Chapter 3 - Notes PDF
Document Details
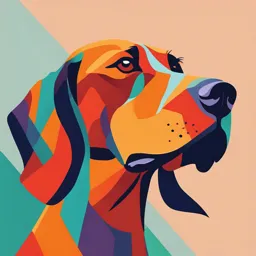
Uploaded by SpellboundLove
Universiteit Gent
Tags
Summary
This chapter details the strategies for designing biocatalysts, focusing on semi-rational engineering. It covers selection and optimization of enzyme templates, as well as the use of statistical and computational methods to make informed choices about mutations.
Full Transcript
3. Semi-rational engineering How to design an industrially relevant biocatalyst? Adapted from: Status of protein engineering for biocatalysts, Bommarius 2011 The ideal industrial biocatalyst should have high specific activity combined with high specificity on the reactant in question, the highest po...
3. Semi-rational engineering How to design an industrially relevant biocatalyst? Adapted from: Status of protein engineering for biocatalysts, Bommarius 2011 The ideal industrial biocatalyst should have high specific activity combined with high specificity on the reactant in question, the highest possible enantioselectivity in case of generating a chiral center, and should be stable at industrial process conditions, that is, often higher than ambient temperature and in partially or wholly nonaqueous solvents. The advent of molecular biology since the late 1970s embodied in protein engineering protocols, progress in crystallography and computational methods has enabled the design of protein catalysts for targeted applications and improvement of desired traits. Despite these technical advances, however, it still remains unclear what the ‘optimal’ strategy for enzyme engineering actually looks like. Indeed, a systematic comparison of the various approaches would be needed to come up with a unified algorithm that combines all of their best features. Nevertheless, some general guidelines have already been formulated, which provide a framework for creative tinkering to maximize one’s success rate on a case-by-case basis. 3-1 Step 1: select the best template As it is extremely difficult to introduce a totally new specificity in an enzyme, the template should preferably already show some (side) activity on the target substrate. It is, therefore, essential to first test the natural variants of a given enzyme and select the one that forms the best starting point. If there are too many, a set of representatives could be picked from different branches of the family tree, possibly guided by sequence logos to ensure that all relevant motifs (“fingerprints”) are covered. Thermophilic microorganisms should get particular attention as source of enzymes, as these typically are more robust and better suited for subsequent manipulations. If the natural diversity does not offer a suitable starting point for enzyme engineering, one could potentially be created through neutral drift, to introduce the necessary promiscuity. Alternatively, one could start directly from the family’s ancestor sequence, although that is not always easy to reconstruct (see further). Step 2: stabilize the template (further) Thermostability is an important parameter in the development of industrially applicable biocatalysts. Furthermore, thermostability has been linked to the ability of an enzyme to tolerate mutations, which are mostly destabilizing. Indeed, at some point during consecutive rounds of mutagenesis and screening, the protein stability can fall below a certain threshold after which proper folding is jeopardized. If the starting point is sufficient (thermo)stable, the chances of running into problems are perhaps relatively slim. Nevertheless, it might be 3-2 worthwhile to check the stability of the obtained mutant and improve it (again) before further use. In contrast, if the starting point is mesophilic, the focus should first be on stabilization. In that respect, it is interesting to note that neutral drift has been found to generate a lot of variants that are considerably more stable. In most cases, the stabilizing mutations were found to the consist of the so-called consensus residue, i.e. the type of aminoacid that occurs most frequently at that position within the whole family. Therefore, starting the engineering project directly from the family’s consensus sequence could be a good strategy (see further). Step 3: semi-rational engineering of the active-site The ultimate goal of semi-rational engineering is to generate “small but smart” libraries that reduce the screening effort while also increasing the hit rate. To that end, the randomization is limited to certain positions (so-called hotspots) that are believed to influence the property under investigation. To modify substrate specificity, for example, mutations should be focused around the active site (although long-distance effects can also come into play). The library can be further downsized by restricting the type of amino acids that are tested, e.g. only certain chemical functionalities (charged, polar,…) or only those that occur naturally at a given position within the whole family. The different strategies to find hotspots and to design smart libraries will form the main focus of the current chapter. 3-3 Step 4: find optimal combination of beneficial mutations Lastly, statistical models can be used to find new and improved combinations of mutations. For example, the ProSAR (PROtein Sequence Activity Relationship) model is able to predict high performing variants that were not sampled experimentally by processing sequence and activity data from just a small number of screened variants containing multiple mutations. The method thus enables identification of beneficial mutations even in variants with reduced functionality. Nowadays, more advanced machine-based learning techniques are constantly being developed, which will be discussed in the next chapter. 3-4 Design of an ancestral/consensus sequence Further reading: Exploring the past and the future of protein evolution, Gumulya 2017 While the possibility of resurrecting ancient proteins was already suggested in 1963 by Linus Pauling, decades have passed before the first synthesis of an ancestral protein was actually undertaken. The power of this approach, termed ancestral sequence reconstruction (ASR), lies in the ability to test evolutionary hypotheses by inferring the sequence of ancient proteins based on their extant descendants. For that goal, several statistical methods are available, but the most powerful and accurate make use of a maximum likelihood algorithm. This allow to calculate the most likely sequence at each node of the phylogenetic tree of an enzyme family considering the three’s topology, i.e. the location and length of branches. The first and still most famous software package that uses this approach is called PAML (Phylogenetic Analysis by Maximum Likelihood) but FastML is a good and user-friendly online alternative. MrBayes, in contrast, makes use of a bayesian algorithm that also evaluates different models (topologies) for the tree itself. A general limitation of all reconstruction methods is the handling of insertions and deletions (indels), often necessitating potentially subjective interpretations to be made by the investigator. Furthermore, as one travels back in time (up to several millions of years), the probability that the node’s sequence is inferred correctly can become very low. For example, even if every position in a 500-residue protein is inferred with an impressive 0.95 probability, the probability that the total sequence is correct is less than 10–11 (the product of the probabilities for all individual sites). Therefore, it may be more reasonable to consider a population of possible ancestors than a single ancestral state. Due to cost and time constraints, only the most likely sequence is typically synthesized and characterized in the lab. However, some variability should be allowed at ambiguous positions, either by synthesizing several individual variants or by constructing the corresponding libraries for screening purposes. 3-5 In 1976, Jensen hypothesized that specialized enzymes have evolved from more generalist (i.e. more promiscuous) primordial forms and that the specificity of modern enzymes has been tuned to an optimal state by natural selection. For example, the ancestor of a fungal glucosidase has been found to show low activity on both maltose and isomaltose, while the current enzyme has lost the ability to hydrolyze the latter and became specialized towards the former. Modern enzymes might no longer offer the required flexibility to modify their specificity by directed evolution. Indeed, careful analysis of mutational pathways in engineering experiments has revealed that sequences first tend to drift back towards their progenitor before a switch to a new activity is achieved. Starting an engineering project directly from an ancestral sequence might thus be a good strategy to ensure that the necessary promiscuity is present. Based on the assumption that the Earth experienced periods of elevated ambient temperature in primordial times, it has also been predicted that ancient proteins may have been generally 3-6 more thermostable than extant forms. Several studies have meanwhile confirmed that the thermal stability profile of an enzyme generally increases as one goes back in time, and this result has been interpreted as evidence of ancient proteins needing to survive a hot ancient global environment. However, the oldest ancestors do not necessarily show the highest thermal stability, meaning that the trait can be readily gained and lost throughout evolutionary history as proteins traverse their separate evolutionary paths to adapt to the local conditions under which they were selected. The increases in denaturation temperature of ∼30°C that have been obtained by resurrecting ancestors are much larger than those typically obtained with other engineering techniques. Moreover, the ancestral resurrection approach allows for the stabilization of protein without requiring detailed structural information. Instead of using the ancestor as the starting point for an engineering project, its sequence can also be used as source of inspiration to introduce relevant mutations into a modern enzyme. The first examples of this strategy evaluated just a few selected substitutions, resulting in moderately improved stabilities and/or specificities. In more recent studies, small libraries have been created that randomly recombine all residues that differ between the enzyme and its ancestor. These libraries were found to contain a remarkably high percentage of active variants (~70%), several of which showed higher activity and/or promiscuity than the starting point. Ancestral libraries therefore comprise a means of focusing diversity to crucial positions and functional residues, thereby facilitating the isolation of new variants by low-throughput screening. The phylogenetic analysis can also be used to identify crucial differences between two modern enzymes without necessarily having to accurately infer their ancestral sequence. Indeed, through the Reconstruction of Evolutionary Adaptive Pathways (REAP), positions can be revealed that show functional divergence, i.e. are conserved in one branch of the tree but are different in another. This approach has some resemblance to the identification of so-called correlated positions in protein families, as will be discussed later in this chapter. 3-7 An alternative to ancestral mutation, but one that is superficially similar and sometimes inappropriately assumed to be effectively the same, is the consensus approach, in which the residue most commonly found at a given position in a protein family is introduced into an extant protein. The consensus approach is commonly used in enzyme engineering to generate protein variants with increased stability, based on the hypothesis that the residue most commonly found at a given position is likely to be the one providing greatest fitness, i.e. commonly the best stability. However, it must be stressed that no information on phylogenetic relationships is incorporated into the consensus approach and that the output can be heavily biased towards certain genera that are overrepresented in sequence databases (or in the environment). Indeed, several studies have demonstrated the superiority of true ASR in terms of producing proteins that can be expressed at high levels and show greater thermal stability. 3-8 Nevertheless, the consensus sequence is much easier to deduce from an alignment and has proven its value on several occasions, either as full-length protein or as source of information to introduce just a few selected mutations. 3-9 Creating ‘focused’ libraries by saturation mutagenesis Further reading: The Crucial Role of Methodology Development in Directed Evolution, Qu 2019 In semi-rational engineering, positions are first identified that are likely to influence the property under investigation. These hotspots are then randomized at the DNA level through a PCR reaction with a primer (mixture) that contains all possible nucleotides at the corresponding codon (referred to as a “degenerate” codon/primer). As a result, all 20 amino acids can be evaluated at the selected hotspot, hence the name site-saturation mutagenesis (SSM). The obtained plasmid mixture is then transformed into a suitable host for enzyme expression and screening. Afterwards, the hits have to be sequenced to reveal which mutation they contain. Although this might seem unnecessary complicated, it certainly is more efficient than introducing the 20 amino acids individually by means of site-directed mutagenesis. Indeed, SSM requires just a single PCR reaction and transformation, after which the mixture can be separated at the colony level. The only downside is that oversampling of these colonies is needed to maximize the chances of having tested all protein variants. For example, a threefold excess of transformants (= 96 colonies or 1 microwell plate, see further) should be screened to ensure 95% coverage of the library. Three-fold oversampling to achieve 95% coverage NNN NNK NDT “Tang” # Codon Colony Codon Colony Codon Colony Codon Colony 1 64 192 32 96 12 36 20 60 2 4,096 12,288 1,024 3,072 144 432 400 1,200 3 262,144 786,432 32,768 98,304 1,728 5,184 8,000 24,000 # number of positions randomized simultaneously After applying SSM to several positions, the beneficial mutations can be joined together. However, this strategy assumes that the effect of individual mutations is simply additive, which is definitely not always true. Diminishing returns may be observed when combining multiple positive mutations. In certain cases, two beneficial amino acid substitutions may even be incompatible with each other, resulting in a final enzyme variant that performs worse. In order to minimize this risk, an approach known as iterative saturation mutagenesis (ISM) is frequently applied. After a first round of saturation and screening, the (best) hit is then used 3-10 as template for the randomization of a next hotspot and so-on. With ISM, the cooperative effect between new mutations and previously identified hits is already taken into consideration during screening. However, the best results can typically be obtained by combinatorial saturation mutagenesis (CSM) where two or more hotspots are randomized simultaneously. By doing so, the optimal combination of mutations that leads to the best (synergistic) result can be found. Unfortunately, the randomization of more than one position at once dramatically increases the size of the library, meaning that CSM is only worth the effort if the hotspots are likely to influence each other through a connection in either structure (e.g. close proximity) or sequence (e.g. co-evolution), and if the throughput of the available screening or selection setup is sufficiently high. A fully randomized codon is represented as NNN, where each of the four bases is equally likely to appear at each of the three nucleotide positions. Indeed, such a degenerate codon actually consists of a mixture of 64 codons that are all used as template for enzyme expression. However, the resulting distribution across the 20 amino acids will be far from uniform, with probabilities ranging from 1/64 (for Met and Trp) to 6/64 (for Arg, Leu, and Ser). Furthermore, there is a 3/64 probability that a premature stop codon will be introduced. In contrast, all 20 amino acids can be generated with just 32 codons using the NNK degeneracy (only allowing for T or G at the third position), which has a more uniform spectrum and results in just a single stop codon. Crucially, it also reduces the screening effort from 192 to 96 colonies for 95% coverage (= 3-fold oversampling) of the genetic diversity. That benefit becomes even more pronounced when randomizing multiple positions simultaneously. 3-11 A further lowering of the screening effort can be achieved with restricted alphabets that code for only a portion of the amino acids, which then should be chosen wisely. A useful tool in that respect is CASTER, an excel sheet that lets the user design the most optimal combination of residues. For example, the NDT degeneracy codes for a balanced set of 12 amino acids that covers all of the chemical properties that are available in a protein (positively or negatively charged, polar, hydrophobic, aromatic). The fundamental disadvantage is, of course, that not every individual amino acid is tested and that small differences can have a major influence on the functionality. Glutamate, for example, is not simply interchangeable with aspartate as the shorter chain length might impede the carboxyl group to reach the substrate. In very specific circumstances, even narrower degeneracies can be considered based on structural or rational considerations. For example, there are degeneracies that put the focus on hydrophilic (VRK, 12 codons, 8 amino acids), hydrophobic (NYC, 8 codons, 8 amino acids), charged (RRK, 8 codons, 7 amino acids) or small (KST, 4 codons, 4 amino acids) residues. Alternatively, a mixture of degenerate primers can be employed to ensure complete coverage of amino acids with (almost) no redundancy. The so-called Tang design (“small-intelligent”) is based on a mixture of four primers, resulting in a zero probability for premature stop codons and a perfectly uniform distribution of 1/20 for each amino acid. In turn, the “22c-trick” uses just three primers that generate only two redundant codons (for valine and leucine) and no stop codons. The downside of these approaches is the additional cost of the supplementary primers, especially if performing CSM where the number of primers increases exponentially. In any case, not all primers (or even the individual codons within each primer) will necessarily anneal with the same efficiency, which could result in a skewed distribution. Therefore, the 3-12 quality of the library should always be checked by sequencing of the plasmid mixture before initiating the screening effort, to allow for further optimization (e.g. by changing the annealing temperature). Hotspot 1 22C NT1 NT2 Hotspot 2 NT3 12 Ta 9 1 The 3 primers are mixed in a ratio of 12/9/1 to generate the most optimal distribution at every nucleotide position. In practice, however, sequencing of the plasmid mixture often shows a skewed distribution in the chromatograms. 3-13 Finding hotspots by sequence analysis A popular strategy to more effectively navigate and identify regions of functionality in protein sequence space has been the use of evolutionary information. Multiple sequence alignment (MSA) has become a standard tool for the exploration of amino acid conservation and variation among a pool of evolutionarily related enzymes. MSA arranges multiple sequences in such a way that similar or identical characters (i.e. amino acids) are positioned together in the same column. To achieve this, gaps are inserted in the sequences. Many MSA programs exist, but the most popular one is probably Clustal Omega, which is integrated into the UniProt database website. An alignment enables the visual identification of positions that are fully conserved, meaning that they are occupied by the same amino acid in all included sequences. Wellconserved amino acids often have a crucial function for catalysis, substrate binding, folding or stability. Therefore, they can be attractive hotspots for engineering in certain cases. For example, conserved positions in binding sites can be great engineering targets when the goal is to change an enzyme's substrate preference. However, they may be better left untouched in other cases (e.g. when the goal is to design more thermostable enzymes without altering their function). A lot of valuable information can be obtained by using MSA to compare the sequences of homologous enzymes with different properties (function A versus function B, high thermostability versus low thermostability, strict versus promiscuous...). The similarities and differences in their sequences may also be linked to their structural or functional similarities and differences. An example of this was given in one of the exercises in chapter 1. To get a better visual overview of the variation among aligned sequences, a sequence logo can be created using online tools such as WebLogo (http://weblogo.threeplusone.com). Each logo consists of a stack of characters, one stack for each position in the sequence. The overall 3-14 height of the stack indicates the sequence conservation at that position, while the height of characters indicates the relative frequency of each amino acid at that position. By making a separate sequence logo for different groups of sequences, these groups can be compared with ease. Another sequence-based strategy that has gained traction in recent years is the analysis of correlated positions (or co-evolving positions). These are groups of positions that appear to mutate together throughout evolution, suggesting that only certain combinations of amino acids are viable at these positions, whereas the wrong combinations result in impaired stability, folding, catalytic activity or specificity. Those wrong combinations are filtered out by natural evolution, so they are not present in the sequences found in databases today. Because these positions seem to be important towards a protein's evolutionary fitness, it makes sense that they could be useful engineering hotspots as well. Correlated positions can be detected by statistical analysis of an alignment, and a few online tools are available to help protein engineers find these correlations easily (e.g. Comulator in 3DM). Analysis of correlation networks is especially useful for discovering important positions that are not easily found by looking at the structure (e.g. in the second shell of the active site), or for verifying whether a mutation at one hotspot may require a compensatory mutation at a different position. 3-15 One of the easiest and most popular approaches for sequence-based enzyme engineering is consensus engineering for obtaining more thermostable variants. It takes advantage of the purifying selection that nature has already applied to all sequences in the MSA. Indeed, destabilizing mutations are purged if the overall stability of the resulting protein falls below a certain threshold. As a result, residues that stabilize a protein tend to be more prevalent than other amino acids at any given position in a protein family. Therefore, it may be possible to stabilize a protein by replacing the residue at several (or even all) positions by the most common residue at that position, i.e. the consensus residue. For example, the full-length consensus sequence of phytase enzymes were found to have an unfolding temperature (Tm) that was 15°C higher than that of even the most thermostable 'parent' phytase. Finding hotspots by structural analysis 1. Protein structures An enzyme's function is usually tightly linked to its three-dimensional structure. Structures are more highly conserved than sequences throughout evolution: many enzymes share the same structure and function, despite a sequence identity as low as 30%. Furthermore, insight in the structure reveals where each residue in the amino acid chain is located, and which residues are in contact with the substrate or with each other. Protein structures can be obtained by a technique called X-ray crystallography. The process starts with the production of a concentrated and pure protein solution, which is then subjected to a wide variety of crystallization conditions by the addition of a range of precipitants. The experimental conditions must be set so that the protein solution gradually becomes supersaturated, resulting in slow but steady crystallization (days or weeks). Obtaining crystals is the least certain step in the process, because there is no way of knowing in advance which conditions may be successful, forcing scientists to rely on trial-and-error. Once crystals of a suitable size are obtained, they are bombarded with X-rays and the diffraction pattern is recorded. Computational methods exist that can interpret the diffraction pattern to infer the threedimensional positions of all atoms in the protein crystal, including the smaller molecules bound inside. All atomic detail structures are collected in a worldwide repository, the Protein Data Bank (PDB, www.rcsb.org). 3-16 Unfortunately, no experimentally determined structure is readily available for most enzymes. It is possible to build a so-called homology model using only the sequence of the target enzyme and the structure of a related homologous enzyme (the 'template'). First, a suitable template has to be found by searching through databases (UniProt, PDB). The identity between target and template should be at least ~30%, although higher values (> 50%) are required to obtain an accurate model. The template and target sequences are then aligned. Using this alignment and the template structure, the template structure can be inferred. Finally, the obtained model is refined by optimizing the amino acid side chain conformations and running energy minimization algorithms. Homology modeling can be done automatically using webservers like swiss-model (https://swissmodel.expasy.org) or modeling software like YASARA. Alternatively, it is possible to use an AlphaFold structure that is generated by a deep learning algorithm, trained on the entire PDB (see chapter 4). Looking at the three-dimensional structure in a molecular visualization program (e.g. PyMOL, YASARA), enzyme engineers most often use their chemical intuition to find relevant hotspots. However, countless computational tools have been developed in recent years to assist them in this undertaking. 2. Hotspots for activity, specificity, selectivity When the desired substrate was bound to the enzyme during crystallization, the direct enzyme-substrate interactions can easily be identified. When the available crystal structures have no ligand, bind a distant homologue of the desired substrate, or hold the ligand in a nonproductive conformation, finding substrate-specific mutational hotspots becomes far more challenging. In those cases, molecular docking can be performed to simulate the binding pose of a ligand within a binding pocket (e.g. AutoDock integrated in YASARA, see exercise in chapter 1). Docking is a standard technique that had enabled the successful prediction of hotspots countless times, but it does have evident shortcomings. Docking typically produces multiple output poses and finding the correct one is not always straightforward. Additionally, 3-17 enzymes may undergo significant structural modifications upon substrate binding, which sometimes makes it impossible for standard docking algorithms to find a correct pose. Crystal structures are static representations of enzymes that were obtained under one specific set of experimental conditions. In reality, enzymes are highly flexible macromolecules and dynamics can play a key role in their functionality, especially upon substrate binding (induced fit) and during catalysis. Crystal structures thus only provide a partial view of their 3D structure. Molecular dynamics (MD) simulations can model the movement of an enzyme in solution, offering insight into its other possible conformational states. They calculate the forces acting on every atom (bonds modeled as springs, van der Waals and electrostatic interactions between atoms,...) and use Newton's law of motion to calculate their accelerations. From these accelerations, their new velocity and position after a small timestep (~fs) can be calculated. The calculation cycle is repeated over and over, until the desired simulation time is reached. MD simulations are very helpful for unveiling modest structural rearrangements of the protein or the movement of a ligand. Sadly, today's personal computers (several ns per day) and high-performance computers (several µs) can barely scratch the time scales of many biological processes. Another aspect of enzyme structures that is often overlooked are the tunnels that connect the bulk solvent to the binding pockets. It may be possible to introduce or improve activity on a 3-18 certain substrate by altering not only the active site, but also the entrance tunnel that the substrate has to pass through. By mutating the amino acids in this tunnel, its shape, physicochemical properties and dynamics can be changed. A software tool called CAVER can visualize such tunnels and identify bottlenecks automatically. A few very simple tools have been developed that combine multiple kinds of structure-based (and sequence-based) information to make suggestions of mutational hotspots, requiring barely any input and knowledge from the user. Hotspot Wizard is a well-known example (https://loschmidt.chemi.muni.cz/hotspotwizard/). It quickly finds binding pockets or tunnels and determines which amino acids are located in these areas. Using information from a MSA of the target enzyme with homologous enzymes, HotSpot Wizard then divides these amino acids into groups that indicate low, moderate or high mutability. 3. Hotspots for stability Stability is an important parameter, which co-determines the economic feasibility of applying an enzyme in an industrial process. High stability is generally considered an economic advantage because of reduced enzyme turnover. In addition, stable enzymes permit the use of high process temperatures, which may have beneficial effects on reaction rates, reactant solubility and the risk of microbial contamination. Unsurprisingly, protein engineering methods have thus been applied to improve enzyme stability ever since enzymes have been used on large industrial scales. Two kinds of thermostability parameters can be distinguished. The first is thermodynamic stability. Folded proteins are in a thermodynamic equilibrium with their unfolded state, as determined by the difference in Gibbs free energy (ΔG) between those two states. The point where a protein's folded and unfolded states are present in equal amounts is called the melting temperature (Tm), which can be measured by differential scanning calorimetry (DSC). In a 3-19 DSC experiment, energy is introduced simultaneously into a sample cell (which contains the protein of interest) and a reference cell (containing only the solvent). Temperatures of both cells are raised identically over time. The difference in the input energy required to match the temperature of the sample to that of the reference is the amount of excess heat absorbed or released by the molecule in the sample. The process of protein unfolding involves the disruption of forces that stabilize the structure, which is associated with heat absorption and can thus be monitored by DSC. Although the sensitivity of DSC is very high, it requires a lot of protein and equipment that is not widely available. Alternatively, the Tm can be estimated by differential scanning fluorimetry (DSF). To do so, the protein is incubated together with a dye that is fluorescent when bound to hydrophobic amino acids which are more prevalent in the inside of a protein, while the temperature is gradually increased. In the resulting fluorescence curve, the melting temperature is found at the infliction point. There are, however, two problems with the measurement of thermodynamic stability in an enzyme engineering context. First of all, both DSC and DSF are difficult to apply as a high-throughput screening procedure because they cannot be performed accurately using cell lysates. Indeed, the presence of contaminating proteins would interfere with the measurements. Second, the concept of reversible thermodynamic unfolding is a rather theoretical one. Most proteins aggregate irreversibly after the initial reversible unfolding step. The second thermostability parameter is kinetic stability, which relates to the time it takes for a protein to denature irreversibly, due to aggregation or proteolysis after unfolding. This parameter is far more relevant in an industrial setting. The most commonly reported measure of an enzyme's kinetic stability is its half-life (t1/2) of denaturation, or the time it takes for the activity to be reduced by half at a defined temperature. Another measure is the temperature at which the activity is reduced by half after incubation for a defined amount of time (T50). Unfortunately, it is not easy to come up with a high-throughput screening procedure that can measure those parameters either. Most stabilizing mutations only cause slight improvements of the kinetic thermostability, while the error margins in a screening setup tend to be quite high 3-20 (e.g. due to evaporation of the reaction medium after prolonged incubation at elevated temperature). It is clear that stability engineering benefits a lot from a (semi-)rational approach where only a limited number of variants are expressed, purified and tested. The rational design of a mutation that will improve the ΔG of folding requires an understanding of the forces underlying the energy balances in the folded as well as the unfolded state. ΔG = ΔH – TΔS indicates that there are principally two ways to stabilize a protein, via ΔH (enthalpy) or ΔS (entropy). In reality, it is nearly impossible to decompose the effect of a mutation in ΔΔH or via ΔΔS terms by reasoning, but it is common practice to do so anyway. Just as with the hotspots for catalytic activity, hotspots for stability can be found by looking at the 3D structure and identifying mutations that may contribute to those terms. "Enthalpic" stabilization can be achieved by introducing salt bridges or hydrogen bonds, improving van der Waals interactions, and so on. An example of "entropic" stabilization is the addition of more rigid amino acids (e.g. proline) that reduce the entropy of the unfolded state. A well-known structure-based stabilization strategy that has seen a lot of success is B-Fit, where the amino acids with the highest B-factor are chosen as hotspots for iterative saturation mutagenesis. This B-factor is included for all atoms except hydrogen in every X-ray structure deposited in the PDB, and it reflects the degree of 'smearing' of electron densities with respect to their average position as a result of thermal motion. In other words, amino acid sidechains with higher B-factors are likely to be more flexible and dynamic. B-fit has been used to increase the half-life of a lipase at 50°C from just 2 minutes for the wild-type, to 16 hours for a variant with 5 mutations. Similarly, other researchers have used molecular dynamics simulations to identify flexible regions that can serve as hotspots for stability enhancements. 3-21 Another strategy that has achieved considerable success is the engineering of disulfide bridges. Disulfide bridges are covalent bonds between the sulfur atoms of the thiol (-SH) groups in two cysteine residues. Upon oxidation, the thiols form a disulfide (S-S) that links the two cysteines and their respective main peptide chains together. They are found predominantly in secreted extracellular proteins, because the redox environment within the cytosol preserves cysteine sulfhydryls in a reduced state. The bonds are formed outside the cell in the presence of oxygen, or in the oxidizing environment of the endoplasmatic reticulum in eukaryotic cells. They thermodynamically stabilize proteins by lowering the entropy of the unfolded state, and bridges that impede the early stages of the unfolding process can drastically contribute to kinetic stability as well. By introducing cysteines at suitable positions in the structure, novel crosslinks can rationally be created. Computational tools have been created that can assess whether the proximity and geometry of a residue pair would be appropriate for disulfide formation after mutation to cysteine (eg. Disulfide by Design). However, not all disulfide bridges increase stability. Stabilizing bridges tend to be located (1) in or between flexible regions, (2) near the protein surface, and/or (3) associated with long loops of > 25 residues; and (4) they should not cause steric hindrance for surrounding amino acids. Given the relevance of flexibility in the design process, disulfide engineering is frequently coupled with analysis of B-factors or molecular dynamics simulations. R1-SH + R2-SH ⇌ R1-S-S-R2 + 2 H+ + 2 eA few computational tools have been developed to predict how a mutation influences ΔG by estimating its impact on several relevant terms such as rigidity (entropy), intramolecular interactions, interactions between the protein and the solvent (water), and so on. FoldX is a popular example, as it is quite easy to use and free for academic purposes (http://foldxsuite.crg.eu/products). Another example is PoPMuSiC, which can conveniently be run from a webserver (https://soft.dezyme.com). A more difficult, but more accurate alternative is Rosetta ddG. With these tools, the (de)stabilizing effect of all possible point mutations of an entire enzyme can be computed in a matter of hours. Only the crystal structure is required as input. It is important to note that although the mutations suggested by FoldX, PoPMuSiC and Rosetta ddG have a much higher success rate than random mutations, these programs should not be seen as a gold-standard for improving thermostability. For example, the performance of FoldX depends drastically on the quality of the used crystal structure, and it seems to be more accurate for the prediction of destabilizing mutations than for the prediction of stabilizing ones. 3-22 The highest hit rates in rational enzyme stabilization seem to be achieved by combining several of the strategies above. One of these hybrid methods is a webserver called PROSS (Protein Repair One-Stop Shop; http://pross.weizmann.ac.il). It first does an evolution-based sequence analysis by ruling out amino acids that are rarely observed in homologs of the target enzyme. Then, Rosetta is used to scan all amino acids that passed the first filter to eliminate the single-point mutations that may destabilize the enzyme. These two steps result in a reduced sequence space, in which all possible point mutations are predicted to be stabilizing. Finally, Rosetta designs optimal combinations of mutations from this reduced sequence space, taking into account the interactions between mutated and unmutated positions. Furthermore, the algorithm is slightly biased towards the consensus residue at each position, thereby including yet another rational stabilization aspect into the process. PROSS has been used to design thermostable variants of the human acetylchlorinesterase. A variant bearing no less than 51 mutations had a 20°C higher thermostability without damaging any other enzymatic properties. A second hybrid method is FireProt, which also combines structurebased filters (FoldX and Rosetta) with evolution-based ones (conservation and correlation). 3-23 Illustrations Examples of epistasis in semi-rational design unveiled by deconvolution 1) Inversion of enantioselectivity An esterase from Bacillus subtilis was discovered that shows activity towards the acetates of tertiary alcohols. When this wild-type esterase is used for kinetic resolution of racemic 1, it shows an enantioselectivity of ER = 42. Semi-rational engineering was done to invert its enantioselectivity. Three positions, all situated near the catalytic triad and pointing towards the active site cavity, were selected for CSM using NNK codons. After screening a few thousand clones on both (S)-1 and (R)-1 using a spectrophotometric assay that detects the release of acetate, the best variant turned out to be the double-mutant E188W/M183C, with an enantioselectivity of ES = 64. Next, the corresponding single mutants were created to identify which mutation contributed the most to the substantial inversion in selectivity. Surprisingly, M193C still preferred the R enantiomer (ER = 16) with similar activity, while E188W showed only modest S enantioselectivity (ES = 26) and very low activity. Thus, only simultaneous saturation mutagenesis allowed the creation of an esterase variant with inverted enantioselectivity and synthetically useful activity. If the researchers had chosen for traditional saturation mutagenesis, or iterative saturation mutagenesis starting from library M193X, it is unlikely that the M193C mutation would have been retained. 3-24 2) Visualization of the fitness landscape of an ISM experiment The group of Manfred Reetz drastically increased the enantioselectivity of an epoxide hydrolase from E = 5 to E = 115 by iterative saturation mutagenesis. Five sets of positions (named A, B, C, D, E) were randomized one by one, with the best hit of each round becoming the template for the next round. Then, they addressed the following question: is A → B → C → D → E the only ISM pathway that could lead to that particular hit, or could these mutations have been obtained in a different order too? To do so, they created and evaluated all possible 'intermediate stages' between the wild-type enzyme and the final variant with mutations at all 5 sites (there are 25 = 32 possible permutations). Using the obtained data, they could then map all of the theoretically possible 5! = 120 pathways leading from the wild-type to the final variant. About half of the pathways were favorable, whereas the others contain at least one local minimum which would have been interpreted as a dead-end in an iterative screening experiment. These results show that (1) ISM can follow many pathways to improved enzymes, (2) different combinations of mutations lead to different non-additive (cooperative or antagonistic) effects, and (3) it is possible to escape dead ends in the fitness landscape by returning to the previous stage in the iterative process, and choosing a different set of mutations to put the evolutionary process back on a positive track. 3-25 Enzyme stability can be engineered by modification of the access tunnel Koudelakova 2013 - Engineering Enzyme Stability and Resistance to an Organic Cosolvent by Modification of Residues in the Access Tunnel The stability of enzymes can be lowered dramatically by the presence of an organic solvent in the reaction mixture. For example, Jiri Damborsky et al. noticed that addition of 40% dimethylsulfoxide (DMSO) decreases the initial catalytic rate of haloalkane dehydrogenase (Dha) tenfold and causes denaturation at 37°C, despite a melting temperature of 50°C in aqueous medium. Dha was mutated by error-prone PCR and ~5,000 clones were screened by colorimetrically monitoring reactions in the presence of 42% DMSO. One of the hits exhibited significanty improved stability while keeping a similar level of catalytic efficiency. This effect was caused by just one mutation in the access tunnel. This finding prompted them to take a closer look at other stable variants of Dha with multiple mutations that had been reported in earlier work. Again, their improved stability was primarily caused by the substitutions that were located in the tunnel region. The crystal structures of Dha and its variants were determined, showing that the bulkier and mostly hydrophobic side chains of introduced substitutions caused a tighter packing of the amino acids in the tunnel. Molecular dynamics simulations with DMSO were carried out, revealing that the mutations shield the active site from the solvent molecules. To further validate the general applicability of the tunnel engineering concept, the stability effects of all possible single point mutations in 26 different proteins were analyzed computationally using FoldX. According to the predictions, a highly stabilizing mutation exists for ~10% of tunnel residues, compared to only ~5% of the residues in other regions. This implies that saturation mutagenesis targeting tunnel residues in enzymes with a buried active site is more likely to produce variants with improved stability than mutagenesis targeting other regions. The study illustrates how an analysis of hits obtained by random directed evolution may eventually lead to a better understanding of the molecular basis of changes to protein properties, which in turn opens up new opportunities for (semi)rational engineering. 3-26