Bio 3.2.PDF
Document Details
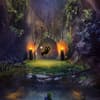
Uploaded by iiScholar
Arizona State University
Full Transcript
Chrapter 3: Designing and Interpreting Fxperiments -: sson 3.2 Statistics :'stistics is the branch of mathematics used to guide experimental design and data collection. Statistics : : s: used to organize, present, analyze, and interpret numericaldata fordescribing group - : ='::teristics,...
Chrapter 3: Designing and Interpreting Fxperiments -: sson 3.2 Statistics :'stistics is the branch of mathematics used to guide experimental design and data collection. Statistics : : s: used to organize, present, analyze, and interpret numericaldata fordescribing group - : ='::teristics, drawing conclusions, and making predictions. l=--:'ctive statistics include measures of a data set's centraltendency (ie, average), such as mean, -e"Cian. and mode. In addition, statistical measures such as range and standard deviation (SD) :-: , :e information regarding the variability (ie, dispersion) of a data set. Figure 3.8 summarizes how -;:- rnedian,mode,andrangearecalculated,andFigure3.gillustratestheconceptofstandard -:' t. r L : Data set; 8, 13, 9,6, 8, 9, 8 Mean (.t ) Median 1. Add numbers to obtain total sum. 1. Order numbers from lowest to highest value. 8+13+9+6+8+9+8=61 6,8,8,8,9,9, 13 2. lf there is an even amount of numbers, add 2. Divide total sum by the number of values the two middle numbers and divide by 2. in the data set. 3. lf there is an odd amount of numbers, the Number of values = 7 middle number is the median. y=61 l7 6,8,8, *,9,9, 13 i=8.7 Vedian = 8 Mode Range 1. Count the frequency of values in the data set. 1. Order numbers from lowest to highest value. >,4 6,8,8,8,9,9, 13 Hs iz Er 2. ldentify minimum and maximum. LLO ,? 8, 8,8,9, 9, , 6 8 I 13 irilinintL.rnr :\ri:,::"rr'i. , Data set values 3. Subtract minimum value from maximum value. 2. Mode is the value that occurs most frequently. Range=13-6 Mode = 8 Range = 7 -- -- 3 8 Calculation of mean, median, mode, and range 79 ChaPier 3: ilesigning and I nierpreting HxP*rinnents or dispersed Standard deviation is a measure of how variable a set of data points are around the mean o E u- LL Length (cm) Length (cm).. Larger range (data Points more Smaller range (data Points moce spread out around the mean) clustered around the mean). Less variation among data Points. More variation among data Points Figure 3.9 Standard deviation' the ways that statistics can be used to reach This lesson examines the components of statistics and pertains to biorogicar research in particurar, statistics are experimentar concrusions. Arthough this resson knowledge of statistics may be tested in used in all areas of scientific reseirch. As such, fundamental multiple sections of the MCAT. 3.2.01 TyPes of HYPotheses designed to test particular hypotheses' Biological research typically involves carrying out experiments research questions that underlie expertments' which, in general terms, are proposed answJrs to the More specifically, a hypothesis is an assumption that is made regarding a relationship between variables of an experiment is to generate data by which being investigated in an experiment. The purpose hypotheses should be rejected or not' A null hypotheses may be tested io determine whether the represent opposite assumptions about the hypothesis (Ho) ano an alternative hypothesis (Ha) relationship being investigated' difference exists between two or more groups with The assumption made in a null hypothesis is that no ln other words, the null hypothesis assumes that respect to the factor being studied in the experiment. the the inde perrdent variable'ln has no effect on the dependent variable' In contrast' "n "*p"riment of the independent variable causes an effect on the alternative hypothesis assumes that manipulation Figure 3'10 summarizet groups in the experiment. dependent variable, resulting in a drfference between the characteristics of null and alternattve hypotheses' 80 Ch*pier 3: llesigning a*d lnterpreting ffixperlrnents Experiment: expose plant seeds to darkness or sunlight and compare plant growth Null hypothesis (Hs) Alternative hypothesis (Ha) Sunlight has no effecl on plant growth Sunf ight promotes plant groMh Seed l{.ii"q l*nd. Typically states the opposite of what States what researchers predict will occur researchers predict will occur Negates Hs Generally presumed to be true prior to Predicts that a statistical difference exists data collection between groups being studied Predicts that no statistical difference exists between groups being studied = ;-re 3.10 Null and alternative hypotheses. l+:.:-se the null hypothesis is presumed to be true before conducting an experiment, researcners -.- = ,7e experimental results to determine whether there is sufficient evidence to reject the null - :::^esis. lf such evidence is lacking, the null hypothesis cannot be rejected (ie, the researchers falfo +:: :te null hypothesis). = : 2.02 Significance 3=tjstical significance pertains to the probability that an experiment's null hypothesis is true given an r::=::able level of uncertainty about the actual situation. Experimental results that are statistically :;-':antareunlikelytobeduetochance(ie,samplingerror). Assuch,theprobabilityofobtaininga :-=::::ally significant result is very low if an experiment's null hypothesis (which asserts that manipulation :-.-: experiment's independent variable has no effect) is indeed true. --: ovalue is widely used to determine the significance of experimental results. A p-value is formally :i'-:: as the probability of observing a given result due to chance alone, assuming that the null - :'::-esis is true. The p-value ranges from 0 to '1 and is generally irrlerpi-eierl as follows, which assumes : :"cnificance level (c) of 0.05: r : > 0,05 signifies a greater than 5% probability that the observed result is due to chance alone (ie, -:: due to an actual association between the variables under study). Values in this range are ;:-erally not considered statistically significant. r : < 3.05 signifies a 5o/o or lower probability that the observed result is due to chance alone. Values - :1is range are typically considered statistically significant. I :::='nining the p-value for an experimental result, researchers can evaluate the exoeriment's null * : ----:sis That is, if the calculated p-value is greaferthan the o chosen before the experiment (eg, : :: :-e null hypothesis cannot be rejected, whereas if the p-value is /ess than or equaltothe :=:+:"nined q, the null hypothesis isrgecfed. Furthermore, rejection of the null hypothesis provides ;: - -: ^g evidence in favor of the experiment's alternative hypothesis. Figure 3.1 1 summarizes the *=-: -::ation of p-values. B1 Chapter 3: Designir"rg and Interpreting Hxperirnentt lf p-value 5 o lf p-value > c Ho is rejected Ho is not rejected Decision about Ho t Convincing evidence I l No convincing evidence I Conclusion about Ho in favor of Ho I i in favor of Ho i."t Themagnitudeofthepva|uere|ativetothesignificanceleve|(q) against a null determines whether there is convincing evidence alternative hypothesis (Ho) hypothesis (Ho) and in favor of an Figure 3.11 Interpretation of p-values' 3.2.03 Errors judgments regarding o""Vr'ir-" data can sometimes lead researchers to make erroneous "-*ur".rrl null hypotheses. That is, researchers sometimes conclude that the data collected in an experiment when it is true (ie, the experimental treatment demonstEate tnat a nuttnypothesis should be rejected Likewise, researchers may fail to reject a null indeed has no effect on the dependent variablej. does produce an effect (ie' the null hypothesit hypothesis when it is true that ihe experimental treatment is false). (ie, reach a false'positive conclusion)' this ty1 when researchers mistakenly reject the null hypothesis researchers mistakenly fail to reject the null hypother of error is called a type I error. conversely, when a type lt error' The probability of (ie, reach a false-negative conclusion), they have committed significance level chosen by the researchers for tht committing a type I error is represented by o ii", tft" a type ll error is represented by 9' hypothesis test), wnereas the'probability of committing concrusion (ie, committing a type I error) b A researcher can reduce the risk of reaching a farse-positiv_e of 0'05)' However' the risk of committing a type I choosing a lower significance level (eg, 0.01 instead ll error. Consequently, use of a lower error is inversely related to the risk of committing a type a farse-negative concrusion (ie, committing a significance rever resurts in an increased risk otieacning of type I and type ll statistical errors' type ll error). Figure 3.12 summarizes the charactertstics 82 Chapter 3: Designlng and Intenpreting Hxperiments Actual status of the null hypothesis (Ho) Hois true Ho is false Rejected a true Ho Rejected a false Ho Reject Ho (reached false-positive (appnc rrii:rilr.: ri i,!-i :;l [.tsion ) I conclusion) 3 o. Type I error.cl ProbabllitV of error = o o c ;- o.9 o oo Fail to ,* Failed to reject a true Ho Failed to reject a false Ho (reached false-negative (x ptr:;r-opriato eon cl us ian ) reject Ho conclusion). Type li error. Probability of error = B : gure 3.12 Type I and type ll errors. !:::istical power refers to the probability that a test for statistical significance will detect an effect resulting ':r manipulation of an independent variable in an experiment, when such an effect exists. In other ',:-3s, power is the probability that the null hypothesis will be rejected in an experiment when a difference :*,., exists between groups (ie, probability that the null hypothesis is correctly rejected). l::ause a type ll error occurs when researchers falto reject the null hypothesis when appropriate (ie, ,-:n the experimentaltreatment does produce an effect), power can also be defined as the probability "-:: type ll error will be avoided. As such, statistical power is mathematically equal to 1 - B (ie, 1 minus :-: crobability of a type ll error occurring), and a hypothesis test with high power (ie, power close to 1) ::::cts false null hypotheses with high sensitivity (Figure 3.13). Actual status of the null hypothesis (Ho) Ho is true Ho is false o - Reject H6 r-_> Type I error e;a i power "S:,t:.itl ; j.i = (ProbabilitY = q; (ProbabilitY=1-F) o ll G tr.9.2 () Failto r Appropriateconclusion i Type ll error o o reject Ho t* (ProbabilitY= 1-o) (probability = B) "Statistical power is the ability to detect (ie, correctly reject) a false H6. =,:ure 3.13 Statistical oower. 83 ehapt*r 3: l)*signing and lntong:reting fixp*rimen:' (o)...However, because o represents the statistical power increases with increased significance level probability of type I error, increasing q resulti in more false-positive conclusions' Researchers can the probability of false-positive conclusions increase statistical power in an experim ent without increasing experiment' by increasing the sample size of the 'ir**1n1gt.1i ;]nii {.;rii:'f fi;i tii{jt!i'-;; in the #omcept #he*$-. 3"H "decreases," "increases," "type l"' and Fill in the blanks in the sentences below with the words "type ll." as statistical power The probability of committing a error increases, which occurs wnJn the level (o) and/or when the sample "g*fican"e probability of a error' size increases. Increased q results in an increased Stllutie';'ti Note: The appendix contains the answer'. - - 3 2 05 Confidence samples are investigated to reach Biological research entails performing experiments in which conclusions l'r]!iexrcllri!.l ;-:i;lr,;i:.:iii:ril; from which the samples were taken' Numerical values that describe mean), whereas numericalvalues that describe samples are referred to as statistics (eg, sample mean). Parameters are often unknown in biological poputationsare called parameters (eg, population typically not available' research because data from entire populations are level (eg, 95%), gives a rang€ A confidence inferval, which is calculated using a specified confidence using data obtained fron *iir.,in *r.li"r' an unknown parameter is-likely found and is determined "i ""ir", The confidence interval contains a statistic that describes the sample (ie, point estimate) an a sample. the uncertainty associated with using a a margin of error on either side of the statistic that reflects sampte to estimate a population parameter (see Figure 3"14)' i"'iariiir.i :.:! =rriii f.irii: t,lsl:l1.lnle Width of confidence intervai Upper Lower confidence confidence limit limit A confidence interval is a range of plausible values for an unknown population parameter Figure 3.14 Components of a confidence interval' represents the percentage of repeated The confidence level used to calculate a confidence interval generate confidence intervals containing the tru' random samples taken from the population that would population parameter. That is, if confidence intervals were calculated from random samples taken these intervals that contain the true numerous times from the same population, the percentage of 84 Chaptnr 3: il*signing and Interpr*ting Hxpcrirn*nt* population parameter would be approximately equal to the confidence level. Figure 3.15 illustrates confidence intervals calculated at the 90% confidence level. True population parameter Eighteen of the twenty samples (90%) have confidence intervals that the true Rotula:lon pa:mel-er lnclude : Figure 3.15 90% confidence intervals. l.:'rfidence intervals can be calculated using measurements of standard error (SE), which pertain to the reliability (ie, precision) of a statistic, such as a sample mean. For example, standard error of the rnean SEM) is a measure of the variability of means obtained from multiple equally sized random samples :=ivn from a population. ln other words, SEM indicates the spread of the sample means (ie, how far -'n the population mean the samplepopulationmeans tend to fall). As such, SEM provides a measurement of -':ertainty around an estimate of a mean. l':nfidence intervals can also be,used to determine statisticalsignificance. Mathematically, the ::'fidence level is equal to 1 minus the significance level (ie, 1 - o). Therefore, if q = 0.05, the ::,rfidence level is 95% (ie, 1 - 0.05 = 0.95). As such, if error bars representing the 95% confidence n='val on a graph do not overlap, the results are statistically significant (see Figure 3.16). Likewise, =--:'bars representing t2 SEM on a graph can be interpreted in the same way because, for large --ples, the 95% confidence interval is calculated as the sample mean plus or minus 1.96 (ie, -2) times :e standard error of the mean. Error bars representing the 95% confidence interval account for measurement enor by providing, with 95% certainty, the range of values in which the true value for a population likely lies Measurement 1 Measurement 2 Measurement 1 Measurement 2. True population values may be the. True population values are likely same for both measurements not the same. Measurements may or may not. Measurements are considered be statistically different statistically different =Eure 3.16 Error bars for the 95% confidence interval. B5 Chapter 3: D*signing and Interpreting Hxperime 3.2.06 ValiditY is intended to te Experimental validity is a measure of the extent to which an experiment tests what it the true state of the scientific issue being and the extent to wrricn the experimental results represent are likely to be trusted (ie, accepted by the studied. lf a scientific experiment is valid, its conclusions scientific community) and applied in scientific decision-making and further research. and applicability c Various types of validity, including the following general types, affect the acceptance biological research findings: o Internal validity is the extent to which the observed effects in an experiment can be attributed to (eg, factor being studied (ie, the independent variable) as opposed to extraneous factors outcome. Experiments with high internal validity confounding varlables) that can influence the provide convincing evidence that causal relationships exist between the experiments' independer and dePendent variables. o External validity pertains to the suitability of applying experimental findings to a more generalize justifiably be applied to a context. tfre resuits of an experiment with high external validity can broader population than that sampled in the experiment' correlated; that is, as o The internal and external validity levels'of an experiment tend to be negatively increases the other decreases. This ndgative correlation exists because factors that increase internal generalizability of the validity (eg, highly controlled laboratory conditions) tend to decrease the of internal exoerimental results to real-world situations. Figure 3.17 summarizes the characteristics validity and external validitY. Internal validitY External validitY Extent to which an exPeriment Extent to which an exPeriment's accuratelY demonstrates and findings can be iustifiablY generalized to a broader context r rneasures a causalrelationshiP ' between vartabbs (ie, in the real world) Contributing factors Contributing factors. Comparison between treatment. Random samPling and control groups. Minimization of differences Random assignment of subjects between exPerimental conditions to treatment and control groups and real-world conditions Rigorous control of extraneous. Replication of experiments variables Figure 3.17 Internal and external validity of scientific experiments. 3.2.07 Statistical Conclusions problems. Scientifi The goal of scientific research is to arrive at valid conclusions regarding scientific generated in scientific experiments. The degre' conclusions are based on statistical analyses of data (ie, accurate, appropriate) is referred to as which these statistics-based conclusions are reasonable statistical conclusion validity (SCV). the degree to w SCV is influenced by various factors, including an experiment's statistical f:ower and met. lf an experiment has low statistical power, SCV is negatively statistical test assumptions are tests are applied to a data set in an attempt to find a test thal affected. Likewise, if multiple statistical without accordingly adjusting the error rate (ie, significan provides a favorable result (ie, data fishing) ievel), SCV decreases. Figure 3.18 summarizes factors that contribute to SCV' 86 Chapter 3: Designing and Interpreting Experiments 3.18 Factors that contribute to statistical conclusion validity.