Case Study 5 - Statistics II IB Past Paper PDF
Document Details
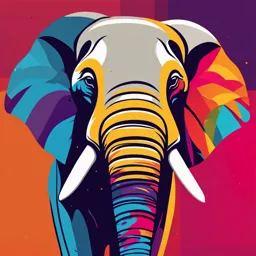
Uploaded by FervidHeliotrope4831
University of Groningen
2024
IB
Tags
Summary
This IB past paper provides a case study on time series analysis using unemployment data (1991-2014). The paper examines moving averages, exponential smoothing, autocorrelation (using Durbin-Watson tests, ACF, and PACF charts), and autoregressive models (AR(1)) to make forecasts and conclusions regarding trends in unemployment.
Full Transcript
Statistics II for IB (24/25) Case Study 5 1 Learning goals Case study 5 › Time series Moving average and exponential smoothing Autocorrelation Autoregressive model › How do we interpret Stata output?...
Statistics II for IB (24/25) Case Study 5 1 Learning goals Case study 5 › Time series Moving average and exponential smoothing Autocorrelation Autoregressive model › How do we interpret Stata output? 2 The data Source: http://databank.worldbank.org/ Data: Unemployment Year: 1991 - 2014 Available files on Nestor about this Case Study: Questions Data Do-file Log-file 3 1. Create a line chart with time on the horizontal and unemployment on the vertical axis. Do any visible tendencies occur? Can we suspect autocorrelation? If so, does it look more like negative or positive? Tendency? Autocorrelation? 4 2. Calculate central moving average of the variable “unemployment” with a span of 3 years. Create a line chart with the result with time on the horizontal axis. Compare it with the previous line chart: does the moving average provide a more or less hectic graph? Why? year observed value moving average Example: Moving Average 1 5 14 13 2 9 9 12 3 13 10 10 10 10 9 4 8 8 8 8 7 Value 5 3 7 6 observed value 6 5 5 moving average 6 10 6 4 3 7 5 2 0 1 2 3 4 5 6 7 Year 5 2. Central moving average Is the moving average more or less hectic? Why? 6 3. Use exponential smoothing to find forecasts based on simple exponential smoothing. What is the estimated α parameter? What’s the estimated α and what is the model in this case? 3. Use exponential smoothing to find forecasts based on simple exponential smoothing. What is the estimated α parameter? Is the exponential smooth line more or less hectic? Why? 4. Regress the variable “unemployment” on “time”. Check the Durbin-Watson test statistics. Using Table 12 in the book can we infer that the variable “unemployment” exhibits autocorrelation? If so, is it positive or negative? (In case the test is inconclusive what sign is more likely?) 4. Durbin-Watson test Durbin-Watson test statistic Cutoff Points: book, Test uncertain Test uncertain Table 12 ρ>0 ρ=0 ρ F = 0.0001 Residual 7.05849045 21.336118593 R-squared = 0.5223 Adj R-squared = 0.4996 Total 14.7763364 22.671651654 Root MSE =.57976 unemployment Coefficient Std. err. t P>|t| [95% conf. interval] lag_unemployment.7243602.1511655 4.79 0.000.4099942 1.038726 _cons 2.548866 1.413863 1.80 0.086 -.3914235 5.489156. estat dwatson Durbin–Watson d-statistic( 2, 23) = 1.568858 Cutoff Test uncertain Test uncertain Points: book, Table 12 ρ>0 ρ=0 ρ chi2 = 0.0000 OPG unemployment Coefficient std. err. z P>|z| [95% conf. interval] unemployment _cons 9.294433.4544244 20.45 0.000 8.403778 10.18509 ARMA ar L1..6979112.1224283 5.70 0.000.4579563.9378662 /sigma.5433993.0837791 6.49 0.000.3791952.7076034 Note: The test of the variance against zero is one sided, and the two-sided confidence interval is truncated at zero. Is the first lag significant? 19 8. Correlograms Correlograms of the residuals Is it justified to include a higher order lag variable? 20 8. Correlograms.. corrgram residuals -1 0 1 -1 0 1 LAG AC PAC Q Prob>Q [Autocorrelation] [Partial autocor] 1 0.1635 0.1675.72521 0.3944 2 -0.1705 -0.2151 1.5499 0.4607 3 0.0555 0.1810 1.6416 0.6500 4 0.0298 -0.0750 1.6694 0.7963 5 -0.0318 0.0400 1.7025 0.8886 6 0.0529 0.0337 1.7994 0.9372 7 -0.0157 0.0269 1.8085 0.9697 8 -0.1231 -0.2038 2.3991 0.9663 9 -0.1798 -0.2632 3.7439 0.9274 10 -0.1061 -0.8488 4.246 0.9356 Does the AR(1) process provide an adequate fit in overall? Correlograms of the residuals Is it justified to include a higher order lag variable? Conclusions › Time series Moving average and Exponential smoothing Autocorrelation: DW test, ACF and PACF Autoregressive model: AR(1) process 22