Biometric Lecture Notes PDF
Document Details
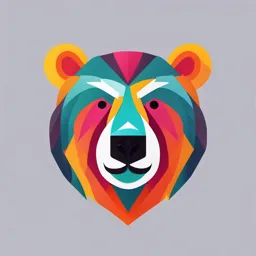
Uploaded by UnbeatableVuvuzela393
Universiti Malaysia Sabah
Tags
Summary
These lecture notes cover biometric topics including ANOVA, correlation, and regression. They provide an introduction to hypothesis testing, p-values, types of errors, standard error, and analyses of variance.
Full Transcript
FC20103 Biometric __________________ Lecture Notes ANOVA, Correlation, Regression __________________ TABLE OF CONTENT 7 HYPOTHESIS AND ANALYSIS 7.1 Hypothesis testing 7.2 p value 7.3 Type of errors 7.4 Standard error 7.5 One-way Analysis of Variance (One-Way A...
FC20103 Biometric __________________ Lecture Notes ANOVA, Correlation, Regression __________________ TABLE OF CONTENT 7 HYPOTHESIS AND ANALYSIS 7.1 Hypothesis testing 7.2 p value 7.3 Type of errors 7.4 Standard error 7.5 One-way Analysis of Variance (One-Way ANOVA) 7.6 Two-way Analysis of Variance (Two-Way ANOVA) 8 CORRELATION AND REGRESSION 8.1 Introduction 8.2 Scatter diagram 8.3 Sample correlation coefficient r 8.4 Linear correlation coefficient 8.5 Regression 8.6 Coefficient of determination 8.7 Linear regression 8.8 Curvilinear regression 7.1 Hypothesis testing Method used for testing a claim about a parameter in a population using data measured in a sample. Figure 7.1 shows four steps to hypothesis testing: Figure 7.1 Four fundamental steps in hypothesis testing. Null hypothesis (H0) - a statement about a population parameter such as the population mean that is assumed to be true. Alternative hypothesis (H1) - a statement that directly contradicts a null hypothesis by stating that the actual value of a population parameter is less than, greater than or not equal to the value stated in null hypothesis. Significance level - a criterion of judgment upon which a decision is made regarding the value stated in a null hypothesis. Test statistic - mathematical formula that allows researchers to determine the likelihood of obtaining sample outcomes if the null hypothesis was true. 7.2 p value Probability of obtaining a sample outcome given that the value stated in the null hypothesis is true. The p-value for obtaining a sample outcome is compared to the level of significance as in Figure 7.2. When the p-value is less than 5% (p <.05), we reject the null hypothesis. When the p-value is greater than 5% (p >.05), we retain the null hypothesis. Significance - the decision to reject or retain the null hypothesis. o When p value >.10, the observed difference is not significant o When p o When p o When p The values in this column represent the significance level (p-value), which indicates the probability that the observed difference in means occurred by chance. Asterisk is placed next to the individual p-values that are less than 0.05 to denote which comparisons are statistically significant. Figure 7.2 Multiple comparison for different means determined using p values from statistical software. 7.3 Type of errors i. o The probability of retaining a null hypothesis that is false. ii. Type I error o The probability of rejecting a null hypothesis that is true. 7.4 Standard error Standard error measures how much sample means vary from the population mean, where it shows the accuracy of estimates when using sample data. When taking multiple samples from a population, sample means will not be exactly the same due to probability. Standard error tells us how much the sample means typically differ from the population mean, by considering factors such as sample size and data spread within each sample. Understanding standard error helps to assess the reliability of the sample mean as an estimate of the population mean. Where: = Standard deviation = Number of samples Example 1: Calculate the standard error for the deviation of 10. Solution: Given: = 10 n = 50 Using the formula: 7.5 One-way Analysis of Variance (One-way ANOVA) A statistical method to test the equality of three or more means simultaneously by examining variances. Assumptions: i. The populations from which the samples were obtained must be normally or approximately normally distributed. ii. The samples must be independent. iii. The variances of the populations must be equal. Hypotheses: o Null hypothesis: All population means are equal. o Alternative hypothesis: At least one mean differs from the others. One way analysis can be calculated in three steps: i. The sum of squares for all samples. ii. The within class and between class cases. iii. Determine degrees of freedom, df [ number of independent that go into the estimate of a parameter]. Fisher Statistic is used to analyze the null hypothesis. Example 2: An educator wants to evaluate the effectiveness of three different teaching methods on student performance in a mathematics course. They randomly assign 15 students into three groups, each group receiving one of the teaching methods. After a semester, the final exam scores (out of 100 points) for each student are as follows: Method A 78 82 77 85 80 Method B 90 85 88 92 91 Method C 70 75 72 68 74 Perform a manual calculation of One-way ANOVA to determine if there are statistically significant differences in the average scores achieved by the different teaching methods. Step 1: Calculate the group means and overall mean. Mean of Method A = = 80.4 Mean of Method B = = 89.2 Mean of Method C = = 71.8 Grand mean = = 80.4667 Step 2: Calculate the sum of squares between groups. Where: = Number of observations = Mean of group i = Grand mean Step 3: Calculate the group means and overall mean. Method A: Method B: Method C: Step 4: Calculate the sum of squares within groups. Where: = Individual observation = Mean of group i Step 5: Calculate the total sum of squares. Step 6: Calculate the degrees of freedom. Degrees of freedom (between groups): Where: = Number of groups Degrees of freedom (within groups): Where: = Total number of observations = Number of groups Degrees of freedom (total): Where: = Total number of observations Step 7: Calculate the mean square between groups. Where: = Sum of squares (between groups) = Degrees of freedom (between groups) Step 8: Calculate the mean square within groups. Where: = Sum of squares (within groups) = Degrees of freedom (within groups) Step 8: Calculate the F value. Where: = Mean square (between groups) = Mean square (within groups) Conclusion: o F-value = 22.1935 o Numerator degrees of freedom (df1) = 2 o Denominator degrees of freedom (df2) = 12 df1 df2 o The F-critical value from the F-table is 3.89. o Since 22.1935 > 3.89, there is no statistically significance , thus accept the null hypothesis. o The results indicate that there are no statistically significant differences among the means of the groups tested. o Tabulated One-way ANOVA table is as follows: Source df Sum of squares (SS) Mean squares (MS) F Between 2 376.93 188.645 22.1935 Within 12 102 8.5 Total 14 478.93 7.6 Two-way Analysis of Variance (Two-way ANOVA) Two-way ANOVA is an appropriate analysis method for a study with a quantitative outcome and two or more categorical explanatory variables. The usual assumptions of Normality, equal variance and independent error apply. Inference for the Two-way ANOVA table involves first checking the interaction p-value to see that we can reject the null hypothesis. If the p-value is smaller than then you can conclude that both factors affect the outcome and that the effect of changes in one factor depends on the level of another factor. The assumptions for the Two-way ANOVA F test for interaction are exactly the same as those of the One-way ANOVA F test with one additional requirement: the number of observations should be the same for all groups. For the hypothesis, the null hypothesis is that there is no interaction, while the alternative hypothesis is that there is interaction. Example 3: A botanist wants to investigate if plant growth is influenced by sunlight exposure and watering frequency. He planted 40 seeds and lets them grow for one month under different sunlight exposure and watering frequency conditions. After one month, he recorded the height of each plant. The results are shown in the following table: Watering Sunlight exposure Frequency None Low Medium High Daily 4.8 5 6.4 6.3 4.4 5.2 6.2 6.4 3.2 5.6 4.7 5.6 3.9 4.3 5.5 4.8 4.4 4.8 5.8 5.8 Weekly 4.4 4.9 5.8 6 4.2 5.3 6.2 4.9 3.8 5.7 6.3 4.6 3.7 5.4 6.5 5.6 3.9 4.8 5.5 5.5 There were five plants grown under each combination of conditions, which are highlighted in the table using a red box. Two-way ANOVA can be calculated by following these steps: Step 1: Calculate the sum of squares for the first factor (watering frequency). First, calculate the grand mean height of all 40 plants: Where: = Grand mean = i: watering frequency, j: individual plant = Total number of observations Now, calculate the mean height for daily watering: Where: = Mean height for daily watering = Sum of heights for daily-watered plants = Number of daily-watered plants Next, calculate the mean height for weekly watering: Where: = Mean height for weekly watering = Sum of heights for weekly-watered plants = Number of weekly -watered plants Next, calculate the sum of squares for the factor A (water frequency) using the following equation: Where: = Sum of squares for the factor A = Number of observations in level j of factor A = Mean of level j of factor A = Grand mean Step 2: Calculate the sum of squares for the factor B (sunlight exposure). First, calculate the mean for no sunlight exposure: Where: = Mean height for no sunlight exposure = Sum of heights for no sunlight exposure = Number of non-sunlight exposed plants Next, calculate the mean for low sunlight exposure: Where: = Mean height for low sunlight exposure = Sum of heights for low sunlight exposure = Number of low sunlight exposed plants Calculate the mean for medium sunlight exposure: Where: = Mean height for medium sunlight exposure = Sum of heights for medium sunlight exposure = Number of medium sunlight exposed plants Calculate the mean for high sunlight exposure: Where: = Mean height for high sunlight exposure = Sum of heights for high sunlight exposure = Number of high sunlight exposed plants Where: = Sum of squares for the factor B = Number of observations in level j of factor B = Mean of level j of factor B = Grand mean Step 3: Calculate the sum of squares within (error). First, calculate the mean values for the combination of watering frequency and sunlight exposure. For daily watering and no sunlight exposure: Where: = Mean height for the combination of daily watering frequency and no sunlight exposure = Sum of heights for the combination of daily watering frequency and no sunlight exposure = Number of the plants from the combination of daily watering frequency and no sunlight exposure For daily watering and low sunlight exposure: Where: = Mean height for the combination of daily watering frequency and low sunlight exposure = Sum of heights for the combination of daily watering frequency and low sunlight exposure = Number of the plants from the combination of daily watering frequency and low sunlight exposure For daily watering and medium sunlight exposure: Where: = Mean height for the combination of daily watering frequency and medium sunlight exposure = Sum of heights for the combination of daily watering frequency and medium sunlight exposure = Number of the plants from the combination of daily watering frequency and medium sunlight exposure For daily watering and high sunlight exposure: Where: = Mean height for the combination of daily watering frequency and high sunlight exposure = Sum of heights for the combination of daily watering frequency and high sunlight exposure = Number of the plants from the combination of daily watering frequency and high sunlight exposure For weekly watering and no sunlight exposure: Where: = Mean height for the combination of weekly watering frequency and no sunlight exposure = Sum of heights for the combination of weekly watering frequency and no sunlight exposure = Number of the plants from the combination of weekly watering frequency and no sunlight exposure For weekly watering and low sunlight exposure: Where: = Mean height for the combination of weekly watering frequency and low sunlight exposure = Sum of heights for the combination of weekly watering frequency and low sunlight exposure = Number of the plants from the combination of weekly watering frequency and low sunlight exposure For weekly watering and medium sunlight exposure: Where: = Mean height for the combination of weekly watering frequency and medium sunlight exposure = Sum of heights for the combination of weekly watering frequency and medium sunlight exposure = Number of the plants from the combination of weekly watering frequency and medium sunlight exposure For weekly watering and high sunlight exposure: Where: = Mean height for the combination of weekly watering frequency and high sunlight exposure = Sum of heights for the combination of weekly watering frequency and high sunlight exposure = Number of the plants from the combination of weekly watering frequency and high sunlight exposure Now, proceed with the calculation of the sum of squared differences. Where: = Sum of squared differences = Individual data points of the combination of the watering frequency and sunlight exposure = Mean of the combination of the watering frequency and sunlight exposure For daily watering and no sunlight exposure: For daily watering and low sunlight exposure: For daily watering and medium sunlight exposure: 1.788 For daily watering and high sunlight exposure: 1.648 For weekly watering and no sunlight exposure: 0.34 For weekly watering and low sunlight exposure: 0.548 For weekly watering and medium sunlight exposure: 0.652 For weekly watering and high sunlight exposure: 1.268 Finally, calculate the sum of squares within (error) by adding up all the individual sum of squared differences for each combination of factors. 7.6944 Step 4: Calculate the total sum of squares. First, calculate the sum of squared differences of the combination factors using the individual plant height and the grand mean. Sum of squared differences for daily watering and no sunlight exposure: Sum of squared differences for daily watering and low sunlight exposure: Sum of squared differences for daily watering and medium sunlight exposure: Sum of squared differences for daily watering and high sunlight exposure: Sum of squared differences for weekly watering and no sunlight exposure: Sum of squared differences for weekly watering and low sunlight exposure: Sum of squared differences for weekly watering and medium sunlight exposure: Sum of squared differences for weekly watering and high sunlight exposure: To find the total sum of squares (SST), sum up all the squared differences calculated for each combination of watering frequency and sunlight exposure. Step 5: Calculate the total sum of squares interaction. Step 6: Calculate the degrees of freedom (df). df for watering frequency: Where: j = The number of levels for watering frequency With 2 levels of watering frequency (daily and weekly), df for sunlight exposure: Where: k = The number of levels for sunlight exposure With 4 levels of sunlight exposure (none, low, medium, and high), df for interaction: Where: j = The number of levels for watering frequency k = The number of levels for sunlight exposure df within (denominator of df): Where: n = The total number of observations j = The number of levels for watering frequency k = The number of levels for sunlight exposure df total: Where: n = The total number of observations Step 7: Calculate the mean square (MS). Mean square for watering frequency: Mean square for sunlight exposure: Mean square interaction: Mean square within: Step 8: Calculate the F-values. F-value for watering frequency: F-value for sunlight exposure: F-value interaction: Step 9: Determine if there is significant difference using the F table. For watering frequency: o F-value = 0.00104 o Numerator degrees of freedom (df) = 1 o Denominator degrees of freedom (df) = 32 df1 df2 (nearest df value to 32) o The F-critical value from the F-table is 4.17. o Since 0.00104 < 4.17, there is statistical significance For sunlight exposure: o F-value = 26.0231 o Numerator degrees of freedom (df) = 3 o Denominator degrees of freedom (df) = 32 df1 df2 (nearest df value to 32) o The F-critical value from the F-table is 2.92. o Since 26.0231 > 2.92, there is no statistical significance For interaction: o F-value = 4.15554 o Numerator degrees of freedom (df) = 3 o Denominator degrees of freedom (df) = 32 df1 df2 (nearest df value to 32) o The F-critical value from the F-table is 2.92. o Since 4.15554 > 2.92, there is no statistical significance Step 10: Tabulated Two-way ANOVA table is as follows: Source df Sum of squares (SS) Mean squares (MS) F Factor A: 1 0.00025 0.00025 0.00104 Watering frequency Factor B: 3 18.77175 6.25725 26.0231 Sunlight exposure Interaction 3 2.99760 0.9992 4.15554 effect Within group 32 7.6944 0.24045 Total 39 29.46400 Summary: These results indicate that watering frequency is the only factor that has a statistically significant effect on plant height. Additionally, the absence of a significant interaction effect suggests that the influence of watering frequency on plant height remains consistent regardless of the sunlight exposure. Example 4: An experiment was run in which 5 replications of 50 blocks of Acacia mangium were planted in each of four (4) soil types in Malaysia. At the end of the first growing season, the heights of the seedlings were measured as an indication of the effect of the soil types to promote height growth. As far as possible, all other growing conditions were kept uniform for each treatment (soil type). Based on the formula given, calculate the total sum of squares. Soil type Replicate Bungor series Malaca series Selangor series Sabah series (cm) (cm) (cm) (cm) 1 1.8 2.6 2.4 2.4 2 1.6 3.2 2.2 2.6 3 2.1 3.3 2.3 2.1 4 1.5 3.6 2.1 2.3 5 2.0 2.8 2.0 3.1 Description Bungor Malaca Selangor Sabah Total Sum 9.0 15.5 11.0 12.5 48.0 Mean 1.8 3.1 2.2 2.5 9.6 x2 16.46 48.69 24.30 31.83 121.28 2 Note: : sum of squares of individual data points; N: number of observations. Solution: Step 1: Calculate the total sum of squares (SST). Where: = Square of individual data points = Sum of all observations = Number of observations from all groups Step 2: Calculate the treatment sum of squares (SSTr). Where: = Number of observations = Mean of group i = Grand mean Step 3: Calculate the error sum of squares (SSE). Where: = Total sum of squares = Treatment sum of squares The error sum of squares can also be found by the following calculations: Where: = Sum of square for all observations = Sum of all observations = Number of observations from all groups Treatment 1 (Bungor soil) Treatment 2 (Malaca soil) Treatment 3 (Selangor soil) Treatment 4 (Sabah soil) Step 4: Calculate the degrees of freedom. Degrees of freedom (between groups): Where: = Number of groups Degrees of freedom (within groups): Where: = Total number of observations = Number of groups Degrees of freedom (total): Where: = Total number of observations Step 5: Calculate the mean square between groups. Where: = Sum of squares (treatment) = Degrees of freedom (treatment) Step 6: Calculate the mean square within groups. Where: = Sum of squares (within groups) = Degrees of freedom (within groups) Step 8: Calculate the F value. Where: = Mean square (treatment) = Mean square (within groups) Conclusion: o F-value = 15.18987 o Numerator degrees of freedom (df1) = 3 o Denominator degrees of freedom (df2) = 16 df1 df2 o The F-critical value from the F-table is 3.24. o Since 15.18987> 3.24, there is no statistically significance , thus accept the null hypothesis. o The results indicate that there are no statistically significant differences among the means of the groups tested. o Tabulated One-way ANOVA table is as follows: Source df Sum of squares (SS) Mean squares (MS) F Between 3 4.50 1.5 15.18987 (Treatment) Within (Error) 16 1.58 0.09875 Total 19 6.08 8.1 Introduction What is correlation? A single number that describes the degree of relationship between two variables. 8.2 Scatter diagram If the straight line is positive in gradient, it is a positive linear correlation. If the gradient is negative, it is a negative correlation. Figure 8.1: Scatter diagrams illustrating positive and negative correlation graphs. 8.3 Sample correlation coefficient r r is a unitless measurement between -1 and 1. If r =1, there is a perfect positive linear correlation. If r = -1, there is a perfect negative linear correlation. If r = 0, there is no linear correlation. 8.4 Linear correlation coefficient r= Where: r = Correlation coefficient n = Number of observations = Sum of the product of x and y = Sum of all squares of values of variable ( )2 = Square of the sum of all the values of variable = Sum of all squares of values of variable ( )2 = Square of the sum of all the values of variable y Where: p = Correlation coefficient d = Difference in ranks between paired observations n = Number of observations or subjects Example 1: Compute the linear coefficient of correlation from the data given in Table 8.1: Table 8.1: Data to compute the linear coefficient of correlation. X Y x x2 y y2 xy 2 6 -14.3 205.4 -8.3 68.7 118.8 3 1 -13.3 177.8 -13.3 176.5 177.1 4 10 -12.3 152.1 -4.3 18.4 52.9 7 4 -9.3 87.1 -10.3 105.8 96.0 9 7 -7.3 53.8 -7.3 53.1 53.4 10 9 -6.3 40.1 -5.3 27.9 33.5 11 14 -5.3 28.4 -0.3 0.1 1.5 12 17 -4.3 18.8 2.7 7.4 -11.8 14 15 -2.3 5.4 0.7 0.5 -1.7 15 10 -1.3 1.8 -4.3 18.4 5.7 16 5 -0.3 0.1 -9.3 86.2 3.1 17 12 0.7 0.4 -2.3 5.2 -1.5 18 18 1.7 2.8 3.7 13.8 6.2 19 13 2.7 7.1 -1.3 1.7 -3.4 23 25 6.7 44.4 10.7 114.8 71.4 24 24 7.7 58.8 9.7 94.4 74.5 25 20 8.7 75.1 5.7 32.7 49.5 27 23 10.7 113.8 8.7 75.9 93.0 28 22 11.7 136.1 7.7 59.5 90.0 29 18 12.7 160.4 3.7 13.8 47.0 30 27 13.7 186.8 12.7 161.7 173.8 Sum=343 Sum=300 Sum=0 Sum=1556.7 Sum=0 Sum=1136.5 Sum=1129 Xmean=16.3 Ymean=14.3 Note: X and Y: variables or data points; x and y: deviation from the mean of X and Y respectively X-Xmean and Y-Ymean; x2 and y2: squared deviations deviation from the mean of X and Y respectively (X-Xmean)2 and (Y-Ymean)2; xy: product of deviations of X and Y (X-Xmean) x (Y-Ymean). Solution: Where: b = Slope of the regression line Where: b = Slope of the regression line = Mean of Y = Estimated value of Y = Mean of X = Estimated value of X Where: r = linear coefficient of correlation x2 = squared deviations deviation from the mean of X (X-Xmean)2 y2 = squared deviations deviation from the mean of Y (Y-Ymean)2 xy = product of deviations of X-Xmean x Y-Ymean 8.5 Coefficient of determination What is the coefficient of determination? Coefficient of determination (R2) is the square of Coefficient of correlation (r2) which measures the direction and strength of a linear relationship between 2 variables, ranging from -1 to 1. Coefficient of determination, R2 = Coefficient of correlation, r2 R2 measures the variance proportion in a dependent variable which is explained by an independent variable, ranging from 0 to 1. The higher the R2, the more useful the equation model. R2 takes on values between 0 and 1. 8.6 Regression What is regression? A statistical analysis assessing the association between two variables. It is used to find the relationship between two variables. 8.7 Linear regression In linear regression, we aim to fit a straight line to a set of data points to model the relationship between a dependent variable (Y) and one or more independent variables (X) as in Figure 8.2. 10 9 8 7 6 5 4 3 2 1 0 0 2 4 6 8 10 Figure 8.2: Linear regression graph. The equation for a simple linear regression with one independent variable is given by: Where: = Sum of the dependent variable = y-intercept of the line (the value of Y when X is 0) = Slope of the line (the change in Y for a unit change in X) = Independent variable The coefficients a and b are determined using two normal equations: o The first equation is for the predicted values of Y ( ): Where: = Sum of the dependent variable = Number of observations = Sum of the independent variable o The second equation involves the product of X and Y: Where: = Sum of the product of X and Y = y-intercept of the line (the value of Y when X is 0) = Slope of the line (the change in Y for a unit change in X) = Sum of the independent variable = Sum of the squares of the independent variable By solving these two equations simultaneously, we can determine the coefficients and in the linear regression model, allowing us to fit a line to the data and make predictions about the dependent variable based on the independent variable. 8.8 Curvilinear regression In curvilinear regression (or nonlinear regression, or polynomial regression), we extend the traditional linear regression model to accommodate curves or nonlinear relationships between variables. While linear regression involves fitting a line to the data, curvilinear regression fits a curve. Polynomial regression is an example of curvilinear regression. A polynomial equation is 2 3 any equation that has raised to integer powers such as and. Polynomial equation has 3 types of equations, quadratic equation, cubic equation and quartic equation. Equations are as follows: =a+b1X+b2X2, quadratic regression equation where is the intercept and 1 and 2 are constants. It produces a parabola as in Figure 8.3. 350 300 250 200 150 100 50 0 0 2 4 6 8 10 12 14 Figure 8.3: Quadratic regression graph. =a + b1X + b2X2 + b3X3, cubic regression equation where is the intercept and 1, 2 and 3 are constants. It produces a S-shaped curve as in Figure 8.4. 7 6 5 4 3 2 1 0 0 1 2 3 4 5 6 7 Figure 8.4: Cubic regression graph. =a + b1X + b2X2 + b3X3 + b4X4, quartic regression equation where is the intercept and 1, 2, 3 and 4 are constants. It produces M or W shaped curve like in Figure 8.5. 16 14 12 10 8 6 4 2 0 0 2 4 6 8 10 12 Figure 8.5: Quartic regression graph.