CIP-StudyGuide-2023-Domain-2.pdf
Document Details
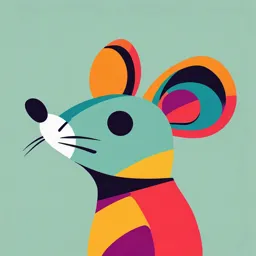
Uploaded by SpellboundTiger
Tags
Full Transcript
DOMAIN 2: The AIIM Official CIP STUDY GUIDE Extracting Intelligence from Information Are you the next Certified Information Professional? This study guide contains the body of knowledge necessary to help prepare you for the Cert...
DOMAIN 2: The AIIM Official CIP STUDY GUIDE Extracting Intelligence from Information Are you the next Certified Information Professional? This study guide contains the body of knowledge necessary to help prepare you for the Certified Information Professional Exam for information professionals to be successful in the Intelligent Information Management era. The AIIM Official CIP STUDY GUIDE Domain 2: Extracting Intelligence from Information Introduction Once content has been created or captured by the organization, we need to extract intelligence from it to provide context to it. We start by looking at metadata – what it is, how to apply it, and how to make it meaningful and accurate. Next, we review taxonomies – how to develop them and how to choose the best one for a particular set of circumstances. We look at new ways to automate how we create metadata and taxonomies, through the use of powerful data recognition and extraction technologies. These in turn can be used to feed analytics and machine learning tools that can add additional insight and help to automate other aspects of information management. Finally, we review approaches to search to ensure users can find the information they need to do their jobs. © AIIM aiim.org/CIP The AIIM Official CIP STUDY GUIDE Domain 2: Information in Context This domain is really the gateway for leveraging and exploiting information in support of the organization’s goals and objectives. Information needs context, and we need to provide that context in a way that doesn’t burden users but instead supports them. This means we need to take full advantage of recognition and analytics technologies to streamline and automate how we develop that context. This domain makes reference to a number of tools, but ultimately the tools have to serve the business needs and outcomes, not drive them. That said, these tools and processes can offer significant benefits in terms of understanding information in new ways and in being able to leverage that intelligence to drive innovation and the customer experience. The Metadata Strategy The Benefits of Metadata Metadata defined. There is no one definition of “metadata” that is internationally and universally agreed – rather, there are many similar definitions or descriptions which mostly cover the same points and you should adopt the one most suitable and relevant to the context of your information management activities and the organization in which you work. The ISO standard 15489, “Records Management,” provides a simple definition of metadata, in its Terms and Definitions section. It defines it as “Data describing context, content and structure of records and their management through time.” The U.S. Department of Defense has a definition of metadata in its DoD 5015.2 standard, which is also similar to the ISO standards, namely “Data describing stored data: that is, data describing the structure, data elements, interrelationships, and other characteristics of electronic records.” This illustrates several of the other purposes served by metadata in ERM systems. Finally, NISO, the U.S. National Information Standards Organization, defines metadata as “Structured information that describes, explains, locates, or otherwise makes it easier to retrieve, use, or manage an information resource.” Metadata is often called data about data or information about information. Perspectives on Metadata The act of entering metadata values is often called “Indexing”, especially when a basic set of values about an item are being captured. It’s also a form of shorthand: “Have you indexed those documents yet?” is easier to say than “Have you entered the metadata for those documents yet?” ISO 23081 is careful to explain that metadata can support different needs, because different views and perspectives on metadata are possible and may coexist. These include: n The business perspective, where at least some of the metadata supports business processes. n The user view, particularly when seeking information, where metadata enable the retrieval and support understanding and interpretation of content. n And the information governance perspective, which includes things like security, privacy considerations, and lifecycle management metadata. © AIIM aiim.org/CIP The AIIM Official CIP STUDY GUIDE Domain 2: Business Value of Metadata The primary value of metadata comes with how it is aligned to and supports specific business goals and objectives. Metadata is a key to organizing content: the term for this is “classification”. Metadata can be used to track things like the dates associated with a document’s associated record schedule. Or, metadata can be used to flag a security setting, validating access and edit rights, and thus controlling distribution. Metadata can also be used to capture users’ rating of content; for example, indicating that content is “valuable” or “useless” or even “dated”. Metadata is an important part of the content capture, creation, and organization phases of the content lifecycle. If associated metadata is not captured while the content is, you will quickly create a collection of content that is difficult to manage, find and retrieve. Metadata is extremely valuable as a search and retrieval enhancing mechanism. Metadata also potentially provides much greater precision to an otherwise free-text query by allowing the user to target a query on a certain field, such as author, subject, date, etc. documents they create or use. In short, metadata is one of the foundations for managing information efficiently and effectively. Metadata can also provide value in the way content interfaces to business processes. For example, if loan applications have to be reviewed in the order that they were submitted, if the date of receipt was not captured as a metadata value, there would be no way to ensure the applications documents were addressed in the right order. If documents need to be processed collectively, metadata provides the foundation for batch processing. For example, if resumes for all employees working on a new project are to be updated to include commentary on the project, tracking an employee’s current project would allow all relevant resumes to be retrieved for a batch update. This also leads to enriched connections between people and content, helping build expert locator bodies of information. Metadata also serves as a point of integration. Different content in different applications, even across different information management systems, becomes “linkable” through common metadata properties and values. A customer or project ID can be consistent across document repositories, as well as Enterprise Resource Planning (ERP) or financial applications. When large volumes of content need to be analyzed, metadata also provides input for business intelligence and analytic tools. For example: the ability to report on the number of invoices coming into the payables department, or the ability to dynamically determine the number of positive customer support calls received in a given time period, are valuable business intelligence insights, but are only possible if those characteristics and values are tracked in metadata. In these two examples, customer IDs and a document type of invoice, or the flags set on a closed customer support call are useful metadata for reporting activities. Finally, metadata can be used to enrich knowledge management based on user profiles. When user profiles are associated with the people adding, using or retrieving content from the information management system, then the metadata associated with those people can be used to find experts on various topics, captured as metadata associated to their profiles and the documents they create or use. © AIIM aiim.org/CIP The AIIM Official CIP STUDY GUIDE Domain 2: The Metadata Strategy Many organizations use metadata. Every system uses metadata to store and retrieve data. But in too many organizations, every system uses similar but different metadata, with the result that different data structures and approaches make information harder to find and manage, not easier. Take a simple example of an employee name. In one system, it’s “first name last name”. In another, it’s “last name (comma) first name”. And in still another, it’s two fields: “First name” and “Last name”. To avoid this, organizations should develop a metadata strategy. The goal of this strategy is to identify and define how metadata will be defined, captured, and managed across systems and processes, such that for any given concept, the metadata used to describe it is used consistently throughout the organization. This will improve findability and the ability to manage content over time; it also helps with emergent processes for discovery and automated management of information using analytics. Guidelines to Determine Metadata Ideally, the metadata design for an organization is based on a structured methodology and approach. However, to get a better idea of how to identify metadata, here are a few high-level guidelines. Based on the content types already identified, determine what is, or should be, available to users to help them find that content. In other words, what do people know at each step of the work process when they retrieve documents? Retrieval of the same content type may be different at different points. For example, you may retrieve a client document based on a client identification number early on, but prefer to use the client’s name when you become more familiar with the client. Don’t just recreate the current way information is retrieved. Create an “ideal” scenario. You may know how to retrieve information based on how the current folder system is set up, but that does not mean that is always the best way. In general, when you create content, you will think about what you created rather than how someone else will want to retrieve it in the future. It’s important to keep both needs in mind in order to avoid costly rework later. Tasks to Determine Metadata Identify documents and metadata that are shared among workgroups or departments. Make lists and content types consistent as much as possible. A record used in payroll – for example an expense report – may be shared with human resources or accounting. But each department may have unique needs or ways to retrieve that record. It can be tempting to make sure all possible scenarios and exceptions are covered when designing metadata. Some exceptions can occur so infrequently that spending time entering properties for the column may not add value. Pay attention to the time it will take you to enter metadata values. If there are too many properties to fill in, your users may try to circumvent the data entry step or store the documents elsewhere. If you don’t want to fill in the data, no one else will either, so consider prioritizing and reducing what metadata is required to what is essential. Analyze existing filing structures – paper documents in file cabinets, existing databases, etc. – since these likely have worked for you in the past. Identify metadata types and formats, such as date, number or text, and align with an enterprise data dictionary. An enterprise data dictionary is a definition of data, formats and data relationships within the organization. Include metadata requirements that may result from system needs as well human needs, such as workflow or integration with other line of business applications. © AIIM aiim.org/CIP The AIIM Official CIP STUDY GUIDE Domain 2: Mandatory vs. Optional Metadata There is a trade-off between having too many mandatory elements (users may find entering their values a chore), and too few (users may enter almost nothing about an item, making its future use and management difficult). Actually, some entries may be conditional, depending on the type of document or record of interest. Thus a “mandatory if applicable” entry is also a valid option described in some standards. This would apply, for example, to a letter or similar item of correspondence, where an entry for the “Addressee” element would be considered mandatory, but not relevant for a project schedule. Similarly, the Location element may be considered mandatory when used for physical objects which can be loaned out or otherwise moved but might be irrelevant for digital records stored in a recordkeeping system. Note that records management staff often want as much mandatory metadata as possible, to achieve a metadata-rich repository. This is often at odds with the user community, who are often not prepared to spend the time and effort entering it! A sensible compromise is necessary, as well as the use of automation techniques, as will be explained later in this module. The Metadata Model There are many ways to develop a metadata model, but they should all include certain elements. n Field name. This should be specific enough that it is unique and understandable. Date is almost always a bad field name – date of what? Rather, Date Paid, Date Published, etc. are clearer. n Data type. A field that is typed as date, numeric, currency, etc. can then be searched for a range, e.g., “all invoices between Jan 1 and Feb 28” or “All expense reports over $1,000”. n Mandatory/optional. Many fields are less useful if they are not mandatory, but if this results in users having to enter many mandatory fields via manual data entry, there will be issues with uptake and completeness. n Source of the data. Is it manual metadata entry, a system or default field, does it come from an external data source, etc.? n Metadata use. What systems use that metadata field in exactly the way it’s been outlined? n Owner or steward. The owner or steward of that metadata and what it’s assigned to. Given the recent and ongoing changes in many jurisdictions relating to privacy and data protection, it may also make sense to identify where fields contain personal or sensitive data. © AIIM aiim.org/CIP The AIIM Official CIP STUDY GUIDE Domain 2: Metadata Standards As you consider the metadata model, you should be aware that there are a number of metadata standards available to consider. Dublin Core, ISO 11179, ISO 23081, and many others offer various lists and structures of metadata elements. For the purposes of this discussion, we don’t recommend any one particular standard, because in all likelihood none of them are an exact fit for a specific organization’s needs. Standards are important, especially when exchanging information with third parties, but it’s also important for the metadata model to reflect any unique needs or perspectives within the organization. Metadata Automation Because of the volume of information being created or captured in most organizations, manual metadata entry is a difficult sell. Users don’t want to do it, and when they do it, they make mistakes. So, one of the elements to include in the strategy is a bias towards automation. It is not necessary to include the specific approaches for this in the strategy, but it’s important to address that metadata will be automated to the extent possible. We address metadata automation in more detail elsewhere in this section. © AIIM aiim.org/CIP The AIIM Official CIP STUDY GUIDE Domain 2: Capturing and Managing Metadata How to Capture and Apply Metadata Systems and Default Fields In this section we discuss how to actually capture and apply metadata to digital objects. There are several different approaches we can take depending on the business context, the systems being used, the presence of existing metadata, etc. So, let’s start with fields where the metadata is already present. System fields are captured automatically by the system in response to some sort of triggering event. For example, when a document is captured into the repository, a unique ID is automatically generated and populated, as is file name or document title or something similar. System fields are generally not updateable. A default field is similar in that it is filled in, but it *is* updateable if warranted. For example, a user captures a document by scanning it into the system. There is a field for date scanned, which is a default field populated with today’s date. There’s another field for the date the image was checked for quality control and released to the repository. That, too, defaults to today’s date, but if it doesn’t get checked until tomorrow, that field could be updated (manually or automatically) to reflect the correct date. © AIIM aiim.org/CIP The AIIM Official CIP STUDY GUIDE Domain 2: Manual Metadata Entry The first and most obvious method for applying metadata is manual data entry. In this approach, the user is required to enter metadata for the document into prescribed profile fields. Manual metadata entry is expensive for a number of reasons. First, it requires someone to do the data entry, who has to be paid a salary and benefits. If these are expected to be authors or the persons capturing information, that takes time away from doing their regular job. Manual data entry is also time consuming and can be expensive to find and correct errors. And even if users are willing to do it, they may not have the background or training to do it correctly. Training and job aids can help, but they won’t be able to prevent typographical errors, selecting the wrong value from a controlled vocabulary, etc. The bottom line is that users don’t want to do it, and if they do, they aren’t generally very good at it. So one way to minimize these issues is to limit the amount of manual metadata capture that is required of end users. We talk about how to improve the quality of metadata, especially manual metadata entry, in another section. Metadata Extraction Next, we can extract metadata directly from the document. In some cases, the repository or application can read the properties and extract that information. This is useful where organizations and users update the properties for new documents. But it can be misleading if they don’t, because many users will use and reuse the same document or PowerPoint template, with the result that the title, subject, and author are the same for thousands of documents. We can also use recognition technologies. The most common of these is optical character recognition and is used almost exclusively for images of scanned documents. In this approach the application renders the characters from the image and attempts to detect patterns of characters within the images. This can be upwards of 99% accurate but depends heavily on the nature of the documents, the quality of the images, and the abilities of both the software and the user. Another type of recognition technology is barcoding. Barcodes are available in 1-d and 2-d flavors and are highly accurate but only hold a limited amount of information, particularly for 1-d barcodes. They are gaining traction in many electronic document applications to aid the recognition and extraction process. And there are specialized recognition technologies available for recognizing and extracting data from audio, video, and other rich media types. These tend to be pricey and specific to the application and certain file formats but may be quite useful for organizations that capture a lot of these types of documents. © AIIM aiim.org/CIP The AIIM Official CIP STUDY GUIDE Domain 2: Inherited Metadata The principle of “inheritance” is a useful way of adding metadata to information objects. When an item is captured into an information management system it will be placed into the appropriate file (or sub-file or volume) within the classification scheme, very probably joining other documents from the same business context and thus maintaining a useful collection of related and relevant documents for a given business area and its business activities. The item will have had values allocated to at least its mandatory metadata elements, and several optional elements may also have entries, depending on the history and use of the item before its declaration as a record. The act of declaration will add additional metadata, for example - who made the declaration and when. However, more metadata can now be applied to the item, based on metadata already present for classes and files in the classification scheme – very commonly this metadata provides particular security settings, and/or retention periods and disposal instructions for items stored in the many files. This metadata is said to be ‘inherited’ by the record, as a consequence of its declaration into a selected file (or volume) in the classification scheme. Metadata from User Logins Software can also be used to look up or capture the user’s details. Many applications can use the Lightweight Directory Access Protocol, or LDAP, or other identity or directory-type applications to capture certain details, such as the current user’s name, job title, department, etc. This could be done in the office productivity application, such that all documents created by the user have that information entered automatically, or in the information management application. Metadata from Other Data Sources Metadata can also be read or copied from existing data sources through a number of mechanisms. While the details vary, the idea is that the data is already stored in a database somewhere, probably de-duplicated and normalized, and that the organization would do well to reuse that data and the effort required to create it rather than entering data into many different applications. This process could be manual or automated; the more automatable, of course, the greater the benefits. Metadata through Workflow If the organization uses workflows to streamline and automate document-centric business processes, those same workflows can often be used to update metadata associated with a particular document. For example, a field called “Status” might have values of “In Draft”, “In Review”, “Approved”, or “Revision Required”. As the publication review and approval workflow progresses, the workflow can update the field to reflect its current status. Similarly, an order processing workflow could use a similar field to display whether the order is “Received”, “Processed”, “Shipped”, or “Delivered”. © AIIM aiim.org/CIP The AIIM Official CIP STUDY GUIDE Domain 2: Metadata through Analytics Analytics can also be used to extract and populate metadata – in this case directly from within the content of a document or record. Here’s an example using an article from the New York Times. You can see that a number of different types of information have been extracted: key sentences; people, organizations, and places; and other key entities. Any of these entities could be used to populate a metadata field. Analytics can also be used to “remediate”, or fill in, missing metadata, for example as part of a system migration. This could be based on the contents of the documents in the old system, inference and mapping from the old system’s metadata to the new one’s; or converting deep hierarchical folder structures into metadata fields and values, among others. © AIIM aiim.org/CIP The AIIM Official CIP STUDY GUIDE Domain 2: The Challenges of Sharing Metadata Across Systems This module will help you to identify the challenges of sharing/propagating metadata across tools and systems. Sharing is Hard! Sharing metadata across the myriad information silos found in the typical organization is extremely important. Effective search and retrieval is based to a significant extent upon complete and correct metadata. Yet in most organizations, finding, retrieving, and using information across systems is all but impossible, due to issues in how each system – or silo – is set up. In most organizations, for any set of systems, these metadata-impacting issues are generally present: n Different terms and values are present in each system. In one, we use the term “Customer name”; in another, “Customer”; and in a third, “Owner”. Similarly, in one system we use a “first name last name” structure, and in another we use “last name (comma) first name”. If controlled vocabularies are in use, it is not uncommon for different systems to have different values listed for the same field. n Different structures. Continuing the example above, in a third system we use *two* fields: “first name” and “last name”. Maybe there’s a “middle name” or “middle initial” field as well. At least the different field names can be mapped; making this system interoperable with the first two requires a more complex solution. n Different security/access controls. Access controls are metadata in a very real sense, and if you can’t access the document, you likely can’t access its metadata either. Again, resolving this becomes a significantly complex exercise. At least as important as the technical constraints are strategic issues. Sharing can be difficult or impossible because of specific legal or regulatory requirements. We’ve discussed privacy and data protection requirements in a number of sections of this course because it touches so many things, and metadata is no exception. Similarly, there may be other organizational constraints such as the need to maintain ethical walls between different parts of the organization due to conflict of interest rules. If you can’t legitimately access a document, you probably shouldn’t be accessing the metadata either. © AIIM aiim.org/CIP The AIIM Official CIP STUDY GUIDE Domain 2: Getting to Enterprise Metadata Interoperability This is a key illustration, pointing out the path to metadata interoperability. At each stage, the content gets more and more interoperable. At the top, we have the typical enterprise with multiple content silos: information management systems, shared drives, line of business systems, etc. We see three concepts: a date of publication, an author, and a subject/topic. Each of these has a different term and, as shown in the third line, a different structure (last name, first initial). At the second level, the organization leverages the Dublin Core metadata standard to ensure consistency and structural interoperability between the silos. All three systems now use the same field names: data, creator, and subject. However, there is still the inconsistency of the semantic values as shown in the third line: while the field names are the same, there are three different date structures; two different creator structures and inconsistent values in those that are similar; and three different values in the subject field for the same thing. The ideal is then at the bottom, where both the fields and their values have been semantically merged to create consistency of both the names of the fields and their contents. There are a number of technical approaches to achieving this; the details are beyond the scope of this course but would likely require a discussion with a number of parties including IT, information architecture, the various information management disciplines, and of course end users. © AIIM aiim.org/CIP The AIIM Official CIP STUDY GUIDE Domain 2: Information Interchange It’s not uncommon for organizations of any size or complexity to have multiple metadata models because of legacy systems, approaches, acquisitions, etc. So how do we deal with this today? The fact is that many standards originated as a means to share or exchange information among enterprises or groups with common interests. So, some large industries, for example, such as the auto and aerospace industries, have set up common content structures to support standardization. So companies that are within these particular areas are able to more easily exchange the information that they have because they follow the same format, the same standard. More industries are following auto and aerospace. Financial services and healthcare are also coming out with standards very similar to these other ones. For a manufacturer, content standards become a mandatory format for anyone delivering to them. They provide consistency and predictability, and adopters can predict the structure of the information that will be provided to them. You can thus define content repositories around those particular standards. The predictability of structure and metadata opens up new opportunities for the automatic processing of content. The more these standards are followed, the more easily it’s going to be for us to do things automatically based on how that content is structured or categorized. How to Improve Metadata Quality Improving Manual Metadata Entry There are a couple of ways to capture metadata – manual and automated. Many organizations still rely on manual metadata entry – by scanner operators, by end users, etc. – in order to capture metadata. As we’ve previously discussed, this is not ideal for several reasons. Nevertheless, if this is the chosen approach, there are a couple of additional steps that can be taken to help improve metadata quality. The first one is to make them mandatory. As noted earlier, mandatory fields can be problematic – but optional fields often don’t get filled in at all. Next, we can create “drop down lists” – aka controlled vocabularies. These are simply lists of terms from which users pick the appropriate entry. For example, most online ordering forms will show countries in such a list, and if you select certain countries, it will show states, provinces, or prefectures in another list. These work best with limited lists of closely related things, such as geographic locations, the departments in your organization, or project names. The benefit of this approach is that users are required to select from the list – no options, no misspellings or variations, etc. We can also validate the data as it’s being entered and captured. Data masking applies a pattern to a field such that any data entered has to match that pattern. This is often used with identification numbers, invoice numbers, dates, credit card numbers, or anything that always used the exact same pattern. We can also significantly improve the quality of metadata through automation. Any time we can automate a task or process, that task is going to be completed more consistently and more efficiently. We address metadata automation in more detail elsewhere in this section. © AIIM aiim.org/CIP The AIIM Official CIP STUDY GUIDE Domain 2: Classification Schemes Findability Introduction to Findability Search and retrieval are key activities for users, and one of the primary means by which they will interact with digital information. Users will have many different ways to search for information within a particular repository. They will be able to search by: n Metadata associated with a record, folder, etc. n Content (full text) – for example text string. Browsing the folders in the classification structure or the nodes in the navigation scheme. We refer to the grouping of files as categorization or classification; while there are nuances, we treat them as interchangeable in this section. These are also referred to as taxonomies; we discuss taxonomies in more depth elsewhere in this section. © AIIM aiim.org/CIP The AIIM Official CIP STUDY GUIDE Domain 2: We can call these the “Three Pillars of Findability.” We address each of these in more detail elsewhere in this section, but let’s review each one briefly. Search vs. Classification There are two main approaches for effective access to stored information – classification or via a search engine. Classification organizes information by storing it, logically-speaking, in collections, folders, or ‘buckets.’ This first categorization approach can be summed up as ‘aggregate and organize.’ By contrast, the second approach uses the power of searching or search engines to find information, and does not recognize or have any need for aggregation. Note that this can apply to metadata or full-text searching. This second approach can be termed ‘Find by raw power.’ This approach also depends on the user knowing what to search for in order to retrieve the desired records; for example, a search for “tank” will retrieve records relating to water storage devices as well as mechanized military vehicles – but would miss records relating to armored personnel carriers (which are not generally referred to as tanks). In a sense, there is a ‘tug of war’ between these approaches. Each approach has its limitations and strengths. © AIIM aiim.org/CIP The AIIM Official CIP STUDY GUIDE Domain 2: Metadata Let’s start by looking at the pros and cons of searching using metadata. Pros: n Metadata search is very flexible. A good searcher can construct very sophisticated searches within or across multiple fields. n Metadata searches are much easier to perform ranged searches on. For example, it’s fairly straightforward to search for all invoices over a particular amount, all emails sent within a certain time period, etc. Cons: n Flexible can mean complex – especially if users aren’t very well trained and familiar with the interface. n Numeric searches require that the applicable fields use the applicable data type. This is often the default, but if a field is a text type as opposed to date, numeric, currency, etc. the searches will not provide the expected results. n Any metadata search requires that the metadata be present – and correct, and correctly spelled, etc. in order to find it. Similarly, no matter how good the metadata is, it may not help a user who has made a typographical error. n Finally, metadata requires a certain consistency in terms. If users can type anything they want into a field… they will. It’s not just spelling, but also when a user enters a completely alternate term that a human can recognize as the same or similar, but a computer cannot. A thesaurus can be valuable in this context, but the preference should be to standardize on specific terms for specific concepts as much as possible. Full-Text Search Here’s a look at the pros and cons of using full-text search. Pros: n You can find any word or phrase in a document, group of documents, throughout a repository, or even across repositories and systems. Cons – and there are a lot of cons to that one pro! n The index being searched is what it is – complete with misspellings, alternate spellings, alternate terms for the same concept, etc. n It’s very easy for users to search incorrectly. First, most users aren’t trained to search anymore – they use a search engine and call it a day. That works when you’re looking for a new recipe, but not so much when you need to find all documents relating to a particular person or function. And even if they can search correctly, users make mistakes and misspellings of their own in the search interface. n Perhaps most importantly, you can only use full-text search on things that have full text to be searched. That means office productivity documents; most (but not necessarily all) PDFs; web pages; and a few other types of information. It generally does not extend to images, audio, or video, though this is starting to change. But you generally can’t search what isn’t there. It also means that the text has to be indexed – this is generally automatic but may not be instant. © AIIM aiim.org/CIP The AIIM Official CIP STUDY GUIDE Domain 2: Classification Finally, let’s look at the pros and cons of using a classification scheme. Pros: n Classification schemes can organize information for ready access. n This allows users to understand what is included – and often what is excluded – from a particular process or domain area. Classification schemes also provide the preferred, or only, terms for a given concept. n Classification schemes often map to how users do their work. n A logical classification scheme will allow users to browse it to find what they are looking for – much like a bookstore. Cons: n Classification schemes are often not as logical or complete as they could be. Organizations and processes change, and it’s hard to keep up at times. n The more complete the classification scheme is, the more complex it is likely to be. n Classification schemes often map to users’ bad habits. It’s not uncommon to see different levels of detail at the same level of a classification scheme, or the seemingly ubiquitous “Other” or “Miscellaneous” category. n If the classification scheme isn’t logical *in the eyes of the users using it*, they will get lost in it and become frustrated. n Ultimately, if the classification scheme is too complex, too unwieldy, too incomplete, or too difficult to understand, users will not use it consistently. The Thesaurus A thesaurus can provide another way to support findability for all three pillars. A thesaurus is a list of terms and their relationships. These relationships can be more complex than a simple parent-child hierarchy. The value of a thesaurus in the information management context depends on how it is used (and how complete and up-to-date it is). For metadata and full-text searching, thesauri can allow users to locate a particular concept using their preferred terms, rather than just the “official” one. For example, a metadata field for a location might have the value of “San Francisco”, “SF”, or “San Fran” on different documents. With a thesaurus and some additional setup, a user could search for any one of those three terms and find all the documents that contain any of them. The Three Pillars of Findability To conclude, then, each approach has its pros and cons – in isolation. What should be apparent is that the best results will come from leveraging all three approaches. If information is classified logically and consistently, with correct and consistent metadata, and a full-text indexing is possible, it should be much easier to find information and ensure that it is the *correct* information. © AIIM aiim.org/CIP The AIIM Official CIP STUDY GUIDE Domain 2: Classification Approaches Classification Scheme – Definition Put simply, a classification scheme is any structure an organization uses for organizing, accessing, retrieving, storing and managing its information. ISO 11179 defines classification schemes as including keywords, thesauri, taxonomies, and ontologies; for our purposes we can add file plan and records retention schedule to that list as well. Most organizations have MANY classification schemes being used at any given time; which one to use depends on who is using it and what the purpose is. Another common and related term is business classification scheme, or BCS. A BCS is nothing more than a classification scheme which is based on an organization’s business functions and activities. Finally, many classification schemes are also referred to as taxonomies or categorization schemes. Effective Classification Schemes The key issue for any classification scheme is its ease-of-use and performance for the users. If users are not happy, (and they won’t be happy if there is poor ease-of-use and / or poor performance) then the whole environment will not be accepted, and the initiative will be deemed to have failed. The way in which the BCS is designed and deployed will be a major factor in the ease-of-use and performance for the users – who, it’s important to remember, may have minimal training in information management best practices. This means that the focus needs to be on ensuring that the classification scheme is: n Easy to use. It reflects the way users work, and, as much as possible, the terms they use. n Concise. Terms or folders that aren’t used shouldn’t be added “just in case” – this will make it more confusing for users. n Predictable. If the classification scheme is logical, users will be able to predict where to file and find information. If it isn’t, users will not be able to find their information and will get frustrated. Finally, every classification scheme will have to change over time as the business and its operations change. So there needs to be a process in place to make required updates when necessary. You should note that the usability of any classification is affected by: n The number of levels in the classification scheme. In general, more than four or five levels can be confusing, but ultimately the number of levels required may depend on the nature, size and complexity of the organization; and the degree of business need for speed and accuracy in control and retrieval of information. n The user interface for the system in which the classification scheme is implemented. There are significant differences in the way that the current products in the marketplace address the requirements for this. n The availability and quality of other retrieval tools. As discussed earlier, while the classification scheme is important, effective metadata and access to indexed full text can significantly improve the findability of business information. © AIIM aiim.org/CIP The AIIM Official CIP STUDY GUIDE Domain 2: Which Classification Approaches? There are two primary considerations when determining which classification approaches to take. The first is the principles of classification – where the primary options are functional; subject/topical; and organizational. n Organizational classification schemes mirror the organizational chart. These are simple to put together but can be difficult to maintain as the organization changes or reorganizes. n Subject/topical classification schemes are often seen in law firms, where all the different practices have similar in concept, but very different in practice, information management needs. Subject or matter-based schemes are often relatively static because the topics or matters don’t change structurally very rapidly. n Functional classification schemes are based on the business functions and activities of the organization. These are easier to maintain than organizational over time; if the organization changes, in all likelihood it will still have an accounts payable function, a recordkeeping function, etc. Generally, a functional principle of classification is preferred. From here on, therefore, we will consider only a functional principle of classification, that is BCSs. The second is deployment, where the key points are hierarchical or tree-style and keyword or thesaurus-based. n Hierarchical or tree-style deployments are very familiar to end users – folders, sub-folders, sub-sub-folders, and the like. This approach assumes that a particular document or record should be filed in one folder, which is not always the case. They are therefore not as powerful and flexible as thesaurus-based approaches, but they are generally more usable for end users. n Thesaurus or keyword-based deployments are much more flexible compared to hierarchical approaches because things can be filed according to numerous relationships using virtual folders, views, or other presentation formats. But they are significantly more difficult for end users to make sense of. © AIIM aiim.org/CIP The AIIM Official CIP STUDY GUIDE Domain 2: Hierarchical Classification Scheme As you can see in this example of a hierarchical tree-structure scheme, we start with a… Level 1 - ‘super function’ or very large area of management responsibility – in this example that’s Financial Services. This is broken down into three smaller functions: n Administration, Accounts Receivable, and Accounts Payable. n Each of these functions is then broken down, in turn, into smaller areas of management responsibility, known as sub functions. You can see that Accounts Payable is shown broken down into the following sub-functions: n Expenses and Invoices. And you can see that each of these sub-functions is made up of activities which are performed within that area of management responsibility – that is within that sub-function. For example, you’ll see that within the Expenses sub-function there are Training Expenses and Travel Expenses activities. So, from the organization’s overall Level 0 ‘mission,’ the hierarchy first decomposes into: super functions (Level 1), then into functions (Level 2) and then into sub-functions (Level 3). Thus, it moves from broad functional areas to narrow functional areas. It then decomposes the smallest sub-function into ‘types’ of activity that make up that sub-function. The level of decomposition needed and appropriate for a particular organization in its classification scheme will depend on the number and complexity of functions it has. © AIIM aiim.org/CIP The AIIM Official CIP STUDY GUIDE Domain 2: Hierarchical Classification Scheme And here’s a graphical representation of what this portion of the classification scheme might look like. Build vs. Buy Finally, one more thought about whether to buy or build a taxonomy or other classification scheme. In practice, both have their pros and cons, and it should be considered as more of a spectrum between the two. Here are four spots on that spectrum to consider. n Buy. You buy a taxonomy or classification scheme and implement it as-is. This approach is much faster to deploy than any of the others, and many industries have these available already today. This approach will help to ensure that you’ll have better interoperability with any third parties, but it may not exactly fit your organization’s ways of working and business requirements. n Modify. In this approach you buy the classification scheme, but then customize it to meet your unique business requirements by adding new elements or removing extraneous ones. n Model. In this approach you look at others’ work – other publicly available schemes, how others in your industry do it (if possible), etc. The question here is really how close those other organizations are to what yours does. n Build. In this approach you develop the entire thing from scratch, using internal and/or external resources. It’s completely custom, so it perfectly meets your needs, but it’s a very labor-intensive and demanding process. © AIIM aiim.org/CIP The AIIM Official CIP STUDY GUIDE Domain 2: Stakeholders for a Classification Scheme This module will help you to Identify the stakeholders for a formal classification scheme. Stakeholders The first step in most implementations is to identify the key stakeholders. For a classification scheme, these would include: n The program owner, in whose area of responsibility the classification scheme will be deployed and who is sufficiently senior in the organization to be able to champion the initiative. n Business unit managers from those areas that will be using the classification scheme. It is their users who will be using it and, in the pilot phase, piloting the scheme so it is in their interest to ensure it meets their needs. Sometimes classifications schemes are only used by one process or function, but many times they are used by multiple groups, each of whose needs need to be taken into account. n Records management. So many of these classification schemes relate to records management that their participation is a must; moreover, in many organizations that is where the expertise and experience in classification scheme development resides. n IT. For any information management program, there will ultimately be a requirement to load or otherwise implement the classification scheme in one or more applications. There will also be business logic to assign, and in most organizations one or both of these come under the purview of IT. The Development Team Experience has shown that the best approach to developing a classification scheme is to include a mixed core team comprising: n In-house staff with experience in the development of classification schemes. As noted, this is often the records management function, but it could be anyone in the organization with the understanding and experience to ensure the classification scheme is complete, correct, at the appropriate level of detail, etc. n People from outside the organization, with significant experience in the development of classification schemes – i.e., consultants. Note that this is not mandatory, particularly if the organization has staff with the necessary expertise and experience. But it is often the case that the organization believes it has more expertise than it actually does, and external resources can both speed the development process and potentially improve its effectiveness. n And of course, users in the relevant areas must be involved. A taxonomy has to be usable by its target audience. Most classification schemes fail – that is, they are less likely to be used consistently – because they are too complex and difficult to use. This is often because they are developed by people with limited understanding of how to create them, or by people with limited understanding of the domain in question. A well-designed taxonomy, one that is usable, needs input from subject matter experts within the target function as well as those with background in information architecture or library science. © AIIM aiim.org/CIP The AIIM Official CIP STUDY GUIDE Domain 2: Extracting Intelligence from Information Extracting Information from Structured Applications Introduction to Structured Data The intent of this discussion is not to make you a database administrator. However, it is important to understand how structured data and databases work in order to manage that data effectively in the context of an information management program. As the name suggests, structured data is data with a structure. That is, the format is well-defined and consists of a number of fields, or rows, and the values within those fields, or columns. The spreadsheet is a very simple example of this. Each complete row, combined with its values, form a single record (which may or may not be the same as a record in the context of formal records management practices). There are many ways in which structured data can be stored, including relational databases, XML databases, and spreadsheets, but they all have a similar internal structure. © AIIM aiim.org/CIP The AIIM Official CIP STUDY GUIDE Domain 2: Structured Data Example Where structured data gets interesting is that in many relational databases, there are multiple tables that relate to each other according to certain fields. This makes it easier to abstract and manage data. For example, let’s say you offer training courses and you want to create a database to store the information about your courses. While you could enter everything into a spreadsheet, if your courses are successful the spreadsheet will quickly become unwieldy. Instead, you could have a database that has one table that lists information about your courses: subject, whether it’s online or in a classroom, the duration of the course, and the cost. You might have another table for student registrations: name, course or courses registered for, whether they’ve completed the course or not. Now, you can run all kinds of queries and reports: how much did a course make, how many students took the course, which courses did a particular student take and did the student complete them, what’s the completion percentage by course or by delivery mode, and many more. The challenge here is that if you determine that the retention for your course details is 2 years from the date of the last course offering, and your customer details are retained for 5 years from the date of last purchase, you’ll lose the entire first table 2 years after the last course is offered. For the next 3 years, that Customer Details table will be missing the data on the courses the student took because the Course ID field from the Course Details table, and all of its data, is gone. This is one of the things that makes structured data so tricky to manage over time. Data vs. Presentment A common issue with structured data is that the data in databases is not really meant to be human- readable, and certainly not by the end user or customer. Take, for example, credit card statements. All of that data is in the database, and when credit card statements are generated to send or present to the customer, formatting is added so that it looks like a statement. If the statement is late, formatting might be added to turn the amount due figures red and bold; if the statement shows zero balance due, formatting might be added to include a special offer. These in turn are done using business rules and logic. But this raises a fundamental question: what’s the record? In other words, is the record just the data, or is it the data combined with how it is presented? If the latter, how can the organization ensure that it can regenerate that presentment a year or five years later if needed, given both the involvement of business logic and the likelihood that the overlay and the presentment rules will change over time? Or do you even need to? Extract Using Native Tools In terms of technology approaches to extracting and capturing structured data, the first thing we should note is that these are all things that would be done by database administrators or someone else in IT. The first approach is to archive structured data using native tools. Most structured database applications and relational database systems have their own built-in mechanisms for extracting data for reporting, migration, etc. The benefit of this approach is that, since the tools are built for that particular application or platform, the data extraction is much more likely to maintain referential integrity between all the moving parts within the system. In other words, no data is being inadvertently lost or orphaned. The challenge is that in most cases these capabilities do not extend to other applications, meaning that the organization would have to extract data from each structured application individually. This was part of the intent of the creation of the Structured Query Language (SQL), but in practice each database management system uses its own flavor of SQL. © AIIM aiim.org/CIP The AIIM Official CIP STUDY GUIDE Domain 2: Extract Using Third-party Tools Another alternative is to extract structured data using third-party tools. Some of these are stand- alone solutions, while others leverage what the main structured data application vendors know about accessing and extracting structured data to make those capabilities available to others. The obvious benefit is the potential for the tool to be able to extract data from different applications within the enterprise. The challenge is that they tend to work well with the most well-known applications, meaning that if yours is an unusual or highly customized application, they may not work as well… or at all. Output Capture The last approach we offer here is to capture structured data in an output format. This approach converts the relational data within the application into a flat data stream, such as XML, or a series of data objects, such as PDFs. This is often how individual statements are generated to send to customers or to post for electronic bill presentment and payment. The benefit of this approach is that the resulting data streams or files are complete and maintain any formatting required at the time the report is run, so they are complete and readable from the customer’s perspective. And if the organization is already doing this, there is no real extra cost in terms of data extraction. But there are some significant disadvantages. First, this is analogous to printing digital records – all of the underlying functionality is stripped from the resulting data objects. If this is for legacy data, this might not be a bad thing, but organizations should be aware. It does create some additional costs and resource requirements in terms of storing and managing the resulting streams or objects. Perhaps most importantly, this approach doesn’t necessarily address underlying performance and compliance issues, UNLESS the data that was output for capture is subsequently archived or deleted. In other words, in many organizations, this creates yet another copy, rather than serving as the copy of record. © AIIM aiim.org/CIP The AIIM Official CIP STUDY GUIDE Domain 2: Extracting Intelligence from Scanned Images Recognition Technologies Scanned images can be analyzed to recognize and extract text or other intelligent content, including company logos. There are a number of ways of doing this; let’s start reviewing some of the different approaches to character recognition. n Optical Character Recognition, or OCR, is commonly used with printed office- type documents. Here, the scanning capabilities are capable of discerning different fonts, including bold and italic, and line and text spacing. Text can typically be extracted and copied into a metadata field or placed in a separate, but associated, text file. This data can then be used to search for a specific image or even “within” an image or PDF. n Intelligent Character Recognition, or ICR, is a sophisticated technique that enables the reading of handwritten characters and may use the adjacent characters or context to improve the recognition rate. n Optical Mark Recognition, or OMR, is a technique for high volume data capture typically from a marked grid on an accurately printed OMR sheet of paper. The OMR input generally translates into “yes or no” or “true/false” responses. OMR is often used on forms; another common application of OMR is in the high volume marking of school examination papers. n Handwritten Character Recognition, or HCR, is similar to Intelligent Character Recognition. HCR scanning is used to interpret poorly defined handwriting as strings of text. Another use of scanning is the detection and interpretation of barcodes, to speed up data input. A form may have a barcode, or the barcode may be on an archive folder, CD case or drawing. Use of barcodes with physical assets can be very effective in ensuring that objects and metadata are matched up for search and retrieval later. Barcodes are available in a variety of formats; some 2D barcodes can store hundreds to thousands of characters of data. © AIIM aiim.org/CIP The AIIM Official CIP STUDY GUIDE Domain 2: Forms Recognition Advanced OCR tools can be set up to recognize zones in order to automate forms processing activities. For example, the top right corner of a form may be a consistently structured customer address block. The zone OCR settings can zoom in on those predictable sections, identifying and capturing the information and automating the contract or form handling. The text recognition can be used not only to later full text search on the imaged documents, but text can also be extracted to pre-populate database, workflow or other structured fields. Intelligent Character Recognition is designed to read and extract text from handwritten documents. This is best suited for constrained handprints – that is, where a field is structured for the user to enter a single letter in each box. In short, forms processing combines many of the different recognition technologies to interpret and extract the information from the scanned form. Today, many scanning software solutions are able to recognize different forms according to their unique characteristics and apply recognition technologies in a more flexible way. In some cases, the solution may even be able to differentiate between different classes of documents and treat them appropriately, reducing or eliminating the need for batch separation. Quality Control It’s important to note that while recognition technologies are robust and mature, they are not foolproof. The accuracy of the recognition will depend heavily on the quality of the scanned image, which in turn depends on the quality of the original document. Many capture applications will include some capabilities for “cleaning up” a scanned image – deskewing, despeckling, removing lines or holes, etc. to make the image as good as possible prior to performing the recognition process. Because of these potential issues, it’s important to perform quality control on the extracted data. When first setting up a capture process, quality control should be performed against 100% of the scanned images and their relevant extracted data. As confidence grows in the process, this could be scaled down somewhat. One of the other ways to improve quality control is to apply data masking and data validation. For example, if the recognition process is being used to recognize a currency amount, the field could be set up to require only numeric values and only in a pattern that matches the currency and numbering pattern used to express currency in that environment. © AIIM aiim.org/CIP The AIIM Official CIP STUDY GUIDE Domain 2: Automating Information Extraction Throughout this section we’ve talked about different approaches for extracting intelligence from information. But it’s important to understand the *why* as well as the what and how. There are several key benefits from automating information extraction: n Accuracy. Humans make errors, and humans are very inconsistent in how they make them. Sometimes it’s not paying attention and entering the wrong amount or transposing digits. Sometimes it’s a typographical error. Sometimes it’s selecting the wrong value from a dropdown list or the wrong date from a calendar picker. Regardless, automation can significantly reduce the number of errors. n Completeness. Humans also aren’t always the best and being complete – that is, ensuring that all fields are filled in (correctly). With mandatory metadata fields we can make staff enter data, but it’s often not good data. Meanwhile, optional fields are not filled in at all, which drives towards making more and more fields mandatory. Automation helps to ensure that all the information is captured, extracted, completed, and managed. n Consistency. As noted above, humans make errors even when their choices are limited. Without those limits, it’s difficult to know how something will be captured, or filed, or what data will be entered into a particular field. Is it San Francisco, or San Fran, or SF, or some kind of location indicator code like CA- 228a? Automation can significantly improve the consistency of these tasks because they occur precisely according to their rules, workflows, values, etc. Here are some more technical benefits from automating information extraction: n Speed. Automation tools work at the speed of software and can work 24/7 without a break. This generally means faster processing in addition to the other benefits. n Scalability. Similarly, working at the speed of software helps the solution to scale up. Processing hundreds of documents an hour is certainly possible for software; how many staff members would be required to complete the same review? n Automation. All of these benefits fall under the umbrella of automation. Automation ensures that things get done, and that they get done correctly, completely, and consistently. This does mean that the entire process needs to be thought through carefully to ensure what happens at each particular stage in the process. It does require up-front work to develop the business rules and business logic required for effective automation. And it often requires, or at least benefits from, technology solutions – but the planning and design work needs to happen before effective automation is possible. As we’ve explained elsewhere in this course, automating a bad process – in this case, extracting intelligence from information – simply means executing a bad process faster. © AIIM aiim.org/CIP The AIIM Official CIP STUDY GUIDE Domain 2: Analytics and Artificial Intelligence Use Cases for Analytics and Artificial Intelligence Structuring the Unstructured Let’s start by defining what we mean by analytics and artificial intelligence. Making content searchable and meaningful happens when free-form text data is structured – and this is a process. Documents, snippets of text, transcribed conversations, i.e., the text data inputs, are transformed by software – applying natural language processing techniques to transform free-format text within documents into core elements, terms, and characteristics. We can then extract the meaning of these entities and use them to do things like automatically classify and route information. These structured outputs are used to derive new insights – to include in search indexes to make document retrieval more relevant, or to create new variables that can be used in predictive analysis. The structured results can be used to score social media postings with sentiment polarity - positive, negative, neutral, unclassified, or classify content that may already exist, or to assign metadata values that describe the material without anyone reading it. So, it’s often said that the point of text analytics is to structure the unstructured data. Of course, to do this, you need born-digital information with a text layer that can be transformed and analyzed. If there are any physical documents to be included, they need to be digitized and have recognition technologies applied to extract the document content. And it helps to have good quality, consistent metadata fields and values, meaning you may need to map alternate or synonymous terms to the preferred term for a particular concept. © AIIM aiim.org/CIP The AIIM Official CIP STUDY GUIDE Domain 2: Text Mining and Analytics Here’s an example you’ve seen already, in the context of metadata extraction. But you can see other things here including named entity extraction (the people, organizations, places, and other entities); topic recognition; and recognition of key sentences that could be used individually or together to summarize even a long, complex document. Simply finding these terms and topics in free text is referred to as text mining; once we have them, we can do other things like sentiment analysis and other more complex sorts of analysis. © AIIM aiim.org/CIP The AIIM Official CIP STUDY GUIDE Domain 2: Analytics Applications Now let’s look at some more specific use cases. The first one we’ll look at is governance broadly. This is still an emerging area, but already we’re seeing organizations leverage analytics to add or correct metadata, detect exact or near duplicate files and detect sensitive types of content such as the credit card information we discussed earlier. This last one can significantly assist in safeguarding information from being breached or removed from the organization, inadvertently or intentionally. You can see that the top three applications refer to adding or correcting metadata. The top one refers to improving searchability within the current system, and the second adds metadata prior to a migration to improve searchability within the new system being migrated to. In other words, we can use analytics to directly improve the findability of enterprise content. Analytics Applications Do you use automated or batch agents to perform any of the following functions? Add or correct metadata to improve searchability 18% Add or correct metadata prior to migration 13% Add or correct metadata to improve alignment 12% Detect duplicate files (by content) 12% Add or correct metadata and Bag 9% Detect security risks and misslocated access rights 10% Detect sensitive or privacy-related content 10% Encrypt or redact sensitive content 6% Detect offensive content (edit) 5% Detect infringing or offensive images/video 2% © AIIM www.aiim.org Identify Value of Information Here’s an example of how we might use content analytics to determine the value of a particular piece of information. We have a document called “Forecast summary_121008.doc” located on a mapped network shared drive at G:\Sales. The traditional approach to reviewing this document is to have a human open the document, review its contents, and determine its business value moving forward. © AIIM aiim.org/CIP The AIIM Official CIP STUDY GUIDE Domain 2: This is OK – as long as someone actually does it. But you can’t have humans manually review thousands or millions of documents on your shared drives or SharePoint or wherever your digital landfills are. In addition, different people may classify the same or similar documents differently. And the same person may classify the same document differently over time; to see this in practice, check the email categorization structure you have set up in your own email inbox. Enter content analytics. In this approach, we would “train” the technology solution to make a better, more consistent distinction between information that should be saved and managed, and information of limited or no value. We start with these steps: n Start by analyzing the content to determine what it is. At the same time, review the retention schedule to get a sense for what needs to be retained and what doesn’t. n Establish classification rules and train the systems with examples. n Use crawlers and recognition engines to evaluate the content and generate a classification. n For content where a high machine confidence factor exists, content is automatically tagged and then staged for migration, either to the appropriate system, or disposition. n For content with low confidence factors, documents are routed to subject matter experts for manual classification. n The results of the manual identification are fed back into the automated algorithms to “teach” the systems better classification. © AIIM aiim.org/CIP The AIIM Official CIP STUDY GUIDE Domain 2: Back to our example, this is how it would work in practice. The analytics engine finds that same document in G:\ Sales. It determines the following: n The document does not appear to be a record because it’s not stored in an approved storage location for records. n The age of the document is 11 years, which seems to be old for a forecast. This is extracted from the date the file was last updated. n The document type is a departmental forecast, based on the naming conventions that were fed into the system. n The system identifies three significant keywords: forecast (from the file name), 2008 (from the file name and date last updated), and draft. This last comes from the DRAFT watermark that appears on every page and which is just metadata to the system. All of these are pulled together and, based on the rules established by humans, the document is given a status of Delete with a 92% confidence rating. Depending on how comfortable the organization is from a risk perspective, the document could be deleted automatically, left alone, or sent to a human for further review. At the very least, though, this approach would identify the most obvious cases for deletion, in bulk, and automatically. Auto-Categorization Auto-categorization tools are often thought of as a technology to assign a lot of metadata to documents without the need for large scale human intervention, saving cost and effort. However, no tools can operate without any human intervention, and quality of results depends on the skill with which the tool is configured and monitored. In migrating very large collections of content into a metadata-and- taxonomy controlled environment these tools can be the only viable strategy for getting a lot of metadata assigned, relatively consistently to content, very quickly. Human beings have neither the patience nor the consistency to do this kind of large scale effort in a short space of time. Auto-categorization tools can also be useful at the search end of the activity chain, especially if common search terms are factored into the auto-categorization activity. Auto-categorization tools use three broad approaches: Business rules. Here, a series of rules are defined by a specialist to say, “given the following criteria, associate the following subject tags to the document.” This is a labor-intensive task and requires a deep knowledge of the content for universal use, but in context-specific cases this can be a very useful strategy. Teaching sets. Here, the administrator compiles a sample set (a “teaching set”) of documents for each concept in the taxonomy. The auto-categorization engine analyzes the content for consistent and characteristic features common to the set using a range of techniques (e.g., word frequency, terms used in combination or close to each other, structural elements), and then goes looking for other documents that match the patterns they have identified. The teaching set approach depends on having sufficient consistent document examples associated with each of your taxonomy concepts, and the auto- categorization engine can lose accuracy if the language and structure of documents relevant to specific concepts “drifts” from the initial training set over time. © AIIM aiim.org/CIP The AIIM Official CIP STUDY GUIDE Domain 2: Semantic analysis. This is a complex pattern sensing technique based on analyzing the contents of documents and the language patterns within them, inferring similarity relationships between documents based on that analysis, and then giving cluster labels to the documents based on rules about where meaningful labels can be found to characterize documents (e.g., in the document titles). In practice, semantic analysis works best as a tool for suggesting categories, which a taxonomy manager can then edit or modify, to connect the content clusters that have been identified with formally researched and tested taxonomy terms. Analytics and the Knowledge Worker Here’s another look at some of the use cases that are specific to knowledge workers, but broadly applicable across industry sectors and locations. This list comes from Content Analyst Company. n Content analytics can apply dynamic clustering to allow for some interesting approaches to how documents are reviewed in bulk. This approach would allow assignment of documents based on topics to those subject matter experts with expertise in a particular topic. n Content-based categorization. This technology attempts to automatically categorize documents into categories based on their content rather than requiring users to manually categorize documents. Again, this goes back to the consistency point made earlier. n Concept search. Traditional search relies on strings of text characters, such as “center” – C-E-N-T-E-R. Traditional search wouldn’t recognize similar terms such as depot, headquarters, downtown, or middle, or even different spellings such as C-E-N-T-R-E. Analytics tools can help to identify concepts based on the term as well as how it’s used in context so that users searching for a particular concept using a particular term also get results or that concept that use different terms. n Email threading. This was one of the original use cases for content analytics in the context of e-discovery. Who knew what, when did they know it, and who did they tell can all be extracted and understood more readily using these tools. n And near-duplicate identification. It’s a simple matter to identify exact duplicates with the same file size, file date/time, and even the identical bitstream. It’s much more challenging to identify minor edits, or Word vs. PDF renditions, and so forth. These tools can help streamline this review process by automatically comparing versions and identifying those discrepancies. © AIIM aiim.org/CIP The AIIM Official CIP STUDY GUIDE Domain 2: Content Analytics for Your Industry This visual was provided by IBM. The use of content analytics has proven business value in obvious use cases like voice of the customer and customer insights. Organizations are actively using analytics to review and analyze customer satisfaction across a variety of information sources and platforms ranging from customer help desk and surveys to social media. Public safety organizations such as police departments rely on analytics to identify and analyze trends in crime rates and response. These are particularly useful for example in community policing and in longer- term initiatives such as anti-gang and anti-terrorism programs. The promise of analytics in healthcare is incredible. From infectious disease outbreak and management, to conducting analysis relating to diseases or treatments, to improving claims management post- treatment, to even identifying trends in helping to prevent readmissions which tend to be more expensive and more acute, analytics is becoming increasingly important to healthcare organizations. Insurance companies have used structured analytics for years to assess risk, provide underwriting, and detect fraud. But the use of content analytics promises to increase access to useful information that is not stored in a relational database to improve these processes. And financial firms can use these analytics processes and tools to identify problematic trends and increase operational efficiency. © AIIM aiim.org/CIP The AIIM Official CIP STUDY GUIDE Domain 2: Issues Associated with Analytics and Artificial Intelligence There are several main issues we’ll look at regarding the effective use of AI. These are: n Resource availability n Training data n Model management n “Black Box” AI n Accuracy Now let’s take a closer look at each of these. Resources Available The first key consideration for analytics and AI is that of resource availability. This consists of two areas of concern. n Computing resources. AI is extraordinarily resource-intensive because of the size of the data sets often involved and the computing tasks associated with performing the various steps involved in the process. It’s only been in the last few years that the pricing has dropped, for two reasons. First, computing power has continued to increase at a rapid rate, including the ability to dedicate processors to these sorts of tasks. Second, Internet bandwidth and cloud processing architectures have made these capabilities available to a much broader audience. n Human resources. All the technology in the world won’t help without the human resources require to: o Make the models o Build the representative data sets o Process and interpret the results o Apply those results to the organization’s actual business issues and desired outcomes o And all the other tasks required to manipulate and manage the data and outputs over time There are numerous studies available decrying the shortage of data scientists, but these are only one cog in the artificial intelligence machine. Existing resources can be brought to bear, but they need training and guidance to do this sort of work effectively. © AIIM aiim.org/CIP The AIIM Official CIP STUDY GUIDE Domain 2: Training Data There was a terrible tragedy a few years ago where a self-driving car (which was using AI to drive, recognize traffic or obstacles, etc.) hit and killed a pedestrian. In this case, the onboard logs showed that the car saw the pedestrian, who was walking a bicycle, but did not brake. The theory is that the car hadn’t been trained to recognize that particular composite – a human pedestrian and a bicycle. This raises a question regarding self-driving cars – how many different possible composites are there that could theoretically be seen on the roads? And for AI, it raises another, similar question: how does the training set measure up to reality? The training set needs to include documents that are similar enough to be readily recognizable as invoices, or contracts, or whatever, while being different enough to be representative of the different variations on the concept. High quality data that is representative of what the organization sees on an ongoing basis is critical to the success of an AI initiative. Model Management Every AI process and project includes the creation of one – or many models. These models are based on reality, but they are not reality and cannot account for every single possible instance or exception. As the saying goes, “All models are wrong; some models are useful.” So the models used for an AI or analytics project might be useful… at the time it’s built, because it is modeling business conditions, constraints, etc. at the time it was built. But things change over time. How the business operates, the legal or regulatory environment, technologies, etc. all change, and these days they seem to change quite quickly. When conditions change, the model needs to be changed as well – if it can be. This means there needs to be active monitoring of how the model is performing, and when it starts to degrade, it either needs to be updated – rules, training data sets, corrections to outputs, etc. – or it needs to be replaced with a new model, and that model needs to have the same input from the business. “Black Box” AI One of the key concerns around AI is that so many of them operate as a sort of “black box” where raw data goes in and intelligence, or a decision, or at least a recommendation comes out the other side – without an understanding of how one led to the other. Was there bias, implicit or explicit? Was the training data sufficiently representative? There are so many different flavors of AI that could be contributing that it becomes difficult to trust the decision. On the other hand, if the decision is explainable and repeatable, this goes a long way towards underscoring the value of the resulting information. This also means that there needs to be effective security to ensure that the data cannot be tampered with before, during, or after the analytical process. This transparency is important for a second reason: it’s required under the law, or at least under some laws and regulations. The Equal Opportunity Credit Act in the U.S. and the General Data Protection Regulation in the European Union both require transparency and an explanation when a negative decision is made on the basis of automated analysis. And they are certainly not alone. Finally, if you don’t know what’s going on with the model, it’s very difficult to improve how it works. © AIIM aiim.org/CIP The AIIM Official CIP STUDY GUIDE Domain 2: Accuracy The last issue is that of accuracy. There is often a misperception that analytics are, or should be, 100% accurate out of the box. This is not true and likely not possible for a variety of reasons including several we’ve discussed already. For some AI-based processes it may be impossible for the results to ever be 100% accurate. So, dispelling this misconception is one of the first things that needs to happen as part of an AI initiative. Now, it is true that analytics can be as accurate as humans, if not more, in some respects (and substantially less in others). Computers are uniquely positioned to make sense out of very subtle patterns, and not very good at image recognition – yet. Perhaps more importantly, even where AI gets something wrong, it will generally get it wrong consistently and repeatedly. Compare this with the variety of ways in which humans can make mistakes! But ultimately organizations need to recognize that analytics and AI are just tools, and their value to the organization will vary significantly depending on the issues we’ve identified here and how they are addressed. This is often one of the most significant issues to overcome in getting buy-in and continuing support for an AI initiative. © AIIM aiim.org/CIP The AIIM Official CIP STUDY GUIDE Domain 2: Artificial Intelligence with Large Language Model (LLM) Organizations that are at the forefront of digital transformation continue adopt to new technologies to improve their operations, manage risks, attract and retain skilled employees, enter new markets, launch new products and services, etc. Digital transformation is a very broad term and includes a plethora of technologies. Artificial Intelligence (AI) is one such technology that is associated with digital transformation. Organizations are implementing AI-related solutions to assess risk, improve customer experience, search for information, identify vulnerabilities in firewalls, etc. As many practitioners know, AI is being used to analyzing content and auto-classify it to improve in information governance, information management, and records management. AI has existed since the early days of computers in academic research, and then later in commercial products, and business services, not to mention in books, movies, and TV shows. AI itself is a generic term that includes several fields of research, products, and services, such as machine learning, machine teaching, expert systems, etc. These research fields have sub-fields of research such as machine vision, deep learning, neural networks, natural language processing, chatbots, and predicative analytics. The research field of machine learning dates back to the 1950s. This is about the time when Arthur Samuel at IBM coined the term “machine learning” in 1959. Teaching and training AI tools depends on vast amounts of data from which researchers and technology vendors develop AI models. The models then use inputs to process the information to produce an output. In the case of machine learning, the AI tool develops its own model, instead of humans explicitly programing an algorithm and training the AI tool. In machine learning, the AI builds its own algorithm, and adapts it to improve the model’s accuracy and predictive outputs. © AIIM aiim.org/CIP The AIIM Official CIP STUDY GUIDE Domain 2: Without getting into too much detail, there are three types of machine learning algorithms tasks. The first is supervised learning when the AI tool processes labelled training data. The second is unsupervised or self-supervised learn when the AI tool processes unlabelled. The third is semi-supervised learning when the AI tool process some labelled data, but mostly unlabelled data. The labelled data tells information about the data such that the AI tool is processing and learning from the data. Language models are an example of machine learning. Learning models use machine learning to improve the accuracy of their predictive outputs as they process the data. The predictive outputs is the next word, based on what the model has already seen. More specifically, a language model is a probabilistic model that predicts the probability of the next word based on the sequence of a previous words the model has seen and learnt. In other words, language models are “probability engines.” When talking about large learning models (LLMs), they use vast amount of textual data – many petabytes of data – scraped from the Internet to learn and predict the next word. Today, LLMs are easily accessible on the Internet to any organization and individual. Many organizations are moving to the cloud and adopting the cloud computing model. The cloud computing consists of three key services – Infrastructure as a Service (IaaS), Platform as a Service (PaaS), and Software as a Service (SaaS). One emerging trend in the could computing model and Artificial Intelligence as a Service (AIaaS). LLMs are an example of AIaaS. As with any technology, LLMs have many benefits, but may also cause many problems if not used correctly or used maliciously. LLMs can help improve auto-classification of content at scale across structured and unstructured content multiple repositories by extracting metadata. The LLM will improve its accuracy of auto- classification over time. Depending on the use case, LLM can auto-classify to improve compliance of sensitive information such as PII, PHI, intellectual property, national security, etc. This can help reduce human involvement so that people can focus on more strategic and other value-added tasks. Another benefit of LLMs is that they can provide quickly the starting of developing something – like an outline for a report, drafting a content for a presentation, suggesting possible steps to solve a problem, developing program code, preparing the list qualifications for a job posting, etc. The next step still requires human involvement to understand the output in the context of the question given to the LLM and then to start improving the draft. In other words, LLMs can help reduce time and effort of the task, but do not entirely eliminate human involvement. LLM also have a downside such having bias. This can be caused by LLM developers having unintended bias. Another source of bias can be data used for the LLM to learn – an example of “garbage in, garbage out.” Another downside is that LLMs can end up fabricating responses that are not factual, incorrect, or unreal. This is referred to as “hallucinations.” Hallucinations occur because LLMs do not have a concept of fact. So, the LLM is predicting the next word based on what the m