Business Mathematics and Statistics - 1 PDF
Document Details
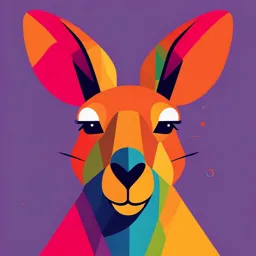
Uploaded by IngeniousHibiscus
Tags
Related
- Simple Linear Regression Analysis PDF
- Business Mathematics & Statistics (MTH 302) Lecture 1 PDF
- Business Mathematics and Statistics Subtitles PDF
- MTH302 Business Mathematics & Statistics Handouts May 2022 PDF
- Econometrics I Past Paper PDF
- BSc Business Administration Mock Exam - Business Mathematics PDF
Summary
This document is a chapter on linear correlation from a business mathematics and statistics textbook. It defines correlation coefficient and covers its properties and the scatter diagram method for determining correlation.
Full Transcript
# Business Mathematics and Statistics - 1 ## Chapter - 1 ### Linear Correlation **Define correlation coefficient and write its properties.** A correlation coefficient is a numerical measure of the degree of association between two random variables. It is denoted by *r(x, y)or ry, or r*. **Prope...
# Business Mathematics and Statistics - 1 ## Chapter - 1 ### Linear Correlation **Define correlation coefficient and write its properties.** A correlation coefficient is a numerical measure of the degree of association between two random variables. It is denoted by *r(x, y)or ry, or r*. **Properties of Correlation Coefficient** * The correlation coefficient *r* is a pure number and is independent of the units of measurement. * *r(x, y) = r(y, x)* * -1 ≤ *r* ≤ 1, or 0 ≤ *r*² ≤ 1 * *r* is independent of the change of origin and scale of measurement *r(x, y) = r(u, v)* where *u = (X-A)/H, v = (Y-B)/K* where *h > 0, k > 0* and *h, k, A, B* are real constants. * Sign of *r* depends on the sign of *Cov(x, y)*. * *r(x, y) = r(-x, -y), r(x, y) = r(-x, y) = -r(x, y)* * If *y = a + bx (b > 0)* or *x = c + dy (d > 0)*, then *r = 1*. * If *y = a + bx (b < 0)* or *x = c + dy (d < 0)*, then *r = -1*. * *r* is the geometric mean of two regression coefficients. * If *x* and *y* are independent, they are uncorrelated, but the converse is not true. ### Scatter Diagram Method The simplest device for ascertaining whether two variables are related is to prepare a dot chart called a scatter diagram. When this method is used, the given data are plotted on a graph paper in the form of dots, i.e., for each pair of *X* and *Y* values, we put a dot, and thus obtain as many points as the number of observations. By looking at the scatter of various points, we can form an idea as to whether the variables are related or not. The greater the scatter of the plotted points on the chart, the lesser is the relationship between the two variables. If all the points lie on a straight line falling from the lower left-hand corner to the upper right-hand corner, correlation is said to be perfectly positive (i.e., *r = 1*). On the other hand, if all the points are lying on a straight line rising from the upper left-hand corner to the lower right-hand corner of the diagram, correlation is said to be perfectly negative (i.e., *r = -1*). If the points are widely scattered over the diagram, it indicates very little relationship between the variables. Correlation shall be positive if the points are rising from the lower left-hand corner to the upper right-hand corner and negative if the points are running from the upper left-hand side to the lower right-hand side of the diagram. If the plotted points lie on a straight line parallel to the X-axis or in a haphazard manner, it shows the absence of any relationship. **Merits** * It is a very easy and non-mathematical method of studying correlation. * It can be easily understood and interpreted. * It is the first step of studying correlation. **Demerits** * By this method, we can get the idea about the direction of correlation, but we cannot get the exact degree of correlation between two variables in terms of some quantification approach. ### Rank Correlation Coefficient Method of Spearman Rank correlation can be defined as the correlation between the ranks assigned to individuals in two characteristics. It is measured by Spearman's Rank Correlation Coefficient (*ρ*): ``` ρ= 1 - (6 Σ d²)/(n(n² - 1) ``` Where *d* stands for the difference between the ranks of the *i*-th individual among the two characters, and *N* stands for the number of paired observations. The value of rank correlation coefficient varies between -1 and +1. -1 implies complete disagreement in the order of ranks, while +1 implies complete agreement in the order of ranks. The above formula is used when ranks are not repeated. **Features** * The sum of the differences of ranks between two variables shall be zero. * Spearman's correlation coefficient is distribution free or non-parametric because no strict assumptions are made about the form of the population from which sample observations are drawn. * The Spearman's correlation coefficient is nothing but Karl Pearson's correlation coefficient between the ranks. Hence, it can be interpreted in the same manner as Pearson's correlation coefficient. **Merits** * This method is simple to understand and easy to apply compared with Karl Pearson's method. The laborious calculations for the product-moment are replaced by rankings. * This method can conveniently be used as a measure of the degree of association between two attributes where measurements on characteristics are not available, but the individuals can be ranked in some order without difficulty. For example, in a group of salesmen, the extent of association between 'intelligence' and 'efficiency' can be easily obtained by arranging the individuals in order of proficiency assessed. * Even when exact measurements are given, the rank correlation method can be applied to ascertain a rough estimate of the degree of correlation. * The method can conveniently be used when the data are irregular or when the extreme items are erratic or inaccurate because rank correlation coefficient is not based on the assumption of normality of data. **Demerits** * This method is applicable only to individual observations rather than to grouped frequency distribution. * Under the ranking method, original values are not taken into account, therefore the result obtained is only approximate. * When the number of observations exceeds 30, the calculations become quite tedious. ### Probable Error The probable error of the coefficient of correlation helps in interpreting its value. With the help of probable error, it is possible to determine the reliability of the value of the coefficient in so far as it depends on the conditions of random sampling. The probable error of the coefficient of correlation is optioned as follows: ``` (PE) = 0.6745 × (1-(r²))/√n) ``` where * *r* is the coefficient of correlation and *N* the number of pairs of observation. * If the value of *r* is less than the probable error, there is no evidence of correlation, i.e., the value of *r* is not at all significant. * If the value of *r* is more than six times the probable error, the coefficient of correlation is practically certain, i.e., the value of *r* is significant. * By adding, and subtracting the value of probable error from the coefficient of correlation, we get respectively the upper and lower limits within which the coefficient of correlation in the population can be expected to lie. Symbolically, p = r +P.E. ### Merits and Demerits of Karl Pearson's Method **Merits** * This method gives a precise and a summary quantitative value which can be interpreted meaningfully. It gives direction as well as the degree of relationship between the two variables. * The coefficient of correlation along with other information helps in estimating the value of the dependent variable from the known value of an independent variable. **Demerits** * This method assumes that there is a linear relationship between the variables under study regardless of the fact whether such relationship exists or not. * Compared to other methods, the computation of correlation coefficient by this method is time-consuming. * The value of the coefficient is unduly affected by extreme items. * The coefficient of correlation may give a misleading picture of the extent of relationships between the variables if the data are not reasonably homogeneous. For example, if the scatter diagram shows the points in separate groups, the correlation coefficient may be very high for all the group taken together, yet it may be close to zero for some groups. ### Uses of Correlation The use of correlation is there both in physical and social sciences, but we shall confine ourselves to the latter, and particularly to the fields of business and economics. * Economic theory and business studies show relationships between variables like price and quantity demanded, advertising expenditure and sales, etc. The correlation analysis helps in deriving precisely the degree and direction of such relationships. * The relationships between variables are studied under various economics laws or the concepts like the law of demand and the elasticity of demand. The advantage of statistical techniques of correlation is that the average of relationships in a series can be summed up in a single value of change called the coefficient of correlation. * "Correlation analysis contributes to the understanding of economic behavior, aids in locating the critically important variables on which others depend, may reveal to the economist the connections by which disturbances spread and suggest to him the paths through which stabilizing forces may become effective. * "The effect of correlation is to reduce the range of uncertainty of our prediction." The prediction based on correlation analysis will be more reliable and near to reality. * The concepts of regression and ratio of variation are also based upon the measure of correlation. ### Standard Error Standard Error is also more commonly used a measure of reliability of the coefficient of correlation. As against the Probable error, which has 50% chance of including similar coefficients calculated from other series drawn from the same population, the Standard Error has 67.45% chance of covering. If 'r' is the observed correlation coefficient in a sample of *N* pairs of observations, its standard error, usually denoted by S.E. (r), is given by: ``` S.E. (r) = √(1-(r²))/√n ``` The probable error of the coefficient of correlation can also be calculated from S.E. of the coefficient of the correlation by the following formula: ``` P.E. = 0.6745 × S.E. (r) = 0.6745 × √(1-(r2)/√n ``` The cause for taking the factor 0.6745 is that in a normal distribution, 50% of the observations lie in the range μ±0.6745σ, where, * μ = mean, σ = Standard Deviation ### Practicals **Based on Karl Pearson** 1. Calculate Pearson's coefficient of correlation between advertisement cost and Sales as per the data given below: | Cost | Sales | |---|---| | 23 | 18 | | 27 | 22 | | 28 | 23 | | 29 | 24 | | 30 | 25 | | 31 | 26 | | 33 | 28 | | 35 | 29 | | 36 | 30 | | 39 | 32 | **Ans** * **Note:** Here smallest value is duducted. | X | Y | u = X-23 | v = Y-18 | u² | v² | uv | |---|---|---|---|---|---|---| | 23 | 18 | 0 | 0 | 0 | 0 | 0 | | 27 | 22 | 4 | 4 | 16 | 16 | 16 | | 28 | 23 | 5 | 5 | 25 | 25 | 25 | | 29 | 24 | 6 | 6 | 36 | 36 | 36 | | 30 | 25 | 7 | 7 | 49 | 49 | 49 | | 31 | 26 | 8 | 8 | 64 | 64 | 64 | | 33 | 28 | 10 | 10 | 100 | 100 | 100 | | 35 | 29 | 12 | 11 | 144 | 121 | 132 | | 36 | 30 | 13 | 12 | 169 | 144 | 156 | | 39 | 32 | 16 | 14 | 256 | 196 | 224 | | 311 | 257 | 81 | 77 | 859 | 751 | 802 | * **From the above table:** ``` r= (nuv - (Σu)(Σv)) / √(nΣu² - (Σu)²) √(nΣv² - (Σv)² ) r = (10 (802) - (81)(77)) / √(10(859) - (81)²) √(10(751) - (77)²) r= 8020 - 6237 / √8590 - 6561 √7510 - 5929 r = 1783/ √2029 √1581 r = 1783/ 45.04 × 39.76 r = 1783/ 1790.79 r = 0.996 ``` 2. For the following data, find correlation coefficient between x and y: | x| y | |---|---| | 1100 | 0.30 | | 1200 | 0.29 | | 1300 | 0.29 | | 1400 | 0.25 | | 1500 | 0.24 | | 1600 | 0.24 | | 1700 | 0.24 | | 1800 | 0.29 | | 1900 | 0.18 | | 2000 | 0.15 | **Ans** | N | Y | (X+100) | (V2100) | (X+100)² | V² | (X+100)V | |---|---|---|---|---|---|---| | 1100 | 0.30 | 11 | 30 | 121 | 900 | 330 | | 1200 | 0.29 | 12 | 29 | 144 | 841 | 348 | | 1300 | 0.29 | 13 | 29 | 169 | 841 | 377 | | 1400 | 0.25 | 14 | 25 | 196 | 625 | 350 | | 1500 | 0.24 | 15 | 24 | 225 | 576 | 360 | | 1600 | 0.24 | 16 | 24 | 256 | 576 | 384 | | 1700 | 0.24 | 17 | 24 | 289 | 576 | 408 | | 1800 | 0.29 | 18 | 29 | 324 | 841 | 522 | | 1900 | 0.18 | 19 | 18 | 361 | 324 | 342 | | 2000 | 0.15 | 20 | 15 | 400 | 225 | 300 | | | ΣY | 155 | ΣV | 2485 | 6325 | 3721 | * **Calculating r** ``` r= (nuv - (Σu)(Σv)) / √(nΣu² - (Σu)²) √(nΣv² - (Σv)² ) r = (10 (3721) - (155) (247)) / √(10(2485) - (155)²) √(10(6325) - (247)²) r= 37210-38285 / √24850-24025 √63250 - 61009 = -1075/ √825 √2241 r= -1075/ √825√2241 r = 1075/ √825 √2241 r= -28.72/ √1359.60 = - 0.79 ``` 3. Find correlation coefficient from the following data: * Σ *x* = 50, Σ (*x* - 40) = Σ (*y* - 50) = 160, Σ (*x* - 50)(*y* - 60) = 256, ȳ = 60, Σ (*x* - 45)² = 656, Σ (*y* - 64)² = 1280 **Ans** * Σ (*x*- 40) = 160 * ... Σ *x* - 40*n* = 160 * ... 50*n* - 40*n* = 160 * ... 10*n* = 160 * … *n*= 16 * Σ *x* = *n**x* = (16) (50) = 800 * Σ *y* = *n*ȳ = (16) (60) = 960 * Σ (*x* - 45)² = 656 * Σ (*x*² - 90*x* + 2025) = 656 * Σ *x*² - 90 Σ *x* + 2025*n* = 656 * Σ *x*² - 90(800) + 2025 (16) = 656 * Σ *x*² = 656 + 72000 - 32400 * Σ *x*² = 40256 * Σ (*y* - 64)² = 1280 * Σ (*y*² - 128 *y* + 4096) = 1280 * Σ *y*² - 128 Σ *y* + 4096*n* = 1280 * Σ *y*² - 128(960) + 4096(16) = 1280 * Σ *y*² = 1280 + 122880 -65536 * Σ *y*² = 58624 * Σ (*x* - 50) (*y* - 60) = 256 * Σ (*xy* - 60 *x* - 50 *y* + 300) = 256 * Σ *xy* - 60 Σ *x* - 50 Σ *y* + 300 *n* = 256 * Σ *xy* - 60 (800) - 50 (960) + 300(16) = 256 * Σ *xy* = 256 + 48,000 + 48,000 - 48,000 * Σ *xy* = 48,256 * **Calculating r** ``` r = (n Σxy - (Σx)(Σy)) / √[n Σx² - (Σx)²][n Σy² - (Σy)²] r = (16 (48256) - (800) (960)) / √[16(40256) - (800)²] [16(58624) - (960)²] r = 772096 - 768000 / √[644096 - 6,40,000] [937984 - 921600] r = 4096 / √[4096] [16384] r = 4096 / 8192 r= 0.50 ``` 4. Find coefficient correlation from the following data: * n = 7, Σ(*x* - 1) = 59, Σ (*y* - 2) = 66, Σ (*x* - 3)² = 337, Σ(*y* - 4)² = 434, xy = 802 **Ans. We Know that** * Σ (*x* - A) = Σ *x* - nA where A = real constant * Σ (*x*- 1) = 59 * Σ*x* - n. 1 = 59 * Σ*x*= 59 + 7 = 66 * Σ(*y*- 2) = 66 * Σ*y* - n22 = 66 * Σ*y* - 722 = 66 * Σ*y* = 66 + 14 = 80 * Σ (*x* - 3)² = 337 * Σ (*x*² - 6 *x* + 9) = 337 * Σ *x*² - 6 Σ *x* + 9 *n* = 337 * Σ *x*² - 6 *66* + 9 *7* = 337 * Σ *x*² - 396 + 63 = 337 * Σ *x*² = 670 * Σ (*y* - 4)² = 434 * Σ (*y*² - 8 *y* + 16) = 434 * Σ *y*² - 8 Σ *y* + 16 *n* = 434 * Σ *y*² - 8 *80* + 16 *7* = 434 * Σ *y*² - 640 + 112 = 434 * Σ *y*² = 434 + 640 - 112 = 962 * **Calculating r** ``` r = (n Σxy - (Σx)(Σy)) / √[n Σx² - (Σx)²][n Σy² - (Σy)²] r = 7 (802) - (66) (80) / √7(670) - (66) √7(962) - (80)² r = 5614 - 5280 / √4690 - 4356 √6734 - 6400 r = 334 / √334 √334 r = 334 / 334 r = 1 ``` 5. For a sample of 20 observations, the correlation coefficient is 0.3. Mean for the variables *x* and *y* are 15 and 20 respectively. The standard deviations for *x* and *y* are 4 and 5 respectively. At the time of calculation, the one observation of *x* is wrongly taken as 17 instead of 27, and the one observation of *y* is wrongly taken as 35, instead of 30. Obtain the corrected correlation coefficients. **Ans.** First, find Σ*x*, Σ*y*, Σ*x*², Σ*y*², Σ*xy* * Σ *x*= *n*. *x* = 20 × 15=300 * Σ*y*= *n*. ȳ = 20 × 20= 400 * Σ*x*² = n (*σx*² + *x*²) = 20(16+225) = 4,820 * Σ*y*² = n (*σy*² + *y*²) = 20 (25+400) = 8,500 ``` r= (Σxy - nxy) / n. *σx*. *σy* 0.3 = (Σxy - 20 x 15 x 20) / 20 x 4 x 5 0.3 x 400 = Σ*xy* - 20 × 15 × 20 .: Σxy = 120 + 6,000=6,120 ``` | Particular | Old | + | New | | - | - | - | - | | Σ *x* | 300 | 27 | 310 | | Σ *y* | 400 | 30 | 395 | | Σ*x*² | 4820 | 729 | 5260 | | Σ*y*² | 8500 | 900 | 8175 | | Σ *xy* | 6120 | 810 | 6335 | ``` r= (n Σxy - (Σx)(Σy)) / √[n Σx² - (Σx)²][n Σy² - (Σy)²] r = 20 x 6335-(310)(395) / √[20× (5260)-(310)²][20 × (8175)-(395)2] r = 1,26,700- 1,22,450 / √[1,05, 200-96,100][1,63,500-1,56,025] r = 4,250 / √9.100 × 7475 r = 4,250 / 8,247.57 r = 0.52 ``` 6. A computer while calculating correlation coefficient between two variables *X* and *Y* from 25 pairs of observations obtained the following results: n = 25, Σ *X* = 125, Σ *X*² = 650, Σ *y* = 100, Σ *Y*² = 460, Σ *x*y = 508. It was, however, discovered at the time of checking that two pairs of observations were not correctly copied. They were taken as (6, 14) and (8, 6) while the correct values were (8, 12) and (6, 8). Prove that the correct value of the correlation coefficient should be 2/3. **Ans.** * Corrected Σ*x* = 125-6-8+8+6=125 * Corrected Σ*y* = 100 -14 - 6 +12 + 8 = 100 * Corrected Σ *x*² = 650 - 6² - 8² + 8² + 6² = 650 * Corrected Σ *y*² = 460-14² - 6² + 12²+ 8² = 436 * Corrected Σ *x*y = 508 - (6 * 14) - (8 *6) + (8 * 12) + (6 * 8) = 520 * **Corrected value of r is given by** ``` r= (n Σxy - (Σx)(Σy)) / √[n Σx² - (Σx)²][n Σy² - (Σy)²] r= 13,000-12,500 / √[16,250-15,625] [10,900-10,000] r= 500 / √625×900 r = 500 / 25 × 30 r= 2/3 ``` 7. The correlation coefficient for a sample from a bivariate population is 0.6 and its Probable Error is 0.05396. Find number of pairs of the sample. Also, find the probable limits for the population correlation coefficient. Also discuss if the value of *r* is significant or not. **Ans** * Here, r = 0.6 and P.E. = 0.05396 ``` P.E. = 0.6745 (1 - r²) / √n 0.05396 = 0.6745 (1 - r²) / √n 0.05396 = 0.6745 (1 - 0.36) / √n 0.05396 = 0.6745 (0.64) / √n √n = 0.43168 / 0.05396 = 8 n = 64 ``` **The probable limits of population correlation coefficient** * r + P.E. * 0.6 ± 0.054 * = 0.546 to 0.654 **Significance of r** * We have *r* = 0.6 and 6P.E. = 6 * 0.05396 = 0.32. Since *r* is much greater than 6P.E., the value of *r* is highly significant. 8. Limits for the population correlation coefficient on the basis of sample were found to be 0.375 and 0.625. Find the number of observation 'n'. **Ans.** * Here, *r* - P.E. = 0.375 ............. (i) * *r* + P.E = 0.625 ............. (ii) * Now, subtract Eq.2 from Eq. 1, we get * -2 P.E. = - 0.25 * P.E = 0.125 * Now put P.E. = 0.125 in Eq.1 * *r* - P.E = 0.375 * *r* - 0.125 = 0.375 * *r* = 0.375 + 0.125 * *r* = 0.5 ``` P.E. = 0.6745 (1 - r²) / √n 0.125 = 0.6745 (1 - 0.25) / √n √n = 0.6745 (0.75) / 0.125 = 4 n = 16 ``` 9. Find the number of observations from the following data: *r* = 0.9, P.E. (*r*) = 0.0128 **Ans.** * Here, *r* = 0.9 and P.E. = 0.0128 ``` P.E. = 0.6745 (1 - r²) / √n 0.0128 = 0.6745 (1 - 0.81) / √n 0.0128 = 0.6745 (0.19) / √n √n = 0.128155 / 0.0128 = 10 n = 10 ``` ### Based on Rank Correlation 10. Find rank coefficient of correlation: | X | Y | |---|---| | 15 | 40 | | 20 | 30 | | 25 | 50 | | 12 | 30 | | 40 | 20 | | 60 | 10 | | 20 | 30 | | 80 | 60 | **Ans.** Let X denote the advertisement cost ('000 Rs.) and Y denote the sales (lakhs): | X | Y | Rank of X | Rank of Y | d= x-y | d² | |---|---|---|---|---|---| | 15 | 40 | 7 | 3 | 4 | 16 | | 20 | 30 | 5.5 | 5 | 0.5 | 0.25 | | 25 | 50 | 4 | 2 | 2 | 4 | | 12 | 30 | 8 | 5 | 3 | 9 | | 40 | 20 | 3 | 7 | -4 | 16 | | 60 | 10 | 2 | 8 | -6 | 36 | | 20 | 30 | 5.5 | 5 | 0.5 | 0.25 | | 80 | 60 | 1 | 1 | 0 | 0 | | | | | | | Σd² = 81.5 | * Here, n = 8. Observation 20 is repeated 2 times in x, m = 2, and observation 30 is repeated 3 times in y, m = 3. ``` r = 1 - ((6 Σd² + (m³ -m)/12) + (m³ -m)/12) / (n(n² - 1)) r = 1-((6 *81.5 + (2³ -2)/12) + (3³ -3)/12) / 8(8² - 1) r = 1-(6 * 81.5 + 0.5 + 2 ) / 8 (64 - 1) r=1- (504) / 504 r = 1 - 1 = 0 ``` 11. Find the number of observations from following data: Σ (*x* - *x*)² = 90, Σ (*x* - *x*)(*y* - *y*) = 60, *σx* = 4, *r*= 0.5 **Ans.** Given *r*= 0.5, Σ (*x* - *x*)(*y* - *y*) = 60 Σ(*x* - *x*)² = 90, *σx* = 4 * **Calculating *n*** ``` s*x* = √(Σ(x - *x*)²/n) s*x* = √(90/n) r= (Σ(x - *x*)(y - *y*)) / (n * σx * σy) 0.5 = 60 / (√(90/n) × 4 × σy) 0.5 = 60 / (√90*n*) * 4 n = 60 / (0.5 * √90 * 4) n = 3.333 ``` 1