 Steps in Problem Solving Problem can be defined formally using five components as follows: 1. Initial state 2. Actions 3. Successor function 4. Goal test 5. Path cost 34 1. Initial state : The initial state is the one in which the agent starts in. Problem Solving by Searching 2. Actions: It is the set of actions that can be executed or applicable in all possible states. A description of what each action does; the formal name for this is the transition model. 3. Successor function : It is a function that returns a state on executing an action on the current state. 4. Goal test : It's a test to see if the present state is a goal state or not. In some cases, a goal test can be performed simply by comparing the current state to the declared goal state, which is known as an explicit goal test. The implicit goal test is used when the state of a problem cannot be described directly and must be generated by doing some computations. Example: In Tic-Tac-Toe game making diagonal or vertical or horizontal combination declares the winning state which can be compared explicitly, but in case of chess game, the goal state cannot be predefined but it’s a scenario called as “Checkmate” which has to be evaluated implicitly. 5. Path cost : It is simply the cost associated with each step to be taken to reach to the goal state. To determine the cost to reach to each state, there is a cost function, which is chosen by the problem solving agent. 3.1.3.2 Algorithm Procedure or method : Problem solving agent, agent may be unknown, space, percept. Result : An Action. Input : P → percept (Environment perception) Static : 1) A → Ab action sequence, initially with null value. 2) S → State – current state. 3) G → Goal – A goal initially null. 4) P → Problem – A real world situation. State – update state (State, Percept) If (s) is empty then do g → Formulate goal/goals P → Formulate problem (s,g) S → Search (p) G First (s) First(s) → G Rest(s) → S Return a Procedure 35 Artificial Intelligence Which search algorithm one should use will generally depend on the problem domain? There are four important factors to consider: Completeness – Is a solution guaranteed to be found if at least one solution exists? Optimality – Is the solution found guaranteed to be the best (or lowest cost) solution if there exists more than one solution? Time Complexity – The upper bound on the time required to find a solution, as a function of the complexity of the problem. Space Complexity – The upper bound on the storage space (memory) required at any point during the search, as a function of the complexity of the problem 3.1.3.3 Solutions to The Problem Solving Problem Solution A well -defined problem with specification of initial state, goal test, successor function, and path coast it can be represented as a data structure and used to implement a program which can search for a goal test. A solution to a problem is a sequence of action chosen by the problem solving agent that leads from the initial state to a goal state. Solution quality is measured by the path cost function. Optimal Solution An optimal solution is the solution with least path cost among all solutions. General sequence followed by a simple problem solving agent is, first it formulates the problem with the goal to be achieved, then it searches for a sequence of actions that would solve the problem, and then executes the actions one at a time. 3.1.3.3 EXAMPLE Examples of Problem Solving By using Solving Problems by Searching, there are five different components for defining a problem. Components for defining a problem 1) Initial State 2) Actions Available 3) Transition Model 4) Path Cost 5) Goal Test Example – 1) Romania Map Problem Map of Romania : Romania is a country in Europe, following are the major cities. 36 Problem Solving by Searching Description of Map Problem 1) The initial state : The initial state for our agent in this case (Romania) might be described as In(Arad). 2) Actions : It gives the possible actions from the current state, in this case the possible actions are (Go(Sibiu), Go(Timisoara), Go(Zerind)}. 3) Transition Model : This is Specified by using a function RESULT(s,a), that returns the state that results from doing action a in state s. RESULT(In(Arad),Go(Zerind)) = In(Zerind). 4) Path Cost : Path cost function assigns a numeric cost to each path, In the present case it is the distance in kilometers. 5) Goal Test : It determines whether the given state is Goal State. Example – 2) Vacuum-Cleaner Problem Vacuum World : 37 Artificial Intelligence 1) State : The state is determined by both the agent location and the dirt locations. There are 8 possible world states. 2) Initial state : Any state can be designated as the initial state. 3) Actions : Each state has just three actions : 1. Left 2. Right 3. Suck. 4) Transition model : Action left takes the agent to Leftmost square, Action Right takes the agent to Rightmost square and the Suck action cleans a dirty square and performs no action on clean square. 5) Goal test : This checks whether all the squares are clean. 6) Path cost : Each step costs 1, so the path cost is the number of steps in the path. Example – 3) 8 Queens Problem 1) States : Any arrangement of 0 to 8 queens on the boards is a state. 2) Initial state : Empty board i.e. No queens on the board. 3) Actions : Adding a queen to any empty square. 4) Transition model : Returns the board with a queen added to the specified square. 5) Goal test : 8 queen are on the board, in such a way that none is in attacking position. Example – 4) Route-finding problem It is same as Romania map problem 1) States : Each state includes a location, the current time, cost of an action (a flight segment) 2) Initial state : This is specified by the user (the starting point) 3) Actions : Take any flight from the current location, in any seat class, leaving after the current time. 38 4) Transition model : The state resulting from taking a flight will have Problem Solving by Searching the flight’s destination as the current location and the destination time as the current time. 5) Goa test : Check if the Final destination has been reached 6) Path cost : This depends on monetary cost, waiting time, flight time, customs and immigration procedures, seat quality, time of day, type of airplane etc. Example – 5) The Travelling Salesperson Problem (TSP) 1) States : All the cities which are to be visited by the salesman. 2) Initial State : Any city can be the initial state. 3) Actions : The agent can visit any city which is not yet visited from the current city. 4) Transition model : The next city becomes the current city 5) Goal test : Check if all the cities have been visited with minimum cost & also the final state is the initial state. 6) Path cost : This depends on the actions the agent has taken throughout the journey. Measuring Problem Solving Performance There are three possible outcomes for any problem. 1) We reach at failure state 2) We reach at solution state 3) Algorithm might get stuck in an infinite loop. Problem solving performance can be evaluated with 4 various factors as follows: 1) Completeness 2) Optimality 3) Time complexity 4) Space complexity There are two Basic Search Strategies Figure : Types of search strategies 39 Artificial Intelligence 1) Uninformed search : It is also known as blind search. In this strategy only the initial and goal state is given and no additional information is available. 2) Informed Search : It is also known as Heuristic search. Some additional information apart from the initial and the goal state is given, through which we know which state out of the explored is more beneficial. 3. 2 UNINFORMED SEARCH STRATEGIES 3.2.1 Introduction (Uninformed Search) They do not have any additional information Information provided in the problem definition only To reach at goal state using different order or length of actions. It does not use knowledge in the processing of search. It is time consuming for getting the solution. It is always complete. It is expensive It requires moderate time It is lengthy for implementation Examples as follows 1. Breadth First Search (BFS) 2. Depth First Search (DFS) 3. Uniform cost search (UCS) 4. Depth Limited Search (DLS) 5. Iterative deepening DFS (IDDFS) 6. Bi-directional search Informed Search Strategies / Heuristic Function It contains information on goal state. It helps in search efficiently. The information is obtained by a function which will helps to estimate how close a current state is from the goal state. It uses the knowledge in the processing of searching. It helps to find the solution quickly. It may be or may not be complete. It is inexpensive It requires less time 40 It gives the direction about the solution. Problem Solving by Searching It is less lengthy to implement. Examples of Informed Search 1) Best First Search 2) Greedy best first Search 4) A* Search 5) Memory bounded heuristic Search 3.2.2 Breadth First Search (BFS) Uninformed Search Strategies: 3.2.2.1 Concept Breadth First Search: The root node is expanded first, then all the successors of the root node are expanded next, then their successors, and so on. The shallowest unexpanded node is chosen for expansion. 3.2.2.2 Implementation This is achieved very simply by using a FIFO (First In First Out) Queue for the frontier. The goal test is applied to each node when it is generated rather than when it is selected for expansion. 3.2.2.3 Algorithm 1. Put the root node on a queue 2. While (queue is not empty) a) Remove a node from the queue if node is a goal node then return success; put all children of node onto the queue; 3. Return failure; 3.2.2.4 Performance Evaluation 1) Completeness : It is complete, provided the shallowest goal node is at some finite-depth. 2) Optimality : It is optimal, as it always finds the shallowest solution. 3) Time complexity : O(bd), number of nodes in the fringe. 4) Space complexity : O(bd), total number of nodes explored. 41 Artificial Intelligence Process of Breadth First Search 42 Problem Solving by Searching 3.2.3 Depth First Search (DFS) 3.2.3.1 Concept Depth first search always expands the deepest node in the current frontier of the search tree. The search proceeds immediately to the deepest level of the search tree, where the nodes have to successors. As the nodes are expanded, they are dropped from the frontier, so then the search backs up the next deepest node that still has unexplored successors 3.2.3.2 Implementation Depth first search uses a LIFO (Last In First Out) queue. A LIFO (Last In First Out) queue means that the most recently generated node is chosen for expansion. 3.2.3.3 Algorithm For Recursive implementation of DFS DFS (c) : Step 1. If node is a goal, return success; Step 2. For each child c of node If DFS ( c ) is successful, Then return success; Step 3. Return fai