Bayes Slides PDF
Document Details
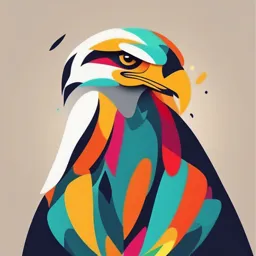
Uploaded by GleefulConcertina3965
Tags
Summary
These slides present Bayesianism, detailing degrees of belief as probabilities, probability calculus rules, conditional probability, and Bayes' rule. It uses examples to illustrate the application of Bayes' Theorem.
Full Transcript
Bayesianism Bayesianism – three key ideas Belief comes in degrees Degrees of belief can be modelled as probabilities/ conform to the basic principles of probability calculus Learning takes place by updating probabilities (degrees of belief) according to Bayes’ rule Degrees of belief as...
Bayesianism Bayesianism – three key ideas Belief comes in degrees Degrees of belief can be modelled as probabilities/ conform to the basic principles of probability calculus Learning takes place by updating probabilities (degrees of belief) according to Bayes’ rule Degrees of belief as probabilities Belief is often viewed as a two-valued states: true/false For Bayesians, belief comes in degrees Probability assignments are more precise that the language of “not”, “very”, “fairly”, etc. Obey basic rules of probability calculus Rules of probability calculus Basic rule 1: Probabilities are numbers between 0 and 1 Basic rule 2: All impossible sentences have probability 0 Basic rule 3: All necessary truths (such as “2 + 2 = 4”) have probability 1 Basic rule 4: If sentences P and Q are logically equivalent, then p(P) = p(Q) Rules of probability calculus (cont.) The negation rule If sentence S has probability p, then its negation not-S (S) has probability 1 – p The disjunction rule (restricted) If sentences R and S are mutually exclusive, then the probability of R or S is p(R) + p(S) The conjunction rule (restricted) If sentences R and S are independent of each other (i.e. the presence of one does not make the other more likely), then the probability of R and S is p(R) p(S) Conditional Probability p(A/B) The probability of A, conditional upon B, is the probability that A holds, relative to the assumption that B holds. E.g. ‘A’ stands for ‘There is thunder’ and ‘B’ for ‘It is raining’, then p(A/B) is the probability that there is thunder, if we assume that it is raining. Bayes’s rule You have some evidence for a hypothesis. Question: How strong is the evidence? -> Given that the evidence holds, how likely is it that the hypothesis is true? -> p(Hypothesis/evidence) or p(H/E) Hypothesis (H) : I have extremely rare disease Evidence (E): Positive test result Likelihood: P(E/H) 99% (very high) Prior: P(H) 1/10000 =.00001 (very low) P (H/E) = [P (E/H) * P(H)] / P(E) Even with a reliable test, there are many false positives.