Chi-Squared Test: Pearson's Chi-Squared Test - PDF
Document Details
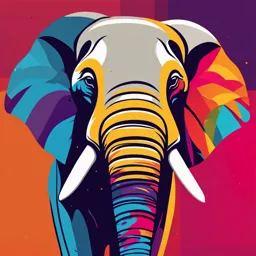
Uploaded by LargeCapacityAntigorite4770
Tags
Summary
The document explains Pearson's Chi-Squared Test, introduced in 1900, designed to identify meaningful patterns in categorical data and differences not due to random chance. The document discusses expectations, observations, and uses an example to define the expected behavior of two categorical variables by using tables to analyze income level and smoking status.
Full Transcript
Chi-Squared Test Pearsonβs π 2 Test The π 2 (βchiβ-squared, pronounced like the last syllable of sky) test allows us to assess if observed diο¬erences in the behavior of categorical data is explained by random chance or represents a meaningful pattern. This method was developed by Karl Pearson and in...
Chi-Squared Test Pearsonβs π 2 Test The π 2 (βchiβ-squared, pronounced like the last syllable of sky) test allows us to assess if observed diο¬erences in the behavior of categorical data is explained by random chance or represents a meaningful pattern. This method was developed by Karl Pearson and introduced in 1900 - it is a foundational method in modern statistics. Expectations v. Observations Given two categorical variables π and π , it often of interest to ask if these two variables are associated with one another. However, categorical data can be challenging to work with because we cannot map the values of categorical data onto some distribution. The π 2 test was developed to give us a way to map observed patterns in the data between categorical variables onto a theoretical distribution, thus allowing us to conduct a signifance test. The goal with the π 2 Test of Independence is to determine if π and π are related to one another (i.e., if they are dependent on one another)? For example, we might want to know if income level (measured categorically) is related to current smoking status. Since both variables are categorical, we do not have a good way of mapping the behavior of either variable on to a theoretical distribution. But, we can define how we expect the distribution of values of π and π within our sample to be if they are not related to one another (i.e., if they are independent of one another). Letβs use an example to display how we can define the expected behavior of two categorical variables if we assume they are independent of one another. Income Level and Smoking Status Letβs say we have recruited 400 people into a study examining the relationship of income and smoking status. We have measured annual income categorically ($50k) and smoking status categorically (not a current smoker, current smoker). Looking at the sample, we see that 100 people reported an income $50k. Further, we see that 100 people reported currently smoking and the other 300 reported not currently smoking. We can start by generating a cross-tabulation we will use as a framework to build our analysis on, like so: The total row and column display the total number of people that fall into each category. The total value in the bottom right thus displays the total number of people in our study. Within the dotted lines represents the number of people who reported each combination of income and smoking. For example, the empty cell in the top left corner represents the number of people who reported an annual income below $20k and who reported not currently smoking. Before we examine the observed disitribution of our data, we can actually map how we expect the variables to be distributed assuming that income and cigarette smoking are not related. Well, if we assume that these two variables are independent (hint: sounds like a null hypothesis), then we would expect the values of both variables would be evenly distributed across both groups. We are able to calculate the expected value in each π βπ cell using the equation, πππ€ π ππππ’ππ , where ππππ€ is the total number of participants in that given row, πππππ’ππ is the total number in that given column, and π is the total number of participants. Letβs calculate the top-left cell below: Here we can see that ππππ€ = 100 , πππππ’ππ = 300 , and π = 400. Thus, the expected number of people reporting income less than $20k who donβt currently smoke is: 300β100 = 75. We will place the expected 400 value in parentheses in the cell like so: We can repeat this process for each cell, resulting in the following table of expected values: Here, we are assuming that the distribution of income is the same among people who donβt currently smoke and those who currently do. Likewise, under our assumption, we are expecting that distribution of current smoking to be the same across each income level (in this case, 75% not current smoking and 25% current smoking). This represents the expected distribution of our two variables under the assumption that they are independent. Once we have established expected values, we can fill in the actual observed values within our sample. The following table now includes the values (not in parentheses) of what we observed in our sample: We can see, right away, that our observations diο¬er from our expected values. We can see that a greater proportion of people making less than $20k currently smoke than expected and a smaller proportion of those making more than $50k than expected. We need a way to quantify this diο¬erence. The diο¬erence between our expected values πΈπππ€,ππππ’ππ and observed values ππππ€,ππππ’ππ within each cell can be understood to represent our signal. This diο¬erence represents how diο¬erent our observed data is from what we expected under our assumption that the two variables are independent. We could try to sum up all of these diο¬erences, like so: β (ππππ€,ππππ’ππ β πΈπππ€,ππππ’ππ ) This would be the sum of the diο¬erence between the expected and observed value in each cell. Interestingly though, this will always equal 0, so it is not a very useful metric. Letβs do the calculation by hand to display that it equals 0, for our example table above: (50 β 75) + (50 β 25) + (160 β 150) + (40 β 50) + (90 β 75) + (10 β 25) = β 25 + 25 + 10 + (β10) + 15 + (β15) =0 + 0 + 0 =0 This is a similar issue we face when we try to calculate standard deviation π for a normally distributed variable and why we have to calculate the variance π 2 first. Importantly, if you take the square of any value (positive or negative), the result will be positive. So if you take the sum of several squares, your result will only be 0 if your data perfectly matches your expected values. We can define this new sum like so: (ππππ€,ππππ’ππ β πΈπππ€,ππππ’ππ )2 β Now, when we take this sum, we get a meaningful value, like so: (50 β 75)2 + (50 β 25)2 + (160 β 150)2 + (40 β 50)2 + (90 β 75)2 + (10 β 25)2 = β 252 + 252 + 102 + (β10)2 + 152 + (β15)2 =625 + 625 + 100 + 100 + 225 + 225 =1900 This is much better and still represents the strength of our signal (i.e., diο¬erence between observed and expected values)! However, we can notice that the size of this value is also dependent on the size of our study sample. We could have sampled twice as many people with an identical proportional distribution of observed results and the strength of the signal would appear much larger, even though the proportional distribution across groups is the same. As such, it is important that we take a step to standardize our calculation of the strength of our signal. We do this by dividing the diο¬erence between each observed and expected value by the expected value. Now, instead of representing the crude diο¬erence between observed and expected values, each term represents the diο¬erence between observed and expected values relative to the expected value. We can define this mathematically like so: 2 (ππππ€,ππππ’ππ β πΈπππ€,ππππ’ππ )2 β π = πΈπππ€,ππππ’ππ In this instance, we are able to calculate: (50 β 75)2 (50 β 25)2 (160 β 150)2 (40 β 50)2 (90 β 75)2 (10 β 25)2 π2 = + + + + + 75 25 150 50 75 25 2 π =625/75 + 625/25 + 100/150 + 100/50 + 225/75 + 225/25 π 2 =48 We have now calculated a standardized value representing the strength of our signal under the assumption that no signal exists. It turns out that this value corresponds to a π 2 distribution with (πππ€π β 1) β (ππππ’πππ β 1) = (3 β 1) β (2 β 1) = 2 β 1 = 2 degrees of freedom. This is very cool, because it allows us to use this metric to assess the probability of our observed data under our assumption that our two variables are not related to one another. But, this should immediately beg the question: what is a π 2 distribution? The π 2 Distribution Earlier, we learned about how the normal distribution arises from how we understand certain natural phenomenon to randomly occur. A normally distributed variable is one where the mean value is the most likely value to observe, where values closer to the mean are more likely than values further from the mean, and where values less than the mean are equally as likely to occur as those greater than the mean. The standard normal distribution (π -distribution) is depicted like so: ## Let's create our x-axis, ranging from -5 to 5, with increments of 0.1 x