Black Swan 6 PDF - Fractality
Document Details
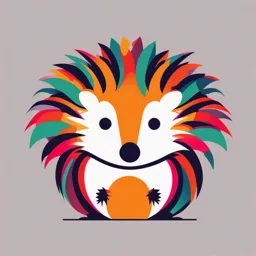
Uploaded by scrollinondubs
Stanford School of Medicine
Tags
Summary
This document describes fractals and their properties. It explains how the concept of fractals applies to various aspects, such as visual arts, music, and poetry. It also discusses the role of computers in generating fractal objects and how fractals relate to randomness. The text also examines the distribution of wealth and the application of fractals to various fields.
Full Transcript
Fractality But rst, a description of fractals. Then we will show how they link to what we call power laws, or scalable laws. Fractal is a word Mandelbrot coined to describe the geometry of the rough and broken—from the Latin fractus, the origin of fractured. Fractality is the repetition of...
Fractality But rst, a description of fractals. Then we will show how they link to what we call power laws, or scalable laws. Fractal is a word Mandelbrot coined to describe the geometry of the rough and broken—from the Latin fractus, the origin of fractured. Fractality is the repetition of geometric patterns at di erent scales, revealing smaller and smaller versions of themselves. Small parts resemble, to some degree, the whole. I will try to show in this chapter how the fractal applies to the brand of uncertainty that should bear Mandelbrot’s name: Mandelbrotian randomness. The veins in leaves look like branches; branches look like trees; rocks look like small mountains. There is no qualitative change when an object changes size. If you look at the coast of Britain from an airplane, it resembles what you see when you look at it with a magnifying glass. This character of self-a nity implies that one deceptively short and simple rule of iteration can be used, either by a computer or, more randomly, by Mother Nature, to build shapes of seemingly great complexity. This can come in handy for computer graphics, but, more important, it is how nature works. Mandelbrot designed the mathematical object now known as the Mandelbrot set, the most famous object in the history of mathematics. It became popular with followers of chaos theory because it generates pictures of ever increasing complexity by using a deceptively minuscule recursive rule; recursive means that something can be reapplied to itself in nitely. You can look at the set at smaller and smaller resolutions without ever reaching the limit; you will continue to see recognizable shapes. The shapes are never the same, yet they bear an a nity to one another, a strong family resemblance. These objects play a role in aesthetics. Consider the following applications: Visual arts: Most computer-generated objects are now based on some version of the Mandelbrotian fractal. We can also see fractals in architecture, paintings, and many works of visual art—of course, not consciously incorporated by the work’s creator. Music: Slowly hum the four-note opening of Beethoven’s Fifth Symphony: ta-ta-ta-ta. Then replace each individual note with the same four-note opening, so that you end up with a measure of sixteen notes. You will see (or, rather, hear) that each smaller wave resembles the original larger one. Bach and Mahler, for instance, wrote submovements that resemble the larger movements of which they are a part. Poetry: Emily Dickinson’s poetry, for instance, is fractal: the large resembles the small. It has, according to a commentator, “a consciously made assemblage of dictions, metres, rhetorics, gestures, and tones.” Fractals initially made Benoît M. a pariah in the mathematical establishment. French mathematicians were horri ed. What? Images? Mon dieu! It was like showing a porno movie to an assembly of devout Eastern Orthodox grandmothers in my ancestral village of Amioun. So Mandelbrot spent time as an intellectual refugee at an IBM research center in upstate New York. It was a f*** you money situation, as IBM let him do whatever he felt like doing. But the general public (mostly computer geeks) got the point. Mandelbrot’s book The Fractal Geometry of Nature made a splash when it came out a quarter century ago. It spread through artistic circles and led to studies in aesthetics, architectural design, even large industrial applications. Benoît M. was even o ered a position as a professor of medicine! Supposedly the lungs are self-similar. His talks were invaded by all sorts of artists, earning him the nickname the Rock Star of Mathematics. The computer age helped him become one of the most in uential mathematicians in history, in terms of the applications of his work, way before his acceptance by the ivory tower. We will see that, in addition to its universality, his work o ers an unusual attribute: it is remarkably easy to understand. A few words on his biography. Mandelbrot came to France from Warsaw in 1936, at the age of twelve. Owing to the vicissitudes of a clandestine life during Nazi-occupied France, he was spared some of the conventional Gallic education with its uninspiring algebraic drills, becoming largely self-taught. He was later deeply in uenced by his uncle Szolem, a prominent member of the French mathematical establishment and holder of a chair at the Collège de France. Benoît M. later settled in the United States, working most of his life as an industrial scientist, with a few transitory and varied academic appointments. The computer played two roles in the new science Mandelbrot helped conceive. First, fractal objects, as we have seen, can be generated with a simple rule applied to itself, which makes them ideal for the automatic activity of a computer (or Mother Nature). Second, in the generation of visual intuitions lies a dialectic between the mathematician and the objects generated. Now let us see how this takes us to randomness. In fact, it is with probability that Mandelbrot started his career. A Visual Approach to Extremistan/Mediocristan I am looking at the rug in my study. If I examine it with a microscope, I will see a very rugged terrain. If I look at it with a magnifying glass, the terrain will be smoother but still highly uneven. But when I look at it from a standing position, it appears uniform—it is almost as smooth as a sheet of paper. The rug at eye level corresponds to Mediocristan and the law of large numbers: I am seeing the sum of undulations, and these iron out. This is like Gaussian randomness: the reason my cup of co ee does not jump is that the sum of all of its moving particles becomes smooth. Likewise, you reach certainties by adding up small Gaussian uncertainties: this is the law of large numbers. The Gaussian is not self-similar, and that is why my co ee cup does not jump on my desk. Now, consider a trip up a mountain. No matter how high you go on the surface of the earth, it will remain jagged. This is even true at a height of 30,000 feet. When you are ying above the Alps, you will still see jagged mountains in place of small stones. So some surfaces are not from Mediocristan, and changing the resolution does not make them much smoother. (Note that this e ect only disappears when you go up to more extreme heights. Our planet looks smooth to an observer from space, but this is because it is too small. If it were a bigger planet, then it would have mountains that would dwarf the Himalayas, and it would require observation from a greater distance for it to look smooth. Likewise, if the planet had a larger population, even maintaining the same average wealth, we would be likely to nd someone whose net worth would vastly surpass that of Bill Gates.) Figures 11 and 12 illustrate the above point: an observer looking at the rst picture might think that a lens cap has fallen on the ground. Recall our brief discussion of the coast of Britain. If you look at it from an airplane, its contours are not so di erent from the contours you see on the shore. The change in scaling does not alter the shapes or their degree of smoothness. Pearls to Swine What does fractal geometry have to do with the distribution of wealth, the size of cities, returns in the nancial markets, the number of casualties in war, or the size of planets? Let us connect the dots. The key here is that the fractal has numerical or statistical measures that are (somewhat) preserved across scales—the ratio is the same, unlike the Gaussian. Another view of such self-similarity is presented in Figure 13. As we saw in Chapter 15, the superrich are similar to the rich, only richer—wealth is scale independent, or, more precisely, of unknown scale dependence. In the 1960s Mandelbrot presented his ideas on the prices of commodities and nancial securities to the economics establishment, and the nancial economists got all excited. In 1963 the then dean of the University of Chicago Graduate School of Business, George Shultz, o ered him a professorship. This is the same George Shultz who later became Ronald Reagan’s secretary of state. FIGURE 11: Apparently, a lens cap has been dropped on the ground. Now turn the page. Shultz called him one evening to rescind the o er. At the time of writing, forty-four years later, nothing has happened in economics and social science statistics—except for some cosmetic ddling that treats the world as if we were subject only to mild randomness—and yet Nobel medals were being distributed. Some papers were written o ering “evidence” that Mandelbrot was wrong by people who do not get the central argument of this book—you can always produce data “corroborating” that the underlying process is Gaussian by nding periods that do not have rare events, just like you can nd an afternoon during which no one killed anyone and use it as “evidence” of honest behavior. I will repeat that, because of the asymmetry with induction, just as it is easier to reject innocence than accept it, it is easier to reject a bell curve than accept it; conversely, it is more di cult to reject a fractal than to accept it. Why? Because a single event can destroy the argument that we face a Gaussian bell curve. In sum, four decades ago, Mandelbrot gave pearls to economists and résumé-building philistines, which they rejected because the ideas were too good for them. It was, as the saying goes, margaritas ante porcos, pearls before swine. FIGURE 12: The object is not in fact a lens cap. These two photos illustrate scale invariance: the terrain is fractal. Compare it to man-made objects such as a car or a house. Source: Professor Stephen W. Wheatcraft, University of Nevada, Reno. In the rest of this chapter I will explain how I can endorse Mandelbrotian fractals as a representation of much of randomness without necessarily accepting their precise use. Fractals should be the default, the approximation, the framework. They do not solve the Black Swan problem and do not turn all Black Swans into predictable events, but they signi cantly mitigate the Black Swan problem by making such large events conceivable. (It makes them gray. Why gray? Because only the Gaussian give you certainties. More on that, later.) THE LOGIC OF FRACTAL RANDOMNESS (WITH A WARNING)* I have shown in the wealth lists in Chapter 15 the logic of a fractal distribution: if wealth doubles from 1 million to 2 million, the incidence of people with at least that much money is cut in four, which is an exponent of two. If the exponent were one, then the incidence of that wealth or more would be cut in two. The exponent is called the “power” (which is why some people use the term power law). Let us call the number of occurrences higher than a certain level an “exceedance”—an exceedance of two million is the number of persons with wealth more than two million. One main property of these fractals (or another way to express their main property, scalability) is that the ratio of two exceedances* is going to be the ratio of the two numbers to the negative power of the power exponent. Let us illustrate this. Say that you “think” that only 96 books a year will sell more than 250,000 copies (which is what happened last year), and that you “think” that the exponent is around 1.5. You can extrapolate to estimate that around 34 books will sell more than 500,000 copies—simply 96 times (500,000/250,000)−1.5. We can continue, and note that around 12 books should sell more than a million copies, here 96 times (1,000,000/250,000)−1.5. FIGURE 13: THE PURE FRACTAL STATISTICAL MOUNTAIN The degree of inequality will be the same in all sixteen subsections of the graph. In the Gaussian world, disparities in wealth (or any other quantity) decrease when you look at the upper end—so billionaires should be more equal in relation to one another than millionaires are, and millionaires more equal in relation to one another than the middle class. This lack of equality at all wealth levels, in a nutshell, is statistical self-similarity. TABLE 2: ASSUMED EXPONENTS FOR VARIOUS PHENOMENA* Phenomenon Assumed Exponent (vague approximation) Frequency of use of words 1.2 Number of hits on websites 1.4 Number of books sold in the U.S. 1.5 Telephone calls received 1.22 Magnitude of earthquakes 2.8 Diameter of moon craters 2.14 Intensity of solar ares 0.8 Intensity of wars 0.8 Net worth of Americans 1.1 Number of persons per family name 1 Population of U.S. cities 1.3 Market moves 3 (or lower) Company size 1.5 People killed in terrorist attacks 2 (but possibly a much lower exponent) * Source: M.E.J. Newman (2005) and the author’s own calculations. Let me show the di erent measured exponents for a variety of phenomena. Let me tell you upfront that these exponents mean very little in terms of numerical precision. We will see why in a minute, but just note for now that we do not observe these parameters; we simply guess them, or infer them for statistical information, which makes it hard at times to know the true parameters—if it in fact exists. Let us rst examine the practical consequences of an exponent. TABLE 3: THE MEANING OF THE EXPONENT Exponent Share of the top 1% Share of the top 20% 1 99.99%* 99.99% 1.1 66% 86% 1.2 47% 76% 1.3 34% 69% 1.4 27% 63% 1.5 22% 58% 2 10% 45% 2.5 6% 38% 3 4.6% 34% Table 3 illustrates the impact of the highly improbable. It shows the contributions of the top 1 percent and 20 percent to the total. The lower the exponent, the higher those contributions. But look how sensitive the process is: between 1.1 and 1.3 you go from 66 percent of the total to 34 percent. Just a 0.2 di erence in the exponent changes the result dramatically—and such a di erence can come from a simple measurement error. This di erence is not trivial: just consider that we have no precise idea what the exponent is because we cannot measure it directly. All we do is estimate from past data or rely on theories that allow for the building of some model that would give us some idea—but these models may have hidden weaknesses that prevent us from blindly applying them to reality. So keep in mind that the 1.5 exponent is an approximation, that it is hard to compute, that you do not get it from the gods, at least not easily, and that you will have a monstrous sampling error. You will observe that the number of books selling above a million copies is not always going to be 8—It could be as high as 20, or as low as 2. More signi cantly, this exponent begins to apply at some number called “crossover,” and addresses numbers larger than this crossover. It may start at 200,000 books, or perhaps only 400,000 books. Likewise, wealth has di erent properties before, say, $600 million, when inequality grows, than it does below such a number. How do you know where the crossover point is? This is a problem. My colleagues and I worked with around 20 million pieces of nancial data. We all had the same data set, yet we never agreed on exactly what the exponent was in our sets. We knew the data revealed a fractal power law, but we learned that one could not produce a precise number. But what we did know—that the distribution is scalable and fractal—was su cient for us to operate and make decisions. The Problem of the Upper Bound Some people have researched and accepted the fractal “up to a point.” They argue that wealth, book sales, and market returns all have a certain level when things stop being fractal. “Truncation” is what they propose. I agree that there is a level where fractality might stop, but where? Saying that there is an upper limit but I don’t know how high it is, and saying there is no limit carry the same consequences in practice. Proposing an upper limit is highly unsafe. You may say, Let us cap wealth at $150 billion in our analyses. Then someone else might say, Why not $151 billion? Or why not $152 billion? We might as well consider that the variable is unlimited. Beware the Precision I have learned a few tricks from experience: whichever exponent I try to measure will be likely to be overestimated (recall that a higher exponent implies a smaller role for large deviations)—what you see is likely to be less Black Swannish than what you do not see. I call this the masquerade problem. Let’s say I generate a process that has an exponent of 1.7. You do not see what is inside the engine, only the data coming out. If I ask you what the exponent is, odds are that you will compute something like 2.4. You would do so even if you had a million data points. The reason is that it takes a long time for some fractal processes to reveal their properties, and you underestimate the severity of the shock. Sometimes a fractal can make you believe that it is Gaussian, particularly when the cutpoint starts at a high number. With fractal distributions, extreme deviations of that kind are rare enough to smoke you: you don’t recognize the distribution as fractal. The Water Puddle Revisited As you have seen, we have trouble knowing the parameters of whichever model we assume runs the world. So with Extremistan, the problem of induction pops up again, this time even more signi cantly than at any previous time in this book. Simply, if a mechanism is fractal it can deliver large values; therefore the incidence of large deviations is possible, but how possible, how often they should occur, will be hard to know with any precision. This is similar to the water puddle problem: plenty of ice cubes could have generated it. As someone who goes from reality to possible explanatory models, I face a completely di erent spate of problems from those who do the opposite. I have just read three “popular science” books that summarize the research in complex systems: Mark Buchanan’s Ubiquity, Philip Ball’s Critical Mass, and Paul Ormerod’s Why Most Things Fail. These three authors present the world of social science as full of power laws, a view with which I most certainly agree. They also claim that there is universality of many of these phenomena, that there is a wonderful similarity between various processes in nature and the behavior of social groups, which I agree with. They back their studies with the various theories on networks and show the wonderful correspondence between the so-called critical phenomena in natural science and the self-organization of social groups. They bring together processes that generate avalanches, social contagions, and what they call informational cascades, which I agree with. Universality is one of the reasons physicists nd power laws associated with critical points particularly interesting. There are many situations, both in dynamical systems theory and statistical mechanics, where many of the properties of the dynamics around critical points are independent of the details of the underlying dynamical system. The exponent at the critical point may be the same for many systems in the same group, even though many other aspects of the system are di erent. I almost agree with this notion of universality. Finally, all three authors encourage us to apply techniques from statistical physics, avoiding econometrics and Gaussian-style nonscalable distributions like the plague, and I couldn’t agree more. But all three authors, by producing, or promoting precision, fall into the trap of not di erentiating between the forward and the backward processes (between the problem and the inverse problem)—to me, the greatest scienti c and epistemological sin. They are not alone; nearly everyone who works with data but doesn’t make decisions on the basis of these data tends to be guilty of the same sin, a variation of the narrative fallacy. In the absence of a feedback process you look at models and think that they con rm reality. I believe in the ideas of these three books, but not in the way they are being used—and certainly not with the precision the authors ascribe to them. As a matter of fact, complexity theory should make us more suspicious of scienti c claims of precise models of reality. It does not make all the swans white; that is predictable: it makes them gray, and only gray.* As I have said earlier, the world, epistemologically, is literally a di erent place to a bottom-up empiricist. We don’t have the luxury of sitting down to read the equation that governs the universe; we just observe data and make an assumption about what the real process might be, and “calibrate” by adjusting our equation in accordance with additional information. As events present themselves to us, we compare what we see to what we expected to see. It is usually a humbling process, particularly for someone aware of the narrative fallacy, to discover that history runs forward, not backward. As much as one thinks that businessmen have big egos, these people are often humbled by reminders of the di erences between decision and results, between precise models and reality. What I am talking about is opacity, incompleteness of information, the invisibility of the generator of the world. History does not reveal its mind to us—we need to guess what’s inside of it. From Representation to Reality The above idea links all the parts of this book. While many study psychology, mathematics, or evolutionary theory and look for ways to take it to the bank by applying their ideas to business, I suggest the exact opposite: study the intense, uncharted, humbling uncertainty in the markets as a means to get insights about the nature of randomness that is applicable to psychology, probability, mathematics, decision theory, and even statistical physics. You will see the sneaky manifestations of the narrative fallacy, the ludic fallacy, and the great errors of Platonicity, of going from representation to reality. When I rst met Mandelbrot I asked him why an established scientist like him who should have more valuable things to do with his life would take an interest in such a vulgar topic as nance. I thought that nance and economics were just a place where one learned from various empirical phenomena and lled up one’s bank account with f*** you cash before leaving for bigger and better things. Mandelbrot’s answer was, “Data, a gold mine of data.” Indeed, everyone forgets that he started in economics before moving on to physics and the geometry of nature. Working with such abundant data humbles us; it provides the intuition of the following error: traveling the road between representation and reality in the wrong direction. The problem of the circularity of statistics (which we can also call the statistical regress argument) is as follows. Say you need past data to discover whether a probability distribution is Gaussian, fractal, or something else. You will need to establish whether you have enough data to back up your claim. How do we know if we have enough data? From the probability distribution —a distribution does tell you whether you have enough data to “build con dence” about what you are inferring. If it is a Gaussian bell curve, then a few points will su ce (the law of large numbers once again). And how do you know if the distribution is Gaussian? Well, from the data. So we need the data to tell us what the probability distribution is, and a probability distribution to tell us how much data we need. This causes a severe regress argument. This regress does not occur if you assume beforehand that the distribution is Gaussian. It happens that, for some reason, the Gaussian yields its properties rather easily. Extremistan distributions do not do so. So selecting the Gaussian while invoking some general law appears to be convenient. The Gaussian is used as a default distribution for that very reason. As I keep repeating, assuming its application beforehand may work with a small number of elds such as crime statistics, mortality rates, matters from Mediocristan. But not for historical data of unknown attributes and not for matters from Extremistan. Now, why aren’t statisticians who work with historical data aware of this problem? First, they do not like to hear that their entire business has been canceled by the problem of induction. Second, they are not confronted with the results of their predictions in rigorous ways. As we saw with the Makridakis competition, they are grounded in the narrative fallacy, and they do not want to hear it. ONCE AGAIN, BEWARE THE FORECASTERS Let me take the problem one step higher up. As I mentioned earlier, plenty of fashionable models attempt to explain the genesis of Extremistan. In fact, they are grouped into two broad classes, but there are occasionally more approaches. The rst class includes the simple rich-get-richer (or big-get- bigger) style model that is used to explain the lumping of people around cities, the market domination of Microsoft and VHS (instead of Apple and Betamax), the dynamics of academic reputations, etc. The second class concerns what are generally called “percolation models,” which address not the behavior of the individual, but rather the terrain in which he operates. When you pour water on a porous surface, the structure of that surface matters more than does the liquid. When a grain of sand hits a pile of other grains of sand, how the terrain is organized is what determines whether there will be an avalanche. Most models, of course, attempt to be precisely predictive, not just descriptive; I nd this infuriating. They are nice tools for illustrating the genesis of Extremistan, but I insist that the “generator” of reality does not appear to obey them closely enough to make them helpful in precise forecasting. At least to judge by anything you nd in the current literature on the subject of Extremistan. Once again we face grave calibration problems, so it would be a great idea to avoid the common mistakes made while calibrating a nonlinear process. Recall that nonlinear processes have greater degrees of freedom than linear ones (as we saw in Chapter 11), with the implication that you run a great risk of using the wrong model. Yet once in a while you run into a book or articles advocating the application of models from statistical physics to reality. Beautiful books like Philip Ball’s illustrate and inform, but they should not lead to precise quantitative models. Do not take them at face value. But let us see what we can take home from these models. Once Again, a Happy Solution First, in assuming a scalable, I accept that an arbitrarily large number is possible. In other words, inequalities should not stop above some known maximum bound. Say that the book The Da Vinci Code sold around 60 million copies. (The Bible sold about a billion copies but let’s ignore it and limit our analysis to lay books written by individual authors.) Although we have never known a lay book to sell 200 million copies, we can consider that the possibility is not zero. It’s small, but it’s not zero. For every three Da Vinci Code–style bestsellers, there might be one superbestseller, and though one has not happened so far, we cannot rule it out. And for every fteen Da Vinci Codes there will be one superbestseller selling, say, 500 million copies. Apply the same logic to wealth. Say the richest person on earth is worth $50 billion. There is a nonnegligible probability that next year someone with $100 billion or more will pop out of nowhere. For every three people with more than $50 billion, there could be one with $100 billion or more. There is a much smaller probability of there being someone with more than $200 billion—one third of the previous probability, but nevertheless not zero. There is even a minute, but not zero probability of there being someone worth more than $500 billion. This tells me the following: I can make inferences about things that I do not see in my data, but these things should still belong to the realm of possibilities. There is an invisible bestseller out there, one that is absent from the past data but that you need to account for. Recall my point in Chapter 13: it makes investment in a book or a drug better than statistics on past data might suggest. But it can make stock market losses worse than what the past shows. Wars are fractal in nature. A war that kills more people than the devastating Second World War is possible—not likely, but not a zero probability, although such a war has never happened in the past. Second, I will introduce an illustration from nature that will help to make the point about precision. A mountain is somewhat similar to a stone: it has an a nity with a stone, a family resemblance, but it is not identical. The word to describe such resemblances is self-affine, not the precise self-similar, but Mandelbrot had trouble communicating the notion of a nity, and the term self-similar spread with its connotation of precise resemblance rather than family resemblance. As with the mountain and the stone, the distribution of wealth above $1 billion is not exactly the same as that below $1 billion, but the two distributions have “a nity.” Third, I said earlier that there have been plenty of papers in the world of econophysics (the application of statistical physics to social and economic phenomena) aiming at such calibration, at pulling numbers from the world of phenomena. Many try to be predictive. Alas, we are not able to predict “transitions” into crises or contagions. My friend Didier Sornette attempts to build predictive models, which I love, except that I can not use them to make predictions—but please don’t tell him; he might stop building them. That I can’t use them as he intends does not invalidate his work, it just makes the interpretations require broad-minded thinking, unlike models in conventional economics that are fundamentally awed. We may be able to do well with some of Sornette’s phenomena, but not all. WHERE IS THE GRAY SWAN? I have written this entire book about the Black Swan. This is not because I am in love with the Black Swan; as a humanist, I hate it. I hate most of the unfairness and damage it causes. Thus I would like to eliminate many Black Swans, or at least to mitigate their e ects and be protected from them. Fractal randomness is a way to reduce these surprises, to make some of the swans appear possible, so to speak, to make us aware of their consequences, to make them gray. But fractal randomness does not yield precise answers. The bene ts are as follows. If you know that the stock market can crash, as it did in 1987, then such an event is not a Black Swan. The crash of 1987 is not an outlier if you use a fractal with an exponent of three. If you know that biotech companies can deliver a megablockbuster drug, bigger than all we’ve had so far, then it won’t be a Black Swan, and you will not be surprised, should that drug appear. Thus Mandelbrot’s fractals allow us to account for a few Black Swans, but not all. I said earlier that some Black Swans arise because we ignore sources of randomness. Others arise when we overestimate the fractal exponent. A gray swan concerns modelable extreme events, a black swan is about unknown unknowns. I sat down and discussed this with the great man, and it became, as usual, a linguistic game. In Chapter 9 I presented the distinction economists make between Knightian uncertainty (incomputable) and Knightian risk (computable); this distinction cannot be so original an idea to be absent in our vocabulary, and so we looked for it in French. Mandelbrot mentioned one of his friends and prototypical heroes, the aristocratic mathematician Marcel- Paul Schützenberger, a ne erudite who (like this author) was easily bored and could not work on problems beyond their point of diminishing returns. Schützenberger insisted on the clear-cut distinction in the French language between hasard and fortuit. Hasard, from the Arabic az-zahr, implies, like alea, dice—tractable randomness; fortuit is my Black Swan—the purely accidental and unforeseen. We went to the Petit Robert dictionary; the distinction e ectively exists there. Fortuit seems to correspond to my epistemic opacity, l’imprévu et non quantifiable; hasard to the more ludic type of uncertainty that was proposed by the Chevalier de Méré in the early gambling literature. Remarkably, the Arabs may have introduced another word to the business of uncertainty: rizk, meaning property. I repeat: Mandelbrot deals with gray swans; I deal with the Black Swan. So Mandelbrot domesticated many of my Black Swans, but not all of them, not completely. But he shows us a glimmer of hope with his method, a way to start thinking about the problems of uncertainty. You are indeed much safer if you know where the wild animals are. * The nontechnical reader can skip from here until the end of the chapter. * By using symmetry we could also examine the incidences below the number. * Clearly, you do not observe 100 percent in a finite sample. * The defense mechanism when you question their work is to say that they “do science, not philosophy” and to berate my approach of worrying about model errors. This is a common trap: people think that science is about formulating predictions (even when wrong). To me, science is about how not to be a sucker. Chapter Seventeen LOCKE’S MADMEN, OR BELL CURVES IN THE WRONG PLACES* What?—Anyone can become president—Alfred Nobel’s legacy—Those medieval days I have in my house two studies: one real, with interesting books and literary material; the other nonliterary, where I do not enjoy working, where I relegate matters prosaic and narrowly focused. In the nonliterary study is a wall full of books on statistics and the history of statistics, books I never had the fortitude to burn or throw away; though I nd them largely useless outside of their academic applications (Carneades, Cicero, and Foucher know a lot more about probability than all these pseudosophisticated volumes). I cannot use them in class because I promised myself never to teach trash, even if dying of starvation. Why can’t I use them? Not one of these books deals with Extremistan. Not one. The few books that do are not by statisticians but by statistical physicists. We are teaching people methods from Mediocristan and turning them loose in Extremistan. It is like developing a medicine for plants and applying it to humans. It is no wonder that we run the biggest risk of all: we handle matters that belong to Extremistan, but treated as if they belonged to Mediocristan, as an “approximation.” Several hundred thousand students in business schools and social science departments from Singapore to Urbana-Champaign, as well as people in the business world, continue to study “scienti c” methods, all grounded in the Gaussian, all embedded in the ludic fallacy. This chapter examines disasters stemming from the application of phony mathematics to social science. The real topic might be the dangers to our society brought about by the Swedish academy that awards the Nobel Prize. Only Fifty Years Let us return to the story of my business life. Look at the graph in Figure 14. In the last fty years, the ten most extreme days in the nancial markets represent half the returns. Ten days in fty years. Meanwhile, we are mired in chitchat. Clearly, anyone who wants more than the high number of six sigma as proof that markets are from Extremistan needs to have his head examined. Dozens of papers show the inadequacy of the Gaussian family of distributions and the scalable nature of markets. Recall that, over the years, I myself have run statistics backward and forward on 20 million pieces of data that made me despise anyone talking about markets in Gaussian terms. But people have a hard time making the leap to the consequences of this knowledge. The strangest thing is that people in business usually agree with me when they listen to me talk or hear me make my case. But when they go to the o ce the next day they revert to the Gaussian tools so entrenched in their habits. Their minds are domain-dependent, so they can exercise critical thinking at a conference while not doing so in the o ce. Furthermore, the Gaussian tools give them numbers, which seem to be “better than nothing.” The resulting measure of future uncertainty satis es our ingrained desire to simplify even if that means squeezing into one single number matters that are too rich to be described that way. The Clerks’ Betrayal I ended Chapter 1 with the stock market crash of 1987, which allowed me to aggressively pursue my Black Swan idea. Right after the crash, when I stated that those using sigmas (i.e., standard deviations) as a measure of the degree of risk and randomness were charlatans, everyone agreed with me. If the world of nance were Gaussian, an episode such as the crash (more than twenty standard deviations) would take place every several billion lifetimes of the universe (look at the height example in Chapter 15). According to the circumstances of 1987, people accepted that rare events take place and are the main source of uncertainty. They were just unwilling to give up on the Gaussian as a central measurement tool—“Hey, we have nothing else.” People want a number to anchor on. Yet the two methods are logically incompatible. FIGURE 14 By removing the ten biggest one-day moves from the U.S. stock market over the past fty years, we see a huge di erence in returns—and yet conventional nance sees these one-day jumps as mere anomalies. (This is only one of many such tests. While it is quite convincing on a casual read, there are many more-convincing ones from a mathematical standpoint, such as the incidence of 10 sigma events.) Unbeknownst to me, 1987 was not the rst time the idea of the Gaussian was shown to be lunacy. Mandelbrot proposed the scalable to the economics establishment around 1960, and showed them how the Gaussian curve did not t prices then. But after they got over their excitement, they realized that they would have to relearn their trade. One of the in uential economists of the day, the late Paul Cootner, wrote, “Mandelbrot, like Prime Minister Churchill before him, promised us not utopia, but blood, sweat, toil, and tears. If he is right, almost all our statistical tools are obsolete [or] meaningless.” I propose two corrections to Cootner’s statement. First, I would replace almost all with all. Second, I disagree with the blood and sweat business. I nd Mandelbrot’s randomness considerably easier to understand than the conventional statistics. If you come fresh to the business, do not rely on the old theoretical tools, and do not have a high expectation of certainty. Anyone Can Become President And now a brief history of the “Nobel” Prize in economics, which was established by the Bank of Sweden in honor of Alfred Nobel, who may be, according to his family who wants the prize abolished, now rolling in his grave with disgust. An activist family member calls the prize a public relations coup by economists aiming to put their eld on a higher footing than it deserves. True, the prize has gone to some valuable thinkers, such as the empirical psychologist Daniel Kahneman and the thinking economist Friedrich Hayek. But the committee has gotten into the habit of handing out Nobel Prizes to those who “bring rigor” to the process with pseudoscience and phony mathematics. After the stock market crash, they rewarded two theoreticians, Harry Markowitz and William Sharpe, who built beautifully Platonic models on a Gaussian base, contributing to what is called Modern Portfolio Theory. Simply, if you remove their Gaussian assumptions and treat prices as scalable, you are left with hot air. The Nobel Committee could have tested the Sharpe and Markowitz models—they work like quack remedies sold on the Internet —but nobody in Stockholm seems to have thought of it. Nor did the committee come to us practitioners to ask us our opinions; instead it relied on an academic vetting process that, in some disciplines, can be corrupt all the way to the marrow. After that award I made a prediction: “In a world in which these two get the Nobel, anything can happen. Anyone can become president.” So the Bank of Sweden and the Nobel Academy are largely responsible for giving credence to the use of the Gaussian Modern Portfolio Theory as institutions have found it a great cover-your-behind approach. Software vendors have sold “Nobel crowned” methods for millions of dollars. How could you go wrong using it? Oddly enough, everyone in the business world initially knew that the idea was a fraud, but people get used to such methods. Alan Greenspan, the chairman of the Federal Reserve bank, supposedly blurted out, “I’d rather have the opinion of a trader than a mathematician.” Meanwhile, the Modern Portfolio Theory started spreading. I will repeat the following until I am hoarse: it is contagion that determines the fate of a theory in social science, not its validity. I only realized later that Gaussian-trained nance professors were taking over business schools, and therefore MBA programs, and producing close to a hundred thousand students a year in the United States alone, all brainwashed by a phony portfolio theory. No empirical observation could halt the epidemic. It seemed better to teach students a theory based on the Gaussian than to teach them no theory at all. It looked more “scienti c” than giving them what Robert C. Merton (the son of the sociologist Robert K. Merton we discussed earlier) called the “anecdote.” Merton wrote that before portfolio theory, nance was “a collection of anecdotes, rules of thumb, and manipulation of accounting data.” Portfolio theory allowed “the subsequent evolution from this conceptual potpourri to a rigorous economic theory.” For a sense of the degree of intellectual seriousness involved, and to compare neoclassical economics to a more honest science, consider this statement from the nineteenth-century father of modern medicine, Claude Bernard: “Facts for now, but with scienti c aspirations for later.” You should send economists to medical school. So the Gaussian* pervaded our business and scienti c cultures, and terms such as sigma, variance, standard deviation, correlation, R square, and the eponymous Sharpe ratio, all directly linked to it, pervaded the lingo. If you read a mutual fund prospectus, or a description of a hedge fund’s exposure, odds are that it will supply you, among other information, with some quantitative summary claiming to measure “risk.” That measure will be based on one of the above buzzwords derived from the bell curve and its kin. Today, for instance, pension funds’ investment policy and choice of funds are vetted by “consultants” who rely on portfolio theory. If there is a problem, they can claim that they relied on standard scienti c method. More Horror Things got a lot worse in 1997. The Swedish academy gave another round of Gaussian-based Nobel Prizes to Myron Scholes and Robert C. Merton, who had improved on an old mathematical formula and made it compatible with the existing grand Gaussian general nancial equilibrium theories— hence acceptable to the economics establishment. The formula was now “useable.” It had a list of long forgotten “precursors,” among whom was the mathematician and gambler Ed Thorp, who had authored the bestselling Beat the Dealer, about how to get ahead in blackjack, but somehow people believe that Scholes and Merton invented it, when in fact they just made it acceptable. The formula was my bread and butter. Traders, bottom-up people, know its wrinkles better than academics by dint of spending their nights worrying about their risks, except that few of them could express their ideas in technical terms, so I felt I was representing them. Scholes and Merton made the formula dependent on the Gaussian, but their “precursors” subjected it to no such restriction.* The postcrash years were entertaining for me, intellectually. I attended conferences in nance and mathematics of uncertainty; not once did I nd a speaker, Nobel or no Nobel, who understood what he was talking about when it came to probability, so I could freak them out with my questions. They did “deep work in mathematics,” but when you asked them where they got their probabilities, their explanations made it clear that they had fallen for the ludic fallacy—there was a strange cohabitation of technical skills and absence of understanding that you nd in idiot savants. Not once did I get an intelligent answer or one that was not ad hominem. Since I was questioning their entire business, it was understandable that I drew all manner of insults: “obsessive,” “commercial,” “philosophical,” “essayist,” “idle man of leisure,” “repetitive,” “practitioner” (this is an insult in academia), “academic” (this is an insult in business). Being on the receiving end of angry insults is not that bad; you can get quickly used to it and focus on what is not said. Pit traders are trained to handle angry rants. If you work in the chaotic pits, someone in a particularly bad mood from losing money might start cursing at you until he injures his vocal cords, then forget about it and, an hour later, invite you to his Christmas party. So you become numb to insults, particularly if you teach yourself to imagine that the person uttering them is a variant of a noisy ape with little personal control. Just keep your composure, smile, focus on analyzing the speaker not the message, and you’ll win the argument. An ad hominem attack against an intellectual, not against an idea, is highly attering. It indicates that the person does not have anything intelligent to say about your message. The psychologist Philip Tetlock (the expert buster in Chapter 10), after listening to one of my talks, reported that he was struck by the presence of an acute state of cognitive dissonance in the audience. But how people resolve this cognitive tension, as it strikes at the core of everything they have been taught and at the methods they practice, and realize that they will continue to practice, can vary a lot. It was symptomatic that almost all people who attacked my thinking attacked a deformed version of it, like “it is all random and unpredictable” rather than “it is largely random,” or got mixed up by showing me how the bell curve works in some physical domains. Some even had to change my biography. At a panel in Lugano, Myron Scholes once got in to a state of rage, and went after a transformed version of my ideas. I could see pain in his face. Once, in Paris, a prominent member of the mathematical establishment, who invested part of his life on some minute sub-sub-property of the Gaussian, blew a fuse—right when I showed empirical evidence of the role of Black Swans in markets. He turned red with anger, had di culty breathing, and started hurling insults at me for having desecrated the institution, lacking pudeur (modesty); he shouted “I am a member of the Academy of Science!” to give more strength to his insults. (The French translation of my book was out of stock the next day.) My best episode was when Steve Ross, an economist perceived to be an intellectual far superior to Scholes and Merton, and deemed a formidable debater, gave a rebuttal to my ideas by signaling small errors or approximations in my presentation, such as “Markowitz was not the rst to …” thus certifying that he had no answer to my main point. Others who had invested much of their lives in these ideas resorted to vandalism on the Web. Economists often invoke a strange argument by Milton Friedman that states that models do not have to have realistic assumptions to be acceptable—giving them license to produce severely defective mathematical representations of reality. The problem of course is that these Gaussianizations do not have realistic assumptions and do not produce reliable results. They are neither realistic nor predictive. Also note a mental bias I encounter on the occasion: people mistake an event with a small probability, say, one in twenty years for a periodically occurring one. They think that they are safe if they are only exposed to it for ten years. I had trouble getting the message about the di erence between Mediocristan and Extremistan through—many arguments presented to me were about how society has done well with the bell curve—just look at credit bureaus, etc. The only comment I found unacceptable was, “You are right; we need you to remind us of the weakness of these methods, but you cannot throw the baby out with the bath water,” meaning that I needed to accept their reductive Gaussian distribution while also accepting that large deviations could occur—they didn’t realize the incompatibility of the two approaches. It was as if one could be half dead. Not one of these users of portfolio theory in twenty years of debates, explained how they could accept the Gaussian framework as well as large deviations. Not one. Confirmation Along the way I saw enough of the con rmation error to make Karl Popper stand up with rage. People would nd data in which there were no jumps or extreme events, and show me a “proof” that one could use the Gaussian. This was exactly like my example of the “proof” that O. J. Simpson is not a killer in Chapter 5. The entire statistical business confused absence of proof with proof of absence. Furthermore, people did not understand the elementary asymmetry involved: you need one single observation to reject the Gaussian, but millions of observations will not fully con rm the validity of its application. Why? Because the Gaussian bell curve disallows large deviations, but tools of Extremistan, the alternative, do not disallow long quiet stretches. I did not know that Mandelbrot’s work mattered outside aesthetics and geometry. Unlike him, I was not ostracized: I got a lot of approval from practitioners and decision makers, though not from their research sta s. But suddenly I got the most unexpected vindication. IT WAS JUST A BLACK SWAN Robert Merton, Jr., and Myron Scholes were founding partners in the large speculative trading rm called Long-Term Capital Management, or LTCM, which I mentioned in Chapter 4. It was a collection of people with top-notch résumés, from the highest ranks of academia. They were considered geniuses. The ideas of portfolio theory inspired their risk management of possible outcomes—thanks to their sophisticated “calculations.” They managed to enlarge the ludic fallacy to industrial proportions. Then, during the summer of 1998, a combination of large events, triggered by a Russian nancial crisis, took place that lay outside their models. It was a Black Swan. LTCM went bust and almost took down the entire nancial system with it, as the exposures were massive. Since their models ruled out the possibility of large deviations, they allowed themselves to take a monstrous amount of risk. The ideas of Merton and Scholes, as well as those of Modern Portfolio Theory, were starting to go bust. The magnitude of the losses was spectacular, too spectacular to allow us to ignore the intellectual comedy. Many friends and I thought that the portfolio theorists would su er the fate of tobacco companies: they were endangering people’s savings and would soon be brought to account for the consequences of their Gaussian-inspired methods. None of that happened. Instead, MBAs in business schools went on learning portfolio theory. And the option formula went on bearing the name Black-Scholes-Merton, instead of reverting to its true owners, Louis Bachelier, Ed Thorp, and others. How to “Prove” Things Merton the younger is a representative of the school of neoclassical economics, which, as we have seen with LTCM, represents most powerfully the dangers of Platoni ed knowledge.* Looking at his methodology, I see the following pattern. He starts with rigidly Platonic assumptions, completely unrealistic—such as the Gaussian probabilities, along with many more equally disturbing ones. Then he generates “theorems” and “proofs” from these. The math is tight and elegant. The theorems are compatible with other theorems from Modern Portfolio Theory, themselves compatible with still other theorems, building a grand theory of how people consume, save, face uncertainty, spend, and project the future. He assumes that we know the likelihood of events. The beastly word equilibrium is always present. But the whole edi ce is like a game that is entirely closed, like Monopoly with all of its rules. A scholar who applies such methodology resembles Locke’s de nition of a madman: someone “reasoning correctly from erroneous premises.” Now, elegant mathematics has this property: it is perfectly right, not 99 percent so. This property appeals to mechanistic minds who do not want to deal with ambiguities. Unfortunately you have to cheat somewhere to make the world t perfect mathematics; and you have to fudge your assumptions somewhere. We have seen with the Hardy quote that professional “pure” mathematicians, however, are as honest as they come. So where matters get confusing is when someone like Merton tries to be mathematical and airtight rather than focus on tness to reality. This is where you learn from the minds of military people and those who have responsibilities in security. They do not care about “perfect” ludic reasoning; they want realistic ecological assumptions. In the end, they care about lives. I mentioned in Chapter 11 how those who started the game of “formal thinking,” by manufacturing phony premises in order to generate “rigorous” theories, were Paul Samuelson, Merton’s tutor, and, in the United Kingdom, John Hicks. These two wrecked the ideas of John Maynard Keynes, which they tried to formalize (Keynes was interested in uncertainty, and complained about the mind-closing certainties induced by models). Other participants in the formal thinking venture were Kenneth Arrow and Gerard Debreu. All four were Nobeled. All four were in a delusional state under the e ect of mathematics—what Dieudonné called “the music of reason,” and what I call Locke’s madness. All of them can be safely accused of having invented an imaginary world, one that lent itself to their mathematics. The insightful scholar Martin Shubik, who held that the degree of excessive abstraction of these models, a few steps beyond necessity, makes them totally unusable, found himself ostracized, a common fate for dissenters.* If you question what they do, as I did with Merton Jr., they will ask for “tight proof.” So they set the rules of the game, and you need to play by them. Coming from a practitioner background in which the principal asset is being able to work with messy, but empirically acceptable, mathematics, I cannot accept a pretense of science. I much prefer a sophisticated craft, focused on tricks, to a failed science looking for certainties. Or could these neoclassical model builders be doing something worse? Could it be that they are involved in what Bishop Huet calls the manufacturing of certainties? TABLE 4: TWO WAYS TO APPROACH RANDOMNESS Skeptical Empiricism and the a- The Platonic Approach Platonic School Interested in what lies outside the Focuses on the inside of the Platonic Platonic fold fold Respect for those who have the guts to “You keep criticizing these models. say “I don’t know” These models are all we have.” Fat Tony Dr. John Thinks of Black Swans as a dominant Thinks of ordinary uctuations as a source of randomness dominant source of randomness, with jumps as an afterthought Bottom-up Top-down Would ordinarily not wear suits Wears dark suits, white shirts; speaks (except to funerals) in a boring tone Prefers to be broadly right Precisely wrong Minimal theory, consides theorizing as Everything needs to t some grand, a disease to resist general socioeconomic model and “the rigor of economic theory;” frowns on the “descriptive” Does not believe that we can easily Built their entire apparatus on the compute probabilities assumptions that we can compute probabilities Model: Sextus Empiricus and the Model: Laplacian mechanics, the school of evidence-based, minimum- world and the economy like a clock theory empirical medicine Develops intuitions from practice, Relies on scienti c papers, goes from goes from observations to books books to practice Not inspired by any science, uses Inspired by physics, relies on abstract messy mathematics and mathematics computational methods Ideas based on skepticism, on the Ideas based on beliefs, on what they unread books in the library think they know Assumes Extremistan as a starting Assumes Mediocristan as a starting point point Sophisticated craft Poor science Seeks to be approximately right across Seeks to be perfectly right in a narrow a broad set of eventualities model, under precise assumptions Let us see. Skeptical empiricism advocates the opposite method. I care about the premises more than the theories, and I want to minimize reliance on theories, stay light on my feet, and reduce my surprises. I want to be broadly right rather than precisely wrong. Elegance in the theories is often indicative of Platonicity and weakness—it invites you to seek elegance for elegance’s sake. A theory is like medicine (or government): often useless, sometimes necessary, always self-serving, and on occasion lethal. So it needs to be used with care, moderation, and close adult supervision. The distinction in the above table between my model modern, skeptical empiricist and what Samuelson’s puppies represent can be generalized across disciplines. I’ve presented my ideas in nance because that’s where I re ned them. Let us now examine a category of people expected to be more thoughtful: the philosophers. * This is a simple illustration of the general point of this book in nance and economics. If you do not believe in applying the bell curve to social variables, and if, like many professionals, you are already convinced that “modern” nancial theory is dangerous junk science, you can safely skip this chapter. * Granted, the Gaussian has been tinkered with, using such methods as complementary “jumps,” stress testing, regime switching, or the elaborate methods known as GARCH, but while these methods represent a good e ort, they fail to address the bell curve’s fundamental aws. Such methods are not scale-invariant. This, in my opinion, can explain the failures of sophisticated methods in real life as shown by the Makridakis competition. * More technically, remember my career as an option professional. Not only does an option on a very long shot bene t from Black Swans, but it bene ts disproportionately from them—something Scholes and Merton’s “formula” misses. The option payo is so powerful that you do not have to be right on the odds: you can be wrong on the probability, but get a monstrously large payo. I’ve called this the “double bubble”: the mispricing of the probability and that of the payo. * I am selecting Merton because I found him very illustrative of academically stamped obscurantism. I discovered Merton’s shortcomings from an angry and threatening seven-page letter he sent me that gave me the impression that he was not too familiar with how we trade options, his very subject matter. He seemed to be under the impression that traders rely on “rigorous” economic theory—as if birds had to study (bad) engineering in order to y. * Medieval medicine was also based on equilibrium ideas when it was top-down and similar to theology. Luckily its practitioners went out of business, as they could not compete with the bottom-up surgeons, ecologically driven former barbers who gained clinical experience, and after whom a-Platonic clinical science was born. If I am alive, today, it is because scholastic top-down medicine went out of business a few centuries ago. Chapter Eighteen THE UNCERTAINTY OF THE PHONY Philosophers in the wrong places—Uncertainty about (mostly) lunch— What I don’t care about—Education and intelligence This nal chapter of Part Three focuses on a major rami cation of the ludic fallacy: how those whose job it is to make us aware of uncertainty fail us and divert us into bogus certainties through the back door. LUDIC FALLACY REDUX I have explained the ludic fallacy with the casino story, and have insisted that the sterilized randomness of games does not resemble randomness in real life. Look again at Figure 7 in Chapter 15. The dice average out so quickly that I can say with certainty that the casino will beat me in the very near long run at, say, roulette, as the noise will cancel out, though not the skills (here, the casino’s advantage). The more you extend the period (or reduce the size of the bets) the more randomness, by virtue of averaging, drops out of these gambling constructs. The ludic fallacy is present in the following chance setups: random walk, dice throwing, coin ipping, the infamous digital “heads or tails” expressed as 0 or 1, Brownian motion (which corresponds to the movement of pollen particles in water), and similar examples. These setups generate a quality of randomness that does not even qualify as randomness—protorandomness would be a more appropriate designation. At their core, all theories built around the ludic fallacy ignore a layer of uncertainty. Worse, their proponents do not know it! One severe application of such focus on small, as opposed to large, uncertainty concerns the hackneyed greater uncertainty principle. Find the Phony The greater uncertainty principle states that in quantum physics, one cannot measure certain pairs of values (with arbitrary precision), such as the position and momentum of particles. You will hit a lower bound of measurement: what you gain in the precision of one, you lose in the other. So there is an incompressible uncertainty that, in theory, will defy science and forever remain an uncertainty. This minimum uncertainty was discovered by Werner Heisenberg in 1927. I nd it ludicrous to present the uncertainty principle as having anything to do with uncertainty. Why? First, this uncertainty is Gaussian. On average, it will disappear—recall that no one person’s weight will signi cantly change the total weight of a thousand people. We may always remain uncertain about the future positions of small particles, but these uncertainties are very small and very numerous, and they average out—for Pluto’s sake, they average out! They obey the law of large numbers we discussed in Chapter 15. Most other types of randomness do not average out! If there is one thing on this planet that is not so uncertain, it is the behavior of a collection of subatomic particles! Why? Because, as I have said earlier, when you look at an object, composed of a collection of particles, the uctuations of the particles tend to balance out. But political, social, and weather events do not have this handy property, and we patently cannot predict them, so when you hear “experts” presenting the problems of uncertainty in terms of subatomic particles, odds are that the expert is a phony. As a matter of fact, this may be the best way to spot a phony. I often hear people say, “Of course there are limits to our knowledge,” then invoke the greater uncertainty principle as they try to explain that “we cannot model everything”—I have heard such types as the economist Myron Scholes say this at conferences. But I am sitting here in New York, in August 2006, trying to go to my ancestral village of Amioun, Lebanon. Beirut’s airport is closed owing to the con ict between Israel and the Shiite militia Hezbollah. There is no published airline schedule that will inform me when the war will end, if it ends. I can’t gure out if my house will be standing, if Amioun will still be on the map—recall that the family house was destroyed once before. I can’t gure out whether the war is going to degenerate into something even more severe. Looking into the outcome of the war, with all my relatives, friends, and property exposed to it, I face true limits of knowledge. Can someone explain to me why I should care about subatomic particles that, anyway, converge to a Gaussian? People can’t predict how long they will be happy with recently acquired objects, how long their marriages will last, how their new jobs will turn out, yet it’s subatomic particles that they cite as “limits of prediction.” They’re ignoring a mammoth standing in front of them in favor of matter even a microscope would not allow them to see. Can Philosophers Be Dangerous to Society? I will go further: people who worry about pennies instead of dollars can be dangerous to society. They mean well, but, invoking my Bastiat argument of Chapter 8, they are a threat to us. They are wasting our studies of uncertainty by focusing on the insigni cant. Our resources (both cognitive and scienti c) are limited, perhaps too limited. Those who distract us increase the risk of Black Swans. This commoditization of the notion of uncertainty as symptomatic of Black Swan blindness is worth discussing further here. Given that people in nance and economics are seeped in the Gaussian to the point of choking on it, I looked for nancial economists with philosophical bents to see how their critical thinking allows them to handle this problem. I found a few. One such person got a PhD in philosophy, then, four years later, another in nance; he published papers in both elds, as well as numerous textbooks in nance. But I was disheartened by him: he seemed to have compartmentalized his ideas on uncertainty so that he had two distinct professions: philosophy and quantitative nance. The problem of induction, Mediocristan, epistemic opacity, or the o ensive assumption of the Gaussian—these did not hit him as true problems. His numerous textbooks drilled Gaussian methods into students’ heads, as though their author had forgotten that he was a philosopher. Then he promptly remembered that he was when writing philosophy texts on seemingly scholarly matters. The same context speci city leads people to take the escalator to the StairMasters, but the philosopher’s case is far, far more dangerous since he uses up our storage for critical thinking in a sterile occupation. Philosophers like to practice philosophical thinking on me-too subjects that other philosophers call philosophy, and they leave their minds at the door when they are outside of these subjects. The Problem of Practice As much as I rail against the bell curve, Platonicity, and the ludic fallacy, my principal problem is not so much with statisticians—after all, these are computing people, not thinkers. We should be far less tolerant of philosophers, with their bureaucratic apparatchiks closing our minds. Philosophers, the watchdogs of critical thinking, have duties beyond those of other professions. HOW MANY WITTGENSTEINS CAN DANCE ON THE HEAD OF A PIN? A number of semishabbily dressed (but thoughtful-looking) people gather in a room, silently looking at a guest speaker. They are all professional philosophers attending the prestigious weekly colloquium at a New York– area university. The speaker sits with his nose drowned in a set of typewritten pages, from which he reads in a monotone voice. He is hard to follow, so I daydream a bit and lose his thread. I can vaguely tell that the discussion revolves around some “philosophical” debate about Martians invading your head and controlling your will, all the while preventing you from knowing it. There seem to be several theories concerning this idea, but the speaker’s opinion di ers from those of other writers on the subject. He spends some time showing where his research on these head-hijacking Martians is unique. After his monologue ( fty- ve minutes of relentless reading of the typewritten material) there is a short break, then another fty- ve minutes of discussion about Martians planting chips and other outlandish conjectures. Wittgenstein is occasionally mentioned (you can always mention Wittgenstein since he is vague enough to always seem relevant). Every Friday, at four P.M., the paychecks of these philosophers will hit their respective bank accounts. A xed proportion of their earnings, about 16 percent on average, will go into the stock market in the form of an automatic investment into the university’s pension plan. These people are professionally employed in the business of questioning what we take for granted; they are trained to argue about the existence of god(s), the de nition of truth, the redness of red, the meaning of meaning, the di erence between the semantic theories of truth, conceptual and nonconceptual representations … Yet they believe blindly in the stock market, and in the abilities of their pension plan manager. Why do they do so? Because they accept that this is what people should do with their savings, because “experts” tell them so. They doubt their own senses, but not for a second do they doubt their automatic purchases in the stock market. This domain dependence of skepticism is no di erent from that of medical doctors (as we saw in Chapter 8). Beyond this, they may believe without question that we can predict societal events, that the Gulag will toughen you a bit, that politicians know more about what is going on than their drivers, that the chairman of the Federal Reserve saved the economy, and so many such things. They may also believe that nationality matters (they always stick “French,” “German,” or “American” in front of a philosopher’s name, as if this has something to do with anything he has to say). Spending time with these people, whose curiosity is focused on regimented on-the-shelf topics, feels sti ing. Where Is Popper When You Need Him? I hope I’ve su ciently drilled home the notion that, as a practitioner, my thinking is rooted in the belief that you cannot go from books to problems, but the reverse, from problems to books. This approach incapacitates much of that career-building verbiage. A scholar should not be a library’s tool for making another library, as in the joke by Daniel Dennett. Of course, what I am saying here has been said by philosophers before, at least by the real ones. The following remark is one reason I have inordinate respect for Karl Popper; it is one of the few quotations in this book that I am not attacking. The degeneration of philosophical schools in its turn is the consequence of the mistaken belief that one can philosophize without having been compelled to philosophize by problems outside philosophy. … Genuine philosophical problems are always rooted outside philosophy and they die if these roots decay. … [emphasis mine] These roots are easily forgotten by philosophers who “study” philosophy instead of being forced into philosophy by the pressure of nonphilosophical problems. Such thinking may explain Popper’s success outside philosophy, particularly with scientists, traders, and decision makers, as well as his relative failure inside of it. (He is rarely studied by his fellow philosophers; they prefer to write essays on Wittgenstein.) Also note that I do not want to be drawn into philosophical debates with my Black Swan idea. What I mean by Platonicity is not so metaphysical. Plenty of people have argued with me about whether I am against “essentialism” (i.e., things that I hold don’t have a Platonic essence), if I believe that mathematics would work in an alternative universe, or some such thing. Let me set the record straight. I am a no-nonsense practitioner; I am not saying that mathematics does not correspond to an objective structure of reality; my entire point is that we are, epistemologically speaking, putting the cart before the horse and, of the space of possible mathematics, risk using the wrong one and being blinded by it. I truly believe that there are some mathematics that work, but that these are not as easily within our reach as it seems to the “con rmators.” The Bishop and the Analyst I am most often irritated by those who attack the bishop but somehow fall for the securities analyst—those who exercise their skepticism against religion but not against economists, social scientists, and phony statisticians. Using the con rmation bias, these people will tell you that religion was horrible for mankind by counting deaths from the Inquisition and various religious wars. But they will not show you how many people were killed by nationalism, social science, and political theory under Stalinism or during the Vietnam War. Even priests don’t go to bishops when they feel ill: their rst stop is the doctor’s. But we stop by the o ces of many pseudo-scientists and “experts” without alternative. We no longer believe in papal infallibility; we seem to believe in the infallibility of the Nobel, though, as we saw in Chapter 17. Easier Than You Think: The Problem of Decision Under Skepticism I have said all along that there is a problem with induction and the Black Swan. In fact, matters are far worse: we may have no less of a problem with phony skepticism. 1. I can’t do anything to stop the sun from nonrising tomorrow (no matter how hard I try), 2. I can’t do anything about whether or not there is an afterlife, 3. I can’t do anything about Martians or demons taking hold of my brain. But I have plenty of ways to avoid being a sucker. It is not much more di cult than that. I conclude Part Three by reiterating that my antidote to Black Swans is precisely to be noncommoditized in my thinking. But beyond avoiding being a sucker, this attitude lends itself to a protocol of how to act—not how to think, but how to convert knowledge into action and gure out what knowledge is worth. Let us examine what to do or not do with this in the concluding section of this book. Chapter Nineteen HALF AND HALF, OR HOW TO GET EVEN WITH THE BLACK SWAN The other half—Remember Apelles—When missing a train can be painful It is now time for a few last words. Half the time I am a hyperskeptic; the other half I hold certainties and can be intransigent about them, with a very stubborn disposition. Of course I am hyperskeptic where others, particularly those I call bildungsphilisters, are gullible, and gullible where others seem skeptical. I am skeptical about con rmation—though only when errors are costly—not about discon rmation. Having plenty of data will not provide con rmation, but a single instance can discon rm. I am skeptical when I suspect wild randomness, gullible when I believe that randomness is mild. Half the time I hate Black Swans, the other half I love them. I like the randomness that produces the texture of life, the positive accidents, the success of Apelles the painter, the potential gifts you do not have to pay for. Few understand the beauty in the story of Apelles; in fact, most people exercise their error avoidance by repressing the Apelles in them. Half the time I am hyperconservative in the conduct of my own a airs; the other half I am hyperaggressive. This may not seem exceptional, except that my conservatism applies to what others call risk taking, and my aggressiveness to areas where others recommend caution. I worry less about small failures, more about large, potentially terminal ones. I worry far more about the “promising” stock market, particularly the “safe” blue chip stocks, than I do about speculative ventures—the former present invisible risks, the latter o er no surprises since you know how volatile they are and can limit your downside by investing smaller amounts. I worry less about advertised and sensational risks, more about the more vicious hidden ones. I worry less about terrorism than about diabetes, less about matters people usually worry about because they are obvious worries, and more about matters that lie outside our consciousness and common discourse (I also have to confess that I do not worry a lot—I try to worry about matters I can do something about). I worry less about embarrassment than about missing an opportunity. In the end this is a trivial decision making rule: I am very aggressive when I can gain exposure to positive Black Swans—when a failure would be of small moment—and very conservative when I am under threat from a negative Black Swan. I am very aggressive when an error in a model can bene t me, and paranoid when the error can hurt. This may not be too interesting except that it is exactly what other people do not do. In nance, for instance, people use imsy theories to manage their risks and put wild ideas under “rational” scrutiny. Half the time I am intellectual, the other half I am a no-nonsense practitioner. I am no-nonsense and practical in academic matters, and intellectual when it comes to practice. Half the time I am shallow, the other half I want to avoid shallowness. I am shallow when it comes to aesthetics; I avoid shallowness in the context of risks and returns. My aestheticism makes me put poetry before prose, Greeks before Romans, dignity before elegance, elegance before culture, culture before erudition, erudition before knowledge, knowledge before intellect, and intellect before truth. But only for matters that are Black Swan free. Our tendency is to be very rational, except when it comes to the Black Swan. Half the people I know call me irreverent (you have read my comments about your local Platoni ed professors), half call me fawning (you have seen my slavish devotion to Huet, Bayle, Popper, Poincaré, Montaigne, Hayek, and others). Half the time I hate Nietzsche, the other half I like his prose. WHEN MISSING A TRAIN IS PAINLESS I once received another piece of life-changing advice, which, unlike the advice I got from a friend in Chapter 3, I nd applicable, wise, and empirically valid. My classmate in Paris, the novelist-to-be Jean-Olivier Tedesco, pronounced, as he prevented me from running to catch a subway, “I don’t run for trains.” Snub your destiny. I have taught myself to resist running to keep on schedule. This may seem a very small piece of advice, but it registered. In refusing to run to catch trains, I have felt the true value of elegance and aesthetics in behavior, a sense of being in control of my time, my schedule, and my life. Missing a train is only painful if you run after it! Likewise, not matching the idea of success others expect from you is only painful if that’s what you are seeking. You stand above the rat race and the pecking order, not outside of it, if you do so by choice. Quitting a high-paying position, if it is your decision, will seem a better payo than the utility of the money involved (this may seem crazy, but I’ve tried it and it works). This is the rst step toward the stoic’s throwing a four- letter word at fate. You have far more control over your life if you decide on your criterion by yourself. Mother Nature has given us some defense mechanisms: as in Aesop’s fable, one of these is our ability to consider that the grapes we cannot (or did not) reach are sour. But an aggressively stoic prior disdain and rejection of the grapes is even more rewarding. Be aggressive; be the one to resign, if you have the guts. It is more di cult to be a loser in a game you set up yourself. In Black Swan terms, this means that you are exposed to the improbable only if you let it control you. You always control what you do; so make this your end. THE END But all these ideas, all this philosophy of induction, all these problems with knowledge, all these wild opportunities and scary possible losses, everything palls in front of the following metaphysical consideration. I am sometimes taken aback by how people can have a miserable day or get angry because they feel cheated by a bad meal, cold co ee, a social rebu , or a rude reception. Recall my discussion in Chapter 8 on the di culty in seeing the true odds of the events that run your own life. We are quick to forget that just being alive is an extraordinary piece of good luck, a remote event, a chance occurrence of monstrous proportions. Imagine a speck of dust next to a planet a billion times the size of the earth. The speck of dust represents the odds in favor of your being born; the huge planet would be the odds against it. So stop sweating the small stu. Don’t be like the ingrate who got a castle as a present and worried about the mildew in the bathroom. Stop looking the gift horse in the mouth—remember that you are a Black Swan. And thank you for reading my book. Epilogue YEVGENIA’S WHITE SWANS Yevgenia Krasnova went into the long hibernation that was necessary for producing a new book. She stayed in New York City, where she found it easiest to nd tranquillity, alone with her text. It was easiest to concentrate after long periods during which she was surrounded by crowds, hoping to run into Nero so she could make a snide remark to him, perhaps humiliate him, possibly win him back. She canceled her e- mail account, switched to writing longhand, since she found it soothing, and hired a secretary to type her text. She spent eight years writing, erasing, correcting, venting her occasional anger at the secretary, interviewing new secretaries, and quietly rewriting. Her apartment was full of smoke, with papers strewn on every surface. Like all artists she remained dissatis ed with the state of completion of her work, yet she felt that she had gone far deeper than with her rst book. She laughed at the public who extolled her earlier work, for she now found it shallow, hurriedly completed, and undistilled. When the new book, which was aptly called The Loop, came out, Yevgenia was wise enough to avoid the press and ignore her reviews, and stayed insulated from the external world. As expected by her publisher, the reviews were laudatory. But, strangely, few were buying. People must be talking about the book without reading it, he thought. Her fans had been waiting for it and talking about it for years. The publisher, who now owned a very large collection of pink glasses and led a amboyant lifestyle, was presently betting the farm on Yevgenia. He had no other hits and none in sight. He needed to score big to pay for his villa in Carpentras in Provence and his dues on the nancial settlement with his estranged wife, as well as to buy a new convertible Jaguar (pink). He had been certain that he had a good shot with Yevgenia’s long-awaited book, and he could not gure out why almost everyone called it a masterpiece yet no one was buying it. A year and a half later, The Loop was e ectively out of print. The publisher, now in severe nancial distress, thought he knew the reason: the book was “too f***ing long!”—Yevgenia should have written a shorter one. After a long but soothing lachrymal episode, Yevgenia thought of the characters in the rainy novels of Georges Simenon and Graham Greene. They lived in a state of numbing and secure mediocrity. Second-rateness had charm, Yevgenia thought, and she had always preferred charm over beauty. So Yevgenia’s second book too was a Black Swan. GLOSSARY Academic libertarian: someone (like myself) who considers that knowledge is subjected to strict rules but not institutional authority, as the interest of organized knowledge is self-perpetuation, not necessarily truth (as with governments). Academia can su er from an acute expert problem (q.v.), producing cosmetic but fake knowledge, particularly in narrative disciplines (q.v.), and can be a main source of Black Swans. Apelles-style strategy: A strategy of seeking gains by collecting positive accidents from maximizing exposure to “good Black Swans.” Barbell strategy: a method that consists of taking both a defensive attitude and an excessively aggressive one at the same time, by protecting assets from all sources of uncertainty while allocating a small portion for high-risk strategies. Bildungsphilister: a philistine with cosmetic, nongenuine culture. Nietzsche used this term to refer to the dogma- prone newspaper reader and opera lover with cosmetic exposure to culture and shallow depth. I extend it to the buzzword-using researcher in nonexperimental elds who lacks in imagination, curiosity, erudition, and culture and is closely centered on his ideas, on his “discipline.” This prevents him from seeing the con icts between his ideas and the texture of the world. Black Swan blindness: the underestimation of the role of the Black Swan, and occasional overestimation of a speci c one. Black Swan ethical problem: Owing to the nonrepeatable aspect of the Black Swan, there is an asymmetry between the rewards of those who prevent and those who cure. Con rmation error (or Platonic con rmation): You look for instances that con rm your beliefs, your construction (or model)—and nd them. Empty-suit problem (or “expert problem”): Some professionals have no di erential abilities from the rest of the population, but for some reason, and against their empirical records, are believed to be experts: clinical psychologists, academic economists, risk “experts,” statisticians, political analysts, nancial “experts,” military analysts, CEOs, et cetera. They dress up their expertise in beautiful language, jargon, mathematics, and often wear expensive suits. Epilogism: A theory-free method of looking at history by accumulating facts with minimal generalization and being conscious of the side e ects of making causal claims. Epistemic arrogance: Measure the di erence between what someone actually knows and how much he thinks he knows. An excess will imply arrogance, a de cit humility. An epistemocrat is someone of epistemic humility, who holds his own knowledge in greatest suspicion. Epistemic opacity: Randomness is the result of incomplete information at some layer. It is functionally indistinguishable from “true” or “physical” randomness. Extremistan: the province where the total can be conceivably impacted by a single observation. Fallacy of silent evidence: Looking at history, we do not see the full story, only the rosier parts of the process. Fooled by randomness: the general confusion between luck and determinism, which leads to a variety of superstitions with practical consequences, such as the belief that higher earnings in some professions are generated by skills when there is a signi cant component of luck in them. Future blindness: our natural inability to take into account the properties of the future—like autism, which prevents one from taking into account the existence of the minds of others. Locke’s madman: someone who makes impeccable and rigorous reasoning from faulty premises—such as Paul Samuelson, Robert Merton the minor, and Gerard Debreu—thus producing phony models of uncertainty that make us vulnerable to Black Swans. Lottery-ticket fallacy: the naïve analogy equating an investment in collecting positive Black Swans to the accumulation of lottery tickets. Lottery tickets are not scalable. Ludic fallacy (or uncertainty of the nerd): the manifestation of the Platonic fallacy in the study of uncertainty; basing studies of chance on the narrow world of games and dice. A-Platonic randomness has an additional layer of uncertainty concerning the rules of the game in real life. The bell curve (Gaussian), or GIF (Great Intellectual Fraud), is the application of the ludic fallacy to randomness. Mandelbrotian Gray Swan: Black Swans that we can somewhat take into account—earthquakes, blockbuster books, stock market crashes—but for which it is not possible to completely gure out the properties and produce precise calculations. Mediocristan: the province dominated by the mediocre, with few extreme successes or failures. No single observation can meaningfully a ect the aggregate. The bell curve is grounded in Mediocristan. There is a qualitative di erence between Gaussians and scalable laws, much like gas and water. Narrative discipline: the discipline that consists in tting a convincing and well-sounding story to the past. Opposed to experimental discipline. Narrative fallacy: our need to t a story or pattern to a series of connected or disconnected facts. The statistical application is data mining. Nerd knowledge: the belief that what cannot be Platonized and studied does not exist at all, or is not worth considering. There even exists a form of skepticism practiced by the nerd. Platonic fold: the place where our Platonic representation enters into contact with reality and you can see the side e ects of models. Platonicity: the focus on those pure, well-de ned, and easily discernible objects like triangles, or more social notions like friendship or love, at the cost of ignoring those objects of seemingly messier and less tractable structures. Probability distribution: the model used to calculate the odds of di erent events, how they are “distributed.” When we say that an event is distributed according to the bell curve, we mean that the Gaussian bell curve can help provide probabilities of various occurrences. Problem of induction: the logical-philosophical extension of the Black Swan problem. Randomness as incomplete information: simply, what I cannot guess is random because my knowledge about the causes is incomplete, not necessarily because the process has truly unpredictable properties. Retrospective distortion: examining past events without adjusting for the forward passage of time. It leads to the illusion of posterior predictability. Reverse-engineering problem: It is easier to predict how an ice cube would melt into a puddle than, looking at a puddle, to guess the shape of the ice cube that may have caused it. This “inverse problem” makes narrative disciplines and accounts (such as histories) suspicious. Round-trip fallacy: the confusion of absence of evidence of Black Swans (or something else) for evidence of absence of Black Swans (or something else). It a ects statisticians and other people who have lost part of their reasoning by solving too many equations. Scandal of prediction: the poor prediction record in some forecasting entities (particularly narrative disciplines) mixed with verbose commentary and a lack of awareness of their own dire past record. Scorn of the abstract: favoring contextualized thinking over more abstract, though more relevant, matters. “The death of one child is a tragedy; the death of a million is a statistic.” Statistical regress argument (or the problem of the circularity of statistics): We need data to discover a probability distribution. How do we know if we have enough? From the probability distribution. If it is a Gaussian, then a few points of data will su ce. How do we know it is a Gaussian? From the data. So we need the data to tell us what probability distribution to assume, and we need a probability distribution to tell us how much data we need. This causes a severe regress argument, which is somewhat shamelessly circumvented by resorting to the Gaussian and its kin. Uncertainty of the deluded: people who tunnel on sources of uncertainty by producing precise sources like the great uncertainty principle, or similar, less consequential matters, to real life; worrying about subatomic particles while forgetting that we can’t predict tomorrow’s crises. I LEARNING FROM MOTHER NATURE, THE OLDEST AND THE WISEST How to make friends among walking people—On becoming a grandmother—The charms of eco-Extremistan—Never small enough —Harvard-Soviet chic I am writing this essay three years after the completion of The Black Swan—which I have kept intact except for a few clarifying footnotes. Since then, I’ve written a dozen “scholarly” papers around some aspects of the Black Swan idea. These are very, very boring to read, since almost all academic papers are made to bore, impress, provide credibility, intimidate even, be presented at meetings, but not to be read except by suckers (or detractors) or, even worse, graduate students. Also, I am making the “what to do next” more salient here—you can take a horse to water and, in addition, you may have to make it drink. So this essay will allow me to go deeper into some points. Like the main text itself, the beginning will be what is called literary, and progressively turn technical. I owe the idea of this book-length essay to Danny Kahneman, toward whom I (and my ideas) have more debt than toward anyone else on this planet. He convinced me that I had obligations to try to make the horse drink. ON SLOW BUT LONG WALKS Over the past three years, my life experienced a bit of change, mostly for the better. Like parties, a book puts you on the envelope of serendipity; it even gets you invited to more parties. During my dark days, I was called a trader in Paris (something extremely vulgaire), a philosopher in London (meaning too theoretical), a prophet in New York (dissingly, because of my then false prophecy), and an economist in Jerusalem (something very materialistic). I now saw myself dealing with the stress of having to live up to the wholly undeserved designations of a prophet in Israel (a very, very ambitious project), a philosophe in France, an economist in London, and a trader in New York (where it is respectable). Such exposure brought hate mail, at least one death threat (by former employees of the bankrupt rm Lehman Brothers*), which I found extremely attering, and, worse than any threat of violence, hourly requests for interviews by Turkish and Brazilian journalists. I had to spend a lot of time writing personalized and courteous notes declining invitations to dinner with suit-wearing current hotshots, suit-wearing archeo-hotshots, suit-wearing proto-hotshots, and the nasty brand of suit-wearing namedroppers. But it also brought some bene ts. I was contacted by like-minded persons, people I would have never dreamed of meeting in the past, or those I did not think existed before, in disciplines completely outside my normal circles, who helped me further my quest with the most unexpected of ideas. I was often reached by people I admired and whose work I knew well, and who became natural collaborators and critics; I will always remember the thrill of getting an unexpected e-mail from Spyros Makridakis of the M-Competition described in Chapter 10, the great debunker of misforecasting, or another one from Jon Elster, the scholar of rare erudition and insights who integrated the wisdom of the ancients into modern social science thinking. I’ve met novelists and philosophical thinkers whose works I had read and admired, like Louis de Bernières, Will Self, John Gray (the philosopher, not the pop psychologist), or Lord Martin Rees; in all four cases I had the peculiar need to pinch myself upon hearing them talking to me about my own book. Then, through a chain of friends of friends, cappuccinos, dessert wines, and security lines at airports, I got to partake of and understand the potency of oral knowledge, as discussions are vastly more powerful than just correspondence. People say things in person they would never put in print. I met Nouriel Roubini (to my knowledge the only professional economist who really predicted the crisis of 2008, and perhaps the only independent thinker in that business). I also found a variety of people I did not know existed, good economists (i.e., with scienti c standards), like Michael Spence and Barkley Rosser. Also Peter Bevelin and Yechezkel Zilber kept feeding me the papers I was looking for without knowing it, the rst in biology, the second in cognitive science—thus they nudged my thinking in the appropriate direction. So I have been dialoguing with many people. My problem is that I found only two persons who can have a conversation during a long walk (and walk slowly): Spyros Makridakis and Yechezkel Zilber. Most people, alas, walk too fast, mistaking walking for exercise, not understanding that walking is to be done slowly, at such a pace that one forgets one is walking—so I need to keep going to Athens (where Spyros lives) in order to indulge in my favorite activity, being a âneur. My Mistakes And of course people will scrutinize the text. After examining messages and reports, I do not feel I need to retract anything in the initial version, or to correct any error (outside of typos and minor factual mistakes), except for two related matters. The rst fault was pointed out to me by Jon Elster. I had written that the narrative fallacy pervades historical analyses, since I believed that there was no such thing as a test of a historical statement by forecasting and falsi cation. Elster explained to me that there are situations in which historical theory can escape the narrative fallacy and be subjected to empirical rejection—areas in which we are discovering docum