Big Data Processing - 2411 - Data Management - PDF
Document Details
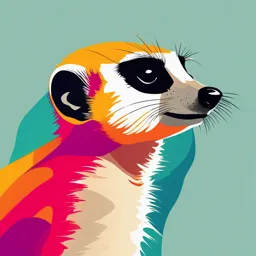
Uploaded by BountifulSatire4895
EAE Business School
null
null
Tags
Summary
This document covers fundamental concepts in data management, including data collection, storage, cleaning, governance, and security. It's an introduction to big data processing suitable for undergraduate-level students.
Full Transcript
Big Data Processing - 2411 01. Data management: basic concepts and fundamentals. eae.es 11 Big Data Processing - 2411 What does DATA MANAGEMENT mean? eae....
Big Data Processing - 2411 01. Data management: basic concepts and fundamentals. eae.es 11 Big Data Processing - 2411 What does DATA MANAGEMENT mean? eae.es 12 Big Data Processing - 2411 Data Management Is the process of collecting, storing, organizing, and maintaining data to ensure it’s accessible, accurate, and ready for analysis. It means understanding how to handle data throughout its lifecycle, from raw data collection to processing and storage, all the way to preparing it for decision-making insights. 13 Big Data Processing - 2411 Key Concepts 1. Data Collection: Gathering data from various sources, like customer databases, sales records, or social media, ensuring it’s relevant and comprehensive for the business problem at hand. 2. Data Storage: Using systems (like databases or data warehouses) to store data securely and systematically. This includes cloud storage solutions that make large-scale data management feasible and scalable. 3. Data Cleaning and Preparation: Ensuring data quality by removing duplicates, fixing errors, and handling missing values so that analyses are accurate and reliable. 14 Big Data Processing - 2411 Key Concepts 4. Data Governance and Security: Establishing policies for data access, privacy, and compliance to protect sensitive information and meet regulatory requirements. 5. Data Integration: Combining data from multiple sources, like CRM systems or marketing platforms, to get a holistic view for analysis. 6. Data Access and Analytics: Making data accessible to the right people at the right time, often through dashboards or analytics tools, to support data-driven decision-making. Understanding these elements helps to effectively use data as a strategic asset, making it easier to derive insights and make informed business decisions. 15 Big Data Processing - 2411 1. Data Collection What It Is: This is the foundational step where you gather data relevant to your business questions or objectives. Data can come from internal sources (like sales records, CRM databases, financial systems) or external sources (like market research, social media, or economic data). Key Steps: Identify Data Sources: Determine where the data comes from, including transaction systems, customer feedback forms, IoT devices, or third-party APIs. In business analytics, data sources should align with your business needs. Define Data Types: Decide if you need structured data (like tables in a database) or unstructured data (like social media posts). Structured data is easier to analyze, while unstructured data often requires pre-processing but can reveal insights like customer sentiment. Select Collection Methods: Common methods include automated data pipelines (for transactional or real-time data), surveys (for customer preferences), and web scraping (for collecting publicly available data). Choose methods based on accuracy, reliability, and ease of integration. Ensure Ethical and Legal Compliance: Be mindful of data privacy laws like GDPR in Europe or CCPA in California. Always obtain data responsibly and, where applicable, anonymize it to protect individual privacy. Why It Matters: Good data collection practices ensure you have reliable data that represents the real-world phenomenon you’re studying. It’s the backbone of any analysis and ultimately impacts the accuracy and quality of your insights. 16 Big Data Processing - 2411 2. Data Storage What It Is: After collecting data, you need a safe, organized space to store it. Storage solutions vary based on data size, type, and access requirements. Key Steps: Choose Storage Solutions: Options include databases (like MySQL or PostgreSQL for relational data), data warehouses (like Snowflake for large, structured data), and data lakes (like Amazon S3 for raw or semi-structured data). Consider Cloud Storage: Cloud solutions (e.g., AWS, Google Cloud, Azure) offer scalable, cost-effective storage and make data accessible from anywhere. They’re also convenient for big data projects that require storage flexibility. Organize Data Structure: Organize your data logically. Data should be easy to locate and access, so apply structures (e.g., database schemas, table names) that facilitate analysis. Ensure Data Backup and Security: Backup mechanisms prevent data loss, and security measures (like encryption and access controls) protect against unauthorized access. Why It Matters: Proper storage ensures data is accessible, secure, and ready for analysis. It allows for efficient processing and retrieval, especially as the volume of data grows. 17 Big Data Processing - 2411 3. Data Cleaning and Preparation What It Is: Also known as “data wrangling” this step ensures that data is in good shape for analysis by fixing errors, standardizing formats, and filling in missing information. Key Steps: Remove Duplicates: Identify and eliminate any duplicate records that could distort analysis. Fix Data Quality Issues: Correct inconsistencies (e.g., formatting differences) and errors (like typos or outliers). Handle Missing Data: Decide how to address gaps. You can remove incomplete rows, fill in missing values (with averages, for example), or use imputation techniques. Transform Data for Analysis: Sometimes, data must be transformed into a suitable format (e.g., converting dates into standard formats or splitting text fields) for further processing. Why It Matters: Clean, high-quality data leads to accurate, reliable analysis, reducing the risk of misleading conclusions. 18 Big Data Processing - 2411 4. Data Governance and Security What It Is: This step involves setting policies and standards to manage data access, privacy, and security, ensuring that only authorized users can access specific data. Key Steps: Define Access Controls: Use role-based access to restrict data based on user roles, keeping sensitive information secure. Set Privacy and Compliance Standards: Data must comply with laws and regulations (e.g., GDPR, HIPAA) and should respect user privacy. Create a Data Usage Policy: Outline how data should be used, shared, and stored within the organization. Policies help prevent misuse and ensure data integrity. Implement Security Protocols: Use encryption, secure passwords, and regular audits to protect data against breaches. Why It Matters: Governance and security ensure data is protected, usable, and compliant with legal standards, safeguarding both the business and its stakeholders. 19 Big Data Processing - 2411 5. Data Integration What It Is: Data integration involves combining data from multiple sources into a cohesive, centralized format, enabling a comprehensive view of the business. Key Steps: Establish Common Data Definitions: Ensure that data fields are consistent across sources. For example, if “customer ID” appears in multiple databases, it should follow the same format. Use ETL Tools: Extract, Transform, Load (ETL) tools like Talend or Informatica facilitate data extraction, cleaning, and loading into a central repository. Ensure Data Synchronization: Data should be updated regularly across systems so that information remains accurate and up-to-date. Resolve Data Conflicts: Handle discrepancies (e.g., different names for the same customer across databases) to ensure that all data aligns. Why It Matters: Integrated data gives a complete picture of business operations, supporting better analytics and decision-making by combining insights from multiple data sources. 20 Big Data Processing - 2411 6. Data Access and Analytics What It Is: The final step is to make data accessible to users (often through dashboards or reports) so they can analyze and derive actionable insights. Key Steps: Implement Business Intelligence (BI) Tools: Tools like Power BI, Tableau, or Looker visualize data for business users, making insights accessible and digestible. Ensure Role-Based Data Access: Only authorized users should access specific data, maintaining privacy and security. Enable Self-Service Analytics: Providing tools and resources for business users to perform their analyses can enhance decision-making across departments. Measure Key Metrics and KPIs: Define relevant metrics, such as customer retention rates or sales growth, to monitor performance and drive decisions. Why It Matters: Proper storage ensures data is accessible, secure, and ready for analysis. It allows for efficient processing and retrieval, especially as the volume of data grows. 21 Big Data Processing - 2411 Data management: basic concepts and fundamentals. DATA LIFECYCLE eae.es 22 Big Data Processing - 2411 Data Life Cycle 23 Big Data Processing - 2411 Example GEOTRACKING COMPANY: 1. Devices in trucks send data to cloud: 2. App To Show Almost Real Time: - Truck/Drivers distance (Kms) - Time in each status (driving, working, waiting & available) - Documents 3. ETL Pipeline to aggregate all collected valuable information 4. Data Storage: Data Warehouse for analytical purposes - Reporting services 24 Big Data Processing - 2411 Deep review of the tool - INFORMATION NEEDED - Distance - Speed - GPS - Brake - Fuel Consumption… - INFORMATION AVAILABLE - Sensors: - Truck: - CANBUS - Trailer: - CANBUS - THERMOGRAPH 25 Big Data Processing - 2411 Data management: basic concepts and fundamentals. DATA STORAGE eae.es 26 Big Data Processing - 2411 Data Storage 1. Introduction to Data Storage 2. Relational Databases: SQL 3. No Relational Databases: NoSQL 4. Data Warehouses 5. Data Lakes 6. Case Study 7. Wrap-Up and Q&A 27 Big Data Processing - 2411 Data Storage 1. Introduction to Data Storage “Data is key to good decisions, but great data unlocks powerful insights that propel impactful actions” Goal: efficient data storage for business insights Key Points: - There are different storage systems: databases, data warehouses, data lakes. - Choosing the right storage for efficient data access and analysis. Discussion: personal experiences with data storage (e.g., spreadsheets, cloud storage). 28 Bases de datos relacionales Big Data Processing - 2411 Se utilizan en: aplicaciones tradicionales, DataWarehouse, ERP, CRM y e-commerce. Productos Data Storage 1. Relational Databases: SQL Almacenan datos cuyas relaciones y esquema están predefinidos, diseñadas para admitir transacciones ACID y conservar la integridad referencial, así como la coherencia de los datos. Database structure: tables, rows, columns, primary and foreign keys. SQL basics: SELECT, INSERT, UPDATE, DELETE. Products: - MySQL, PostgreSQL, MariaDB, Oracle, SQL Server - Amazon Aurora, Amazon RDS, Amazon Assistance: AI that helps to créate a database: Discussion: Simple SQL exercise on a sample database (e.g., employee or transportation data). 29 Big Data Processing - 2411 Incapacidad de manejo de los datos del Método Tradicional Data Mining vs Big Data Relational Database Management Systems (RDBMS) - Terabytes y Petabytes de datos → no puede con ellos - Se necesitan cada vez Máquinas más potentes (más procesadores y memoria), y hacen poco viable su implementación - 80% de los datos recopilados son semi-estructurados o no estructurados, con lo cual no puede analizarlos - No puede lidiar con la velocidad de entrada de datos 30 Big Data Processing - 2411 Distributed Storage Storage and processing of big volumes of data with speed and low cost. DISTRIBUTED COMPUTING 31 Big Data Processing - 2411 ¿Qué es BIG DATA? DISTRIBUTED STORAGE 32 Big Data Processing - 2411 DATA STORAGE SOLUTIONS Most commonly used Big Data Storage Solutions - Hadoop - Elasticsearch - Mongo db - Hbase - Cassandra - Neo4J 33 Big Data Processing - 2411 Data management: basic concepts and fundamentals. DATA CLEANING AND PREPARATION eae.es 34 Big Data Processing - 2411 Data Cleaning and Preparation Overview: Often called "data wrangling," this process focuses on getting your data ready for analysis by correcting errors, harmonizing formats, and dealing with missing values. Key Processes: 1.Eliminate Duplicates: Detect and remove repeated entries that could skew analytical results. 2.Resolve Data Quality Issues: Address inconsistencies such as mismatched formats or errors, including typos and extreme outliers. 3.Address Missing Values: Determine how to handle gaps in your data—options include removing incomplete entries, substituting missing values (e.g., with averages), or applying advanced imputation techniques. 4.Prepare Data for Analysis: Modify and format data as needed, such as standardizing date formats, splitting combined text fields, or reorganizing datasets for easier analysis. Importance: Accurate, well-prepared data ensures dependable insights and minimizes the risk of drawing incorrect conclusions. 35 Big Data Processing - 2411 Data Cleaning and Preparation Beyond Basics: Once the foundational cleaning is done, advanced techniques can further optimize your data for analysis and enhance the quality of your insights. Key Advanced Steps: 1.Feature Engineering: 1. Create new variables or modify existing ones to uncover additional insights. 2. Example: Deriving age from a date of birth or calculating a profitability ratio. 2.Normalization and Scaling: 1. Adjust numerical data to a common scale without distorting relationships. 2. Example: Scaling income data to fall between 0 and 1 for machine learning models. 3.Outlier Treatment: 1. Use statistical methods to identify and handle outliers that could bias results. 2. Approaches: Winsorization, clipping, or applying robust statistical techniques. 4.Data Enrichment: 1. Integrate additional data sources to provide more context. 2. Example: Augmenting sales data with weather information for trend analysis. 5.Automating the Process: 1. Leverage tools or scripts (e.g., Python, R) to automate repetitive cleaning and preparation tasks, improving efficiency and consistency. Why It’s Critical: Advanced preparation ensures your data is not just clean but also tailored to the analytical techniques you plan to use, enabling deeper insights and more effective decision-making. 36 Big Data Processing - 2411 Data Cleaning and Preparation STEPS: 1. WHAT DO WE WANT? WHY WE ARE DOING THIS ANALYSIS? 2. WHAT DATA DO WE HAVE? UNDERSTANDING DATA A. STRUCTURED AND SEMISTRUCTURED DATA B. UNSTRUCTURED DATA A: FIRST STEPS ARE GOING TO BE PERFORMED WITH AN ETL TOOL THAT WE ALL HAVE IN OUR COMPUTERS BUT NEVER NEW WE HAD IT. We are going to dive in a tool that everybody has in its computer: POWERQUERY FOR EXCEL. What we are going to learn is also valid for a business inteligence tool like POWER BI. 37 Big Data Processing - 2411 Data Cleaning and Preparation STEPS: 1. What do we want? Why we are doing this analysis? 2. What data do we have? Understanding data A. Structured and semistructured data B. Unstructured data 3. Beyond the basics: outlier treatment, new variables, enrich data 4. Automate: scripts r/Python 5. Connecting those scripts with Workflows or cronjobs/scheduled tasks 38 Big Data Processing - 2411 Data management: basic concepts and fundamentals. DATA GOVERNANCE AND SECURITY eae.es 39 Big Data Processing - 2411 4. DATA GOVERNANCE AND SECURITY Establishing policies for data access, privacy, and compliance ensures sensitive information is protected and regulatory requirements are consistently met. This foundation supports trustworthy, ethical, and efficient use of data across the organization. ACCESS CONTROL: Defines clear permissions and roles to ensure that only authorized individuals can access specific datasets, minimizing the risk of unauthorized use or breaches. PRIVACY: Implements robust measures to safeguard personal and sensitive information, adhering to frameworks such as GDPR, HIPAA, or CCPA to build trust with stakeholders. COMPLIANCE: Aligns data management practices with legal and industry regulations, ensuring that data usage meets all standards for security, auditability, and accountability. TRANSPARENCY: Creates clear documentation and audit trails for how data is collected, stored, shared, and processed, promoting organizational integrity and readiness for regulatory audits. RISK MANAGEMENT: Proactively identifies vulnerabilities and establishes protocols to prevent, detect, and respond to potential data breaches or misuse. Through strong governance and security practices, organizations can not only protect their data assets but also foster a culture of responsibility, mitigate risks, and enhance the reliability of their data-driven initiatives. 40 Big Data Processing - 2411 Data management: basic concepts and fundamentals. DATA INTEGRATION eae.es 41 Big Data Processing - 2411 5. DATA INTEGRATION Combining data from multiple sources, such as CRM systems, marketing platforms, or financial databases, creates a unified and holistic view of organizational information. This enables seamless analysis, improved collaboration, and more strategic decision-making. CONNECTIVITY: Ensures smooth and reliable access to various data sources, facilitating real-time or scheduled synchronization to keep information up-to-date across systems. TRANSFORMATION: Standardizes, cleanses, and enriches data during integration, ensuring consistency, accuracy, and usability for downstream analytics and reporting. VISIBILITY: Provides stakeholders with a comprehensive, 360-degree view of operations, customers, and performance by breaking down silos between disparate data systems. EFFICIENCY: Automates data workflows and reduces manual effort, accelerating the availability of integrated data for timely insights and action. By enabling the seamless merging of data, integration supports scalable analytics, drives operational excellence, and aligns cross-functional objectives, all while ensuring compliance and governance of data assets. 42 Big Data Processing - 2411 Data management: basic concepts and fundamentals. DATA ACCESS AND ANALYTICS eae.es 43 Big Data Processing - 2411 6. DATA ACCESS AND ANALYTICS Making data accessible to the right people at the right time ensures that stakeholders can act on accurate, timely information, often through dashboards, reports, or analytics tools, fostering a culture of data- driven decision-making across the organization. NOTIFICATIONS: Serve as proactive alerts, ensuring that users are immediately informed of significant changes, anomalies, or opportunities within the data, enabling swift action and reducing response times. REPORTS: Provide structured, in-depth insights with formulas, graphs, and data visualizations, summarizing historical trends and key performance metrics to inform strategy and operations. DASHBOARD: Interactive dashboards consolidate and visualize data in real-time, making complex information more accessible and actionable. By bringing businesses closer to their data, dashboards empower users to monitor performance, identify patterns, and make informed decisions with agility. This approach to data access and analytics aligns with broader data management goals, including ensuring data integrity, compliance, and scalability to support evolving business needs. 44 Big Data Processing - 2410 02. Introduction to massive data processing: infrastructures, types, development and applications eae.es 45 Big Data Processing - 2410 1. INTRODUCTION TO BIG DATA INFRASTRUCTURES 2. DISTRIBUTED COMPUTING 3. HADOOP ECOSYSTEM 4. DATA PROCESSING: BATCH & STREAM PROCCESSING 5. CLOUD COMPUTING FOR BIG DATA 46 Big Data Processing - 2410 1. INTRODUCTION TO BIG DATA INFRASTRUCTURES HDFS (Hadoop Distributed File System) Definition: HDFS is a distributed file system. It combines the storage capacity of multiple distributed machines into a single, very large system. It allows creating directories and storing data in files in a distributed manner across multiple machines. Key Features of HDFS: Distributed: Data is stored across multiple machines, making it scalable and fault-tolerant. Horizontally Scalable: New machines can be added to the system to increase storage and processing capacity. Cost-Effective: Allows starting with simple, low-cost machines and scaling up as needed. Fault Tolerant: Designed to handle hardware failures without data loss or downtime. High Throughput: Optimized for large-scale data processing, ensuring efficient performance. 47 Big Data Processing - 2410 1. INTRODUCTION TO BIG DATA INFRASTRUCTURES Explosion in the amount of data generated by: Applications: Social networks, Web applications, Mobile apps, IoT devices, Log files, etc. It is necessary to explore methods that enable: Collect and Produce: Apps: Web, Mobile, IoT 1.Transmitting and distributing data between distributed applications (Message brokers: RabbitMQ, ActiveMQ, Kafka) Analyze and Transmit and Extract Distribute: 2.Storing and securing data in a distributed manner Knowledge: Data Mining, Brokers like RabbitMQ, (Technologies: Hadoop HDFS, NoSQL databases such as Cassandra, MongoDB, HBase, Machine Learning Big ActiveMQ, Kafka Elasticsearch, etc.) Data 3.Processing and analyzing data in a distributed way to extract knowledge for decision-making 1. Big Data Processing: Batch Processing (e.g., MapReduce, Spark) and Stream Process: Batch Store: HDFS, Processing (e.g., Kafka Stream, Flink, Storm, Samza) and Stream Processing NoSQL, SQL 2. Data Mining and Machine Learning: TensorFlow, DeepLearning4J, Weka, etc., for knowledge extraction. 4.Analyzing and visualizing decision-making indicators (Using Big Data visualization tools) 48 Big Data Processing - 2410 1. INTRODUCTION TO BIG DATA INFRASTRUCTURES Big Data: The 3 V's 1.Volume 1. The quantity of data (Measured in Petabytes - PO) 2.Variety 1. Different formats of data (Structured: 20%, Unstructured: 80%) 1. Examples: Text, CSV, XML, JSON, Binary files, Databases, etc. 3.Velocity 1. The frequency at which data is generated 1. Example: Twitter 1. Every second, approximately 5,900 tweets are sent on the Twitter microblogging platform. 2. This represents 504 million tweets per day or 184 billion tweets per year. 3. This mass of information contributes to the stream of "Big Data" published by humanity daily on the internet. 49 Big Data Processing - 2410 1. INTRODUCTION TO BIG DATA INFRASTRUCTURES Big Data: 5 V's 1.Volume 1. The amount of data. Volumen 2.Variety 1. The diversity of data formats and types. 3.Velocity Value Variety 1. The speed at which data is generated and arrives. Big 4.Veracity Data 1. Reliability and credibility of the collected data (from reliable sources). 5.Value 1. The profit and knowledge that can be extracted from the data. Veracity Velocity 2. Transforming data into actionable value. 50 Big Data Processing - 2410 1. INTRODUCTION TO BIG DATA INFRASTRUCTURES EXAMPLE OF PROBLEM: TRANSPORT COMPANY 1. Volume 4. Veracity The company generates a vast amount of data from its operations: Ensuring data accuracy and reliability is critical: GPS tracking data from thousands of trucks. Verifying sensor data for accurate temperature readings in cold- Fuel consumption records. chain logistics. Delivery schedules for hundreds of customers daily. Ensuring customer delivery addresses are correct to avoid delays. Sensor data (temperature, humidity) for perishable goods. Electronic Proof of Delivery (ePOD) and invoices. Filtering out inaccurate GPS data caused by signal loss. 2. Variety Using reliable data sources (e.g., government traffic databases) to The data comes in diverse formats: avoid false routing decisions. Structured Data: Customer orders, delivery schedules, financial transactions. 5. Value Semi-Structured Data: GPS logs, IoT sensor readings, and email Extracting actionable insights from the data to drive profitability: communications. Unstructured Data: Customer feedback, driver reports, and images of Optimization: Use Big Data to reduce fuel costs by optimizing damaged goods. routes and eliminating empty miles. 3. Velocity Customer Satisfaction: Predict delivery times more accurately, The speed at which the data is generated and needs processing is crucial: improving customer trust and loyalty. Real-time GPS updates (every second) to track trucks' locations. Predictive Maintenance: Use IoT data from trucks to predict Continuous temperature monitoring for refrigerated goods. and prevent breakdowns, minimizing downtime. Real-time alerts for delivery delays, accidents, or maintenance issues. Strategic Decisions: Analyze demand trends to reposition Daily route optimization based on traffic and weather data. trucks more effectively, reducing idle time. 51 Big Data Processing - 2410 DISTRIBUTED COMPUTING Big Data in Everyday Life: A Chef Processing Orders This analogy explains Big Data processing using the example of a chef handling orders in a restaurant: 1.Orders : 1. Just like a restaurant receives multiple orders from customers, Big Data systems collect large amounts of incoming data from various sources. Job 2. Example: Online orders, reservation data, or customer preferences. 2.Job: 1. Each order represents a "job" that needs to be completed. 2. 3. The chef (Big Data system) processes these jobs based on priorities, resources, and capacity. Example: The chef prioritizes meals based on preparation time and delivery requirements. orders Delivery 3.Processing: 1. The chef processes the orders by cooking the meals, akin to Big Data systems processing raw data into actionable insights. 2. Example: In Big Data, this might involve analyzing customer trends or optimizing operations. 4.Storage: 1. Just like the chef uses a refrigerator to store ingredients for future use, Big Data systems store processed or raw data for later analysis. 2. Example: Data stored in databases (HDFS, NoSQL) or cold storage for long-term archiving. 5.Delivery: 1. After processing, the chef delivers the meals to customers. Similarly, Big Data delivers results (insights) to end-users or decision-makers. 2. Examples: Reports, dashboards, or notifications send to stakeholders storage 52 Big Data Processing - 2410 Cooks Sauce Cooks Meat DISTRIBUTED COMPUTING Assembles Shuffling and Reducing Output Input Data Mapping Phase HDFS Sorting Phase Distributed Food Assigning Tasks Prepare for next Distributed Storage Combining results Shelf phase storage 53 Big Data Processing - 2410 3. BIG DATA ECOSYSTEM – APACHE HADOOP Hadoop is a free and open-source framework written in Java, designed to facilitate the creation of massively distributed applications across thousands of nodes. At the storage level: Distributed storage of data (petabytes of data) with HDFS (Hadoop Distributed File System). At the processing level: Data processing with MapReduce. Supports fundamental features: High availability Scalability Fault tolerance Recovery after failure Security (HPC: High-Performance Computing) The core Hadoop framework is composed of the following modules: Hadoop Distributed File System (HDFS): A system for distributed file storage. Hadoop YARN (Yet Another Resource Negotiator): A system for cluster resource management. Hadoop MapReduce: Distributed data processing. 54 Big Data Processing - 2410 3. HADOOP ECOSYSTEM: DATA MANAGEMENT DATA ACCESS DATA PROCESSING DATA STORAGE DISTRIBUTED PROGRAMMING DISTRIBUTED LEARNING/SCHEDULING 55 Big Data Processing - 2410 3. HADOOP ECOSYSTEM: HDFS DATA LEARNI DISTRIBUTED MANA Hadoop MapReduce PROGRAMMING NG GEME Apache Hbase NT Apache Spark Apache Hive Oozie Mahout Sqoop DATA DATA PROCESSING Storm STORAGE DATA Flume MANA DATA GEME ACCESS NT DATA STORAGE 56 Big Data Processing - 2410 Enumeración de las más habituales: - Ecosistema Hadoop 1-HDFS 2-Hadoop MapReduce 3-Apache Hbase 4-Apache Spark 5-Apache Hive 6-Oozie 7-Mahout 8-Sqoop 9-Storm 10-Flume 57 Big Data Processing - 2410 4. DATA PROCESSING – BATCH AND STREAM Batch Processing (Traitement par lots): Definition: Batch processing refers to the processing of blocks of already stored data over a specific period. Example: Processing all the transactions performed by a financial company over the span of a week. Characteristics: The data contains millions of records for each day. These records can be stored as: Text files (e.g., CSV format). Records stored in systems like HDFS, SQL databases, or NoSQL databases. Framework Examples: MapReduce (Hadoop): A framework for batch data processing. Spark: Another framework designed to process large batches of data efficiently. 58 Big Data Processing - 2410 4. DATA PROCESSING – BATCH AND STREAM Stream Processing (Real-Time Processing): Unlike batch processing, where data has a defined start and end and is processed once all the data is available, stream processing is designed for continuously flowing data in real time, arriving endlessly for days, months, years, or indefinitely. Stream processing allows: Real-time data processing: Data can be handled as soon as it is generated. Instant analysis results: Stream processing feeds data directly into analytics tools, enabling immediate insights. 59 Big Data Processing - 2410 4. DATA PROCESSING – BATCH AND STREAM Stream Processing Approaches 1. Native Streaming (Real-Time Processing): Definition: o Each incoming record is processed immediately as it arrives, without waiting for other data. Examples: o Technologies: Storm, Flink, Kafka Streams, Samza. 2. Micro-Batch Processing: Definition: o Incoming records over short intervals (e.g., seconds) are grouped into small batches and processed together with minimal delay. Examples: o Technologies: Spark Streaming, Storm-Trident. 60 Big Data Processing - 2410 4. DATA PROCESSING – STREAM PROCESSING – STRONG AND WEEK POINTS BTW NATIVE STREAMING AND MICRO-BATCH PROCESSING Native Streaming: Micro-Batch Processing: Strong Points: Strong Points: Real-Time Results: Immediate processing makes it ideal for Efficiency: Processing small batches reduces overhead applications requiring instant insights (e.g., fraud detection, live monitoring). compared to handling individual events. Low Latency: Data is processed as soon as it arrives, ensuring Simpler Model: Easier to implement and manage, as it minimal delays. follows a more structured batch-like approach. Granular Processing: Handles each event independently, Integration: Compatible with existing batch processing which is useful for real-time applications. systems (e.g., Hadoop) for hybrid workflows. Weak Points: Weak Points: Complexity: Requires more sophisticated programming models Latency: Slight delays are introduced due to batch for event-by-event processing. intervals, making it less suitable for strict real-time Resource Intensive: Constant processing can strain system requirements. resources. Granularity: Lacks the fine-grained processing of native Fault Tolerance: Ensuring data consistency in case of failures streaming, as events are grouped into batches. is more challenging. 61 Big Data Processing - 2410 4. DATA PROCESSING – STREAM PROCESSING – CHOOSING THE RIGHT APPROACH Choosing the Right Approach Native Streaming: Best for applications that demand immediate action, such as financial fraud detection or live stock market analysis. Micro-Batch Processing: Suitable for near-real-time analytics and applications where small delays are acceptable, such as dashboard updates or periodic reports. 62 Big Data Processing - 2410 4. BIG DATA ECOSYSTEM …KEEPS EXPANDING The Big Data ecosystem has expanded with many tools, such as: Stream Processing: Apache Storm: A framework for computation and distributed processing of data streams (Stream Processing). Apache Flink: A framework for computation and distributed processing of data streams (Stream Processing). Apache Spark: A framework for distributed Big Data processing, offering an alternative to MapReduce and supporting Stream Processing. Apache Kafka Streams: A real-time streaming platform for distributed applications and message systems. Apache Zookeeper: A system for managing the configuration of distributed systems to ensure coordination between nodes. NoSQL Databases (SQBD NoSQL): Apache HBase: A distributed NoSQL database for structured storage in large tables. → NEEDS HDFS!! MongoDB: A NoSQL database (Not Only SQL) where data is stored in a distributed manner across nodes in JSON document format. Cassandra: A NoSQL database (Not Only SQL) where data is stored in a distributed manner across nodes in JSON document format. Elasticsearch: A distributed and multi-entity search engine accessible through a REST interface. Hazelcast: A distributed in-memory cache and NoSQL system, also functioning as a message application system. 63 Big Data Processing - 2410 4. BIG DATA ECOSYSTEM …KEEPS EXPANDING The Big Data ecosystem has expanded with many tools, such as: Apache Pig: A high-level platform for creating MapReduce applications using the Pig Latin language (which resembles SQL) instead of writing Java code. Apache Hive: A data warehouse infrastructure for analysis and querying using a language similar to SQL. Apache Phoenix: A relational database engine built on top of HBase. Apache Impala: A SQL query engine from Cloudera for systems based on HDFS and HBase. Apache Flume: A system for collecting and analyzing log files. Apache Sqoop: Command-line tools for transferring data between relational databases (RDBMS) and Hadoop. Apache Oozie: A workflow scheduling tool for managing Hadoop data processing workflows. 64 Big Data Processing - 2410 5. INTRODUCTION FOR CLOUD COMPUTING IN BIG DATA Cloud computing provides on-demand access to scalable computing resources, including storage, compute power, and applications. 1. Why the Cloud for Big Data? Scalability, Cost Effectiveness, Flexibility, Global Access 2. Key Cloud Service Models for Big Data: Infrastructure as a Service (IaaS): Platform as a Service (PaaS): Software as a Service (SaaS): 3. Benefits of Cloud Computing for Big Data Elastic Scalability, Cost Optimization, Built-In Tools, Reduced Time to Market: Disaster Recovery: 4. Cloud Platforms for Big Data AWS, Azure, GCP, IBM, Oracle, Cloudera 5. Tools and Frameworks for Big Data in the Cloud Data storage, Data Processing and Data Integration 6. Real-World Use Cases Social Media Analysis, IoT Data Processing, E-commerce Analytics 65 Big Data Processing - 2410 5. INTRODUCTION FOR CLOUD COMPUTING IN BIG DATA 7. Challenges and Considerations Data Security and Privacy: Highlight concerns about storing sensitive data in the cloud. Discuss encryption and compliance solutions offered by providers. Cost Management: warn about potencial overuse of resources without proper cost monitoring tools. Data Transfer: discuss potential latency and costs associated with moving large datasets to/from the cloud 8. Future Trends: Infrastructure as a Service (IaaS): Serverless Computing: serverless architectures (e.g., AWS Lambda, Azure Functions) simplify Big Data workloads by abstracting infrastructure. AI and Big Data Integration: Cloud-based AI/ML tools like Google AI Platform and AWS SageMaker. Multi-Cloud and Hybrid Models: Using multiple cloud providers or a combination of on-premises and cloud for flexibility. 66 Big Data Processing - 2410 Opciones de Servicios en Entornos Cloud 67 Big Data Processing - 2410 03. Application deployment. Development of scalable applications eae.es 68 Big Data Processing - 2410 How to deploy this applications: 1. Manual installations: install Hadoop and Spark (Link1) y multinode 2. Could computing distributions: services used where installation and scalability is simpler and easily scalable. 1. Cloudera 2. Hortonworks 3. Google 4. AWS 5. MICROSOFT 6. IBM Best things are the scallability. You can create them for a particular use case and when you want deploy it and increase the size as you need. When you stop needing it, you can erase it and you Will not be charged for any of it. 69 Big Data Processing - 2410 How to deploy this applications: 1. What do I need to know to install manually? a. basic cmd/bash commands b. download the software needed - Desired Software - Dependencies needed: for example Java c. setup properly the machine I. Environmental variables II. Networking: ip addresses III. comunication protocols: ssh (keys for security encriptions) d. install and verify prerequisits (verify versions with commands like: java --version, python3 -- version, pip…, system utilities: curl, wget, unzip …) e. Install the Big Data Tool a. Follow the installation guide for the software a. extract downloaded binaries b. move to a suitable directory c. Run anysetup scripts provided b. Create system services for tools that run continuously (Linux.service in 70 etc/systmemd/system, Windows use task Scheduler Big Data Processing - 2410 How to deploy this applications: 1. What do I need to know to install manually? f. Configure the Tool i. Modify configuration files as required: core-site.xml, hdfs-site.xml, and mapred-site.xml for Hadoop. spark-defaults.conf, spark-env.sh for Spark. ii. Set appropriate resource limits: Memory allocation Number of threads iii. Enable logging: Specify log levels (INFO, DEBUG, ERROR) Configure log rotation g. Validate the Installation i. Run basic commands to test functionality: Hadoop: hadoop version, hdfs dfs -ls / Spark: spark-submit --versión ii. Verify services are running: Check processes with ps or tasklist. Use web UIs (e.g., Hadoop ResourceManager, Spark Master). 71 Big Data Processing - 2410 How to deploy this applications: 1. What do I need to know to install manually? h. Optimize for Performance i. Adjust system limits (ulimit, kernel parameters). ii. Optimize JVM settings for Java-based tools: Example: -Xms2g -Xmx4g iii. Enable caching and compression for large data handling i. Set Up Monitoring i. Install monitoring tools: JMX Exporter, Prometheus, Grafana for metrics. Log analyzers like Elasticsearch and Kibana. ii. Monitor system resources: Disk usage, CPU, memory, network throughput. j. Document the Process i. Keep a log of installation steps and configurations for future reference. ii. Create a backup of configuration files. 72 Big Data Processing - 2410 Deploy Spark Option 1: Virtual machine (in a Linux OS) - Steps: - Install VM Virtualbox and Ubuntu - Follow this steps in this video to install spark: link Option 2: - using docker: pyspark jupyterlab -- run it with a command: docker run -p 8888:8888 -p 4040:4040 -e JUPYTER_ENABLE_LAB=yes -v /c/Users/marti/OneDrive/Documentos/Notebooks:/home/jovyan/work jupyter/pyspark-notebook 73 Big Data Processing - 2410 Deploy AirFlow Option 1: Virtual machine (in a Linux OS) - Steps: - Install VM Virtualbox and Ubuntu - Follow this steps in this video to install spark: link Option 2: - using docker Option 3: - docker compose 74 Big Data Processing - 2410 Applications Deployment: Development of Scalable Applications for Big Data Processing eae.es 75 Big Data Processing - 2410 Development of Scalable Applications for Big Data Processing Designing for High-Volume, High-Velocity & High Variety Data Big Data Applications must handle high velocity (streaming), massive volumes, and diverse data. Example: Netflix processes petabytes daily for recommendations and analytics. Why Scalability Matters? Real-time analytics, efficient storage, and seamless scaling. Why is critical in Big Data? - Manage exponential data growth - Enable real-time insights for streaming data - Quickly adapt to new data sources and frameworks - Ensure high availability and fault tolerance 76 Big Data Processing - 2410 Development of Scalable Applications for Big Data Processing: SCALING: VERTICAL vs. HORIZONTAL UPGRADING RAM/CPU/STORAGE ADDING MORE NODES 77 Big Data Processing - 2410 Development of Scalable Applications for Big Data Processing: CHALLENGUES: - Managing distributed systems. - Sharding and partitioning data across nodes. - Ensuring fault tolerance. - Balancing compute vs. storage requirements. - Handling varying workloads effectively. BEST PRACTICES FOR BIG DATA SCALABILITY: - Use distributed frameworks like Apache Spark, Kafka, or Flink. - Leverage cloud-based storage (e.g., Amazon S3, Google BigQuery). - Optimize data pipelines for streaming frameworks. - Design for modularity and scalability in architecture. 78 Big Data Processing - 2410 Development of Scalable Applications for Big Data Processing: TOOLS & TECHNICS Tools and Techniques for Big Data Scalability 1. Distributed Storage HDFS (Hadoop Distributed File System): A reliable and scalable storage system for managing large datasets. Amazon S3: Cloud-based object storage with high scalability and durability. Google Cloud Storage: A highly available, secure, and scalable storage option for big data processing. 2. Data Processing Frameworks Apache Hadoop: Batch processing for large-scale data analysis. Apache Spark: Unified analytics engine for large-scale data processing with in-memory computation. Apache Flink: Stream and batch processing framework optimized for real-time analytics. 3. Streaming Tools Apache Kafka: Distributed event streaming platform for real-time data pipelines. AWS Kinesis: Scalable and fully managed platform for streaming data processing. Apache Pulsar: Multi-tenant, high-performance message broker for streaming and queuing. 79 Big Data Processing - 2410 Development of Scalable Applications for Big Data Processing: TOOLS & TECHNICS: 4. Workflow Orchestration and Automation Apache Airflow: A platform to programmatically author, schedule, and monitor workflows. Apache NiFi: Data flow automation for system integration, transformation, and routing. Luigi: Python-based workflow management system designed for batch processes. Prefect: Modern workflow orchestration platform with an emphasis on simplicity and reliability. 5. Monitoring and Optimization Prometheus: Open-source monitoring and alerting toolkit for system metrics. Grafana: Visualization and analytics software for monitoring dashboards. Datadog: Cloud-based monitoring and performance tracking for infrastructure and apps. Elastic Stack (ELK): Log management and analytics platform for monitoring and troubleshooting. 80 Big Data Processing - 2410 Development of Scalable Applications for Big Data Processing: TOOLS & TECHNICS 6. ETL and Data Integration Talend: Data integration platform for transforming, cleansing, and loading data. Informatica: Enterprise-grade data integration and management. dbt (Data Build Tool): Transform data inside the warehouse with SQL-based workflows. 7. Cloud and Resource Management Kubernetes: Container orchestration for managing scalable deployments. Terraform: Infrastructure as code (IaC) tool for provisioning scalable infrastructure. Cloud Dataflow: Google’s managed service for stream and batch processing pipelines 81 Big Data Processing - 2410 Development of Scalable Applications for Big Data Processing: REAL CASE STUDIES: - Netflix: Real-time pipelines with Spark and Kafka for recommendations. - Uber: Scalable architecture for ride-matching and analytics. - Twitter: Handling millions of tweets/second with distributed systems. 82 Big Data Processing - 2410 Development of Scalable Applications for Big Data Processing: STEPS TO BUILD BIG DATA APPLICATIONS: 1. Design modular data pipelines (ingestion, processing, storage). 2. Test with real-world data to identify bottlenecks. 3. Build fault-tolerant systems with recovery mechanisms. 4. Implement data partitioning for distributed workloads. CONCLUSION - Scalability ensures big data systems grow with business needs. - Proper design supports real-time analytics and batch processing. 83 Big Data Processing - 2410 Development of Scalable Applications for Big Data Processing: QUESTIONS OR THOUGHTS? - Discuss ideas on scalable big data applications. - Explore strategies for your projects. 84 Big Data Processing - 2410 4. Types of Big Data processing to model business logic eae.es 88 Big Data Processing - 2410 Real use case: Phase I: You are data analysts working for a transportation 1. Analyze the downloaded data and determine which files company, assisting area managers with reporting tasks. contain the information needed to meet the fleet manager’s The fleet manager reaches out to you, explaining that requirements. they need to annually submit information on the total 2. Create a single CSV file combining all relevant files (using kilometers traveled by each truck to a government Python) and save it in your sandbox at the following location: agency. ETL-Python/data/Output/typefilename_combined.csv The company has vehicles registered under two separate entities: i. Each team will work in 2 pairs: 2 people uses python and 2 PowerQuery (Excel). Company A and Company B. Unfortunately, the telemetry data for the vehicles was Analyze the combined content and provide a summary of the lost during a database migration. You come up with the idea of retrieving this information from the daily information. CSV reports that are emailed. You manage to extract these files from the emails and Phase II: save them in the directory: Design the Data Architecture to ensure the company ETL-Python/data collects the information needed to their use Teams of 4. 89 Big Data Processing - 2410 Real use case solution: Find the key data points: As you were able to see, we need at least this too informations - Distance - Odometer Why? Sometimes there are jumps in the odometer, and needs to be tackeled with the addition of Distance. The Odometer max - min from the year has to be very close to the Sum of all distances registered. Design the Data Pipeline to ensure you have always the most suitable. Choose the appropriate tools to guarantee a good job. 1. Collect all data 2. Process it 3. Load it into a data base or a semistructured document 4. Present it 90 Big Data Processing - 2410 05. Models, architectures, tools, and high-level languages for massive data processing. eae.es 91 Big Data Processing - 2410 Cluster Computing Types of Clusters High availability Minimize shutdown time and provide uninterrupted service when a node fails. Load Balancing They are designed to distribute the workload among nodes, ensuring that tasks are shared and executed as soon as possible. This way, if any node fails, the workload is balanced across other nodes to complete the task 92 Big Data Processing - 2410 Cluster Computing Cluster Structure Symmetric Symmetric clusters are those in which each node functions as an independent computer, capable of running applications. They are part of a subnet, and additional computers can be added without issues. Asymmetric Asymmetric clusters have a head node that connects to all the worker nodes. In this configuration, the system depends on the head node, which acts as a gateway to the data/worker nodes. 93 Big Data Processing - 2410 Distribution Models Replication A copy of the same dataset is stored across different nodes. Sharding The process of partitioning and distributing data across different nodes. Two partitions of the same data are never placed on the same node. Replication & Sharding Both can be used independently or together. 94 Big Data Processing - 2410 Distribution Models Replication Models Master-Slave Model In this model, a master node controls the replication process, sending copies of the data to slave nodes and keeping track of where each replica is stored. If the master node fails, the entire system may become inoperable. To mitigate this risk, a secondary master node (failover master) should be implemented to take over in case of failure, ensuring continuous operation. This model is commonly used for read-heavy workloads, where the master handles updates, and slaves serve read requests. Peer-to-Peer Model In this model, there is no central master node. Instead, all nodes are equal, and replication is typically used for read operations rather than writes. This approach enhances redundancy and load balancing, making it ideal for distributed systems where high availability is a priority. However, without a central authority, consistency management can be more complex, requiring conflict resolution mechanisms. Key Considerations Performance & Scalability: The Master-Slave model is efficient for structured workloads but introduces a single point of failure, whereas Peer-to-Peer systems provide more resilience at the cost of complexity. Use Cases: Master-Slave is often used in traditional databases and enterprise applications, while Peer-to-Peer is more common in decentralized networks, content distribution systems, and blockchain-based architectures. 95 Big Data Processing - 2410 Distributed systems Examples: Storage: Explanation of HDFS and how Distributed Systems work: link Processing: ETL Pipeline: AirFlow, Spark [EMR (cloud computing)] + Load in Snowflake [Data Warehouse solution (cloud storage)] link 96 Big Data Processing - 2410 When Distributed Systems May Not Be the Right Solution Transactional Workloads with Random Data: If the task involves processing jobs in a transactional manner with unpredictable data access patterns, distributed systems may introduce unnecessary complexity and overhead. Non-Parallelizable Workloads: When tasks cannot be broken down and executed in parallel, distributing them across multiple nodes does not provide any advantage and may even degrade performance. Low-Latency Data Access Requirements: If the system demands extremely fast access to data with minimal delays, a centralized architecture or in-memory processing might be a better choice than a distributed approach. Handling a Large Number of Small Files: Distributed systems are optimized for large-scale data processing, but managing a high volume of small files can introduce inefficiencies due to metadata overhead and excessive disk I/O operations. Intensive Computation with Minimal Data: When workloads involve heavy computations but operate on small datasets, the cost of data transfer and synchronization across nodes can outweigh the benefits of distribution, making a local or specialized computing solution more efficient. 97 Big Data Processing - 2410 Use Case: SmartHome & Buildings - IOT world Intro to SmartHome & Building system. What data we can get What we do with it? Let’s think!! 98 Big Data Processing - 2410 High level programming languages Python SQL (Structured Query Language) ‒ Widely used due to its ease of use and extensive ‒ Essential for querying large datasets in distributed ecosystem of libraries for data processing (e.g., Pandas, databases like Hive, Presto, and Google BigQuery. Dask, PySpark). ‒ Used for structured data transformations and aggregations in ‒ Supports machine learning and data analytics big data pipelines. frameworks like TensorFlow and Scikit-learn. Julia Scala ‒ High-performance computing language optimized for ‒ Designed for functional and object-oriented programming, numerical analysis. making it ideal for distributed data processing. ‒ Growing adoption in big data analytics and machine learning. ‒ Native language for Apache Spark, ensuring high Go (Golang) performance and efficient parallelism. ‒ Designed for high concurrency and performance, making it Java useful in distributed computing. ‒ Strongly typed and widely adopted for enterprise-level ‒ Used in large-scale data processing systems like InfluxDB applications. and CockroachDB. ‒ Used in Hadoop and Apache Beam, providing scalability Rust and robustness. ‒ Memory-safe and optimized for performance, making it R suitable for large-scale real-time data processing. ‒ Preferred in statistical computing and data visualization. ‒ Used in distributed data platforms like Vector and Timely ‒ Integrates well with SparkR for large-scale analytics. Dataflow. 99 Big Data Processing - 2410 High level programming languages PERSONAL RECOMMENDATION: Start with Python and SQL Where to Begin 1. Learn Python and SQL: Python is versatile, easy to learn, and widely used in data processing. SQL is essential for querying and managing large datasets efficiently. 2. Work on Practical Projects: Apply what you learn to real-world projects that interest you. Start with simple data analysis, ETL pipelines, or automation scripts. 3. Build a Structured Programming Mindset: Understanding how to structure your code and solve problems is more important than just learning a language. A well-organized thought process will help you adapt to new tools and technologies. 4. Expand Your Knowledge When Needed If a project requires another language or tool, you’ll be prepared to learn it quickly.AI-powered development tools will make learning and implementation even easier in the future. Final Thought: The Idea Matters Most The most critical aspect is having a clear idea of what you want to achieve. Technology is just a tool—what really matters is how you use it to solve problems and create value. GOOD LUCK AND ENJOY YOUR RIDE! 100