Behavioral Finance Notes PDF
Document Details
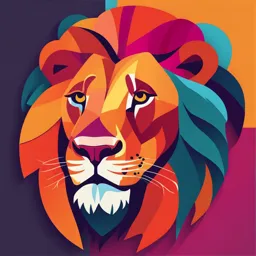
Uploaded by SoftAnemone3200
Tags
Summary
These notes cover behavioral finance topics, including mispricing in markets, and the impact of macroeconomic experiences on investor risk preferences.
Full Transcript
Limits to Arbitrage and Behavioral Finance Lamont and Thaler (2003): Can the Market Add and Subtract? Mispricing in Tech Carve-Outs Main Idea The paper tests for market mispricing after equity carve-outs, where a parent company partially spins off a subsidiary through an IPO. A mino...
Limits to Arbitrage and Behavioral Finance Lamont and Thaler (2003): Can the Market Add and Subtract? Mispricing in Tech Carve-Outs Main Idea The paper tests for market mispricing after equity carve-outs, where a parent company partially spins off a subsidiary through an IPO. A minority of the subsidiary’s shares are sold to the public, while the majority are retained by the parent and distributed to its current shareholders at a specified ratio. 𝑃0 𝑝𝑎𝑟𝑒𝑛𝑡−𝑥∗𝑃𝑂 𝑆𝑢𝑏𝑠𝑖𝑑𝑖𝑎𝑟𝑦 The value of the stub is calculated as: 𝑠𝑡𝑢𝑏 = → if the stub is negative, it signals a gross mispricing, 𝑃0 𝑃𝑎𝑟𝑒𝑛𝑡 as the implied value of the parent’s remaining business is less than zero. Example: In the 3Com-Palm case, 3Com’s implied stub value was deeply negative, even though 3Com’s non-Palm assets were valuable. Findings 1. Mispricing Evidence: Multiple cases of negative stubs were identified, with mispricing persisting for months. For 3Com-Palm, the stub was negative immediately after Palm's IPO, implying that 3Com's non-Palm assets had a negative $22 billion valuation. o Mechanisms: - Short-Sale Constraints: Shorting Palm shares was costly or unavailable due to institutional frictions, limiting arbitrage opportunities, - Demand-Driven Pricing: Irrational investor demand for Palm shares, driven by speculative sentiment and the "greater fool theory," pushed its price above fundamental value. 2. Investment Strategy Performance: Theoretical arbitrage strategy: short the subsidiary and long the parent to profit from the mispricing. This yielded high returns, with 3Com's returns exceeding Palm’s by 30-33% on average. Short interest (shares sold short/total shares) increased over time, reflecting rising costs and scarcity of shares for borrowing o Mechanisms: - Synthetic Shorting with Options: Arbitrageurs could create synthetic short positions using call/put options, but deviations from put-call parity (caused by short-sale constraints) reflected the limits of this strategy. - Market Segmentation: Institutional ownership reduced share availability for shorting, while high turnover indicated liquidity constraints. 3. Persistence of Mispricing: Mispricing remained for months despite high visibility in financial media. Noise trader risk and speculative demand delayed the correction of the stub to fundamental value o Mechanisms: - Noise Trader Risk: Speculative activity by irrational investors increased volatility and prolonged the price deviation. - Sluggish Adjustment: Limits to arbitrage, such as high implementation costs and market frictions, slowed the market’s ability to correct the mispricing. Conclusions Violations of Market Efficiency: Negative stubs represent clear evidence of market inefficiencies and the violation of the law of one price. Limits to Arbitrage: Practical constraints, particularly short-sale costs and institutional frictions, prevented arbitrageurs from correcting the mispricing, resulting in persistent anomalies. Investor Irrationality: Speculative behavior (e.g., the greater fool theory) led to systematic deviations from fundamental values. Market Segmentation: Constraints on arbitrage activity created fragmented markets, where subsets of irrational investors could drive prices further away from fundamentals. Malmendier & Nagel (2011): Depression Babies: Do Macroeconomic Experiences Affect Risk Taking? Main Idea The paper examines how individuals' macroeconomic experiences, particularly past returns in stock and bond markets, shape their risk preferences and financial behavior. Using data from the Survey of Consumer Finances (1960–2007), it shows that individuals who have experienced low stock returns demonstrate lower risk tolerance, reduced stock market participation, and smaller allocations of liquid assets to stocks. Similarly, low bond returns correlate with lower bond market participation. The experience hypothesis argues that personal financial decisions are heavily influenced by past macroeconomic events, with recent experiences having stronger effects (recency bias), though distant experiences still matter. Findings 1. Relationship Between Experienced Returns and Risk Preferences: Individuals with higher experienced stock market returns show higher risk tolerance and greater willingness to take financial risks. o Mechanism: A positive and significant weighting parameter (λ\lambdaλ) indicates that recent experiences are weighted more heavily when forming risk preferences, reflecting recency bias. However, even distant returns retain some influence, showing persistence of past experiences in shaping behavior. 2. Stock Market Participation: Experienced stock returns are positively correlated with stock market participation rates. A shift from the 10th to 90th percentile of experienced returns (6.2% to 11.9%) increases stock market participation by 10.2 percentage points. o Mechanism: High past returns lead to greater optimism about future returns (beliefs channel). These expectations encourage stock market participation and increased allocation of liquid assets to stocks. 3. Stock vs. Bond Allocations: Individuals increase stock exposure relative to bonds when historical stock returns outperform bond returns. Conversely, low bond returns discourage participation in the bond market. o Mechanism: Cognitive reinforcement from past investment payoffs (behavioral learning) influences asset allocation. Stock market optimism drives substitution effects, leading investors to prefer stocks over bonds. 4. Actual vs. Counterfactual Participation: The study compares actual participation rates to counterfactual scenarios where all returns are weighted equally. Notable differences emerge, especially after booms (e.g., 1960s, 1990s) and recessions (e.g., late 1970s). o Mechanism: Experienced returns have a significant causal effect on participation rates, indicating that lifetime financial behavior is not fully explained by rational models of risk. Conclusions Macroeconomic Experiences Shape Risk-Taking: Individuals’ willingness to take risks is significantly influenced by lifetime asset returns, with a stronger impact from recent experiences. Behavioral Biases Drive Financial Decisions: Recency bias causes individuals to overweight recent returns while underweighting historical data, leading to systematic deviations from rational expectations. Persistent Effects of Major Events: While recent events dominate, distant experiences like the Great Depression leave a lasting, albeit fading, imprint on financial behavior over decades. Implications for Behavioral Finance: Beliefs and preferences are dynamic and shaped by experiences, challenging traditional models of static risk preferences. The findings underscore the need to account for behavioral learning and psychological biases in understanding asset pricing and market behavior. Corporate Finance – Mergers and Acquisitions Rhodes-Kropf, Robinson, and Viswanathan (2005): Valuation Waves and Merger Activity Main Idea The paper investigates how market misvaluation (measured by high market-to-book ratios, M/B) drives merger waves and influences merger activity, the acquirer-target relationship, and the method of payment. The market-to-book ratio (M/B) is decomposed into: 1. Firm-specific deviations from contemporaneous sector pricing. 2. Sector-wide deviations from long-run pricing. 3. Long-run value to book (fundamental value). The study tests behavioral and rational theories explaining merger waves, including: o Neoclassical Q Theory: High M/B ratios reflect productive redeployment of assets. o Correlated Asymmetric Information (RKV): Valuation errors correlated with synergy errors lead to overestimated synergies. o Behavioral Theory (Shleifer-Vishny): Overvalued acquirers use overpriced stocks to acquire less overvalued targets, benefiting short-term target managers. Findings 1. Overvalued Firms as Acquirers: Overvalued firms acquire less overvalued firms, typically during market or sector valuation waves. o Mechanism: Firm-specific errors are higher for acquirers than targets. Rational target managers misinterpret high valuations as evidence of synergies, consistent with RKV. Behavioral biases drive overvaluation, as acquirers use overpriced stocks to acquire. 2. Role of Payment Method: Stock-based transactions dominate during overvaluation waves. Cash targets are undervalued, stock targets are overvalued. Cash acquirers are less overvalued than stock acquirers. o Mechanism: Stock is preferred when acquirers are significantly overvalued. Rational target managers accept overvalued stock under asymmetric information (RKV), while behavioral theory suggests short-term gains motivate target managers (Shleifer-Vishny). 3. Merger Intensity and Sector Overvaluation: Merger waves cluster in sectors with high time-series valuation errors. o Mechanism: Sector-wide overvaluation drives aggregate merger intensity, consistent with the hypothesis that sector-level bubbles fuel M&A activity. 4. Mismatch in Growth Prospects: Low long-run value-to-book acquirers buy high value-to-book targets. o Mechanism: Overvalued acquirers seek growth opportunities, but these deals often destroy long-term value due to misvaluation. 5. Counterexamples to Q Theory: High M/B firms acquire other high M/B firms, contradicting neoclassical predictions of "high buys low." o Mechanism: Short-term valuation errors dominate, overshadowing long-run growth opportunities. Conclusions Misvaluation as a Key Driver of M&A: Overvaluation explains approximately 15% of merger activity, particularly in overvalued sectors and during merger waves. Transaction Medium Matters: Stock-financed deals reflect higher valuation errors, aligning with behavioral predictions about overvaluation exploitation. Acquirer-Target Dynamics: Acquirers have higher firm-specific valuation errors than targets, consistent with both behavioral and rational theories. Implications for Behavioral Finance: o Behavioral Biases: Managerial overconfidence and short-termism exacerbate misvaluation-driven mergers. o Limits to Rational Models: Neoclassical Q Theory cannot fully explain merger activity during valuation waves. Baker, Pan, Wurgler (2012): The Effect of Reference Point Prices on Mergers and Acquisitions Main Idea The paper examines whether recent peak stock prices of targets act as reference points (anchors) in determining M&A offer prices, affecting the probability of deal success and the reaction of bidder shareholders. Offer prices often cluster around 52-week highs, despite these peaks lacking fundamental relevance. The study investigates the role of anchoring bias in: 1. Offer price determination. 2. Deal success probability. 3. Bidder shareholders' announcement returns. 4. Merger waves during high market returns. Findings 1. Anchoring Bias in Offer Prices: Offer prices cluster at or just above 52-week highs, showing a statistically significant jump when exceeding this reference point. o Mechanisms: - Target’s Perspective: Target managers use the 52-week high as a benchmark to justify negotiation stances or mitigate litigation risks. - Bidder’s Perspective: Anchors simplify valuation and justify offers to shareholders, especially during valuation uncertainty. 2. Probability of Deal Success: The probability of deal success increases by 4.4% to 6.4% when the offer exceeds the 52-week high. o Mechanism: Psychological loss aversion: Target shareholders are reluctant to sell at prices below the recent high, seeing it as a “loss” compared to the peak. 3. Bidder Shareholder Reaction: Bidder shareholders react negatively when the offer price is tied to the 52-week high. A 10% increase in the premium linked to the 52-week high reduces the bidder’s announcement return by 2.45%. o Mechanism: Bidder shareholders view price peaks as a signal of overpayment, suggesting value destruction rather than synergies. 4. Merger Waves and Anchors: Merger waves align with periods of high market returns, where more targets trade closer to their 52-week highs, making peak-based offers easier to justify. o Mechanism: High market valuations encourage anchoring, as targets near their reference points are perceived as easier to acquire at "fair" prices. Conclusions Anchoring in M&A Offer Prices: The 52-week high serves as a reference point, significantly influencing offer prices, even though it lacks fundamental economic justification. Impact on Deal Success: Anchoring increases the likelihood of deal completion, reflecting psychological biases in negotiation and decision-making. Bidder Shareholder Concerns: Anchoring-based offers lead to negative reactions from bidder shareholders due to perceived overpayment. Implications for Behavioral Finance: Anchoring highlights bounded rationality in M&A activity, challenging traditional valuation-based models. Giglio and Shue (2014): No News is News: Do Markets Underreact to Nothing? Main Idea The paper explores how markets incorporate the information contained in the passage of time during merger announcements. Specifically, it analyzes how variations in hazard rates (probabilities) of merger completion and withdrawal affect returns. It finds that completion hazard rates vary significantly over time (hump-shaped), while withdrawal hazard rates remain constant. Investors underreact to the informational content of time passage, leading to predictable return patterns after merger announcements. Findings and Mechanisms 1. Hazard Rates and Completion/Withdrawal: Completion hazard rates rise to 6% per week by event week 20, then decline to zero by the end of the first year. Withdrawal hazard rates remain flat at 1%. o Mechanism: The time passed since announcement serves as a proxy for deal likelihood. When no explicit news is reported, the market incorrectly assumes constant probabilities, failing to adjust prices based on real hazard rates. 2. Market Returns and Hazard Rates: Returns are positively correlated with hazard rates. When hazard rates are high, returns are high; when hazard rates are low, returns are low. o Mechanism: Behavioral underreaction: Investors underestimate the probability of completion when hazard rates are high and overestimate when they are low, resulting in mispricing. Rational markets would adjust prices to reflect updated probabilities, but bounded rationality delays this adjustment. 3. High vs. Low Hazard Periods: Investment strategies focusing on high-hazard periods yield alphas of 10.5%, significantly higher than strategies focusing on low-hazard periods (3.3%). o Mechanism: High-hazard periods benefit from market surprises as deals complete faster than anticipated. Limits to arbitrage prevent full exploitation of these anomalies, particularly for small-cap, illiquid stocks. 4. Testing Rational Explanations: Risk factors (systematic, downside, and idiosyncratic risks) and trading frictions fail to explain the observed return patterns. o Mechanism: Rational models (e.g., varying risk premia) do not account for the event-time variation in returns. Behavioral underreaction provides a better explanation. Conclusions Behavioral Underreaction: Markets fail to fully incorporate the informational content of time passage after merger announcements. Returns are predictable based on hazard rates, which contradicts market efficiency. Implications for Arbitrage: Limits to arbitrage, such as high transaction costs and low liquidity, restrict sophisticated investors from fully correcting mispricing. Abnormal Returns: The strategy focusing on high-hazard periods delivers consistent excess returns, highlighting opportunities for active trading strategies. Corporate Finance – CEO Overconfidence Ben-David, Graham, and Harvey (2013): Managerial Miscalibration Main Idea The paper investigates managerial miscalibration, defined as the systematic underestimation of the range of potential outcomes, reflecting excessive confidence in predictive accuracy. Miscalibration arises because: o Managers overestimate their ability to predict the future. o Managers underestimate the volatility of random outcomes Using a 10-year dataset of CFO forecasts for S&P 500 returns, the paper measures miscalibration and examines its impact on corporate actions such as investment intensity, leverage, and project return forecasting (IRR). Findings 1. Are CFOs Miscalibrated? Finding: Only 36% of realized S&P 500 returns fall within CFOs' 80% confidence intervals, far below the expected 80%. o Mechanism: - Overprecision: CFOs underestimate the range of possible outcomes, narrowing their confidence intervals. - Volatility Underestimation: Imputed volatility from CFO forecasts is centered at 4%, while realized volatility is around 12%. - Market Conditions: Miscalibration worsens during volatile periods (e.g., high VIX), as CFOs fail to adjust forecasts appropriately. 2. Impact on Corporate Planning and Forecasting Miscalibration spills over to corporate decision-making, with narrower project return estimates, higher investment intensity, and greater leverage. o Mechanism: - Behavioral Spillover: CFOs carry their overconfidence into project planning, leading to over-optimistic IRR estimates. - Overconfidence in Risk Management: Miscalibrated market forecasts reinforce bold corporate policies, especially in investment-heavy firms. 3. Effect on Corporate Investments and Leverage Long-term overprecision correlates with increased corporate investment, while short-term miscalibration drives higher leverage. o Mechanism: - Horizon Bias: CFOs perceive more control over short-term risks, leading to overconfident borrowing decisions. For long- term projects, they underestimate uncertainty but allow for modest parameter uncertainty, spurring excessive capital expenditures. 4. Do Miscalibrated CFOs Drive Corporate Policies or Vice Versa? Firms with miscalibrated CFOs exhibit bolder investment and leverage policies, but reverse causality cannot be entirely ruled out. o Mechanism: - Behavioral Transmission: Miscalibrated CFOs influence corporate strategies when taking office, although bold firm strategies might also reinforce CFO miscalibration. Conclusions CFOs Are Severely Miscalibrated: They underestimate market volatility, particularly during volatile periods, and overestimate their predictive abilities. Spillover to Corporate Policies: Miscalibration affects internal rate of return estimates, leading to excessive investment and leverage. Behavioral Finance Implications: Miscalibration highlights the role of overconfidence in financial decision-making, with significant consequences for corporate governance and market dynamics. Malmendier and Tate (2005): CEO Overconfidence and Corporate Investment Main Idea The study examines the impact of CEO overconfidence on corporate investment behavior. Overconfident CEOs are characterized by their overestimation of their companies' potential and their reluctance to issue new equity due to the belief that their stock is undervalued. This leads to distortions in corporate investment: Overinvestment: When internal funds are abundant, overconfident CEOs tend to overinvest, as they overestimate project returns. Underinvestment: When reliant on external financing, they curtail investment due to perceived high costs of issuing equity. The key hypotheses are: 1. Investments by overconfident CEOs are more sensitive to cash flows compared to their less confident peers. 2. This sensitivity is more pronounced in equity-dependent (financially constrained) firms. Measuring Overconfidence Three measures are used based on CEO personal portfolio behavior: 1. Holder 67: CEOs are classified as overconfident if they hold stock options that are more than 67% in-the-money past the vesting period (year 5) at least twice. 2. Longholder: CEOs who hold options until their last year of expiration, showing an expectation of continued stock price increases. 3. Net Buyer: CEOs who are net buyers of their company’s stock during their first five years, indicating an unwillingness to reduce exposure to company-specific risk. Alternative explanations for these behaviors, such as insider information, signaling, lower risk aversion, tax motives, and procrastination, were tested and rejected. Findings 1. Cash Flow Sensitivity of Overconfident CEOs: Overconfident CEOs show a significantly higher sensitivity of investment to cash flow compared to their peers. o Mechanism: - Overconfident CEOs overestimate project returns and rely heavily on internal funds to finance investments. - Internal cash flows reduce reliance on external financing, mitigating the perceived "costliness" of equity issuance. - As a result, investment levels rise disproportionately with cash flow availability. 2. Impact on Equity-Dependent Firms: The cash flow sensitivity of investment is most pronounced in financially constrained firms (e.g., small, young firms, low credit ratings, low dividend payouts). o Mechanism: - These firms depend on equity for external financing, but overconfident CEOs are reluctant to issue equity due to their belief that the firm’s stock is undervalued. - This reluctance amplifies underinvestment when internal funds are insufficient, further constraining growth opportunities. 3. Consistency Across Measures of Overconfidence: Overconfidence, measured by Holder 67, Longholder, and Net Buyer metrics, consistently correlates with higher cash flow sensitivity of investment. o Mechanism: - CEOs holding options beyond rational benchmarks (Holder 67) or until expiration (Longholder) signal overconfidence in their ability to boost future stock performance. - CEOs who increase their equity holdings (Net Buyer) reveal their inflated optimism about their company’s value. - These personal portfolio behaviors spill over into corporate decision-making, reinforcing investment distortions. 4. Equity Aversion and Underinvestment: Overconfident CEOs curtail investment when external equity financing is required. o Mechanism: - The perception of equity undervaluation increases the perceived cost of external financing. - Overconfident CEOs thus prioritize internal funds, leading to underinvestment in equity-dependent scenarios. Conclusions Overconfidence Drives Investment Distortions: Overconfident CEOs overinvest when internal funds are available due to overestimated project returns and perceived low risk. Conversely, they underinvest when external financing is needed, as they view equity as undervalued. Higher Cash Flow Sensitivity: Investments by overconfident CEOs are more sensitive to cash flows than those of less confident CEOs, particularly in financially constrained (equity-dependent) firms. Equity Aversion: Overconfident CEOs’ reluctance to issue equity exacerbates underinvestment, especially for firms with limited access to debt or other funding sources. Consistency Across Measures: The findings are robust across all three measures of overconfidence (Holder 67, Longholder, Net Buyer), linking personal portfolio decisions to corporate investment behavior. Implications for Governance: Stronger corporate governance and independent oversight are necessary to counteract the inefficiencies caused by overconfident decision-making, particularly in firms where equity financing plays a crucial role. Hirshleifer, Low, and Teoh (2012): Are Overconfident CEOs Better Innovators? Main Idea The study explores whether firms hire overconfident CEOs because they might enhance shareholder value by fostering greater innovation. Overconfident CEOs are more likely to invest heavily in risky projects, particularly in innovative industries, where their biases may lead to higher growth opportunities. However, their tendency to overestimate cash flows and underestimate risks may result in suboptimal decisions in less innovative settings. Proxies for Overconfidence 1. Options-based measure: Based on the tendency of CEOs to retain vested options even when they are significantly in-the-money. This indicates confidence in the firm’s future performance. Persistent behavior implies overconfidence. 2. Press-based measure: CEOs are labeled overconfident if the number of media articles describing them as confident exceeds those using cautious terms. Only 8.12% of CEOs were classified as overconfident under this measure. Proxies for Innovation 1. Input Measures: R&D expenditures scaled by assets. 2. Output Measures: Number of patents. Total patent citations, adjusted for industry and year to account for time-truncation biases. Findings 1. Higher Stock Return Volatility Firms led by overconfident CEOs exhibit higher daily stock return volatility (by 20 basis points per day, translating to 3% annually). o Mechanism: - Overconfident CEOs are more likely to take on risky, uncertain projects that have a higher probability of extreme outcomes (either success or failure). - Their overestimation of project potential amplifies firm risk, leading to greater stock return variability. 2. Increased R&D Investment Overconfident CEOs allocate a higher proportion of assets to R&D expenditures. o Mechanism: - Overconfidence drives CEOs to view innovation as a source of competitive advantage and future growth. - Their belief in the firm's superior ability to innovate motivates them to prioritize investments in uncertain but potentially transformative R&D projects, even at the expense of short-term financial stability. 3. Higher Innovation Output (Patents and Citations) Overconfident CEOs are associated with 9%-28% more patents and 17%-40% more patent citations (adjusted for time and industry biases). o Mechanism: - Overconfident CEOs are more willing to commit resources to high-risk, high-reward innovative projects. - By embracing uncertainty, they enable projects that rational, risk-averse managers might avoid, leading to greater innovation output and recognition through citations. 4. Industry-Specific Effect of Overconfidence The positive impact of overconfidence on innovation output and efficiency is significant only in innovative industries (e.g., tech, pharmaceuticals). In less innovative industries, this relationship is not observed. o Mechanism: - Innovative industries offer more opportunities for growth through risky, exploratory projects, which align with the risk- taking tendencies of overconfident CEOs. - In non-innovative industries, overconfident CEOs are more likely to misallocate resources toward projects with lower returns or unnecessary acquisitions, leading to inefficiencies. 5. Causal Relationship Between Overconfidence and Innovation The positive effects of overconfidence on innovation persist even after excluding the initial years of a CEO's tenure, suggesting that results are not purely due to firms hiring overconfident CEOs for innovative roles. o Mechanism: - Overconfidence is a persistent trait that influences decision-making. Overconfident CEOs take on more innovation-oriented risks independent of the firm’s specific growth opportunities. - This persistence indicates that overconfidence itself, rather than an endogenous matching process (e.g., firms hiring risk- taking CEOs for innovative roles), drives the observed innovation outcomes. 6. Better Utilization of Growth Opportunities Overconfident CEOs in innovative industries effectively convert growth opportunities into firm value, as evidenced by higher Tobin’s Q and industry P/E ratios. o Mechanism: - By pursuing high-risk, high-reward projects, overconfident CEOs capitalize on innovation-driven growth opportunities, increasing firm value in industries where such opportunities are plentiful. - Their optimism helps overcome organizational inertia and risk aversion, allowing firms to remain competitive in rapidly evolving sectors. Conclusions Positive Effects in Innovative Industries: Overconfident CEOs enhance innovation and firm value by increasing R&D investments and innovation output. They are particularly effective in industries with strong growth opportunities, where their willingness to take risks aligns with the industry’s needs. Negative Effects in Non-Innovative Industries: In sectors lacking significant innovation opportunities, overconfident CEOs may misallocate resources to low-value projects, harming firm performance. Strategic Implications: Firms in innovation-driven industries benefit from hiring overconfident CEOs, while those in traditional industries must weigh the potential downsides carefully. Robust corporate governance is essential to mitigate risks associated with overconfidence in less suitable contexts. Investments – Financial Bubbles Hussam, Porter & Smith (2008): Can bubbles be rekindled with experienced subjects? Main Idea This paper explores whether price bubbles in experimental asset markets, eliminated by experience, can be reignited under modified conditions. The key focus is to test the robustness of learning and error elimination in asset trading when environmental variables like liquidity, participant composition, and dividend uncertainty change. Findings 1. Experience reduces bubbles in stable environments but not when conditions change. o Mechanism: In a stable environment, repeated exposure allows participants to internalize the fundamental asset value through reduced uncertainty about price behaviors. However, changes in liquidity or dividend uncertainty disrupt learned patterns, leading to speculative behavior and rekindling of bubbles. 2. High liquidity sustains bubble amplitude. o Mechanism: Increased initial cash relative to available shares fuels excessive bidding, creating a high amplitude (peak relative to fundamental value). This simulates real-world market conditions where excess liquidity supports prolonged overvaluation. 3. Variance in dividend distributions contributes to rekindling. o Mechanism: Higher variability in payouts increases uncertainty about future asset values. This uncertainty promotes speculation, as participants cannot confidently assess fundamental value, amplifying price deviations. 4. Mixing experienced and new participants reignites bubbles. o Mechanism: Introducing mixed cohorts disrupts collective learning from prior sessions. Experienced participants lose the stabilizing influence of trading with familiar peers, while new participants introduce fresh speculative behaviors. 5. Bubble characteristics evolve with changing conditions: o Amplitude: Rekindling treatments yield amplitudes comparable to inexperienced groups. o Turnover: Higher cash endowments drive frequent trades, increasing turnover to levels observed in inexperienced settings. o Duration: Bubbles are shorter in rekindled scenarios, as experienced traders foresee crashes and exit earlier. Experimental Design: 1. Baseline Treatment: o Asset with a 15-period lifespan. o Four potential dividends: {0, 8, 28, 60}; expected payout = 24. o Stable environment, repeated for 3 sessions with identical participant groups. 2. Rekindle Treatment: o Increased liquidity: Higher cash endowments, fewer shares. o Increased dividend variance: {0, 1, 8, 28, 98}; expected payout = 27. o Mixed participant groups in later sessions. Conclusions: Experience eliminates bubbles in stable environments but fails when critical market parameters, like liquidity or dividend uncertainty, change. High liquidity and payout variance are key drivers of persistent bubbles. Real-world implications include environments such as technology booms or quantitative easing, where bubbles may arise despite experienced participants. Griffin, Harris, Shu, and Topaloglu (2011): Who Drove and Burst the Tech Bubble? Main Idea The authors investigate the role of institutional and individual investors during the DotCom bubble (1997–2000). The study identifies: Institutional investors as primary drivers of the price run-up in technology stocks during the bubble. Individuals as the major buyers post-burst, reflecting poor timing. The study also examines whether institutions contributed to mispricing or traded based on fundamentals, using data on NASDAQ technology firms. Findings 1. Run-Up Phase (1997–2000): Institutions were the largest buyers, accounting for 63.6% of technology stock purchases, compared to 19.4% via mutual fund flows and 17% by individuals directly. o Mechanism: - Institutional purchases were discretionary, often targeting high-price-to-sales (P/S) ratio stocks, suggesting speculative behavior. - Hedge funds, independent investment advisors, and mutual funds were the most aggressive buyers. - Their participation increased demand, driving prices further from fundamental values. 2. Market Peak and Immediate Aftermath: Around the March 2000 peak, institutions began coordinated selling, while individuals, particularly discount brokerage clients, accelerated their buying. o Mechanism: - The sell-off aligns with the Abreu and Brunnermeier (2003) model, where rational arbitrageurs ride bubbles until a coordinated selling effort occurs. - Individuals exhibited poor timing by purchasing as prices collapsed. 3. Post-Peak Phase (2000–2001): Individuals accounted for 49% of tech purchases post-burst, while institutions shifted to non-tech stocks. o Mechanism: Institutions displayed greater sophistication by reallocating capital, leaving individuals holding depreciating assets. 4. Institutional Role in Mispricing: Institutional buying led to larger post-peak reversals in returns, especially for the largest firms (top 25% by market cap). o Mechanism: - Institutions moved prices away from fundamentals through aggressive trading, consistent with speculative motives. - For overpriced equity carve-outs, institutions actively purchased before clear mispricing was corrected, fueling bubbles further. 5. Response to News: Institutional trades correlated with returns on both news and no-news days, disproving the hypothesis that trades were purely reactions to news. o Mechanism: Institutional behavior contributed to momentum, amplifying price trends rather than aligning with fundamentals. Conclusions Role of Institutions: Sophisticated investors (e.g., hedge funds, mutual funds) actively participated in speculative trading during the run-up, undermining the notion that institutions always counteract mispricing. Institutions drove the bubble and burst by first inflating prices and then triggering the collapse via coordinated selling. Role of Individuals: Individuals exhibited poor market timing by buying during the crash, sustaining the demand for depreciating assets. Broader Implications: The results challenge the assumption of market efficiency and highlight the destabilizing potential of sophisticated investors. They also underscore the need for understanding speculative motives and coordinated arbitrage in bubble dynamics. Cheng, Raina & Xiong (2014): Wall Street and the Housing Bubble Main Idea The study investigates whether midlevel managers in securitized finance were aware of the housing bubble and an impending market crash in 2004–2006. Using personal home transaction data, it examines whether their behavior differed from control groups (S&P 500 analysts and lawyers) and tests four hypotheses: 1. Market timing: Securitization agents were more likely to divest homes during the bubble period. 2. Cautiousness: They were less likely to acquire additional housing exposure. 3. Performance: Their overall housing portfolio performed better. 4. Conservative consumption: Purchases were more conservative relative to their income. Findings 1. Securitization Agents Increased Housing Exposure Contrary to the cautious behavior hypothesis, securitization agents increased their exposure by purchasing second homes and upgrading to more expensive homes. o Mechanism: This behavior aligns with over-optimism and belief distortions common in their environment. Job- related incentives may have amplified their optimism, as rising house prices benefited their work, fostering groupthink and willful blindness about potential risks. 2. No Evidence of Market Timing Securitization agents did not divest homes during the bubble and failed to time the market compared to control groups. o Mechanism: The high transaction costs of divesting primary homes and lack of clear information on when the bubble would burst may have deterred them from taking action. Their behavior highlights limited belief in a nationwide crash or underestimation of systemic risks. 3. Performance Lagged Behind Control Groups Their housing portfolios underperformed equity analysts, with 4.5% lower returns. o Mechanism: Poor portfolio performance indicates they overestimated market stability and the persistence of housing prices. This reflects their belief distortions and reliance on short-term bonuses without adequately hedging risks. 4. Lack of Conservative Consumption Purchases during the bubble were not conservative relative to their incomes, even among second homes. o Mechanism: Securitization agents likely overestimated their future incomes, driven by high bonuses during the boom. The financial system's structural biases, which rewarded short-term gains over long-term sustainability, reinforced this behavior. 5. No Significant Location or Financing Differences Their transactions showed no unique patterns in terms of location or financing terms. o Mechanism: This suggests that their behavior was not based on specific market knowledge but rather influenced by widespread over-optimism within their professional and social environments. Conclusions Market Awareness: Private home transaction data provides little evidence that securitization agents anticipated the housing market collapse earlier than others. Belief Distortions: Findings emphasize how professional environments may foster collective overconfidence and willful ignorance, impacting individual behavior. Policy Implications: Altering compensation structures alone is insufficient; better financial instruments are needed to encourage awareness of tail-risk situations. Systemic Risk: The study raises concerns about Wall Street’s ability to process information rationally and highlights the interaction between distorted beliefs and incentives as drivers of the housing bubble. Investments – Individual Investors Frazzini and Lamont (2008): Dumb Money: Mutual Fund Flows and the Cross-Section of Stock Returns Main Idea The paper investigates how mutual fund flows from individual investors impact future stock returns. It reveals that: 1. Funds experiencing higher inflows (purchased more by individuals) subsequently underperform funds with outflows. 2. This behavior reflects the "dumb money" effect, where individual investors systematically invest in funds that have performed well in the past but underperform in the future. The authors link this behavior to behavioral biases and demonstrate that these patterns are economically significant, challenging market efficiency. Findings 1. Performance of High-Inflow Funds Funds with the highest inflows experience subsequent underperformance, while outflow funds outperform. o Mechanism (Behavioral Biases): - Trend Chasing: Individual investors extrapolate past returns into the future, allocating more to funds with high historical performance. - Overconfidence and Representativeness: Investors overreact to recent performance, mistakenly believing it reflects manager skill rather than luck or market trends. 2. Mutual Fund Flows and Stock Returns Stocks held by funds receiving large inflows experience lower future returns than stocks held by funds with outflows. o Mechanism (Limits to Arbitrage): Price Pressure Hypothesis: Large inflows into certain funds create upward price pressure on the stocks they buy, pushing prices above fundamental value. When inflows diminish, these overvalued stocks correct downward, leading to underperformance. 3. Economic Magnitude of the "Dumb Money" Effect The effect is significant—investing in low-inflow funds generates 0.96% higher returns annually than investing in high- inflow funds, even after controlling for risk factors. o Mechanism (Market Inefficiencies):Behavioral Trading Errors: Individual investors' flow decisions distort asset prices, creating predictable patterns that persist because sophisticated arbitrageurs are constrained by risks and costs of shorting overvalued stocks. 4. Investor Characteristics and Flows Retail investors (as opposed to institutions) drive the "dumb money" effect by disproportionately directing flows toward high-inflow funds. o Mechanism (Information and Behavior): Lack of Sophistication: Retail investors rely on backward-looking information (e.g., past performance rankings) without considering forward-looking fundamentals. 5. Implications for Fund Managers Fund managers have incentives to focus on short-term performance to attract flows, even if this strategy does not align with long-term investor interests. o Mechanism (Agency Problems): Career Concerns and Compensation: Fund managers prioritize strategies that attract inflows (e.g., holding trendy stocks) rather than maximizing long-term returns for clients. Conclusions Behavioral Biases Drive Flows: Retail investors allocate funds based on past performance, contributing to systematic mispricing in mutual fund markets. Impact on Returns: Stocks heavily bought by high-inflow funds subsequently underperform, reflecting temporary overvaluation. Market Inefficiency: The "dumb money" effect challenges market efficiency and highlights the persistent influence of behavioral biases in financial markets. Policy Implications: Financial education and improved disclosure may mitigate the adverse effects of biased fund flows on market outcomes. Chang, Solomon, and Westerfield (2016): Cognitive Dissonance, Delegation, and the Disposition Effect Main Idea The paper investigates the cognitive dissonance-based mechanism driving individual investors’ behavior, specifically the contrasting disposition effect for stocks (non-delegated assets) and reverse-disposition effect for mutual funds (delegated assets). Key findings are that delegation shifts the psychological cost of realized losses to fund managers, allowing investors to sell losing mutual funds while avoiding losses in individual stocks. Behavioral Mechanism The core mechanism revolves around cognitive dissonance, defined as the discomfort when holding conflicting beliefs or cognitions, particularly regarding self-concept. Investors manage this dissonance by: 1. Rationalizing their decisions to protect their self-image. 2. Shifting blame to external agents, like fund managers, in delegated assets. Findings 1. Disposition Effect in Stocks Investors exhibit the disposition effect for non-delegated assets like stocks, selling winners more frequently than losers. o Mechanism: Realizing losses directly harms investors' self-concept of being competent decision-makers, making them hold onto losers and avoid admitting mistakes. 2. Reverse-Disposition Effect in Mutual Funds In mutual funds, the disposition effect reverses; investors sell losing funds more frequently than winning ones. o Mechanism: Delegation allows investors to externalize blame to fund managers for poor performance, reducing the psychological cost of realizing losses. 3. Effect of Delegation on Asset Classes The reverse-disposition effect strengthens with greater perceived delegation. For passively managed funds (e.g., index funds), where the manager’s role is minimal, the effect weakens and aligns with stocks. o Mechanism: Delegation provides a scapegoat for poor outcomes, mitigating cognitive dissonance. 4. Experimental Evidence When investors are required to justify their initial investment decisions (story treatment), cognitive dissonance increases, amplifying both the disposition effect in stocks and the reverse-disposition effect in funds. o Mechanism: Justification highlights conflicting beliefs, intensifying the dissonance and the need for resolution. 5. Manager Salience and Reverse-Disposition Making fund managers more salient (fire treatment) increases the reverse-disposition effect in mutual funds. o Mechanism: Highlighting the intermediary role reinforces the psychological safety net, encouraging investors to sell losing funds. Conclusions Cognitive dissonance is a key driver of the disposition and reverse-disposition effects. Delegation influences investor behavior by providing psychological tools to shift blame. Implications for behavioral finance include understanding the psychological costs in financial decision-making and reconsidering portfolio management strategies for intermediaries. Sensoy (2009) – Performance Evaluation and Self-Designated Benchmark Indexes in the Mutual Fund Industry Main Idea The paper investigates the use of self-designated benchmarks in the U.S. equity mutual fund industry and their impact on investor behavior and fund flows. Self-designated benchmarks, chosen by mutual funds, often do not match their actual investment style. This creates "mismatched" benchmarks. Performance relative to these mismatched benchmarks significantly influences fund flows, even when better performance measures (such as those adjusted for fund style) are available. The evidence suggests these benchmarks are strategically chosen to boost cash inflows rather than to improve investors' evaluation accuracy. Findings 1. Prevalence of Mismatched Benchmarks: About 31.2% of mutual funds have benchmarks that do not align with their investment style. These mismatched benchmarks result in a lower R² (70.6%) compared to a better-suited "corrected" benchmark (82.6% R²), indicating less explanatory power of returns. o Mechanism: Funds strategically select benchmarks that make their performance look better to attract inflows. Investors may rely heavily on these self-designated benchmarks, despite their inefficiency in capturing the fund's style. 2. Impact on Fund Flows: Performance relative to mismatched benchmarks significantly influences subsequent inflows, even when controlling for more accurate measures like Fama-French alphas. Beating the mismatched benchmark leads to higher inflows, while failing it has little impact. o Mechanism: Behavioral biases such as bounded rationality (limited investor sophistication) and framing effects (investors focusing on relative performance rather than true risk-adjusted returns) drive investor decisions. 3. Rationality of Responses to Benchmarks: Investors' reliance on mismatched benchmarks does not align with theories of optimal performance evaluation, which prioritize risk-adjusted returns. o Mechanism: Behavioral finance insights suggest irrational investor behavior—misinterpreting benchmark performance as an indicator of fund quality—leads to capital misallocation. 4. Strategic Benchmark Choice by Funds: Funds with mismatched benchmarks benefit from increased flows (2.3% of assets per year), amounting to 14.6% of the average annual flow for these funds. Mismatched benchmarks are more common in high-fee and large funds, where the financial incentive to attract flows is stronger. o Mechanism: Principal-agent theory indicates funds exploit investor naivety to increase management fees, aligning managerial incentives with fund inflows rather than investor returns. Behavioral Concepts and Mechanisms Bounded Rationality: Investors lack the ability or resources to evaluate the appropriateness of benchmarks, making them susceptible to strategic framing by funds. Framing Effects: The way performance is presented relative to benchmarks influences investor decisions, irrespective of whether the benchmarks are suitable. Strategic Misrepresentation: Funds actively use mismatched benchmarks to create a perception of superior performance. Conclusions Investor Behavior: Investors, especially unsophisticated ones, heavily rely on self-designated benchmarks. This reliance results in suboptimal allocation of resources, as fund flows do not align with risk-adjusted returns. Fund Strategies: Mutual funds strategically select mismatched benchmarks to inflate perceived performance and attract inflows. Larger funds and high-fee funds are more likely to employ this strategy, leveraging their market position to exploit investor behavior. Implications for Market Efficiency: The findings challenge the market efficiency hypothesis by highlighting how mutual fund flows respond irrationally to mismatched benchmarks. Addressing these inefficiencies would require regulatory changes or better investor education on benchmark selection and fund evaluation. Investments – Institutional Investors Goyal and Wahal (2008) - The Selection and Termination of Investment Management Firms by Plan Sponsors Main Idea The paper investigates how pension fund plan sponsors make decisions regarding hiring and firing investment management firms. It examines: 1. Whether hiring decisions lead to superior returns post-hiring. 2. The opportunity costs of firing managers relative to the performance of hired managers. 3. Behavioral biases and institutional factors driving these decisions. The authors find that plan sponsors engage in return-chasing behavior when hiring but fail to achieve excess returns afterward. Similarly, fired managers often perform no worse than the newly hired managers. Findings 1. Hiring Decisions Plan sponsors typically hire investment managers based on superior past performance. However, post-hiring excess returns are zero on average. o Mechanism: This behavior reflects performance-chasing bias, a cognitive bias where decision-makers overemphasize recent performance trends without considering regression to the mean. 2. Firing Decisions Managers are fired for various reasons, such as underperformance, organizational changes, or firm-related issues (e.g., personnel turnover). Notably, the post-firing performance of terminated managers is statistically similar or better than newly hired managers. o Mechanism: Loss aversion and agency conflicts drive this process. Sponsors fire managers to signal accountability and deflect blame for underperformance. 3. Round-Trip Analysis In 412 cases of “round trips” (hiring a new manager and firing an old one), the opportunity costs of firing decisions are high. The returns of fired managers would have been similar to or better than those of newly hired managers after considering transition costs. o Mechanism: Overconfidence in their ability to time manager changes and job preservation motives drive this inefficiency. 4. Influence of Plan Sponsor Characteristics Larger plans tend to achieve better post-hiring returns, potentially due to better selection processes or negotiation leverage. Sponsors sensitive to "headline risk" (public scrutiny) show poorer performance, likely due to conservative or reactive strategies. 5. Transition Costs Transition costs (legal, administrative, and opportunity costs) can erode up to 2% of the mandate size during manager transitions. o Mechanism: These costs are underestimated in decision-making due to a focus on short-term outcomes over long- term strategy. Behavioral Mechanisms 1. Overconfidence: Sponsors believe they can time the market by hiring and firing managers effectively. 2. Loss Aversion: Sponsors terminate underperforming managers to avoid admitting failure. 3. Herding Behavior: Sponsors follow trends or recommendations from consultants, exacerbating return-chasing. Conclusions Plan sponsors’ hiring decisions fail to generate value due to reliance on past performance, which lacks predictive power for future returns. Firing decisions often lead to opportunity losses, as fired managers perform comparably to newly hired managers. Behavioral biases, including overconfidence, loss aversion, and agency conflicts, are significant drivers of inefficiencies in the hiring and firing process. Transition costs and opportunity costs associated with hiring and firing managers highlight the need for improved decision- making frameworks and governance. Fang, Peress, and Zheng (2014): Media Coverage of Stocks and Mutual Funds’ Trading and Performance Main Idea The study investigates whether media coverage influences the trading and performance of professional investors, focusing on mutual fund managers. Hypotheses: 1. Limited Attention Hypothesis: Media attention serves as a cognitive shortcut for fund managers, leading to more trades in media-covered stocks. 2. Sophistication Hypothesis: Professional managers, with access to superior information, are unaffected by media coverage. 3. Flow-Catering Hypothesis: Fund managers buy media-covered stocks to attract investor inflows. Findings 1. Media Coverage Influences Buys, Not Sells Mutual funds buy high-media-coverage stocks more frequently than low-coverage ones, but no significant effect is observed for sells. o Mechanism: Limited Attention—Fund managers face high search costs for identifying stocks to buy but less so for sells, as they only sell from their current holdings. 2. Negative Relationship Between Propensity to Buy Media-Covered Stocks and Fund Performance Funds that exhibit a strong propensity to buy media-covered stocks underperform by 1.1% to 2.8% annually compared to funds with weak propensities. o Mechanism: Attention-Driven Behavior—Trading decisions are influenced by media attention rather than superior information, leading to poor investment performance. 3. Media Coverage Affects Trades Regardless of Content The number of articles (positive or negative tone) matters more than the content for driving trades. o Mechanism: Salience and Availability Heuristic—Media coverage makes certain stocks more noticeable to managers, increasing trade volumes irrespective of news tone. 4. Managerial Turnover Reduces Propensity to Buy Media-Covered Stocks Funds with managerial changes see a significant drop in their tendency to buy media-covered stocks. o Mechanism: Behavioral Bias Persistence—Limited attention is a characteristic of individual managers rather than institutional policy. 5. Flow-Catering Hypothesis Rejected Funds buying media-covered stocks do not experience higher inflows, ruling out flow-catering as the primary driver. o Mechanism: Focus on Manager Bias—The results emphasize that limited attention among managers, not catering to investors, drives the trading patterns. Behavioral Concepts Underpinning Findings 1. Limited Attention: A cognitive constraint where media coverage lowers search costs but leads to non-optimal trading decisions. 2. Salience Bias: High media coverage makes certain stocks more noticeable, leading to disproportionate attention. 3. Availability Heuristic: Managers rely on easily accessible information, like media coverage, for decision-making. Conclusion Mutual funds exhibit limited attention bias, leading to disproportionate trading in media-covered stocks and subsequent underperformance. Media attention drives trades based on salience rather than rational analysis of stock fundamentals. These findings challenge the assumption of superior sophistication among institutional investors and highlight the persistent cognitive limitations even in professional settings. Pool, Stoffman, and Yonker (2015): Social Interactions and Mutual Fund Portfolios Main Idea The study examines how social interactions influence the investment decisions of mutual fund managers. Fund managers living in close physical proximity (neighbors) tend to have significantly higher similarities in portfolio holdings and trading behaviors compared to those living in the same city but not as close. Social networks transmit value-relevant information, leading to profitable investment decisions, but this effect is most prominent for difficult-to-research stocks with lower informational efficiency. Behavioral Mechanisms 1. Social Influence and Information Sharing:Fund managers within close proximity are more likely to share information through informal social networks. Peer interactions facilitate the dissemination of investment ideas, particularly in settings where managers might meet casually (e.g., neighborhoods, cultural events). 2. Homophily: Similar characteristics (e.g., ethnicity, age, fund management experience) amplify the likelihood and effectiveness of information exchange. 3. Quid Pro Quo Expectations: Sharing information may be motivated by expectations of reciprocal benefits in the future. 4. Localized Decision-Making: Geographic proximity increases the likelihood of shared access to informal and formal information sources, enhancing portfolio overlaps. Proxies for Social Interaction Residential proximity: Managers living within a specific distance threshold are classified as neighbors. Ethnic or cultural similarity, co-location within fund families, or shared educational backgrounds also serve as proxies for social network strength. Findings 1. Portfolio Similarity: Neighboring managers have 12% greater portfolio overlap compared to managers in the same city but not physically proximate. This effect is 5 times stronger than merely being in the same city and about two-thirds as strong as working for the same fund family. o Mechanism: Social interactions foster informal sharing of stock ideas and strategies, especially in local settings. 2. Trade Similarity: Trades made by neighboring managers show a 14% higher overlap compared to managers from the same media market. o Mechanism: Informal networks help in identifying and acting on new investment opportunities simultaneously. 3. Profitability of Shared Information: A long-short strategy based on stocks purchased minus those sold by neighboring managers yields a significant positive abnormal return of 6–7% annually. o Mechanism: Socially transmitted information tends to focus on hard-to-research stocks, which are less informationally efficient. 4. Impact of Manager Characteristics: Common ethnic background or shared fund family amplifies the overlap by as much as 30% relative to unconnected neighbors. o Mechanism: Homophily enhances trust and communication within networks, increasing information exchange efficacy. 5. Robustness to Endogeneity: The overlap in holdings is strongly tied to social proximity and remains significant even when controlling for fund characteristics and media effects. o Mechanism: Social influence is distinct from broader community effects like shared media or localized investor relations. 6. Selective Effects in Hard-to-Research Stocks: Socially influenced trades are more profitable for low-analyst-coverage stocks and stocks with limited public information. o Mechanism: The informal sharing of proprietary insights and idiosyncratic analyses offers a competitive advantage in these less efficient markets. Conclusions Social interactions play a critical role in shaping mutual fund managers' portfolios, with neighboring managers significantly influencing each other’s decisions. Information transmitted through these networks generates measurable value, particularly in under-researched segments of the market. The study highlights the dual role of professional investors as both competitive and cooperative agents within the financial system. Policy Implication: Understanding these dynamics can inform regulations and the design of mechanisms aimed at improving market efficiency and mitigating potential biases introduced by social