BIOSTATS LEC PRELIMS.docx
Document Details
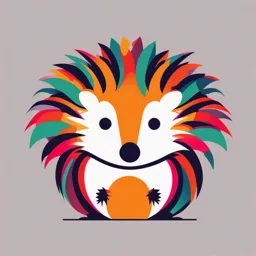
Uploaded by PeerlessBarbizonSchool
Tags
Full Transcript
**[BIOSTATS LEC PRELIMS M1]** **BASIC STATISTICAL AND BIOSTATISTICAL TERMS** **[Importance of Data Collection]** \* Data collection is a crucial part of any scientific investigation \* Goal is to collect valuable evidence that enables analysis and leads to sound and valid answers **[Data Collec...
**[BIOSTATS LEC PRELIMS M1]** **BASIC STATISTICAL AND BIOSTATISTICAL TERMS** **[Importance of Data Collection]** \* Data collection is a crucial part of any scientific investigation \* Goal is to collect valuable evidence that enables analysis and leads to sound and valid answers **[Data Collection Process]** \* Involves preparation, analysis, and conclusion \* Preparation involves considering context, data source, and sampling method **[Statistical Thinking]** \* Requires critical thinking and ability to make sense of results \* Demands more than just executing complicated calculations **[Text Overview]** \* Will help develop statistical thinking skills through examples, exercises, and discussions \* Begins with basic definitions, introduces epidemiology, and discusses data collection and presentation methods commonly used in social, behavioral, and scientific research **[Data]** \* A collection of observations, such as measurements or survey responses \* A single data value is called a \"datum\" (rarely used) \* The term \"data\" is plural, so it\'s correct to say \"data are\...\" not \"data is\...\" **[Statistics]** \* The science of: \+ Planning studies and experiments \+ Obtaining data \+ Organizing, summarizing, presenting, analyzing, and interpreting data \+ Drawing conclusions based on data **[Population]** \* The complete collection of all measurements or data being considered \* Typically, the population is the complete collection of data we want to make inferences about **[Census]** \* The collection of data from every member of the population **[Sample]** \* A subcollection of members selected from a population **[Process Involved in a Statistical Study]** 1\. \*\*Prepare\*\* \* Define the context and purpose of the study \* Identify the source of the data and potential biases \* Determine the sampling method (biased or unbiased) 2\. \*\*Analyze\*\* \* Graph the data to visualize patterns and trends \* Explore the data to identify outliers, missing values, and distributions \* Apply statistical methods to obtain results using technology 3\. \*\*Conclude\*\* \* Determine whether the results have statistical significance (unlikely to occur by chance) \* Determine whether the results have practical significance (make a meaningful difference) **[Definitions]** 1\. \*\*Voluntary Response Sample\*\*: A sample where respondents decide whether to participate 2\. \*\*Statistical Significance\*\*: A result that is unlikely to occur by chance (5% or less) 3\. \*\*Practical Significance\*\*: A result that makes a meaningful difference, but may not be statistically significant **[Analyzing Data: Potential Pitfalls]** 1\. \*\*Misleading Conclusions\*\*: Avoid making statements that are not justified by the statistical analysis 2\. \*\*Sample Data Reported Instead of Measured\*\*: Take measurements yourself instead of asking subjects to report results 3\. \*\*Loaded Questions\*\*: Avoid survey questions that are not worded carefully 4\. \*\*Order of Questions\*\*: Avoid unintentionally loading survey questions with the order of items being considered 5\. \*\*Nonresponse\*\*: Avoid nonresponse rates by ensuring subjects are available and willing to respond 6\. \*\*Percentages\*\*: Avoid citing misleading or unclear percentages **[Basic Types of Data]** 1\. \*\*Parameter\*\*: A numerical measurement describing a characteristic of a population 2\. \*\*Statistic\*\*: A numerical measurement describing a characteristic of a sample **[Quantitative (Numerical) Data]** 1\. Represent counts or measurements 2\. Consist of numbers 3\. Examples: \* Scores on a test \* Heights and weights \* Amount of money spent **[Categorical (Qualitative or Attribute) Data]** 1\. Consist of names or labels, not numbers 2\. Do not represent counts or measurements 3\. Examples: \* Gender (male/female) \* Color (red, blue, green) \* Marital status (married, single, divorced) **MORE EXAMPLES:** 1. 2. 3. **[Discrete Data]** 1\. Quantitative data with a finite or countable number of values 2\. Examples: \* Counting process (e.g. number of physical examinations given) \* Countable infinite values (e.g. number of tosses of a coin before getting tails) 3\. Characteristics: \* Finite or countable number of values \* Can be counted individually **[Continuous Data]** 1\. Quantitative data with infinitely many possible values 2\. Examples: \* Continuous scale (e.g. lengths of distances from 0 cm to 12 cm) \* Volume of blood drawn (between 0 mL and 50 mL) 3\. Characteristics: \* Infinitely many possible values \* Impossible to count individual values **[Levels of Measurement]** 1\. \*\*Nominal Level\*\*: Data consists of names, labels, or categories only. Cannot be arranged in order. 2\. \*\*Ordinal Level\*\*: Data can be arranged in order, but differences between data values are not meaningful. 3\. \*\*Interval Level\*\*: Data can be arranged in order, and differences between data values are meaningful. No natural zero starting point. 4\. \*\*Ratio Level\*\*: Data can be arranged in order, differences between data values are meaningful, and there is a natural zero starting point. **[Big Data]** \* Big data refers to large and complex data sets that require special analysis tools. \* Data science involves applying statistics, computer science, and software engineering to analyze big data. **[Missing Data]** \* Missing data can be either \*\*Missing Completely at Random (MCAR)\*\* or \*\*Missing Not at Random (MNAR)\*\*. \* Methods for correcting missing data include deleting cases, imputing missing values, and using regression analysis. **[Design of Experiments]** \* \*\*Randomization\*\*: Assigning subjects to treatment groups through a random process. \* \*\*Blinding\*\*: Keeping subjects unaware of the treatment they are receiving. \* \*\*Replication\*\*: Repeating an experiment multiple times to increase accuracy. \* \*\*Gold Standard\*\*: Randomization with placebo/treatment groups is considered the most effective experimental design. **[Collecting Sample Data]** \* \*\*Simple Random Sampling\*\*: Selecting a sample where every possible sample has the same chance of being chosen. \* \*\*Systematic Sampling\*\*: Selecting every kth element in a population. \* \*\*Convenience Sampling\*\*: Selecting a sample based on ease of access. \* \*\*Stratified Sampling\*\*: Dividing a population into subgroups and selecting a sample from each subgroup. \* \*\*Cluster Sampling\*\*: Selecting clusters from a population and selecting all members from those clusters. \* \*\*Multistage Sampling\*\*: Selecting samples in multiple stages using different methods. **[Observational Studies]** \* \*\*Cross-Sectional Study\*\*: Observing data at one point in time. \* \*\*Retrospective Study\*\*: Collecting data from a past time period by going back in time. \* \*\*Prospective Study\*\*: Collecting data in the future from groups that share common factors. **[Experiments]** \* \*\*Confounding\*\*: When an effect is seen, but it\'s unclear what caused it. \* \*\*Randomized Block Design\*\*: Assigning treatments to subjects within blocks of similar characteristics. \* \*\*Matched Pairs Design\*\*: Comparing two treatment groups by using subjects matched in pairs. \* \*\*Rigorously Controlled Design\*\*: Carefully assigning subjects to treatment groups to minimize confounding factors. **[Sampling Errors]** \* \*\*Sampling Error\*\*: Discrepancy between a sample result and the true population result due to chance fluctuations. \* \*\*Nonsampling Error\*\*: Error resulting from human error or biased methods. \* \*\*Nonrandom Sampling Error\*\*: Error resulting from using a non-random sampling method. **IMPORTANCE OF BIOSTATISTICS AND HEALTH STATISTICS** **[What is Biostatistics?]** 1\. Biostatistics is the branch of statistics that interprets scientific data generated in health sciences, including public health. 2\. Its goal is to make valid inferences from data to solve problems in public health. 3\. Biostatistics uses statistical methods to conduct research in biology, public health, and medicine. 4\. Biostatisticians collaborate with other scientists and researchers to make sense of complex data. **[Role of Biostatisticians]** 1\. Biostatisticians translate complex data into valuable information for public health decisions. 2\. They develop statistical methods for clinical trials, observational studies, longitudinal studies, and genomics. 3\. Responsibilities include designing experiments, collecting and analyzing data, interpreting results, and making meaningful generalizations. **[What is Informatics?]** 1\. Informatics is an emerging field that combines science, mathematics, probability, and statistics with computer science. 2\. It is used to make advances in public health and medicine. 3\. Health informatics deals with resources, devices, and methods for storing, using, and retrieving information. 4\. Public health informatics applies informatics in surveillance, prevention, preparedness, and health promotion. **[Role of Systems Analysts in Informatics]** 1\. Systems analysts write and troubleshoot software used by biostatisticians and researchers. 2\. They may conduct research, design databases, and develop algorithms for processing and analyzing information. 3\. Responsibilities include incorporating bioinformatics/biostatistics into data analysis tools, developing quality workflow metrics, and working with scientists to develop project plans. **[Importance of Statistical Competencies for Medical Research Learners]** 1\. Medical researchers and health scientists need to be literate in biostatistics to design research studies, analyze data, and report results. 2\. Biostatistics is increasingly used in medical research, and a lack of understanding of statistical methods can lead to mistakes in research or practice. 3\. Statistical competencies are essential for medical research learners to define statistical education and curricula. **[Role of Biostatistics in Medical Research]** 1\. Biostatistics is the study of human health and disease, with applications in biomedical laboratory research, clinical medicine, and health promotion. 2\. Biostatisticians help researchers design studies, analyze data, and interpret results. 3\. Biostatistics is used to make sense of data collected to decide whether a treatment is working or to find factors that contribute to diseases. **[Why Are Statistics Important in Healthcare?]** 1\. Statistics are used in healthcare organizations to measure performance outcomes and implement continuous quality improvement programs. 2\. Government health and human service agencies use statistical information to measure the overall health and well-being of populations. 3\. Statistics are necessary for determining resource allocation, needs assessment, quality improvement, and product development in healthcare. **[Benefits of Statistical Competencies]** 1\. Valid statistical information minimizes the risks of healthcare trade-offs. 2\. Statistical analysis is critical to production efficiency and allocation. 3\. Statistics are important for pharmaceutical and technology companies to develop product lines that meet the needs of the populations they serve. **THE SAMPLE SIZE AND SAMPLING TECHNIQUE** **[Sample Size]** 1\. The sample size is the proportion of the general population that is participating in the study. 2\. A lower sample size means a higher margin of error and lower confidence level, making data less reliable. 3\. A larger sample size means a more \"statistically significant\" result, but may not necessarily be practically important. **[Calculating Sample Size]** 1\. There are various methods for calculating sample size, including Cochran\'s formula and Excel. 2\. Online calculators can also be useful for determining sample size. **[Sampling Techniques]** 1\. Sampling techniques can be divided into two groups: probability sampling and non-probability sampling. 2\. Probability sampling includes techniques such as simple random sampling, stratified sampling, and cluster sampling. 3\. Non-probability sampling includes techniques such as convenience sampling and snowball sampling. **Key Points to Remember** 1\. The sample size affects the reliability and confidence of the results. 2\. A larger sample size does not always mean more accurate results. 3\. The choice of sampling technique depends on the nature and objectives of the study. **METHODS OF COLLECTING, PRESENTING, ORGANIZING, AND SUMMARIZING DATA** **[Data Collection]** 1\. Data collection is the systematic process of gathering and measuring information from various sources. 2\. Principal tools for collecting information include surveys, interviews, focus groups, and web analytics. 3\. Data collection methods can be influenced by the project and needs. Presentation of Data 1\. The presentation of data is important for effective communication and interpretation. 2\. There are three main forms of data presentation: \* Textual presentation: presenting data in text format \* Data tables: presenting data in rows and columns \* Diagrammatic presentation: presenting data using diagrams, illustrations, images, or graphs **[Organizing and Summarizing Data]** 1\. Organizing and summarizing data is important for effective interpretation. 2\. Data can be organized and summarized using various methods, including: \* Arranging values on the basis of one variable \* Dividing data into groups by the values of one variable \* Using tables, graphical, and numerical methods \* Frequency distribution tables **Key Points to Remember** 1\. Accurate data collection is important for reliable results. 2\. The presentation of data should be clear and easy to interpret. 3\. Organizing and summarizing data is important for effective interpretation. 4\. The choice of representation for a collection of data depends on the nature of the data (numerical or non-numerical).