bioinformatics quiz 2ACpt2.odt
Document Details
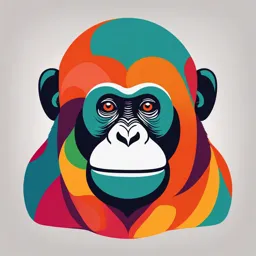
Uploaded by ReliableMookaite1890
Full Transcript
These cause change in allele frequencies leading to evolution of populations: Genetic drift, stronger in small population size Mutation Migration Non-Random Mating ex) inbreeding Selection How do the 5 forces change allele frequencies? Genetic drift reduces diversity. This leads to ran...
These cause change in allele frequencies leading to evolution of populations: Genetic drift, stronger in small population size Mutation Migration Non-Random Mating ex) inbreeding Selection How do the 5 forces change allele frequencies? Genetic drift reduces diversity. This leads to random fixation of one allele. The probability of which allele is fixed depends on its initial frequency. Probability of fixation of allele A=f(A) Genetic drift stronger in smaller populations Random process and outcomes will differ. Mutation New mutations introduce variation into a population. Migration Is dispersal followed by breeding with offspring. Homogenizes allele frequencies in populations. if migration is between previously isolated populations, it will lead to admixture. Makes allele frequencies more similar. Less migration=more divergence Non-random mating A mating system in which at least some individuals are more or less likely to mate with individuals of a particular genotype than with individuals of other genotypes. Assortative mating is when pair bonding is based on an observer able phenotype. Alleles associated with the phenotype will increase in frequency. Inbreeding Mating between relatives Results in excessive homozygotes Does NOT change allele frequencies, only reduces the number of heterozygotes. Harmful due to rare recessive detrimental alleles that become homozygous. Increased change of rare genetic disorders Inbreeding depression Caused by an excess homozygous genotype with recessive detrimental alleles in individuals. Florida Panthers: Inbreeding depression linked to the following: Decreased survival Increased mortality of cubs Reduced number of offspring by females Increased sperm abnormalities Selection Natural selection Hereditary differences among organisms; different ability to survive and reproduce Based on fitness, relative ability of genotypes to survive and reproduce Consequence of: Hereditary differences among organisms Different abilities to survive and reproduce. There can be different kinds of selection. Directional selection: Directional selection occurs when individuals homozygous for one allele have a fitness greater than that of individuals with other genotypes and individuals homozygous for the other allele have a fitness less than that of individuals with other genotypes. In another population, it may be more advantageous to have more eggs, so this would shift the curve to the right towards “many”. Fitness Relative ability of genotypes to survive and reproduce. Relative fitness Measures the comparative contribution of each parental genotype to the pool of offspring genotypes, in each generation. Selective coefficient (S) Refers to selective disadvantage of a disfavored genotype. S=1-W, W=relative fitness Under NO natural selection, allele frequencies stay the SAME between generations. Bottleneck It is a special case of genetic drift due to temporary reduction of population size. Occurs when a population is reduced in size, changing allele frequencies in the future generations with a loss of variation. Estimating population structure A common index is the fixation index (Fst) Is an estimate of genetic divergence between populations, determines migration It will compare how alleles are distributed among versus within populations. Fst=AP(WI+AI+AP) AP= estimated variance in allele frequencies among populations AI=estimated variance in allele frequencies among individuals WI= estimated variance in allele frequencies within individuals Fst = 0.0 to 0.05, no to low structure (high migration) Fst = 0.05 to 0.25, moderate structure Fst = 0.25 to 0.50, high structure Fst = 0.50 to 0.75, very high structure Fst = 0.75 to 1.0, virtually no migration, completely different alleles Fst=HIGHER=populations with LESS migration between them Fst=LOWER=populations with MORE migration between them Estimate of connectivity Fst measures the degree of genetic differentiation among subpopulations within a larger population. It quantifies the proportion of the total genetic variation that is attributable to differences between subpopulations, making it a useful tool for assessing population structure. CM Centimorgans Map unit to measure genetic linkagel distance between chromosomes Linkage Disequilibrium (LD) Nonrandom association of alleles at 2 or more loci Where does LD on chromosomes come from? The closer 2 loci (Locus A and Locus B) are on a chromosome: The LESS likely there will be a cross over between them. The MORE likely their alleles will occur together on a chromosome. If 2 loci are very close to each other on a chromosome, then they will nearly always have their alleles inherited together, forming what are called haplotypes. Coefficient of Linkage Disequilibrium, D Difference between the frequency of gametes carrying the pair of alleles A and B at 2 loci (pAB ) and the product of the frequencies of those alleles (pA and pB ) Measures to what extent 2 alleles on DIFFERENT LOCI are associated together, relative to that expected by chance. D=pAB – pApB Linkage Block Areas of chromosomes divided into segments with strong linkage disequilibrium Most chromosomes are divided into segments with strong LD, which we call linkage blocks. Within a linkage block, alleles on all loci are highly correlated. A linkage block with a unique set of alleles always together is referred to as a haplotype. For each linkage block, there are relatively small #s of haplotypes in one population. Alleles on the loci within each linkage block are associated together forming haplotypes. Human chromosomes have linkage blocks from 100s to 100,000s bp long. Haplotypes are identified by genotyping single nucleotide polymorphisms. A few representative TAG SNPs can be genotyped to capture the common haplotypes present in populations. TAG SNP: single nucleotide polymorphism correlated with all variants in that linkage block. Genetic linkage is the associated of alleles form different loci because they are on same linkage block. Haplotype Alleles on different loci inherited together; when two loci close together less liekly for recombination and thus will be inherited together Normally identifeid by genotyping SNP Genotype-Phenotype Association Direction association Indirect association Inheritance Models Penetrance The risk of disease in a given individual (g) The percentage of individuals that actually show the expected phenotype. Expressivity: extent or magnitude of that phenotype expression For GWAS, models are needed to specify the expected relationship between the genotype and phenotype. The following models are assuming genetic penetrance parameter g and biallelic locus with alleles A and a Multiplicative models Disease risk is increased g-fold with each additional A allele. Additive model Risk of disease is increased g-fold for genotype a/A and by 2g-fold for genotype A/A Recessive model Two copies of allele are required for g-fold. Common dominant model Either one or two copies of allele A are required for g-fold increase in disease risk Polygenic model (complex traits) Numerous alleles and genes contribute small amounts to disease risk. Two common approaches to find gene: Linkage Mapping and GWAS Linkage Mapping Genes are mapped by typing markers in families with diseases/trait values within pedigrees. in any family, disease alleles will be within 19-20cM of marker (cM is about the % recombination) markers are spaced every 10cM. GWAS An association study that surveys most of the genome for casual genetic variation Do not need prior information on pathways/genes that affect phenotype Variants are genotyped across genome and compared to phenotype information. Correlations between alleles and diseases are “associations.” A genetic map is used to identify casual genes in LD with significant SNPs in region. GOAL is to map casual mutations to chromosomes. For many complex phenotypes and diseases, any one locus has only a modest contribution Quantitative traits: cumulative action of many genes and the environment There are many challenges: Power Comprehensiveness Interpretation Analysis Advantages: Can be applied to quantitative and complex traits. Very dense SNP assays are now available that cover the entire genome. Relatively inexpensive because you still take advantage of linkage so you do not need to sequence all variants. Do not need prior information on pathways/genes that affect phenotype. Haplotype blocks utilized and not necessary to sample all loci in each haplotype block to find associations Identify SNPs in haplotype block Sample representative SNPs in unaffected groups Sequence genes in that haplotype block to find casual mutation Mendelian Inherited Trait A carrier passes on disease to half of his/her offspring. Most diseases are not inherited in this fashion, they are polygenic and complex. It was too hard to construct pedigrees. Haplotype Blocks Used to find genes affecting phenotypes. It is not necessary to sample all loci in each haplotype block to find significant associations. Enable large-scale GWAS. Identify SNPs in haplotype blocks sample representative SNPs in affected and unaffected groups à sequence genes in that haplotype block to find casual mutation. Study Design SNP assays Currently there are human SNP chips that assay >1 million SNPs. Need to include some low frequency SNPs as these are the ones that likely contribute proportionately more to diseases. Population samples Need to have SNP assay developed for the population you are testing. Important to repeat GWAS with another set of samples. Subset can be retested with a denser SNP assay. Challenges Genes with modest effects require genotyping of thousands of individuals. Must correct for multiple hypothesis testing because each NSP is an independent test. Bonferroni correction N=# of independent tests pcorrected = 1 – (1-puncorrected)n simplifies to: pcorrected = (puncorrected)/n The Bonferroni correction tries to avoid any false-positive errors. It seeks to control the family-wise error rate (FWER), the probability that any of the test results is a false positive. Odds Ratio Measure of effective size, or the strength of association Odds=P/(1-P) where P=probability of event Odds ratio= odds(event:exposure)/odds(event:lack of exposure) Multi-stage approach A multi-stage approach can reduce genotyping costs while maintaining power. Permutation To find more appropriate p-value threshold Avoiding false positives Random effects that result in false low p values can be reduced by: Multi-stage population analysis Permutation testing to find the best p-value threshold. Other sources of error: Systematic bias in study design Population stratification due to admixture Technical artifacts Cases and controls not genotyped together. Missing data if particular genotypes such as heterozygotes are more likely to be scored. Population stratification Different ethnic groups have different disease prevalence and allele frequencies. Grouping ethnic subgroups in one population creates a stratified population via admixture. Combining 2 or more populations with different allele frequencies into one group If one ethnic group has greater incidence of a disease, and stratification is NOT taken into account, all alleles that have higher frequencies in that population will be in association with the disease. It is critical to take population structure into account when doing GWAS. What is in a genome? Around 45% of the human genome is derived from repetitive elements. Repeated elements are short/long patterns of nucleic acids (DNA or RNA) that occur in multiple copies throughout the genome. Tandem repeats: short DNA sequences, further classified into micro and minisatellites Transposable elements: DNA transposons and retrotransposons Only 30% of sequence in a genome from genes Less than 2% are from coding exons. There are also long noncoding RNA, genes produce functional product Around 70% of the genome is “intergenic” (between genes) Simple repeats Transposons Retrotransposons (SINES and LINES) Conserved noncoding regions. >200bp conserved 99% or greater similarity in all vertebrates. Regulatory DNA Regulatory sequences which transcripts bind to (regulatory elements) not part of gene Structural regions Centromeres and telomeres Variation in Genomes Microsatellites Insertion/deletion numbers of tandem repeats Length difference in repeat units Micro-satellites consist of a short, repetitive motif such as, CACACACA, which is repeated multiple times in tandem. The number of repeats can vary between individuals and populations. Single nucleotide polymorphism Mutation replacing nucleotide. 1-bp substitution in sequence Deletion Loss of a chromosomal segment Deletions do NOT revert because the DNA is gone. Duplication Repetition of a chromosomal segment Inversion A change in the direction of genetic material along a single chromosome Genetic material may remain the same but is rearranged. Translocation A segment of one chromosome becomes attached to a nonhomologous chromosome. The genetic material may remain the same but is rearranged. Deletion, duplication, inversion, and translocation are easy to characterize with NGS. Mobile elements actively move around. 500,000 of L1 transposons in human genome L1 transposons, also known as retrotransposon, are found in genomes of many organisms and humans. L1 transposons use a copy and paste mechanism to move within the genome. This means they are first transcribed into RNA and then reverse transcribed into DNA by an enzyme called reverse transcriptase. The resulting DNA copy is then inserted into a new location of the genome. Since they can insert themselves into different locations in the genome, they have potential to cause genetic variation. Occasionally insert into a genome Hemophilia A caused by L1 insertion in factor VIII gene Altered splicing Altered splicing, also known as alternative splicing, is a molecular process that occurs during gene expression. It involves the selective inclusion or exclusion of different exons from a pre-messenger RNA transcript to generate multiple protein isoforms from a single gene. This process allows a single gene to produce multiple functional protein variants within distinct properties. Altered splicing in MAPT genes that produce Tau proteins cause frontotemporal dementia. Illumina sequencing workflow is composed of 4 basic steps: Sample prep, cluster generation, sequencing, and data analysis Fragment DNA+ add adapters Add DNA to follow cell containing complimentary oligios that bind to adapter Bridge amplification; cluster formation Fluorescently tagged nucleotides added to initiate sequencing; laser added to flow cell Raw images processed into FASTQ files Illumina vocab: Adapter Known primers added to ends of DNA fragments in illumina work flow Barcode Enables simultaneous sequencing of multiple samples in illumina. Short DNA sequences added to individual frags and act as molecular taf for tracking of that respective frafment Contained in adpdaters during amplifciation Flow cell Glass slide with oligos that attach to adapters Cluster Formed after bridge amplification, multiple copies of same DNA strand on flow cell Color determines the base each cycle Each cluster generates one single end read or two paired end reads Cycle In illumina, aids in identifying the base interpreted from the color of a cluster on the flow cell. Base calling from Raw Data The identity of the base is interpreted from the color of a cluster “A spot” on the flow cell during each cycle. The certainty of the base is determined by how distinct and clear the spot is Each cluster generates one single-end read or two paired-end reads. FASTQ Files Raw images are processed into FASTQ files The format is a txt file with four lines per read There can be millions of reads per text file Quality values in ASCII characters for the read in the same order as the bases in line 2 Line 1: starts with @ character and the sequence identifier for the read follows. also, can be referred to as the name. Line 2: The nucleotide sequence for that read Line 3: Starts with the + character and is optionally followed by the reads sequence identifier. This you can often ignore. Line 4: Has the quality values in ASCII characters for the read in same order as the base in line 2 Phred quality score It is a measure of the quality of the identification of the nucleobases generated by automated DNA sequencing. can be used to compare the efficacy of different sequencing methods. Best score to obtain is 30--> 0.10% is used to indicate the measure of base quality in DNA sequencing. High consistency of a sequenced base is indicated by greater values of Phred. The quality score of a base, also known as a Phred or Q score, is an integer value representing the estimated probability of an error, that the base is incorrect. ASCII quality characters in left-to-right increasing order of quality Assessing Read quality Generates quality reports: Distribution of rad length and quality score Trim/remove reads based on chosen criteria: Does downstream software use quality data? How sensitive is your analysis to errors? Shorter sequences Barcodes Adapters Primers Fragments of vectors For shorter sequences: For <15bp, blast can fail to find matches Use blast-short, or simple match script to trim reads Longer sequences Vectors Host bacteria Contamination from pathogens mtDNA chloroplasts For longer reads, search/map reads against database of contaminating sequences and REMOVE them In some cases, you should REMOVE DUPLICATE sequences that arise during the PCRs stage of DNA library preps Applications for Instruments: Sanger- older-style sequencer Sequencing of specific gene segments in 10s-100s of samples Microsatellite genotyping (forensics, population structure) Illumina (NGS)- shorter reads, lots of sequence, higher accuracy De novo genome assembly Genotyping by sequencing (RADseq, target selection, amplicons) RADseq is a method for SNP detection in genomes and it can identify polymorphic variants adjacent to restriction enzyme digestion sites. Can also be used in association mapping, genetic mapping, and estimation of allele frequencies. Copy number variation/structural differences CNV is the term to describe a molecular phenomenon in which sequences of the genome are repeated, and the number of repeats varies between individuals of the same species. Transcriptome and differential expression Refers to the protein-coding part of an organism’s genome. It refers to the set of RNA molecules such as mRNA, tRNA, and rRNA, and other noncoding RNA molecules that are present in cells. Differential expression is different gene expression and is the process where different genes are activated in a cell, giving that cell a specific purpose that defines its function. Metagenomes Is the study of the structure and function of entire nucleotide sequences isolated and analyzed from all the organisms in a bulk sample. barcoding Pac bio (NGS)- longer reads, less sequence, higher errors De novo bacterial/viral genome assembly BAC sequencing Is an engineered DNA molecule used to clone DNA sequences in bacterial cells. Re-sequencing to improve de novo assembly of eukaryotic genomes Approach to De Novo genome assembly De novo sequencing refers to sequencing a novel genome where there is no reference sequence available for alignment. Sequence reads are assembled as contigs, and the coverage quality of de novo sequence data depends on the size and continuity of the contigs (ie, the number of gaps in the data). Cuts chromosome into small 400-500bp pieces. Reconstructing the sequence from short reads Overlap reads to reconstruct the sequence of the chromosomal segment Reads from same part of chromosome align together Read depth or Coverage needs to be built on accurate contigs Calculating coverage ( C): The likelihood that you will not have any reads for a specific site Probability [site not covered]=E-c String graph In de novo assembly, refers to overlapping path to construct full sequence contig Nodes are reads Edges are overlaps Weights are lengths of non-overlapping prefix Find overlapping path to get full sequence contig A string graph is constructed by adding an edge for every pair of overlapping reads. A single node corresponds to each read and reaching that node while traversing the graph is equivalent to reading all the bases up to the end of the read corresponding to the node . Sequence obtained from consensus In de novo assmeblu obtained from contigs. Each consensus based obtained by weighted voting which incorporates quality scores. For example, lets say you have a heterogenous tumor and you want to confirm the short reads are accurate that you have obtained. You can make primers to amplify that section and then use PCR to amplify that region, then sequence it in a different platform and if you see the same sequence again, then you can confirm it. Assembly and coverage: Low coverage High coverage Ways to assess how good your assembly is: % error Compare with subset of loci from known reference Number of Contigs The more contigs the more fragmented Length of contigs and scaffolds N50 and max N50 Contigs: If you line up ALL contigs LONGEST to SHORTEST, add them up until you have 50% of total assembly. You pick the shortest one in that set. Collapsed Contig Means that reads from different parts of chromosome that has the same sequence are mapped/aligned together in one location of contig merging the reads to the potential repeat boundaries resolving collapsed Contigs repeats cause contigs that are occurring in multiple paths. there are 2 possible solutions for resolving collapsed contigs: sequence through repeat if the read length is longer than repeat, the conflicting node is removed from the graph. Sines:100-700bp Lines:7,000bp determine distance between unique contigs. try to see how far apart the two unique sequences are from each other Resolving repeats Mate Pair DNA libraries Fragment DNA contigs 1&2, circularize together, fragment and select pieces that ligated and sequenced. Assembly using short reads combine standard libraries at high coverage and large mate libraries at low coverage to assemble larger contigs Comparisons between assembly programs When comparing assembly programs, you need to consider speed and in addition to error, you need to consider the number of contigs, along with contig length. Velvet is the best assembly program Constructs whole-genome sequences from short DNA sequence reads, such as those generated by NGS platforms. Genome assembly program that is the fastest and one of the most accruat short read assemblers Genome Re-sequencing Goal: To get data on genetic variation by mapping rads to a known reference sequence: SNPs INDELs Structural variants Structural variation: The distance and orientation between paired reads provides information on structure. On Illumina sequencing flow cell, Bridge amplification is used to copy each DNA fragment many times for its abundance to be high enough to be sequenced. Bridge amplification Each cluster is where the sequence is being called and that comes from a single DNA molecule The C refers to the mean of the number of aligned reads that stack, at a particular site in the reference sequence. If you have a genome assembly with contigs of the following length, what's the N50? Contig 1:5mb Contig 2:25 mb Contig 3:40mb Contig 4 :30 mb Count up length of all contigs, 100Mb. Then you start adding up, 40+30=70, which already broken it up in half. The N50 is 30. What are the two best ways you can generate large scaffolds in genomes with many repeats? Sequences mate pair libraries Sequence with a method that generates very long reads Whole Genome vs. Enrichment Whole genome sequencing is straight forward, you are sequencing the entire genome. Enrichment is a subset of genes or regions of the genome are isolated and sequenced. Target enrichment works by capturing genomic regions of interest by hybridization to target-specific biotinylated probes, which are then isolated by magnetic pulldown. For most studies, you need to obtain allele frequency information. Sequencing of entire genomes are often not necessary, which leads to our goal. The goal is to sequence the SAME regions with INFORMATIVE VARIANTS in many individuals. The cost of the whole genome sequence for populations of many organisms is getting cheaper. Primary cost saving approaches for genome variation at population level: Low coverage whole genome sequencing of individuals 0.5x-5x genome coverage of 10s of individuals Can be done by pooling them into 1 library Reduced-representation sequencing: used in smaller proprtion of the population Targeted enrichment 30x-300x coverage of <1% of genome in 10-50 individuals Restriction enzyme digestion and sequencing >100x coverage of 0.1% of the genome in 100s of individuals Things to consider when it comes to sequencing: Capture amount How much of the genome do you target? Specificity How many reads will map to the targets? Variability How will coverage vary across targets? Reproducibility How will data from different library preps and sequencing runs compare? Cost and ease How many samples can you sequence with your resources? Input DNA How much DNA do you need vs have? Calculating coverage A key parameter when it comes to successful data generation Step 1: Add all the base pairs of the segments that you want to sequence Step 2: Multiply the coverage you want by the total segment length Step 3: Multiply by the number of samples you want Step 4: Identify platform that gives you roughly that much data Advantages to Low/Ultra low coverage sequencing You have data for the entire genome for multiple individuals You have a greater chance of detecting all the alleles Genome sequencing of Pooled DNA This is very efficient if you can do this by analyzing just simply by comparing populations Combine 10-100 samples and into one library pool Sequence at 0.5X per sample Get population allele frequency information: The number of reads per allele is proportional to its frequency Can detect rare alleles but sequencing error bigger issue Advantages: Save time and money on library preps Can sequence more samples Disadvantage: No individual information Targeted Enrichment PCR: a form of targeted enrichment PCR is used to amplify target regions in all samples 3 general strategies: Uniplex PCR 10-20kb long-range PCR of region Multiplex PCR Separate PCRs for each locus 10-400bp Hybridization: another form of targeted enrichment Solid Platform Well established approach developed for microarrays Hybridization DNA to oligonucleotide probes Specialized equipment and limited to 24 samples roughly Need a lot of DNA Identification of mutation causing Miller syndrome Exome capture via hybridization to a microarray 27.9Mb, spanning 160,000 exons 2 affected siblings, 2 affected unrelated, compared with human HapMap Sort out functional rare variants found in affected individuals with inheritance pattern Identified DHODH as a gene carrying casual mutation Restriction Enzyme Prepared Libraries Also called RadSeq Shear DNA with restriction enzyme which cuts chromosomal segments at specific places Ligate adapters bad barcodes to the restriction sites Sequence 100-150bp adjacent to the restriction sites Results in reads generated for the same sections randomly distributed in genome Then map reads to reference genome, get consensus sequences, and genotype variable SNPs at loci “RadTag” For whole genome coverage goal is to genotype 30-40k SNPs Steps: 1.Cut extracted DNA with restriction enzymes 2.Ligate adapters and barcode 3.Pool samples, size, select, PCR amplify, purify 4.Sequence on Illumina single end or paired end 5.Map reads to reference and genotype. Reads stacking on 1 cut site is one locus How can you identify region with phenotypic effect? Calculate diversity estimates on sliding windows across chromosomal regions Look for low, or high, levels of diversity Heterozygosity Nucleotide diversity Private alleles Regions of linkage disequilibrium (haplotype blocks) Divergence between populations (Fst) Identify structural rearrangements/CNVs Selective sweeps A selective sweep refers to the rapid increase in frequency of a specific genetic variant (Allele) due to positive natural selection. Sbf and ApeKI Both are restriction enzymes that shear DNA at specific sites GPR158 Allele related to meatbolism in bobcats;reduced energy expenditure and affects obsesity; more variable in north LECT2 Allele related to bob cat metabolism; abdominal fat sorage and lipid metabolism; compeltely different in North vs. South bobcats TRPM Allele related to noxious heat detection in bobcats; variable between north. Vs south Schematic difference between low coverage whole genome versus targeted enrichment