Brownspeed Health Care Employee Retention PDF
Document Details
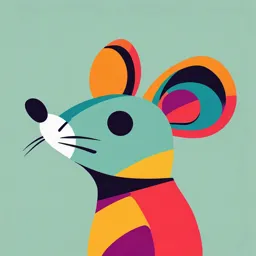
Uploaded by JudiciousDetroit6938
IIM Ahmedabad
2020
Owen Hall Jr
Tags
Summary
This case study examines the challenges of employee retention in the US healthcare industry. Brownspeed Health Care (BHC) faces high employee turnover and seeks solutions, focusing on compensation and cost of living adjustments. The case highlights the importance of using data and analytics for effective business strategies.
Full Transcript
W20467 BROWNSPEED HEALTH CARE: EMPLOYEE RETENTION USING PREDICTIVE ANALYTICS Owen Hall Jr. wrote this case solely to provide material for class discussion. The author does not intend to illustrate either effective or ineffective handling of a man...
W20467 BROWNSPEED HEALTH CARE: EMPLOYEE RETENTION USING PREDICTIVE ANALYTICS Owen Hall Jr. wrote this case solely to provide material for class discussion. The author does not intend to illustrate either effective or ineffective handling of a managerial situation. The author may have disguised certain names and other identifying information to protect confidentiality. This publication may not be transmitted, photocopied, digitized, or otherwise reproduced in any form or by any means without the permission of the copyright holder. Reproduction of this material is not covered under authorization by any reproduction rights organization. To order copies or request permission to reproduce materials, contact Ivey Publishing, Ivey Business School, Western University, London, Ontario, Canada, N6G 0N1; (t) 519.661.3208; (e) [email protected]; www.iveycases.com. Our goal is to publish materials of the highest quality; submit any errata to [email protected]. i1v2e5y5pubs Copyright © 2020, Ivey Business School Foundation Version: 2020-05-29 In October 2019, Mary Tadwell, the chief executive officer of Brownspeed Health Care (BHC), opened the annual meeting of the board of directors at the company’s headquarters in Orlando, Florida. She started the meeting with the main challenge affecting the entire US health care industry: employee turnover. Curbing high employee turnover rates had become the top priority for BHC’s clients. Tadwell turned to Johnny Stonebrook, BHC’s chief analytical officer, to provide some details on the issue. Stonebrook stated that according to his research, the health care sector had reached nearly full employment. In fact, the number of unfilled requisitions across most employment categories was increasing. Current employee turnover trends could be attributed to the recent booming economy and continued social and political turbulence, as witnessed in the nation’s ongoing national debate on the future of the US health care system. Stonebrook identified compensation as one critical factor contributing to employee turnover. He suggested that analyzing the rise in the country’s cost of living (COL) might be a good place to start developing an overall employee retention plan for all BHC clients. A successful solution for BHC clients would mean new opportunities and revenues for BHC. As Stonebrook concluded his talk, Tadwell directed him to pursue the plan and present his recommendations within two weeks. HEALTH CARE INDUSTRY EMPLOYEE RETENTION Domestic spending in the US health care industry was projected to reach over 19 per cent of the country’s gross domestic product within the next five years. Over that same period, global spending on health care was projected to rise to approximately $10 trillion, for an annual growth rate of over 5 per cent.1 These trends would continue to create more health care jobs, at a time when existing slots were going unfilled.2 Over the next decade, the projected demand for health care workers was expected to grow at a rate three 1 Stephanie Allen, “2019 US and Global Health Care Industry Outlook,” Deloitte, January 2019, accessed November 1, 2019, www2.deloitte.com/global/en/pages/life-sciences-and-healthcare/articles/global-health-care-sector-outlook.html. 2 Ed Salsberg, “Health Care Jobs Projected to Continue to Grow Far Faster Than Jobs in the General Economy,” Health Affairs (blog), May 9, 2018, accessed November 1, 2019. www.healthaffairs.org/do/10.1377/hblog20180502.984593/full. This document is authorized for use only in Prof. M P Ram Mohan & Prof. Viswanath Pingali's Senior Management Programme (SMP-BL13) 2024 at Indian Institute of Management - Ahmedabad from Apr 2024 to Oct 2024. Page 2 9B20E010 times higher than the rest of the domestic economy. In 2018, hospitals had experienced the highest turnover rate in over a decade. Since 2014, the average hospital had replaced nearly 90 per cent of its workforce.3 High turnover rates were one of the main factors driving up health care costs. As senior leadership and human resources (HR) managers throughout the health care sector were aware, retaining good employees through a variety of incentives was much more cost-effective than recruiting and training replacement employees with high capabilities. Finding quality replacements in the current environment was a key issue, both for hospitals and for non-hospital operations, including physician’s offices, medical laboratories, and nursing home facilities (see Exhibit 1). These trends clearly underscored the challenges associated with employee retention. BHC AND HEALTH CARE INDUSTRY EMPLOYEE RETENTION BHC was founded in 2010 and headquartered in Orlando, Florida. The company, which offered consulting services to clients throughout the health care sector, had seen considerable revenue growth for several years. Most of the growth was attributable to its work in helping resolve ongoing issues in the US health care system. For example, BHC was asked by many clients to help develop plans to address rising costs and continuing employee turnover issues. Specifically, most HR managers in health care felt that solving employee turnover issues was their first priority. The US health care sector was experiencing a growing number of problems, as witnessed in an ongoing national debate on the future direction of the industry. The problems included rising health care costs, an aging population, and high rates of employee turnover. Domestic spending in the US health care industry was projected to reach over 19 per cent of the country’s gross domestic product within the next five years. Over that same period, global spending on health care was projected to rise to approximately $10 trillion, for an annual growth rate of over 5 per cent.4 These trends would continue to create more health care jobs, at a time when existing slots were going unfilled.5 Over the next decade, the projected demand for health care workers was expected to grow at a rate three times higher than the rest of the domestic economy. According to the US Bureau of Labor Statistics, demand for registered nurses was expected to grow by 15 per cent through the middle of the next decade. This was in addition to the nearly 1 million vacancies that were expected to occur over the same period.6 The employee turnover rate had also increased, rising 5 per cent over the previous decade to reach record levels of almost 20 per cent per year.7 In 2018, hospitals had experienced the highest turnover rate in over a decade. Since 2014, the average hospital had replaced nearly 90 per cent of its workforce.8 Employee burnout (i.e., overwork and stress), caused mainly by staff shortages, was a major factor driving the turnover. Some estimates suggested that over 50 per cent of physicians had exhibited at least one burnout 3 Brian Colosi, National Health Care Retention and RN Staffing Report (East Petersburg, PA: NSI Nursing Solutions, March 2019), accessed November 1, 2019, www.nsinursingsolutions.com/Documents/Library/NSI_National_Health_Care_Retention _Report.pdf. 4 Stephanie Allen, “2019 US and Global Health Care Industry Outlook,” Deloitte, January 2019, accessed November 1, 2019, www2.deloitte.com/global/en/pages/life-sciences-and-healthcare/articles/global-health-care-sector-outlook.html. 5 Ed Salsberg, “Health Care Jobs Projected to Continue to Grow Far Faster Than Jobs in the General Economy,” Health Affairs (blog), May 9, 2018, accessed November 1, 2019. www.healthaffairs.org/do/10.1377/hblog20180502.984593/full. 6 “Registered Nurses,” US Bureau of Labor Statistics, accessed November 1, 2019, www.bls.gov/ooh/healthcare/registered-nurses.htm. 7 Megan Wells, “Healthcare Turnover Rates in 2018,” Daily Pay, November 14, 2018, accessed November 1, 2019, https://business.dailypay.com/blog/employee-turnover-rates-in-the-healthcare-industry. 8 Colosi, op. cit. This document is authorized for use only in Prof. M P Ram Mohan & Prof. Viswanath Pingali's Senior Management Programme (SMP-BL13) 2024 at Indian Institute of Management - Ahmedabad from Apr 2024 to Oct 2024. Page 3 9B20E010 symptom.9 If the problem of turnover in the health care sector was not addressed, the issue would negatively affect both patient care and health outcomes. High turnover rates were also one of the main factors driving up health care costs. As senior leadership and human resources (HR) managers throughout the health care sector were aware, retaining good employees through a variety of incentives was much more cost-effective than recruiting and training replacement employees with high capabilities. Finding quality replacements in the current environment was a key issue, both for hospitals and for non-hospital operations, including physician’s offices, medical laboratories, and nursing home facilities (see Exhibit 1). These trends clearly underscored the challenges associated with employee retention. Stonebrook had uncovered various contributing factors to this challenge, including inadequate compensation, job dissatisfaction, and a lack of growth opportunities. He concluded that the compensation component of his overall strategy could be addressed relatively quickly, whereas job satisfaction and growth opportunities were a longer-term organizational challenge. He realized that adjusting compensation based on a COL factor would give the appearance of fairness throughout each organization. COL was often defined as the average cost of purchasing goods and services, such as food, housing, and transportation, for an accepted standard of living. However, these costs varied considerably throughout the country. For example, the COL in states such as Iowa and Georgia was 20 per cent below the national average. In comparison, the COL in California was a startling 41 per cent higher than the national average.10 To prepare his report to the CEO and board of directors, Stonebrook began by developing a framework to forecast the national consumer price index. He would then formulate specific COL forecasts for each BHC client. Stonebrook had to identify the most effective forecasting approach for his task. He also needed the United States’ Bureau of Labor Statistics monthly consumer price index for 2009 to 2019,11 which could be downloaded into an Excel spreadsheet for his purposes (see Exhibit 2). As Stonebrook knew, the national consumer price index was considered a measure of the country’s inflation, as experienced by consumers. It was used to calculate COL adjustments, among other things, by the Social Security Administration and various labour unions.12 However, the consumer price index was not a COL index, and it did not consider how consumers changed their buying patterns as they adjusted to relative price changes (i.e., substitution). Stonebrook planned to introduce his plan for COL adjustments to a cross section of BHC customers, as a test for overall employee retention strategy. If the response was favourable, he would then roll out the service to all BHC clients. However, Stonebrook saw this initiative as only the first step in providing BHC clients with a broad range of forecasting services and employee retention strategies by using the developed methodology. TIME SERIES FORECASTING Forecasting referred to the process of estimating future events. It was a fundamental practice for all aspects of organizational management. There were two key considerations regarding the accuracy of most 9 Audrey Lyndon, “Burnout among Health Professionals and Its Effect on Patient Safety,” Agency for Healthcare Research and Quality, Patient Safety Network, January 1, 2015, accessed November 1, 2019, https://psnet.ahrq.gov/perspective/burnout-among-health-professionals-and-its-effect-patient-safety. 10 Andrew Lisa, “The Cost of Living Across America, Mortgages 101,” GO Banking Rates, January 31, 2019, accessed November 1, 2019, www.gobankingrates.com/saving-money/home/cost-of-living-by-state/#5. 11 “Inflation & Prices,” US Bureau of Labor Statistics, accessed November 1, 2019, www.bls.gov/data/#prices. 12 “Latest Cost-of-Living Adjustment,” Social Security Administration, accessed November 1, 2019, www.ssa.gov/OACT/COLA/latestCOLA.html. This document is authorized for use only in Prof. M P Ram Mohan & Prof. Viswanath Pingali's Senior Management Programme (SMP-BL13) 2024 at Indian Institute of Management - Ahmedabad from Apr 2024 to Oct 2024. Page 4 9B20E010 forecasting models. First, the level of accuracy tended to decrease over time. Second, forecasting of group events tended to have greater accuracy than forecasting for individual events. Time series forecasting was a subset of predictive analytics. It was based on the idea that past events could be a good indicator of future events. One of the most commonly used time series forecasting models, which was available in Microsoft Excel, was called “exponential smoothing” (see Exhibits 3, 4, and 5). This technique continuously updated a forecast with new data and came in three versions: one-parameter, two- parameter (including trend effects), and three-parameter (including trend and seasonal effects). Microsoft Excel contained various forecasting models, including FORECAST.EST, which Stonebrook decided to use in developing his forecasting for values for BHC. Specifically, FORECAST.EST was designed to automatically determine the optimal values for the smoothing coefficients. The computational goal was to determine the coefficient values that minimized mean square error, which was defined as the average of the sum of the errors (i.e., the difference between the actual values and forecasted values) squared.13 OVERVIEW OF RETENTION STRATEGIES Most HR professionals considered compensation increases an effective means to retain employees, especially during times of very low unemployment rates. Compensation increases could be applied using two basic strategies: salary adjustments and salary bonuses. The decision to offer employees a salary increase or a bonus was usually made based on the organization’s local conditions. For example, BHC clients could apply the developed forecasting methodology to add a COL adjustment to their employee compensation rates based on the conditions in the local economy where the client operated. The effect of these compensation adjustments on the organization’s overall operational costs would be easily offset by a reduction in the costs of recruiting and training replacement employees. There were various other employee retention strategies that BHC clients could consider, including educational tuition reimbursement, incentive compensation, promotion, and enhanced retirement benefits. In particular, education tuition reimbursement supported ongoing education and professional development, which was essential for ensuring exceptional patient outcomes, improving productivity, and providing employee growth opportunities. PREPARING THE PLAN In preparing his plan, Stonebrook decided to focus on two basic tasks. His first step was to develop a two- year, monthly-based consumer price index forecast. His second step would be to identify specific employee retention strategies to recommend to BHC clients. Stonebrook would accomplish the first task using Microsoft Excel’s FORECAST.ETS algorithm. For the second task, he would elicit both short-term (e.g., compensation) and longer-term (e.g., career development) plans for BHC clients, with the goal to reduce their employee turnover rates. Stonebrook set to work to begin preparing the plan that he would present to Tadwell and to the BHC board of directors in two weeks. 13 “Mean Square Error: Definition and Example,” Statistics How To, accessed November 1, 2019, www.statisticshowto.datasciencecentral.com/mean-squared-error. This document is authorized for use only in Prof. M P Ram Mohan & Prof. Viswanath Pingali's Senior Management Programme (SMP-BL13) 2024 at Indian Institute of Management - Ahmedabad from Apr 2024 to Oct 2024. Page 5 9B20E010 EXHIBIT 1: UNEMPLOYMENT RATE IN THE US HEALTH CARE SECTOR (2009–2018) 7 6.4 5.9 5.8 5.9 6 5.5 Unemployment Rate (%) 5 4.4 3.8 3.6 4 3.2 3.0 3.0 2.9 2.8 3 2.4 2.6 2.1 1.8 2 1.5 1.5 1.2 1 0 2009 2010 2011 2012 2013 2014 2015 2016 2017 2018 Hospital Non-Hospital Source: Prepared by the case author with information from “Unemployed Persons by Industry, Class of Worker, and Sex,” US Bureau of Labor Statistics, accessed April 28, 2020, www.bls.gov/cps/aa2009/cpsaat26.pdf. EXHIBIT 2: SAMPLE CPI-W DATABASE (2019) Month CPI-W Values January 245.133 February 246.218 March 247.768 April 249.332 May 249.871 June 249.747 July 250.236 August 250.112 September 250.251 October 250.894 November 250.644 December 250.452 Note: CPI-W = Consumer Price Index for urban wage earners and clerical workers. The CPI-W is a variation of the consumer price index, compiled by the US Bureau of Labor Statistics, that tracks retail prices in relation to urban hourly wage earners and clerical workers. Source: Prepared by the case author with information from “Databases, Tables, and Calculators by Subject,” US Bureau of Labor Statistics, accessed April 28, 2020, https://data.bls.gov/pdq/SurveyOutputServlet. This document is authorized for use only in Prof. M P Ram Mohan & Prof. Viswanath Pingali's Senior Management Programme (SMP-BL13) 2024 at Indian Institute of Management - Ahmedabad from Apr 2024 to Oct 2024. Page 6 9B20E010 EXHIBIT 3: SIMPLE EXPONENTIAL SMOOTHING MODEL (ONE-PARAMETER MODEL) The one-parameter (simple) exponential model requires only three basic inputs: the actual values, the forecasted variable values from the previous period, and a smoothing coefficient. The following equation presents the standard form of the one-parameter exponential smoothing model: Ft = a × At – 1 + (1 – a) × Ft – 1 where: Ft = Forecast value for period t Ft – 1 = Forecast value for period t – 1 At = Actual value for period t a = Smoothing coefficient (0 < a < 1) The smoothing coefficient (a) ranges in value between 0 and 1. Using a smoothing coefficient of 0 results in a forecast equal to the previous forecast value. A smoothing coefficient of 1 yields a forecast equal to the previous actual value. The most appropriate smoothing coefficient value depends on the nature of the time series data. Generally, larger coefficient values result in a quicker response in the forecast values, and smaller alpha values yield a slower response. Typically, a small coefficient value is appropriate for volatile data, and larger values can be used for stable data. Basically, the one-parameter model can only be used to forecast one period beyond the last actual value. This model can be easily implemented in Microsoft Excel. The model however, is not effective when a trend is present. The forecasted values tend to lag actual values. Determining the best smoothing coefficient value can be based on an examination of the forecasting errors. Source: Prepared by the case author based on formulation from information in the case. This document is authorized for use only in Prof. M P Ram Mohan & Prof. Viswanath Pingali's Senior Management Programme (SMP-BL13) 2024 at Indian Institute of Management - Ahmedabad from Apr 2024 to Oct 2024. Page 7 9B20E010 EXHIBIT 4: DOUBLE EXPONENTIAL SMOOTHING MODEL (TWO-PARAMETER MODEL) The two-parameter (double) model can be used to develop forecasts over several future periods. This model is often referred to as Holt’s two-parameter smoothing model. Optimal values for the smoothing coefficient (a) and the trend coefficient (b) can be determined using the mean squared error. Forecasts beyond the range of the database are generated by adding the last smoothed value to the last trend value, where the trend value is multiplied by the number of periods into the future. The following equations present the standard form of the two-parameter exponential model: Ft = a × At – 1 + (1 – a) × (Ft – 1 + Tt – 1) Tt = b × (Ft – Ft – 1) + (1 – b) × Tt – 1 Ft + 1 = Ft + T t The following relationship can be used for generating forecasts: Ft + p = Ft + P × Tt where: Ft + 1 = Forecast value for period t + 1 Ft = Forecast value for period t Tt = Trend value for period t At = Actual value for period t a = Smoothing coefficient (0 < a < 1) b = Trend coefficient (0 < b < 1) P = Periods to be forecast Source: Prepared by the case author based on formulation from information in the case. This document is authorized for use only in Prof. M P Ram Mohan & Prof. Viswanath Pingali's Senior Management Programme (SMP-BL13) 2024 at Indian Institute of Management - Ahmedabad from Apr 2024 to Oct 2024. Page 8 9B20E010 EXHIBIT 5: HOLT-WINTERS EXPONENTIAL SMOOTHING MODEL (THREE-PARAMETER MODEL) The three-parameter (Holt-Winters) model, which includes a seasonal component, can be used to forecast multiple periods, much like the two-parameter model. There are two modelling sub-options: additive and multiplicative. The following equations present the standard form of the three-parameter exponential model (additive form): Ft = a × (At – 1 ÷ St – s) + (1 – a) × (Ft – 1 + Tt – 1) Tt = b × (Ft – Ft – 1) + (1 – b) × Tt – 1 St = c × (At – Ft) + (1 – c) × St – s Ft + 1 = Ft + Tt + St The following relationship can be used for generating forecasts: Ft + p = Ft + P × Tt + St + p – s where: Ft + 1 = forecast value for period t + 1 Ft = forecast value for period t Tt = Trend value for period t St = Seasonal value for period t At = Actual value for period t a = Smoothing coefficient (0 < a < 1) b = Trend coefficient (0 < b < 1) c = Seasonal coefficient (0 < c < 1) P = periods to be forecast s = length of the seasonal cycle (e.g., quarterly – s = 4). Microsoft Excel’s FORECAST.ETS model, which was based on the additive option, can be used to generate forecasts where the values for a, b, c are internally optimized. Source: Prepared by the case author based on formulation from information in the case. This document is authorized for use only in Prof. M P Ram Mohan & Prof. Viswanath Pingali's Senior Management Programme (SMP-BL13) 2024 at Indian Institute of Management - Ahmedabad from Apr 2024 to Oct 2024.