Cladistics (Koch) PDF
Document Details
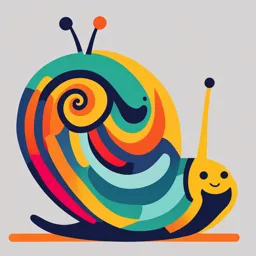
Uploaded by AppreciableDjinn4959
Universität Bonn
Tags
Summary
This document is a study of cladistics, a method for classifying organisms based on shared derived characteristics. It examines the concepts of homology, character analysis, and tree construction, with practical examples and case studies.
Full Transcript
Species → names are binomial: Consist of the name of the genus and a specific epithet. Aristotelian definition → definitio fi(a)t per genus proximum et differentiam specificaI: A genus-differentia definition is a type of intensional definition, and it is composed of two parts:...
Species → names are binomial: Consist of the name of the genus and a specific epithet. Aristotelian definition → definitio fi(a)t per genus proximum et differentiam specificaI: A genus-differentia definition is a type of intensional definition, and it is composed of two parts: o A genus → an existing definition that serves as a portion of the new definition. All definitions with the same genus are considered members of that genus; o The differentia → the portion of the definition that is not provided by the genus. Nomenclatural author of Homo sapiens → Carl von Linné (1707-1778): Species description in Systema naturae (1758). Species (voucher/type material): Must be deposited in a museum or collection for reference, together with the species description, which must be published in a publicly available manner. Universal meter: A copy of the "provisional" meter installed in 1796-1797, located in the wall of a building, 36 rue de Vaugirard, Paris; A platinum bar of 1 meter length was deposited in the Museum National d'Histoire Naturelle in Paris for universal reference. Taxonomy: Information science; Hypothesis-based science: o Characters, character distribution, species delimitation, phylogenetic relationship; Intersubjective testability. What is a species? Resemblance of the shapes of their parts or the whole body → Aristotele (350 BC); A species is a community, or a number of related communities, whose distinctive characters are, in the opinion of a competent systematist sufficiently definite to entitle it, or them, to a species name → Regan (1878-1943) → not testable; All members of a species are able to produce fertile offspring under natural conditions → biological species concept, new synthesis. Testability easy for sympatric species (overlapping geographical area), difficult for allopatric species (geographically isolated). Why do we need species at all? o Species are the entities of generalisation: ▪ Data from a sufficient number of individuals can be regarded as representative for all members of a species. Hennig's species concept: Interbreeding over time can lead to a reproductive barrier; Some populations are wiped out by parasites, predators, etc; Left populations will continue inbreeding creating new traits and species. Phylogenetic systematics: Only to natural entities in nature → species and monophyletic groups (taxa); Autapomorphies substantiate taxa (derived trait unique to a given taxon). Evolutionary novelty; Synapomorphies reveal sister groups (same mother/ancestor). Character that evolved in the common stem species of two species; Plesiomorphies → former autapomorphies, evolved earlier than in the last common stem species. Any "old" character that evolved prior to the last common stem species; Linnean classification causes monophyletic (single common ancestor and of its descendants), paraphyletic (single common ancestor and some of its descendants) and polyphyletic (does not include a recent common ancestor, taxa may have more than one ancestor between them) taxa → only the former present natural entities in nature; Fossil taxa can be included; Out group → every other group in the periphery of the chosen group. Allows to polarise characters in term of primary (plesiomorphy) and derivate (autapomorphy). Homology and observation assessment: Evolutionary evaluation: o Identity → evolutionary transition without modification; o Similarity: ▪ Evolutionary transition with modification (transformation): ▪ Evolutionary novelty → homoplasy, convergent evolution. The decision is not always easy → are bird wings homoplasious (evolved convergently), because they serve the same function to able to move in a medium of low density or are they homologous, because the common ancestor of all extant birds evolved wings to conquer a new ecological niche? Statements on homology are always hypotheses that must be substantiated: Rationales are: o Position; o Special quality of structures as being composed of the same or mostly the same substructures; o Evidence for intermediates from ontogenesis or the fossil record; 1. Identical position; 2. Identical composition; 3. Identical development; 4. Extinct intermediates. Homology is a rational term: The wings of a bird and a bat are: o Homologous as anterior body appendage; o Homoplasious (convergently evolved) as wings. Recognition and identification of morphological structures is always along with detecting known patterns and thus with experience → morphological diagnostics. Morphology as a diagnostic science has to cope with complex structures: Requires: o Knowledge of terms; o Criteria to recognise morphological features: ▪ Topographical position; ▪ Shape/form; ▪ Substructure; o Experience in recognition; Why are we able to recognise structures as identical across borders? o Abstraction during recognition of features within and across species: ▪ Conservative substructures are recorded; ▪ Variation is neglected. Ontologies for standardising structure concepts: The resource description framework (RDF): o Subject → property → object; o RDF descriptions allow coping with the overwhelming structural complexity Cladistics: Construction of dendrograms from character/taxa datasets using the maximum parsimony method: o Computational to improve: ▪ Scope of data collection; ▪ Objectivity; ▪ Transparency; 1. Compilation of characters and their states in a matrix; 2. Cladistic analysis of the matrix based on optimality criterion (maximum parsimony); 3. Character optimisation → evolutionary inferences from optimal tree(s). Species must find a trade-off between functional and historical constraints. Homology assumptions are tested in cladistic analyses (e.g. wing evolution in birds): The optimal tree(s) confirms the homology assumption, if it proves to be congruent with other characters in support of the view that: o Birds form a monophylum (Aves); o The basal lineages among birds comprise birds capable of flying; The optimal tree(s) rejects the homology assumption, if it proves to be incongruent with other characters that support the view: o Birds are para- or polyphyletic; o Flightless species are resolved as basal lineages among birds; False homology assumptions cause conflict (inconsistencies) in a dataset; Phylogeny interference is an iterative cycle during which primary homology hypotheses are tested for congruence; Primary homology hypotheses need to be well-reasoned: o Rationales are: ▪ Relative position; ▪ Special quality of structures as being composed of the same or mostly of the same substructures; ▪ Evidence for intermediates from ontogenesis or the fossil record; ▪ Applies to Hennigian argumentation in the same manner as to cladistics; Abstraction during structure analysis within and across species to recognise patterns → degree of correspondence: o Conservative substructures are recorded; o Variation is neglected. The evolution of segmented body plans in animals: True segmentation → how many substructures must be arranged serially? o 1 pair of coelomic cavities? At least in embryos? o 1 pair of metanephridia? o 1 pair of parapodia/limbs? o 1 pair of ganglia (ladder-like CNS)? o 1 pair of longitudinal muscles (myomeres); o 1 pair of chaetae? A common pattern in gene expression? o A corresponding set of oscillating segmentation genes (molecular "segmentation" clock)? Objection → are the substructures logically independent? Choice of terminal taxa: Ground pattern approach: o If clades above the species level are used as terminals, the coded states: ▪ Either are assumed for all members of this clade; ▪ Refer to the putative ancestral state of this clade (stem- species); o In either case, the stated codings refer to assumptions, not to observations; Exemplar approach → species as terminal taxa: o The coding of character states should preferably refer to species, because: ▪ They represent the basic "entities of generalisation" in biology: ▪ The observations are reproducible. Polymorphic character state (trait consisting of two stages): All character states in a matrix refer: o Either to the exemplar species stated as terminal taxa; o In case of terminals above the species rank (families, orders, etc) to the putative stem-species or the terminal taxon → unless stated otherwise; Polymorphisms in a matrix accordingly specify: o Either intraspecific variation; o Uncertainty about the ancestral state for a supraspecific terminal. Applicability versus missing states: In cladistic analyses, inapplicable and missing states are treated in the same manner. In terms of knowledge representation, however, this distinction is fundamentally important; Inapplicable → structure need the presence of another structure but this structure it is not present. Coding strategies: Absent/present coding: o 0 = absent, 1 = present; o Observations are conceptualised in the character description → "diagnostic coding", usually preferred by taxonomists; Multistate coding: o 0 = state 1, 1= state 1, 2 = state 3, etc; o Observations are described as specific character states → e.g. a certain structure is rectangular, circular, or oval; Example: o Wings → (0) absent, (1) present; o Anterior limbs as wings → (0) absent, (1) present: o Anterior limbs made of bones as wings → (0) absent, (1) present: ▪ Anterior limbs made of bones as wings, composition → (0) 3 radii, (1) 5 radii, with patagium; o Anterior limbs as wings mad of 3 radii and feathers → (0) absent, (1) present; o Anterior limbs as wings made of 5 radii and patagium → (0) absent, (1) present; o Do penguins have wings? And ostriches? All these codings differ in their homology statement and applicability; Functional terms are generally problematic for character descriptions: o Potential solution in our example: ▪ Anterior limbs made of 3 bony radii and feathers, function → (0) as wings for flying, (1) as fins for swimming, (2) involved in fast running to keep balance and as brakes (and ritual purposes during mating); Ordering characters to weight specific transformations → 1 step transformations preferred; Problem → absent/present vs distinct states refer to different kinds (interpretations vs observations) that should not be mixed within a single character description; Solution → discrimination of neomorphic (mutated gene produce a novel function) and transformational characters (Sereno coding). Transformational character states must be discrete: Character a → state 0 = circular, state 1 = oval; Character a → state 0: r1 = r2, state 1: r1 = 1.5r2, state 2: r1 = 2r2, state 3 r1 = 3r2; Intergradational variation: o For continuous data it is absolutely necessary to transform them into discrete character states → e.g. by stating clear, non-overlapping ranges. One attempt to delimitate range as discrete states is the cluster analysis; Characters must be logically independent. Logical independence of characters: Ambiguity → both states are potentially amorphic (first image); Sereno coding → only 1 state is potentially apomorphic (unique to a group or species, second image). Tree calculation: Maximum parsimony: o The optimal (most parsimonious) tree is the one that implies the least number of character (state) transformations → the shortest tree; o Expressed in the tree length → number of evolutionary steps. The conflict between competing optimal trees is expressed in a consensus tree: The strict consensus tree only depicts the nodes present in all optimal trees; all other nodes are collapsed; The strict consensus tree is a "suboptimal" tree and should never be used for tracing character evolution (optimisation). Solution of the conflicts → more taxa, more characters: The optimal (most parsimonious) tree is the one with the least number of evolutionary steps and is therefore preferred. Trees with a longer length are considered "suboptimal"; Consensus trees are per se "suboptimal" → unresoled nodes increase the number of evolutionary steps, the consensus thus is longer than the optimal trees used to calculate the consensus. Tree calculation → search algorithms: Exact search → all possible trees are calculated; Heuristic search → many random subsets of all possible trees are calculated. Rooted vs unrooted trees: Composition of a phylogenetic tree: o Nodes interconnected by branches; o The branching pattern is dichotomous; o The basal most node is the root (node); o A terminal node is a leaf (node); Nodes represent stem species, branches their stem lineage. Rooted and unrooted trees do not differ in their tree length: A root is only needed to determine the direction of change. For the calculation of the shortest tree(s) the direction is irrelevant. Algorithms for heuristic searches: Stepwise addition → Wagner trees: o Based on an "initial tree" made of 3 taxa, additional taxa are added stepwise. when all taxa are included in the tree, its final length is calculated and kept in the memory; o This procedure is iterated until about 1,000 replicates are produced; Rearrangements: o For each replicate retrieved from stepwise addition, about 100 new trees are randomly produced by rearrangement of any node in teh replicate; o This branch swapping in the replicates randomly produces a subset of maximum 100,000 possible trees that are calculated for their length; o SPR → subtree Pruning and Regrafting; o TBR → Tree Bisection and Reconnection; o Branch swapping: ▪ Local rearrangement of taxa → NNI (Nearest-Neighbour Interchange); ▪ Global rearrangement after sectioning and random reconnection of the sections. Evaluation of the optimal tree(s): Indices and algorithms to determine: o Congruence → Consistency Index (CI), Homoplasy Index (HI), Retention Index (RI); o Robustness → Bootstrap support/Jackknife support, Bremer Support; o Sensitivity → Weighting characters. Character evolution → optimisation: Polarity: o Character states are interpreted on the optimal tree(s) as transformations: ▪ From present to absent → loss; ▪ From absent to present → gain; ▪ From large to short → reduced; ▪ From short to large → enlarged; ▪ Present after reversal → regain; ▪ Etc; Ambiguity: o Transformations are considered ambiguous if two possible interpretations are equally likely: ▪ Fast transformation (AccTran = Accelerated Transformation): Changes occur early at deeper nodes but may reverse to ancestral state; ▪ Slow transformation (DelTran = Deleted Transformation): The ancestral state is primarily maintained, changes occur convergently; Ancestral states. The standard documentation in publications: The single optimal tree or the strict consensus of the optimal trees, including stats (tree length, CI, RI, RSC, etc) and nodal support values; Optimisation of all unambiguous transformations on optimal tree (not on strict consensus); Optional → tree resolutions retrieved from sensitivity analyses (implied weighting). In cladistics, molecular data seem to outbalance morphological data, but why? Quantity of characters → the largest morphological matrices include about 3,000 characters; Simplicity of genetic sequences (ATCG) → allows application of evolutionary models. Molecular phylogenetics: How to compute phylogenetic relationships with information from molecular genetics → nucleotide or protein sequences. Case studies: Hypotheses on the origin of Darwin's finches: o Multiple colonisations by different finch species? ▪ Different species of Darwin's finches should have closest relatives elsewhere; o Single colonisation then diversification? ▪ Darwin's finches should form monophyletic group; o Colonisation from Isla de Coco? ▪ Cocos finch should be sister species to a clade with all other Darwin's finches; o Phylogenetic evidence supports single colonisation; Human evolution: o Multiregional model of human origins: ▪ Homininae across Old World were a single species connected by gene flow; ▪ Multiregional differences are the result of local adaptation; o Out-of-Africa model of human origins: ▪ All human populations are derived from recent African ancestry; o Phylogenetic tree strongly supports Out-of-Africa scenario; Human immunodeficiency virus (HIV): o Three separate jumps from apes to human. Building a phylogeny with genetic data: Each nucleotide may be informative; But homoplasy (convergence) is common: o Only 4 possible character states (A, T, C, G); Genes differ in their rates of evolution: o Slowly evolving genes useful for distantly related species; o Rapidly evolving genes useful for closely related lineages. Steps of a phylogenetic analysis: Identify/select genetic markers; Alignment of sequences; Selection of analysis method(s) → modelling of sequence evolution; Getting support statistics; Timing of evolutionary events → molecular clock; Interpreting patterns of evolutionary change. Genetic markers for phylogenetic studies: Depending on the level of analysis, will be selected: o More variable markers (populations/related taxa); o More conserved markers (higher ranking taxa). Genetic loci have their own genealogy: Alleles in populations coalesce to a common ancestor → model of population genetics that traces all alleles of a gene in a sample from a population to a single ancestral copy shared by all members of the population. Coalescence time varies for alleles of different genes: Gene trees do not always match species trees: Homology of genes: Only homologous traits are used as information in phylogenetic studies; Genes can be homologous in two different ways because they have a common ancestor due to: o Species splits (orthologs) → separation of two populations with the ancestral gene into two species → speciation; o Gene duplications (paralogs) → gene duplication of the ancestral gene within a lineage. Orthologous and paralogous genes: Orthologs and paralogs are both homologous → they have a common ancestor (before species split or before gene duplication); Only orthologous genes are used in phylogenetic reconstruction → otherwise we reconstruct the history of gene duplications in a gene family and not that of species splits in a group of species. Orthologous and paralogous haemoglobins: How to identify orthologs in large genomic datasets: Genomic datasets (whole set of genes/protein sequences): o All genes will be compared to the set from another organism → with the blast method; o Only those which have reciprocal best hits with each other will be rated as orthologs (mouse 1 & rat 4, mouse 5 and rat 8, mouse 8 and rat 2); Alternatively use a set of predefined markers, that have a good chance to consist of only single copy orthology → using BUSCO (Benchmarking Universal Single-Copy Orthology). What are alignments? Alignments provide: o Positional homology hypotheses inside sequences; o Homologous nucleotide or amino acid sequences of different organisms are aligned to get corresponding positions of single sites in the sequence; o Hypothesis → nucleotide positions are homologous. In/dels (Insertionen/Deletionen): Unaligned sequences are often different in length → due to Indels; For an alignment, gaps have to be included, corresponding Indel-events. Nucleotide substitutions: The probability of different substitution types differs; Alignment procedure can follow rules to minimise rare substitution events. Some definitions: Some facts: Several different alignments are possible for a given pair of sequences; Two sequences can always be aligned (even random sequences): o Different sequence alignments can be scored. Alignment strategy trade-off: Scoring matches (+) and gaps (-): Addition of gaps itself scores negatively but this may be outweighed by increasing the number of matches. Gap cost: Ɣ(g) = - gd; Differentiating gap opening cost and elongation cost: o Ɣ(g) = -d - (g – 1)e; Ɣ(g) = cost of gap with length g; d = gap opening cost; e = gap elongation cost: g = length of gap; Gap opening cost is much higher than gap elongation cost. Pairwise alignments with dot plots: Smith-Waterman-Algorithm (pairwise alignment): Finds the best scoring local alignment(s) for a pair of sequences; Best scoring local alignments. Needleman-Wunsch-Algorithm (pairwise alignment): Finds the best scoring global alignment(s) for a pair of sequences; Best scoring full sequence alignment. Multiple alignments: Exact strategy: o All possible multiple alignments are scored and the best one is chosen (too much computing time when >10); Heuristic method: o Try to find a good solution that comes close to the best one in a short amount of time; Approximation alignments: o All combinations of pairwise alignments will be generated; o From pairwise distances a "guide tree" is calculated; o Following this tree the sequences will be aligned on by one. Problems with multiple alignments: A "guide tree" guides the alignment procedure. The tree is quickly calculated and probably guides an erroneously alignment by making false assumptions; Solution → start with several different starting trees; Different parameters lead to different alignments → regions with many gaps may better be excluded from the analysis; Heuristic methods probably do not find the best scoring alignment. Alignment tool for data base search → BLAST: "Sequence query" is cut into "words" of 11 nucleotides (all possible words) → this list is compared to all database entries; BLAST (Basic Local Alignment Search Tool): Hits will be tested for elongation; Several hits for the same database entry → addition of scores. How to describe a tree without a graph: Newick format; We can rotate a tree around each node and obtain the same topology. Some other trees: Cladogram: o Branch length without meaning; o Only important thing is topology; Phylogram: o Branch lengths reflect evolutionary change (substitutions); Ultrametric tree: o Internal nodes reflect time of branching → when the split happened. Reconstruction methods of phylogenetic trees from sequence alignments: Maximum parsimony approach → least number of changes over time; Distance based methods → pairwise comparison of sequences; Maximum likelihood → best explanation according to substitution model; Bayesian methods → as maximum likelihood but with tree evaluation. Principle of parsimony: The simplest explanation tends to be the best one; In phylogenetics: o The same character state in two species is most easily explained by being inherited from the last common ancestor of both species. Maximum Parsimony (MP) method: Evaluating all alignment positions for substitutions and selection of the tree hypothesis which minimises the number of changes; Using alignments, each site of the alignment is a character; and the corresponding nucleotides are the character states; Together with a tree topology we can estimate the number of changes. Tree length → number of changes of character states: e.g. sum of substitutions for each position of the aligned sequences; Maximum parsimony method tries to find the shortest tree for a given character set. Optimality criterion: The shortest tree needs the least number of substitution hypotheses; Least number of homoplasious events. Example of an analysis: We may discriminate between phylogenetically informative and non- informative characters; Only characters with * are informative → only characters needed to evaluate trees; Only informative characters differ in their "score" for different trees; Uninformative sites (invariable and singleton). Only phylogenetically informative characters are needed to evaluate trees → computationally faster to omit non-informative sites from the alignment. All possible trees will be evaluated for their length → number of character changes: The shortest tree is the most parsimonious explanation of the evolution of the character set → a good hypothesis for the evolutionary history. When there are too many trees for an exact search, heuristic search strategies will be used → only a subset of trees is tested. Distance methods: Distances → differences counted in pairwise comparison of aligned sequences; Distances may reflect evolutionary history; Sequence data may be analysed as: o Distances → number of differences; o Single characters. Differences to character-based methods (like maximum parsimony): No ground pattern reconstruction; Some information is lost by transforming characters to distances. Tree reconstruction with distances: UPGMA → Unweighted Pair-Group Method with Arithmetic Mean; Neighbour-joining method. UPGMA: A simple clustering method; Originally developed for phenotypic similarities; A tree is step-wise reconstructed: o Two sequences which are most similar are combined on a branch → becomes a composite sequence; o A new distance matrix is built with the composite sequence → mean distance to the composed sequence; o Again, the two most similar sequences are selected; Problem for UPGMA: o Differences in substitution rate → wrong topology (long-branch attraction). Neighbour-Joining Method: Clustering method; Step by step neighbours will be found which minimise total length of the tree; Very similar to UPGMA, but a correction is done by adding a mean difference to all of the other taxa: For all taxa A, B, C, D, the mean distance u(X) is computed: o u(A) = [Dist(A,B) + Dist(A,C) + Dist (A,D)]/n-2; All distances will be corrected (X.Y)-u(X)-u(Y), the rest is similar to UPGMA. Distance between two sequences: L → length of alignment; D → differences; Visible difference (p distance): o p = D/L. Nucleotide substitutions: Single substitution; Multiple substitutions; Parallel substitutions; Convergent substitutions; Back substitutions. Difference between expected and visible substitutions: Difference between visible and real distance: Visible nucleotide differences (p) and real number of nucleotide substitutions (evolutionary distance, Dist); Dist is computed from p → transformation/correction with evolutionary model. Number of substitutions per time follows a Poisson distribution: Jules-Kantor Model → transformation from p distance: 3 4𝑝 Dist = - 4 ln [1 − 3 ]; p = rate of change. Usually, the models only handle substitutions → insertions/deletions are not modelled. Transitions are more likely to occur than transversions → less energy required. Models of nucleotide substitution: Estimation of substitution probabilities; Not directly measurable; Comparative methods/statistics. Simple evolutionary models: Primary assumptions: o Probability of substitutions (Pik) constant over time; o Base composition is constant over time; More assumptions: o Evolution of sequences follows Markov model: ▪ Substitutions in different lines are completely independent from each other; ▪ Pik in any sequence position is independent from any previous change; ▪ Homogeneous model → model does not change in different lineages. Models: Jukes and Cantor → 1 parameter: o Transitions and transversions have the same probability to occur; Kimura two parameter (K2P): General time reversible (GTR): Comparison of real data to different models of nucleotide substitution: Too many variables have to be estimated, add more errors in assumptions → realistic models give better results in phylogenetic analysis (too few parameters give unrealistic models). Maximum likelihood methods: Trying to combine the best parts of: o Distance methods → substitution models; o Maxximum parsimony → character based. Computing likelihood: Suppose we are given the followings: o Tree topology; o Observed data, X = {a:G, b:G, c:T, d:G}; o Ancestral sequences Y = {e:?, f:?, g:?}: ▪ Without ancestral sequence you need to try them all; o Parameters, x = {, tae, tbe, tcf, tdf, teg, tfg}; Likelihood = Pr(teg) x Pr(tfg) x Pr(tae) x Pr(tbe) x Pr(tcf) x Pr(tdf). Computational efficiency: How much computational is needed? For our example (compute likelihood of 1 tree): o 3 internal nodes → 43 = 64 possible sets of ancestral states; o For each set of ancestral states, we need to multiply 7 terms → because there are 7 nodes in the tree; In general: o If there are n input sequences, there are n-1 internal nodes → 4n-1 possible sets of ancestral states; o For each set of ancestral states, we need to multiply n+n-1 = 2n-1 terms; o These computations have to be done for many trees; Impractical to perform this exponential number of operations: o Only solvable by dynamic programming approaches. Solving the small likelihood problem: Then how to find the optimal parameter values? o Start with a random estimate of Ө; o Apply a hill climbing algorithm: ▪ Change the value of a parameter so that the likelihood is increased; ▪ Repeat itself for each parameter in turn, for multiple iterations; ▪ Will reach maximum if there is a single peak → this is true in many real situations, though theoretical cases can be constructed in which this is not true; o Finding optima in a complex parameter landscape by running several analyses with different starting points. Every kind of method yields a tree → but how reliable it is? Testing trees: o Resampling methods: ▪ Evaluate the robustness of internal branches through reconstruction of many trees from subsets of databases derived from the original dataset → bootstrapping; o Bootstrapping (1st step): ▪ From the original dataset, a number of "pseudoreplicates" is generated: o Pseudoreplicate: ▪ Each column of the new data matrix is filled with a random column of the old one; ▪ In the end some of the columns (alignment positions) are not found, others one time, two times, or even more; o Bootstrapping (2nd step): ▪ All of the pseudoreplicates are subject to phylogenetic analysis, yielding the best tree for each pseudoreplicate; o Bootstrapping (3rd step): ▪ Combining the branching information of all trees in one tree (consensus tree); o Bootstrap proportion: ▪ Frequency of a specific branch (monophylum) occurring in the set of trees generated from the pseudoreplicates. Number of the branches of the consensus tree; o Consensus trees: ▪ Combine information out of two or more different trees. Kinds of consensus trees: Strict consensus trees; Majority-rule consensus tree. Strict consensus trees: This tree has only those splits that are found in all of the different trees; All other branches will be shown in polytomies. Majority-rule consensus tree: Such a tree has only split that are found in more than 50% of the different trees → often the frequency of internal branches is given in form of numbers near the branches.