Behavioral Science S1 PDF
Document Details
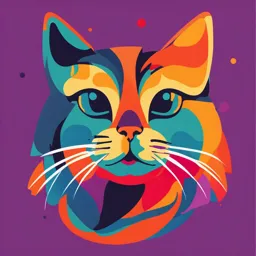
Uploaded by MercifulPiano5661
Universität Basel
Tags
Summary
This document provides an introduction to experimental design in behavioral science. It defines key concepts such as independent and dependent variables, and discusses different types of experiments. The document also delves into experimental versus correlational methods and concludes by discussing various experimental design types.
Full Transcript
S1. Introduction Snoddgrass, pp.18-30: Independent variable = the one that is manipulated Dependent variable = outcome. The question of interest is whether the behavior we measure is dependent on the variable we manipulated. You can think of the independent variable as the...
S1. Introduction Snoddgrass, pp.18-30: Independent variable = the one that is manipulated Dependent variable = outcome. The question of interest is whether the behavior we measure is dependent on the variable we manipulated. You can think of the independent variable as the cause of the behavior, and the dependent variable as its e ect. The hypothesis is tested by an experiment. Two Types of Experiments: True experiments: 1) there be at least two conditions or groups (experimental group and control group); 2) the independent variable is manipulated by the experimenter. Correlational or observational studies: 1) both variables whose relationships are sought can take on a number of di erent values; 2) one variable does not necessarily have to depend on or be caused by, the second variable (correlation is not causation) Arti cial (the experimenter has complete control over which subject is given which treatment) and natural (the experimenter has no control, independent variables are properties of the subject) experiments There is a natural experiment for every arti cial experiment. A confounded variable is one that is correlated with the independent variable and thus can be responsible for the e ect in the question. Experimental Versus Correlational Methods In a natural experiment, the variable in the question is divided into two or more categories. These categories may be truly dichotomous (male, female) or may lie along a continuum but be divided into groups by the experimenter (whether the person drank co ee for lunch or not). When we measure the degree of relationship between two continuous or quasi-continuous variables, we use a rule correlational method. Independent, Control, and Dependent Variables A Variable is anything that can take on a number of values. Independent variables are those that are either manipulated or selected by the experimenter; Control variables are those held constant by the experimenter across the conditions of an experiment (subject variables: sex, intelligence, age, socioeconomic status, family history, etc; environmental variables: room in which the experiment is conducted, the sex and attitude of the experimenter, the time of the day, time of the year, etc); Dependent variables are those measured by the experimenter as some selected behavior of the subjects (people participating) An independent variable can take on any number of values (called levels), but the minimum number is two. One of the levels is often the control condition in which nothing is manipulated. Types of Experimental Designs We can classify experimental designs along at least three dimensions: 1) the number of independent variables that are manipulated; fi ff ff ff fi ff 2) The number of levels of the independent variable or variables that are used. Levels are the values of the independent variable that are used in the experiment. Each value or level is also called a condition; 3) Whether the same subjects receive all conditions or di erent subjects are randomly assigned to the di erent conditions. Randomized design or randomized groups design: di erent subjects are assigned at random to the di erent conditions of an experiment. Repeated measures or matched groups design: same subjects participate in all conditions of an experiment or di erent subjects are matched on some basis across conditions. Matched group design: if the number of conditions or levels is small (usually only two) subjects are paired on some set of criteria related to the dependent variable. Mixed design: when there are two independent variables, one may be randomized and one may be matched. Generally, matched designs are preferred to randomized designs (the same subject or pair of subjects functions as their own controls, automatically controlling for the potentially confounding e ects of variables such as IQ, genetic history, and environment). Dependent variables are normally measured on an interval scale: it’s possible to compute averages (arithmetic means) on the data. Examples: number of correct responses, reaction time, and response on a seven-point rating scale of the questionnaire. These three aspects of the experimental design determine the appropriate statistical test. A Single Independent Variable Random Groups Design with Two Levels: Two levels of a single independent variable, two independent groups of subjects. Examples: comparing reaction time performance of neurotic and normal subjects. The independent variable is subject status (neurotic versus normal). There are two levels, or conditions, of the independent variable, but a single subject participates in only one condition. The appropriate statistical test is an independent groups t test. Matched or Related Measures Design with Two levels: Two levels of a single independent variable, a single subject participates in both conditions or each of two subjects paired on some criterion participates in one condition (statistically, members of a matched pair are treated as though they were the same person - the scores are paired). In a matched or repeated measures design, we have the advantage of statistically controlling some of the variance resulting from individual di erences. Example (same subject participates in both conditions): comparing the speed of learning a list of words for the same subject under alcohol and no-alcohol conditions. Independent variable: alcohol consumption. Example of experiment in which subjects are paired or matched: comparing the IQs of the rst and second-born children from the same families. Independent variable: birth order. In both designs, the appropriate statistical test is a matched pairs t test. Random Groups Design with More than Two Levels: ff ff ff ff ff ff fi ff More than two conditions of an independent variable, a di erent group of subjects is run in each condition. Example: comparing school performance of students in four di erent educational programs (e.g., open classroom, individualized studies, etc.). The independent variable is the educational program. A single subject participates in only one condition. The appropriate statistical test is a one-way randomized analysis of variance (ANOVA). Repeated Measures Design with More than Two Levels: More than two conditions of a single independent variable, each subject participates in all conditions. Example: comparison of patients on a memory task before, during and after therapy. The independent variable is status of therapy. The appropriate statistical test is a one-way repeated measures analysis of variance. Two Independent Variables Example: we are interested in both type of drug and drug dosage: we might want to test four types of drugs at each of three dosage levels - low, medium and high - in all possible combinations. This results in a total of twelve (4x3 = 12) conditions. When there are two independent variables, both may be random, both may have repeated measures, or one may be random and one may have repeated measures. Regardless of whether one or both factors (independent variables) have repeated measures, each may vary on any number of levels. If we let a refer to the number of levels of factor A and b refer to the number of levels of factor B, then the total number of di erent conditions in a two-factor experiment is equal to the product of a x b. Completely Randomized Design: Example: subjects are asked to learn a set of material and then are tested for recall at one of three retention intervals - immediately, one hour later, and 24 hours later. The investigator also manipulates the meaningfulness of the material, using both sentences and nonsense syllables. Each subject is tested with only one retention interval and one type of material, so this is a completely randomized 3 x 2 design. Completely Repeated Measures Design: Example: recall of words as a function of both their imagery and their frequency in print is measured, in which two levels of imagery (high and low) and three levels of frequency (high, medium, and low) are factorially combined in a 2 x 3 design. Each subject is tested on his or her ability to memorize words from all six categories. Mixed Design: Often used when one of the variables is nature’s own, such as sex, race, birth order, or some personality characteristics (they can be selected, but not manipulated). These become the random-group independent variable, subjects are assigned only to one group (male or female, etc). ff ff ff Interaction in Two-Factor Experiments: One of the major purposes of manipulating two independent variables is to determine whether they interact. By interaction, we mean that the e ect of moving from one level to another in the st factor is not the same for each level of the second factor. For example, adding salt to unsalted pork roast usually increases taste preference, whereas adding salt to unsalted ice cream usually decreases taste preference. In this case, salt interacts with food type. Shadish, Cook, & Campbell (2002): pp: 3-12: De ning Cause, E ect, and Causal Relationships That which produces any simple or complex idea, we denote by the general name cause, and that which is produced, e ect. A cause is that which makes any other thing, either simple idea, substance or mode, begin to be; and an e ect is that, which had its beginning from some other thing. Inus condition - an insu cient but non-redundant part of unnecessary but su cient condition. Most causes are more accurately called inus condition. Many factors are usually required for an e ect to occur, but we rarely know all of them and how they relate to each other. To di erent degrees, all causal relationships are context dependent, so the generalization of experimental e ects is always at issue. In an experiment, we observe what did happen when people received a treatment. The counterfactual (contrary to fact) is knowledge of what would have happened to those same people if they simultaneously had not received treatment. An e ect is the di erence between what did happen and what would have happened. How do we know if cause and e ect are related? A causal relationship exists if (1) the cause preceded the e ect, (2) the cause was related to the e ect, and (3) we can nd no plausible alternative explanation for the e ect other than the cause. These three characteristics mirror what happens in experiments in which (1) we manipulate the presumed cause and observe an outcome afterward; (2) we see whether variation in the cause is related to variation in the e ect; and (3) we use various methods during the experiment to reduce the plausibility of other explanations for the e ect, along with ancillary methods to explore the plausibility of those we cannot rule out. Experiments are the best method to study causal relationships (better than correlational studies, cause sometimes it’s impossible to know which of two variables came rst). Correlation does not prove causation. Experiments explore the e ects of things that can be manipulated (dose of a medicine, number of children in a classroom, etc). Nonmanipulable events (e.g., the explosion of a supernova) or attributes (e.g., people’s ages, their raw genetic material, biological sex) cannot be causes in experiments. Analogue experiments can sometimes be done on nonmanipulable cause, that is, experiments that manipulate an agent that is similar to the cause of interest (instead of changing a person’s race (impossible), it’s possible to chemically induce skin pigmentation changes in volunteer individuals). Causal description - describing the consequences attributable to deliberately varying a treatment ( icking a light switch - obtaining illumination) Causal explanation - clarifying the mechanisms through which and the conditions under which that causal relationship holds (closing an insulated circuit). Experiments are very good with causal descriptions but do less well with causal explanations. So causal explanation is an important route to the generalization of causal descriptions because it tells us which features of the causal relationship are essential to transfer to other situations. There is a close parallel between descriptive and explanatory causation and molar (meaning something takes as a whole rather than in parts. An analogy is to physics, in which molar might refer to the fi fl ff ff ff fi ff ff ff ffi ff ff ff ff ff ff ff fi fi ff ffi ff ff properties or motions of masses, as distinguished from those of molecules or atoms that make up those masses) and molecular causation. Optional: Introduction from Kahneman Introduction to the topic of the book: intuition mistakes, errors of judgment and choice. History of how Kahneman worked with his colleague Amos Tversky: The whole topic started when the professors tried to answer the question «Does a human have an intuitive understanding of statistics?» They found out that the answer is no, but with certain reservations. Our intuition is unreliable. But we make many decisions and assumptions based on our intuition. Kahneman and Tversky started studying heuristic errors (heuristics is understood as a set of techniques and methods that facilitate and simplify the solution of cognitive, constructive, practical tasks). They faced the following heuristic distortions (mistakes in predictions): - judging by similarity (instead of statistical facts); - heuristic accessibility: the desire to rely on the ease of sorting information in memory. We judge statistical probability and frequency by how easy for us it is to remember a similar case); Normal tasks where we make mistakes by using heuristics: calculating the probability of events, predicting the future, evaluating hypotheses, and predicting frequency. Before their study two positions were generally accepted. Firstly, people are mostly rational and, as a rule, think reasonably. Secondly, most deviations from rationality are explained by emotions: for example, fear, attachment or hatred. The new studies show that we cannot assume this: there are constant errors of thinking of normal people and they are caused more by the mechanism of thinking itself than by a violation of the thinking process under the in uence of emotions. Then they studied decision-making in conditions of uncertainty. They spent days inventing choice problems and looking to see if their intuitive preferences coincided with the logic of choice. Here, as well as in the study of value judgments, there were systematic deviations in their own decisions and intuitive preferences, which constantly violated the rational rules of choice. The article «Prospect Theory: An Analysis of Decision under Risk» has become a base for behavioral economics. Actual Studies: Slow and Fast Thinking How does expert intuition work? "The situation gave a hint, the hint gave the expert access to the information stored in memory, and the information gave an answer. Intuition is nothing but recognition.» Correct intuitive guesses arise when experts, having learned to recognize familiar elements in a new situation, act accordingly. - A ect heuristic: when decisions and judgments are made directly based on feelings of liking and dislike, with little or no thought or argument. When a person faces a new task and has the right knowledge, intuition recognizes the situation, and the intuitive solution that comes to mind is likely to be correct. When the question is di cult and there is no quali ed solution, intuition still has a chance: the answer will come to mind quickly, but it will be the answer to another question. The investment director was faced with a di cult question: "Should I invest money in Ford shares?" But his choice determined the answer to another question, easier and more akin to the original one: "Do I like Ford cars?" This is the essence of intuitive heuristics: when faced with a di cult question, we answer an easier one, usually without noticing the substitution. A spontaneous search for an intuitive solution is not always successful: from time to time neither a rationally justi ed answer nor a heuristic guess comes to mind. In such cases, we often switch to a slower and deeper form of thinking that requires a lot of e ort. This is the "slow thinking" (System 2) mentioned in the book title. Quick thinking (System 1) includes both variants ff ffi ffi fi fi ffi ff fl of intuition, that is, expert knowledge and heuristics, as well as all those absolutely automatic actions of the brain in the eld of perception and memory that allow you to remember the capital of Russia or determine that there is a lamp on the table. The Book Structure The book studies the importance of System 1 and its interaction with System 2. It contains 5 sections: 1. System 1 and System 2 description 2. The problem: why is it so di cult to think statistically? 3. More details about di culties of statistical thinking (why we think we know the right answer when we’re mistaken) 4. Nature of decision-making. Why people’s decisions don’t follow rational rules (as assumed in economic theories), trends in the isolated consideration of problems, and the e ects of framing (setting frames), when decisions are made due to insigni cant features associated with the task of choice. 5. Di erences between two «me»: feeling «me» and remembering «me» and how their interest don’t coincide. ff ffi fi ffi fi ff