Business Statistics Chapter 12 PDF
Document Details
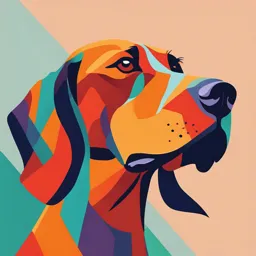
Uploaded by PleasedObsidian8581
2020
Ken Black
Tags
Summary
This document is chapter 12 of a business statistics textbook titled 'Business Statistics' by Ken Black, tenth edition. The chapter covers simple regression analysis and correlation, including concepts, calculations, and examples.
Full Transcript
Business Statistics Tenth Edition Ken Black Chapter 12 Simple Regression Analysis and Correlation Copyright ©2020 John Wiley & Sons, Inc. Learning Objectives (1 of 2) 1. Calculate the Pearson product-moment correlation coefficient to dete...
Business Statistics Tenth Edition Ken Black Chapter 12 Simple Regression Analysis and Correlation Copyright ©2020 John Wiley & Sons, Inc. Learning Objectives (1 of 2) 1. Calculate the Pearson product-moment correlation coefficient to determine if there is a correlation between two variables. 2. Explain what regression analysis is and the concepts of independent and dependent variables. 3. Calculate the slope and y-intercept of the least squares equation of a regression line and from those, determine the equation of the regression line. 4. Calculate the residuals of a regression line and from those determine the fit of the model, locate outliers, and test the assumptions of the regression model. 5. Calculate the standard error of the estimate using the sum of squares of error, and use the standard error of the estimate to determine the fit of the model. Copyright ©2020 John Wiley & Sons, Inc. 2 Learning Objectives (2 of 2) 6. Calculate the coefficient of determination to measure the fit for regression models, and relate it to the coefficient of correlation. 7. Use the t and F tests to test hypotheses for both the slope of the regression model and the overall regression model. 8. Calculate confidence intervals to estimate the conditional mean of the dependent variable and prediction intervals to estimate a single value of the dependent variable. 9. Determine the equation of the trend line to forecast outcomes for time periods in the future, using alternate coding for time periods if necessary. 10. Use a computer to develop a regression analysis, and interpret the output that is associated with it. Copyright ©2020 John Wiley & Sons, Inc. 3 12.1 Correlation (1 of 4) Correlation: a measure of the degree of relatedness of variables Do the stocks of two airlines rise and fall in any related manner? How strong is the correlation between the producer price index and the unemployment rate? Are sales related to population density? Pearson Product-Moment Correlation Coefficient ( x )( y ) ( x − x )( y − y ) xy − n r= = ( x − x )2 ( y − y )2 x − 2 ( x) 2 y − 2 ( y) 2 n n Measure of the linear relationship between variables: o r = 0 means that there is no linear relationship between the variables o r = +1 means that there is perfect positive correlation o r = −1 means that there is perfect negative correlation Copyright ©2020 John Wiley & Sons, Inc. 4 12.1 Correlation (2 of 4) Copyright ©2020 John Wiley & Sons, Inc. 5 12.1 Correlation (3 of 4) TABLE 12.2: Computation of r for the Economics Example Example: What is the Day Interest Rate x Futures Index y x2 y2 xy measure of correlation 1 7.43 221 55.205 48,841 1,642.03 between the interest rate of 2 7.48 222 55.950 49,284 1,660.56 federal funds and the commodities futures index? 3 8.00 226 64.000 51,076 1,808.00 4 7.75 225 60.063 50,625 1,743.75 It does not matter in 5 7.60 224 57.760 50,176 1,702.40 calculation which 6 7.63 223 58.217 49,729 1,701.49 variable is x or y 7 7.68 223 58.982 49,729 1,712.64 For this example, r = 8 7.67 226 58.829 51,076 1,733.42.815, which shows a 9 7.59 226 57.608 51,076 1,715.34 high degree of 10 8.07 235 65.125 55,225 1,896.45 correlation between the 11 8.03 233 64.481 54,289 1,870.99 fed funds rate and the 12 8.00 241 64.000 58,081 1,928.00 futures index over this 12-day period Σx = 92.93 Σy = 2,725 Σx2 = 720.220 Σy2 = 619,207 Σxy = 21,115.07 (92.93)(27.25) Correlation does not (21,115.07) − r= 12 =.815 imply causation (92.93) 2 (2725) 2 (720.22) − (619.207) − 12 12 Copyright ©2020 John Wiley & Sons, Inc. 6 12.1 Correlation (4 of 4) Excel or Minitab can be used to calculate the correlation coefficient Minitab also gives the p-value for significance of the correlation coefficient Copyright ©2020 John Wiley & Sons, Inc. 7 Quick recap regarding testing Ho: r=0 H1: r0 p=0.001 (previous slide) p>0.05 => Do not reject Ho, e.g., no relation p Reject Ho, e.g., there is a relation Copyright ©2020 John Wiley & Sons, Inc. 8 12.2 Introduction to Simple Regression Analysis (1 of 3) Regression analysis: the process of constructing a mathematical model or function that can be used to predict or determine one variable by another variable or other variables The most elementary regression model is called simple regression or bivariate regression Two variables; one variable is predicted by another variable o Dependent variable: the variable to be predicted; designated as y o Independent variable: the predictor, or explanatory, variable; designated as x In simple regression analysis, only a straight-line relationship between two variables is examined Copyright ©2020 John Wiley & Sons, Inc. 9 12.2 Introduction to Simple Regression Analysis (2 of 3) TABLE 12.3: Airline Cost Data Example: Can the cost of flying a commercial airliner be predicted using Number of Passengers COST ($1,000) regression analysis? 61 4.280 63 4.080 Many variables are related to cost 67 4.420 One possible variable is number of 69 4.170 passengers, which is related to the 70 4.480 flying weight of the plane 74 4.300 The costs and associated number of 76 4.820 passengers for twelve 500-mile 81 4.700 commercial airline flights using 86 5.110 Boeing 737s during the same season of 91 5.130 the year are collected 95 5.640 Next step is to make a scatter plot of 97 5.560 the data Copyright ©2020 John Wiley & Sons, Inc. 10 12.2 Introduction to Simple Regression Analysis (3 of 3) The scatter plot shows a positive, linear relationship Data also appears to “fit” well around a line, as shown in the close-up plot Since the relationship appears linear, the next step is to estimate the regression line Copyright ©2020 John Wiley & Sons, Inc. 11 12.3 Determining the Equation of the Regression Line (1 of 4) Equation of the Simple Regression Line Where ŷ = 0 + 1 x ŷ = the predicted value of y b0 = the sample y intercept b1 = the sample slope For any specific dependent variable value, yi , yi = 0 + 1 x1 + i Unless the points being fitted by the regression equation are in perfect alignment, the regression line will miss at least some of these points εi is the error of the regression line in fitting these points β0, β1 are the underlying values of the population (probabilistic) model; in practice, we observe the sample (deterministic) values, 𝑏0 , 𝑏1 Copyright ©2020 John Wiley & Sons, Inc. 12 12.3 Determining the Equation of the Regression Line (2 of 4) The analyst must determine the values for 𝑏0 , 𝑏1 Least squares analysis: a process whereby a regression model is developed by producing the minimum sum of the squared error values Slope of the Regression Line ( x)( y ) xy − ( x − x )( y − y ) xy − nx y n b1 = = = ( x − x ) 2 x − nx 2 2 2 ( x) 2 x − n The expression in the numerator, ( x − x )( y − y ), is denoted as S xy The expression in the denominator, ( x − x ) 2 , is denoted as S xx SS xy Thus the slope can also be written as b1 = SS xx Copyright ©2020 John Wiley & Sons, Inc. 13 12.3 Determining the Equation of the Regression Line (3 of 4) The slope of the Number of Passengers Cost ($1,000) regression line must be x y x2 xy calculated before the 61 4.280 3,721 261.080 intercept 63 4.080 3,969 257.040 67 4.420 4,489 296.140 y Intercept of the 69 4.170 4,761 287.730 Regression Line 70 4.480 4,900 313.600 b0 = y − b1 x = y − b1 ( x) 74 4.300 5,476 318.200 76 4.820 5,776 366.320 n n 81 4.700 6,561 380.700 For the airplane data, 86 5.110 7,396 439.460 91 5.130 8,281 466.830 first the sums of 95 5.640 9,025 535.800 squares are calculated 97 5.560 9,409 539.320 Σ x = 930 Σ y = 56.690 Σ x2 = 73,764 Σ xy = 4462.220 Copyright ©2020 John Wiley & Sons, Inc. 14 12.3 Determining the Equation of the Regression Line (4 of 4) Then the regression slope and intercept can be calculated SS xy = xy − ( x )( y ) = 4462.22 − (930)(56.69) = 68.745 n 12 SS xx = x 2 − ( x) 2 = 73, 764 − (930) 2 = 1689 n 12 SS xy 68.745 b1 = = =.0407 SS xx 1689 y x 56.69 930 b0 = − b1 = − (.0407) = 1.57 n n 12 12 yˆ = 1.57 +.0407 x The slope of this regression line is.0407 o Because the y values are in $1,000 denominations, the slope is really $40.70 o One interpretation of the slope in this problem is that for every unit increase in x (every person added to the flight of the airplane), there is a $40.70 increase in the cost of the flight One interpretation of the y-intercept, which is 1.570 or $1,570, is that even if there were no passengers on the flight, it would still cost $1,570 to fly the plane for the given distance Copyright ©2020 John Wiley & Sons, Inc. 15 12.4 Residual Analysis (1 of 7) How can the analyst determine whether the regression line is a good fit? One method is to use TABLE 12.5: Predicted Values and Residuals for the Airline Cost Example the values of the x Number of Passengers Cost ( $1, 000 ) Predicted Value Residual variable to predict the x y yˆ y − yˆ y values 61 4.280 4.053.227 Residual: each 63 4.080 4.134 −.054 difference between the actual y values and the 67 4.420 4.297.123 predicted y values; the 69 4.170 4.378 −.208 error of the regression 70 4.480 4.419.061 line at a given point, 74 4.300 4.582 −.282 y − yˆ 76 4.820 4.663.157 81 4.700 4.867 −.167 The least squares 86 5.110 5.070.040 regression line 91 5.130 5.274 −.144 minimizes the sum 95 5.640 5.436.204 of squared residuals 97 5.560 5.518.042 ( y − yˆ ) = −.001 Copyright ©2020 John Wiley & Sons, Inc. 16 12.4 Residual Analysis (2 of 7) The scatter plot below shows the residuals relative to the regression line Copyright ©2020 John Wiley & Sons, Inc. 17 12.4 Residual Analysis (3 of 7) Using Residuals to Test the Assumptions of the Regression Model Regression analysis makes the following four assumptions about the regression model: 1. The model is linear 2. The error terms have constant variances 3. The error terms are independent 4. The error terms are normally distributed Residual plot: a type of graph in which the residuals for a particular regression model are plotted along with their associated value of x as an ordered pair, ( x, y − yˆ ) Copyright ©2020 John Wiley & Sons, Inc. 18 12.4 Residual Analysis (4 of 7) This plot suggests that the model is not linear Figure 12.9 Nonlinear Residual Plot These plots suggest that the errors do not have a constant variance Figure 12.10 Nonconstant Error Variance These plots suggest that the errors are not independent Figure 12.11 Graphs of Nonindependent Error Terms Copyright ©2020 John Wiley & Sons, Inc. 19 12.4 Residual Analysis (5 of 7) For the airline example, the residual plot appears healthy There are no particular patterns shown by the residual plot Figure 12.8 Excel Graph of Residuals for the Airline Cost Example Copyright ©2020 John Wiley & Sons, Inc. 20 12.4 Residual Analysis (6 of 7) Using the Computer for Residual Analysis Minitab’s residual graphic analyses for a regression model developed to predict the production of carrots in the U.S. per month by the total production of sweet corn The graph on the upper right is a plot of the residuals versus the fits o Note that this residual plot “flares out” as x gets larger, showing a variance that is not constant The graph in the upper left is a normal probability plot of the residuals o A straight line indicates that the residuals are normally distributed Copyright ©2020 John Wiley & Sons, Inc. 21 12.4 Residual Analysis (7 of 7) Using the Computer for Residual Analysis Minitab’s residual graphic analyses for a regression model developed to predict the production of carrots in the U.S. per month by the total production of sweet corn The normal distribution is confirmed by the graph on the lower left, which is a histogram of the residuals (shown here) Copyright ©2020 John Wiley & Sons, Inc. 22 12.5 Standard Error of the Estimate (1 of 2) The standard error of the estimate provides a single estimate of the regression error SSE Se = n−2 where SSE, the sum of squares error, is SSE = ( y − yˆ ) 2 = y 2 − b0 y − b1 xy The SSE is the sum of the squared residuals o Minimized by the least squares regression process The standard deviation of the error of the regression model o Can be used to create confidence intervals o Can be used to identify outliers Copyright ©2020 John Wiley & Sons, Inc. 23 12.5 Standard Error of the Estimate (2 of 2) For the airline TABLE 12.6: Determining SSE for the Airline Cost Example example, Table 12.6 Number of Passengers Cost ( $1, 000 ) Residual ( y − yˆ ) 2 shows the calculation x y y − yˆ of the SSE 61 4.280.227.05153 63 4.080 −.054.00292 The standard error of 67 4.420.123.01513 the estimate is: 69 4.170 −.208.04326 70 4.480.061.00372 SSE.31434 −.282 Se = = =.1773 74 4.300.07952 n−2 10 76 4.820.157.02465 81 4.700 −.167.02789 86 5.110.040.00160 91 5.130 −.144.02074 95 5.640.204.04162 97 5.560.042.00176 ( y − yˆ ) = −.001 ( y − yˆ ) 2 =.31434 Copyright ©2020 John Wiley & Sons, Inc. 24 12.6 Coefficient of Determination (1 of 2) Coefficient of determination (r2): the proportion of variability of the dependent variable (y) accounted for or explained by the independent variable (x) SSE SSE b12 SS xx r2 = 1− = 1− = 2 ( y) 2 SS yy SS yy y − n 0 r2 1 (.0407016) 2 (1689) For the airline example, r = 2 =.899 3.11209 Almost 90% of the variation in cost can be explained by the number of passengers Copyright ©2020 John Wiley & Sons, Inc. 25 12.6 Coefficient of Determination (2 of 2) Relationship Between r and r2 The coefficient of determination is equal to the correlation coefficient (r) squared Thus the correlation coefficient can be found by taking the square root of the coefficient of determination, but the sign will not be right if there is a negative correlation o Slope of regression line shows whether there is a positive or negative correlation Copyright ©2020 John Wiley & Sons, Inc. 26 12.7 Hypothesis Tests for the Slope of the Regression Model and Testing the Overall Model (1 of 5) Testing the Slope Test to determine whether the slope coefficient is significantly different from zero o Does the x variable add value to the prediction of y? Step 1: H0: β1 = 0 Ha: β1 ≠ 0 Figure 12.14 t Test of Slope from Airline Cost Example Copyright ©2020 John Wiley & Sons, Inc. 27 12.7 Hypothesis Tests for the Slope of the Regression Model and Testing the Overall Model (2 of 5) Step 2: t Test of Slope b1 − 1 t= sb se sb = SS xx SSE se = n−2 where ( x ) 2 β1 = the hypothesized SS xx = x2 − n slope df = n − 2 Copyright ©2020 John Wiley & Sons, Inc. 28 12.7 Hypothesis Tests for the Slope of the Regression Model and Testing the Overall Model (3 of 5) Step 3: Let α =.05 Step 4: For a two tailed test with α =.05 and n − 2 = 12 − 2 = 10 df, the critical t value is 2.228 Steps 5 and 6: Using the data from the airline cost table, b1 − 1.0407 − 0 t= = = 9.43 sb.1773 (930) 2 73764 − 12 Steps 7 and 8: Conclude that the slope is significantly different from zero, since 9.43 > 2.228 Thus knowing number of passengers helps to explain the cost of a flight Copyright ©2020 John Wiley & Sons, Inc. 29 12.7 Hypothesis Tests for the Slope of the Regression Model and Testing the Overall Model (4 of 5) Testing the Overall Model F test for the overall significance of the model (as in ANOVA) o With a single x variable, the F test and the t test for the slope are testing the same thing o Same hypothesis as for the t test o For a single independent variable, F = t 2 o For the airline cost example, F = (9.43) 2 = 88.92 o The critical value for F.025,1,10 = 4.96 Numerator df = k = 1, the number of independent variables, and denominator df = n − k − 1 = 10 o Conclude that the overall model has explanatory power Copyright ©2020 John Wiley & Sons, Inc. 30 12.7 Hypothesis Tests for the Slope of the Regression Model and Testing the Overall Model (5 of 5) General version of the F test SSreg df reg MSreg F= = SSerr MSerr df err where dfreg = k = the number of independent variables dferr = n − k − 1 Values for the F test can be found in the ANOVA table from Minitab or Excel Copyright ©2020 John Wiley & Sons, Inc. 31 12.9 Using Regression to Develop a Forecasting Trend Line (1 of 7) Business analysts often use historical data with measures taken over time in an effort to forecast what might happen in the future, using time-series data, defined as data gathered on a particular characteristic over a period of time at regular intervals Time-series data are likely to contain any one or combination of four elements: trend, cyclicality, seasonality, and irregularity Cyclicality, seasonality, and irregularity are examined in Chapter 15 Trend: the long-term general direction of data Copyright ©2020 John Wiley & Sons, Inc. 32 12.9 Using Regression to Develop a Forecasting Trend Line (2 of 7) Determining the Equation of the Trend Line TABLE 12.8: Ten-Year Sales Data for Huntsville Chemicals As an example, consider the time-series Year Sales ($ millions) sales data over a 10-year time period 2010 7.84 for the Huntsville Chemicals Company 2011 12.26 The measurements (sales) are taken 2012 13.11 over time and that the sales figures 2013 15.78 are given on a yearly basis 2014 21.29 2015 25.68 A linear trend line in forecasting is a 2016 23.80 special case of simple regression 2017 26.43 where the y or dependent variable is 2018 29.16 the variable of interest 2019 33.06 Copyright ©2020 John Wiley & Sons, Inc. 33 12.9 Using Regression to Develop a Forecasting Trend Line (3 of 7) First, the regression equation is calculated using the usual methods TABLE 12.9: Determining the Year x Sales y x2 xy Equation of the Trend Line for the Huntsville Chemicals Company 2010 7.84 4,040,100 15,758.40 Sales Data 2011 12.26 4,044,121 24,654.86 ( x )( y ) (20,145)(208.41) 2012 13.11 4,048,144 26,377.32 xy − n 420,062.11 − 10 2013 15.78 4,052,169 31,765.14 b1 = = ( x) 2 (20,115) 2 40, 461, 405 − x2 − n 10 2014 21.29 4,056,196 42,876.06 220.165 2015 25.68 4,060,225 51,745.20 = = 2.6687 82.5 2016 23.80 4,064,256 47,980.80 2017 26.43 4,068,289 53,309.31 b0 = y − b x = 208.41 − (2.6687) 20,145 = −5,355.26 n 1 n 10 10 2018 29.16 4,072,234 58,844.88 2019 33.06 4,076,361 66,748.14 Σx = 20,145 Σy = 208.41 Σx2 = 40,582,185 Σxy = 420,062.11 Equation of the Trend Line: yˆ = −5,355.26 + 2.6687 x Copyright ©2020 John Wiley & Sons, Inc. 34 12.9 Using Regression to Develop a Forecasting Trend Line (4 of 7) The regression equation is ŷ = −5,355.26 + 2.6687 x The slope, 2.6687, means that for every yearly increase in time, sales increases by an average of $2.6687 (million) The intercept would represent company sales in the year 0, which in this problem has no meaning The r2 value is.963; high values are typical of data with a strong trend Copyright ©2020 John Wiley & Sons, Inc. 35 12.9 Using Regression to Develop a Forecasting Trend Line (5 of 7) The graph shows the data and the fitted trend line Copyright ©2020 John Wiley & Sons, Inc. 36 12.9 Using Regression to Develop a Forecasting Trend Line (6 of 7) Forecasting Using the Equation of the Trend Line The company wishes to predict sales in 2022 ŷ (2022) = −5,355.26 + 2.6687(2022) = 40.85 Recall that extrapolating outside the original time frame can be inaccurate Common in forecasting Copyright ©2020 John Wiley & Sons, Inc. 37 12.9 Using Regression to Develop a Forecasting Trend Line (7 of 7) Alternate Coding for Time Periods Using dates, such as 2010-2019, can get large and hard to manually calculate Recoding the data, such as replacing 2010-2019 with 1-10, gives a different intercept but the same slope ŷ (13) = 6.1632 + 2.6687(13) = 40.86, which is the same forecast as previously calculated Copyright ©2020 John Wiley & Sons, Inc. 38 12.10 Interpreting the Output (1 of 2) Although manual computations can be done, most regression problems are analyzed by using a computer Copyright ©2020 John Wiley & Sons, Inc. 39 Quick recap regarding testing Ho: beta1=0 H1: beta10 p=0.000 (previous slide) p>0.05 => Do not reject Ho, e.g., no relation p Reject Ho, e.g., there is a relation Copyright ©2020 John Wiley & Sons, Inc. 40 12.10 Interpreting the Output (2 of 2) Copyright ©2020 John Wiley & Sons, Inc. 41