Chapter 7. Asynchronous Messaging PDF
Document Details
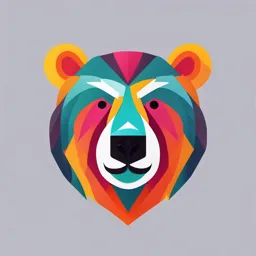
Uploaded by IdyllicResilience5759
Newgiza University
Tags
Summary
This document discusses asynchronous messaging in distributed systems. It explains the concepts of producers, consumers, and message brokers, highlighting their role and function in facilitating communication. The document also introduces RabbitMQ as an example of a messaging system.
Full Transcript
**Chapter 7. Asynchronous Messaging** ===================================== Inevitably for a distributed systems book, I've spent a fair bit of time in the preceding chapters discussing communications issues. Communication is fundamental to distributed systems, and it is a major issue that architec...
**Chapter 7. Asynchronous Messaging** ===================================== Inevitably for a distributed systems book, I've spent a fair bit of time in the preceding chapters discussing communications issues. Communication is fundamental to distributed systems, and it is a major issue that architects need to incorporate into their system designs. So far, these discussions have assumed a synchronous messaging style. A client sends a response and waits for a server to respond. This is how most distributed communications are designed to occur, as the client requires an instantaneous response to proceed. Not all systems have this requirement. For example, when I return some goods I've purchased online, I take them to my local UPS or FedEx store. They scan my QR code, and I give them the package to process. I do not then wait in the store for confirmation that the product has been successfully received by the vendor and my payment returned. That would be dull and unproductive. I trust the shipping service to deliver my unwanted goods to the vendor and expect to get a message a few days later when it has been processed. We can design our distributed systems to emulate this behavior. Using an asynchronous communications style, clients, known as producers, send their requests to an intermediary messaging service. This acts as a delivery mechanism to relay the request to the intended destination, known as the consumer, for processing. Producers "fire and forget" the requests they send. Once a request is delivered to the messaging service, the producer moves on to the next step in their logic, confident that the requests it sends will eventually get processed. This improves system responsiveness, in that producers do not have to wait until the request processing is completed. In this chapter I'll describe the basic communication mechanisms that an asynchronous messaging system supports. I'll also discuss the inherent trade-offs between throughput and data safety---basically, making sure your systems don't lose messages. I'll also cover three key messaging patterns that are commonly deployed in highly scalable distributed systems. To make these concepts concrete, I'll describe [RabbitMQ](https://oreil.ly/j9eHD), a widely deployed open source messaging system. After introducing the basics of the technology, I'll focus on the core set of features you need to be aware of in order to design a high-throughput messaging system. **Introduction to Messaging** ============================= Asynchronous messaging platforms are a mature area of technology, with multiple products in the space.[**1**](https://learning.oreilly.com/library/view/foundations-of-scalable/9781098106058/ch07.html#ch01fn19) The venerable IBM MQ Series appeared in 1993 and is still a mainstay of enterprise systems. The Java Messaging Service (JMS), an API-level specification, is supported by multiple JEE vendor implementations. RabbitMQ, which I'll use as an illustration later in this chapter, is arguably the most widely deployed open source messaging system. In the messaging world, you will never be short of choice. While the specific features and APIs vary across all these competing products, the foundational concepts are pretty much identical. I'll cover these in the following subsections, and then describe how they are implemented in RabbitMQ in the next section. Once you appreciate how one messaging platform works, it is relatively straightforward to understand the similarities and differences inherent in the competition. Messaging Primitives -------------------- Conceptually, a messaging system comprises the following: This scheme is illustrated in [Figure 7-1](https://learning.oreilly.com/library/view/foundations-of-scalable/9781098106058/ch07.html#a_simple_messaging_system). A simple messaging system ###### **Figure 7-1. A simple messaging system** A message broker is a service that manages one or more queues. When messages are sent from producers to a queue, the broker adds messages to the queue in the order they arrive---basically a FIFO approach. The broker is responsible for efficiently managing message receipt and retention until one or more consumers retrieve the messages, which are then removed from the queue. Message brokers that manage many queues and many requests can effectively utilize many vCPUs and memory to provide low latency accesses. Producers send messages to a named queue on a broker. Many producers can send messages to the same queue. A producer will wait until an acknowledgment message is received from the broker before the send operation is considered complete. Many consumers can take messages from the same queue. Each message is retrieved by exactly one consumer. There are two modes of behavior for consumers to retrieve messages, known as *pull* or *push*. While the exact mechanisms are product-specific, the basic semantics are common across technologies: - - Generally, utilizing the push mode when available is much more efficient and recommended. It avoids the broker being potentially swamped by requests from multiple consumers and makes it possible to implement message delivery more efficiently in the broker. Consumers will also acknowledge message receipt. Upon consumer acknowledgment, the broker is free to mark a message as delivered and remove it from the queue. Acknowledgment may be done automatically or manually. If automatic acknowledgment is used, messages are acknowledged as soon as they are delivered to the consumer, and before they are processed. This provides the lowest latency message delivery as the acknowledgment can be sent back to the broker before the message is processed. Often a consumer will want to ensure a message is fully processed before acknowledgment. In this case, it will utilize manual acknowledgments. This guards against the possibility of a message being delivered to a consumer but not being processed due to a consumer crash. It does, of course, increase message acknowledgment latency. Regardless of the acknowledgment mode selected, unacknowledged messages effectively remain on the queue and will be delivered at some later time to another consumer for processing. Message Persistence ------------------- Message brokers can manage multiple queues on the same hardware. By default, message queues are typically memory based, in order to provide the fastest possible service to producers and consumers. Managing queues in memory has minimal overheads, as long as memory is plentiful. It does, however, risk message loss if the server were to crash. To guard against message loss---a practice known as data safety---queues can be configured to be persistent. When a message is placed on a queue by a producer, the operation does not complete until the message is written to disk. This scheme is depicted in [Figure 7-2](https://learning.oreilly.com/library/view/foundations-of-scalable/9781098106058/ch07.html#persisting_messages_to_disk). Now, if a message broker should fail, on reboot it can recover the queue contents to the state they existed in before the failure, and no messages will be lost. Many applications can't afford to lose messages, and hence persistent queues are necessary to provide data safety and fault tolerance.  ###### **Figure 7-2. Persisting messages to disk** Persistent queues have an inherent increase in the response time for send operations, with the trade-off being enhanced data safety. Brokers will usually maintain the queue contents in memory as well as on disk so messages can be delivered to consumers with minimal overhead during normal operations. Publish--Subscribe ------------------ Message queues deliver each message to exactly one consumer. For many use cases, this is exactly what you want---my online purchase return needs to be consumed just once by the originating vendor---so that I get my money back. Let's extend this use case. Assume the online retailer wants to do an analysis of all purchase returns so it can detect vendors who have a high rate of returns and take some remedial action. To implement this, you could simply deliver all purchase return messages to the respective vendor *and* the new analysis service. This creates a one-to-many messaging requirement, which is known as a publish--subscribe architecture pattern. In publish--subscribe systems, message queues are known as *topics*. A topic is basically a message queue that delivers each published message to one of more subscribers, as illustrated in [Figure 7-3](https://learning.oreilly.com/library/view/foundations-of-scalable/9781098106058/ch07.html#a_publishen_dashsubscribe_broker_archit). A publish--subscribe broker architecture ###### **Figure 7-3. A publish--subscribe broker architecture** With publish--subscribe, you can create highly flexible and dynamic systems. Publishers are decoupled from subscribers, and the number of subscribers can vary dynamically. This makes the architecture highly extensible as new subscribers can be added without any changes to the existing system. It also makes it possible to perform message processing by a number of consumers in parallel, thus enhancing performance. Publish--subscribe places an additional performance burden on the message broker. The broker is obliged to deliver each message to all active subscribers. As subscribers will inevitably process and acknowledge messages at different times, the broker needs to keep messages available until all subscribers have consumed each message. Utilizing a push model for message consumption provides the most efficient solution for publish--subscribe architectures. Publish--subscribe messaging is a key component for building distributed, event-driven architectures. In event-driven architectures, multiple services can publish events related to some state changes using message broker topics. Services can register interest in various event types by subscribing to a topic. Each event published on the topic is then delivered to all interested consumer services. I'll return to event-driven architectures when microservices are covered in [Chapter 9](https://learning.oreilly.com/library/view/foundations-of-scalable/9781098106058/ch09.html#microservices).[**2**](https://learning.oreilly.com/library/view/foundations-of-scalable/9781098106058/ch07.html#ch01fn20) Message Replication ------------------- In an asynchronous system, the message broker is potentially a single point of failure. A system or network failure can cause the broker to be unavailable, making it impossible for the systems to operate normally. This is rarely a desirable situation. For this reason, most message brokers enable logical queues and topics to be physically replicated across multiple brokers, each running on their own node. If one broker fails, then producers and consumers can continue to process messages using one of the replicas. This architecture is illustrated in [Figure 7-4](https://learning.oreilly.com/library/view/foundations-of-scalable/9781098106058/ch07.html#message_queue_replication). Messages published to the leader are mirrored to the follower, and messages consumed from the leader are removed from the follower.  ###### **Figure 7-4. Message queue replication** The most common approach to message queue replication is known as a leader-follower architecture. One broker is designated as the leader, and producers and consumers send and receive messages respectively from this leader. In the background, the leader replicates (or mirrors) all messages it receives to the follower, and removes messages that are successfully delivered. This is shown in [Figure 7-4](https://learning.oreilly.com/library/view/foundations-of-scalable/9781098106058/ch07.html#message_queue_replication) with the *replicate* and *remove* operations. How precisely this scheme behaves and the effects it has on broker performance is inherently implementation, and hence product dependent. With leader-follower message replication, the follower is known as a hot standby, basically a replica of the leader that is available if the leader fails. In such a failure scenario, producers and consumers can continue to operate by switching over to accessing the follower. This is also called *failover*. Failover is implemented in the client libraries for the message broker, and hence occurs transparently to producers and consumers. Implementing a broker that performs queue replication is a complicated affair. There are numerous subtle failure cases that the broker needs to handle when duplicating messages. I'll start to raise these issues and describe some solutions in Chapters (https://learning.oreilly.com/library/view/foundations-of-scalable/9781098106058/ch10.html#scalable_database_fundamentals) and (https://learning.oreilly.com/library/view/foundations-of-scalable/9781098106058/ch11.html#eventual_consistency) when discussions turn to scalable data management. ###### Warning Some advice: don't contemplate *rolling your own* replication scheme, or any other complex distributed algorithm for that matter. The software world is littered with failed attempts to build application-specific distributed systems infrastructure, just because the solutions available "don't do it quite right for our needs" or "cost too much." Trust me---your solution will not work as well as existing solutions and development will cost more than you could ever anticipate. You will probably end up throwing your code away. These algorithms are really hard to implement correctly at scale. **Messaging Patterns** ====================== With a long history of usage in enterprise systems, a [comprehensive catalog of design patterns](https://oreil.ly/DuhYA) exists for applications that utilize messaging. While many of these are concerned with best design practices for ease of construction and modification of systems and message security, a number apply directly to scalability in distributed systems. I'll explain three of the most commonly utilized patterns in the next sections. Competing Consumers ------------------- A common requirement for messaging systems is to consume messages from a queue as quickly as possible. With the [competing consumers pattern](https://oreil.ly/WHatQ), this is achieved by running multiple consumer threads and/or processes that concurrently processes messages. This enables an application to scale out message processing by horizontally scaling the consumers as needed. The general design is shown in [Figure 7-7](https://learning.oreilly.com/library/view/foundations-of-scalable/9781098106058/ch07.html#the_competing_consumers_pattern). The competing consumers pattern ###### **Figure 7-7. The competing consumers pattern** Using this pattern, messages can be distributed across consumers dynamically using either the push or a pull model. Using the push approach, the broker is responsible for choosing a consumer to deliver a message to. A common method, which, for example, is implemented in RabbitMQ and ActiveMQ, is a simple round-robin distribution algorithm. This ensures an even distribution of messages to consumers. With the pull approach, consumers simply consume messages as quickly as they can process them. Assuming a multithreaded consumer, if one consumer is running on an 8-core node and another on a 2-core node, we'd expect the former would process approximately four times the amount of messages of the latter. Hence, load balancing occurs naturally with the pull approach. There are three key advantages to this pattern, namely: Support for competing consumers will be found in any production-quality messaging platform. It is a powerful way to scale out message processing from a single queue. Exactly-Once Processing ----------------------- As I discussed in [Chapter 3](https://learning.oreilly.com/library/view/foundations-of-scalable/9781098106058/ch03.html#distributed_systems_essentials), transient network failures and delayed responses can cause a client to resend a message. This can potentially lead to duplicate messages being received by a server. To alleviate this issue, we need to put in place measures to ensure idempotent processing. In asynchronous messaging systems, there are two sources for duplicate messages being processed. The first is duplicates from the publisher, and the second is consumers processing a message more than once. Both need to be addressed to ensure exactly-once processing of every message. The publisher part of the problem originates from a publisher retrying a message when it does not receive an acknowledgment from the message broker. If the original message was received and the acknowledgment lost or delayed, this may lead to duplicates on the queue. Fortunately, some message brokers provide support for this duplicate detection, and thus ensure duplicates do not get published to a queue. For example, the ActiveMQ Artemis release can [remove duplicates](https://oreil.ly/WZnGm) that are sent from the publisher to the broker. The approach is based on the solution I described in [Chapter 3](https://learning.oreilly.com/library/view/foundations-of-scalable/9781098106058/ch03.html#distributed_systems_essentials), using client-generated, unique *idempotency key* values for each message. Publishers simply need to set a specific message property to a unique value, as shown in the following code: ClientMessage msg = session.createMessage(true); UUID idKey = UUID.randomUUID(); // use as idempotence key msg.setStringProperty(HDR\_DUPLICATE\_DETECTION\_ID, idKey.toString() ); The broker utilizes a cache to store idempotency key values and detect duplicates. This effectively eliminates duplicate messages from the queue, solving the first part of your problem. On the consumer side, duplicates occur when the broker delivers a message to a consumer, which processes it and then fails to send an acknowledgment (consumer crashes or the network loses the acknowledgment). The broker therefore redelivers the message, potentially to a different consumer if the application utilizes the competing consumer pattern. It's the obligation of consumers to guard against duplicate processing. Again, the mechanisms I described in [Chapter 3](https://learning.oreilly.com/library/view/foundations-of-scalable/9781098106058/ch03.html#distributed_systems_essentials), namely maintaining a cache or database of idempotency keys for messages that have been processed. Most brokers will set a message header that indicates if a message is a redelivery. This can be used in the consumer implementation of idempotence. It doesn't guarantee a consumer has seen the message already. It just tells you that the broker delivered it and the message remains unacknowledged. Poison Messages --------------- Sometimes messages delivered to consumers can't be processed. There are numerous possible reasons for this. Probably most common are errors in producers that send messages that cannot be handled by consumers. This could be for reasons such as a malformed JSON payload or some unanticipated state change, for example, a *StudentID* field in a message for a student who has just dropped out from the institution and is no longer active in the database. Regardless of the reason, these *poison messages* have one of two effects: - - In either case, assuming consumer acknowledgments are required, the message remains on the queue in an unacknowledged state. After some broker-specific mechanism, typically a timeout or a negative acknowledgment, the poison message will be delivered to another consumer for processing, with predictable, undesirable results. If poison messages are not somehow detected, they can be delivered indefinitely. This at best takes up processing capacity and hence reduces system throughput. At worst it can bring a system to its knees by crashing consumers every time a poison message is received. The solution to poison message handling is to limit the number of times a message can be redelivered. When the redelivery limit is reached, the message is automatically moved to a queue where problematic requests are collected. This queue is traditionally and rather macabrely known as the *dead-letter queue*. As you no doubt expect by now, the exact mechanism for implementing poison message handling varies across messaging platforms. For example, Amazon Simple Queue Service (SQS) defines a policy that specifies the dead-letter queue that is associated with an application-defined queue. The policy also states after how many redeliveries a message should be automatically moved from the application queue to the dead-letter queue. This value is known as the maxReceiveCount. In SQS, each message has a ReceiveCount attribute, which is incremented when a message is not successfully processed by a consumer. When the ReceiveCount exceeds the defined maxReceiveCount value for a queue, SQS moves the message to the dead-letter queue. Sensible values for redelivery vary with application characteristics, but a range of three to five is common. The final part of poison message handling is diagnosing the cause for messages being redirected to the dead-letter queue. First, you need to set some form of monitoring alert that sends a notification to engineers that a message has failed processing. At that stage, diagnosis will comprise examining logs for exceptions that caused processing to fail and analyzing the message contents to identify producer or consumer issues.