Artificial Intelligence: A Modern Approach (4th Edition) - PDF
Document Details
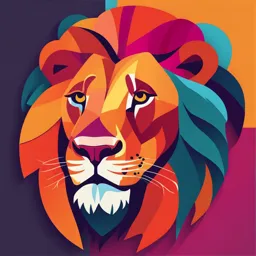
Uploaded by EasiestMimosa
Georgia Institute of Technology
Peter Norvig and Stuart Russell
Tags
Summary
This textbook, "Artificial Intelligence: A Modern Approach", details problem-solving techniques within artificial intelligence. It focuses on search algorithms, exploring how agents solve problems by considering sequences of actions to reach goals. The book delves into different types of problems and solutions, suitable for undergraduate study.
Full Transcript
CHAPTER 3 SOLVING PROBLEMS BY SEARCHING In which we see how an agent can look ahead to find a sequence of actions that will even- tually achieve its goal. When the correct action to take is not immediately obvious, an agent may need to to plan ahead: to conside...
CHAPTER 3 SOLVING PROBLEMS BY SEARCHING In which we see how an agent can look ahead to find a sequence of actions that will even- tually achieve its goal. When the correct action to take is not immediately obvious, an agent may need to to plan ahead: to consider a sequence of actions that form a path to a goal state. Such an agent is Problem-solving called a problem-solving agent, and the computational process it undertakes is called search. agent Problem-solving agents use atomic representations, as described in Section 2.4.7—that Search is, states of the world are considered as wholes, with no internal structure visible to the problem-solving algorithms. Agents that use factored or structured representations of states are called planning agents and are discussed in Chapters 7 and 11. We will cover several search algorithms. In this chapter, we consider only the simplest environments: episodic, single agent, fully observable, deterministic, static, discrete, and known. We distinguish between informed algorithms, in which the agent can estimate how far it is from the goal, and uninformed algorithms, where no such estimate is available. Chapter 4 relaxes the constraints on environments, and Chapter 5 considers multiple agents. This chapter uses the concepts of asymptotic complexity (that is, O(n) notation). Readers unfamiliar with these concepts should consult Appendix A. 3.1 Problem-Solving Agents Imagine an agent enjoying a touring vacation in Romania. The agent wants to take in the sights, improve its Romanian, enjoy the nightlife, avoid hangovers, and so on. The decision problem is a complex one. Now, suppose the agent is currently in the city of Arad and has a nonrefundable ticket to fly out of Bucharest the following day. The agent observes street signs and sees that there are three roads leading out of Arad: one toward Sibiu, one to Timisoara, and one to Zerind. None of these are the goal, so unless the agent is familiar with the geography of Romania, it will not know which road to follow.1 If the agent has no additional information—that is, if the environment is unknown—then the agent can do no better than to execute one of the actions at random. This sad situation is discussed in Chapter 4. In this chapter, we will assume our agents always have access to information about the world, such as the map in Figure 3.1. With that information, the agent can follow this four-phase problem-solving process: Goal formulation: The agent adopts the goal of reaching Bucharest. Goals organize Goal formulation behavior by limiting the objectives and hence the actions to be considered. 1 We are assuming that most readers are in the same position and can easily imagine themselves to be as clueless as our agent. We apologize to Romanian readers who are unable to take advantage of this pedagogical device. 64 Chapter 3 Solving Problems by Searching Oradea 71 Neamt Zerind 87 75 151 Iasi Arad 140 92 Sibiu Fagaras 99 118 Vaslui 80 Rimnicu Vilcea Timisoara 142 111 Pitesti 211 Lugoj 97 70 98 85 Hirsova Mehadia 146 101 Urziceni 75 138 86 Bucharest Drobeta 120 90 Craiova Eforie Giurgiu Figure 3.1 A simplified road map of part of Romania, with road distances in miles. Problem formulation Problem formulation: The agent devises a description of the states and actions nec- essary to reach the goal—an abstract model of the relevant part of the world. For our agent, one good model is to consider the actions of traveling from one city to an adja- cent city, and therefore the only fact about the state of the world that will change due to an action is the current city. Search Search: Before taking any action in the real world, the agent simulates sequences of actions in its model, searching until it finds a sequence of actions that reaches the goal. Solution Such a sequence is called a solution. The agent might have to simulate multiple se- quences that do not reach the goal, but eventually it will find a solution (such as going from Arad to Sibiu to Fagaras to Bucharest), or it will find that no solution is possible. Execution Execution: The agent can now execute the actions in the solution, one at a time. ◮ It is an important property that in a fully observable, deterministic, known environment, the solution to any problem is a fixed sequence of actions: drive to Sibiu, then Fagaras, then Bucharest. If the model is correct, then once the agent has found a solution, it can ignore its percepts while it is executing the actions—closing its eyes, so to speak—because the solution Open-loop is guaranteed to lead to the goal. Control theorists call this an open-loop system: ignoring the percepts breaks the loop between agent and environment. If there is a chance that the model is incorrect, or the environment is nondeterministic, then the agent would be safer using a Closed-loop closed-loop approach that monitors the percepts (see Section 4.4). In partially observable or nondeterministic environments, a solution would be a branching strategy that recommends different future actions depending on what percepts arrive. For example, the agent might plan to drive from Arad to Sibiu but might need a contingency plan in case it arrives in Zerind by accident or finds a sign saying “Drum Închis” (Road Closed). Section 3.1 Problem-Solving Agents 65 3.1.1 Search problems and solutions A search problem can be defined formally as follows: Problem A set of possible states that the environment can be in. We call this the state space. States The initial state that the agent starts in. For example: Arad. State space Initial state A set of one or more goal states. Sometimes there is one goal state (e.g., Bucharest), sometimes there is a small set of alternative goal states, and sometimes the goal is Goal states defined by a property that applies to many states (potentially an infinite number). For example, in a vacuum-cleaner world, the goal might be to have no dirt in any location, regardless of any other facts about the state. We can account for all three of these possibilities by specifying an I S -G OAL method for a problem. In this chapter we will sometimes say “the goal” for simplicity, but what we say also applies to “any one of the possible goal states.” The actions available to the agent. Given a state s, ACTIONS (s) returns a finite2 set of Action actions that can be executed in s. We say that each of these actions is applicable in s. Applicable An example: ACTIONS (Arad) = {ToSibiu, ToTimisoara, ToZerind}. A transition model, which describes what each action does. R ESULT (s, a) returns the Transition model state that results from doing action a in state s. For example, R ESULT (Arad, ToZerind) = Zerind. An action cost function, denoted by ACTION -C OST (s, a, s′ ) when we are programming Action cost function or c(s, a, s′ ) when we are doing math, that gives the numeric cost of applying action a in state s to reach state s′. A problem-solving agent should use a cost function that reflects its own performance measure; for example, for route-finding agents, the cost of an action might be the length in miles (as seen in Figure 3.1), or it might be the time it takes to complete the action. A sequence of actions forms a path, and a solution is a path from the initial state to a goal Path state. We assume that action costs are additive; that is, the total cost of a path is the sum of the individual action costs. An optimal solution has the lowest path cost among all solutions. In Optimal solution this chapter, we assume that all action costs will be positive, to avoid certain complications.3 The state space can be represented as a graph in which the vertices are states and the Graph directed edges between them are actions. The map of Romania shown in Figure 3.1 is such a graph, where each road indicates two actions, one in each direction. 2 For problems with an infinite number of actions we would need techniques that go beyond this chapter. 3 In any problem with a cycle of net negative cost, the cost-optimal solution is to go around that cycle an infinite number of times. The Bellman–Ford and Floyd–Warshall algorithms (not covered here) handle negative-cost actions, as long as there are no negative cycles. It is easy to accommodate zero-cost actions, as long as the number of consecutive zero-cost actions is bounded. For example, we might have a robot where there is a cost to move, but zero cost to rotate 90o ; the algorithms in this chapter can handle this as long as no more than three consecutive 90o turns are allowed. There is also a complication with problems that have an infinite number of arbitrarily small action costs. Consider a version of Zeno’s paradox where there is an action to move half way to the goal, at a cost of half of the previous move. This problem has no solution with a finite number of actions, but to prevent a search from taking an unbounded number of actions without quite reaching the goal, we can require that all action costs be at least ǫ, for some small positive value ǫ. 66 Chapter 3 Solving Problems by Searching 3.1.2 Formulating problems Our formulation of the problem of getting to Bucharest is a model—an abstract mathematical description—and not the real thing. Compare the simple atomic state description Arad to an actual cross-country trip, where the state of the world includes so many things: the traveling companions, the current radio program, the scenery out of the window, the proximity of law enforcement officers, the distance to the next rest stop, the condition of the road, the weather, the traffic, and so on. All these considerations are left out of our model because they are irrelevant to the problem of finding a route to Bucharest. Abstraction The process of removing detail from a representation is called abstraction. A good problem formulation has the right level of detail. If the actions were at the level of “move the right foot forward a centimeter” or “turn the steering wheel one degree left,” the agent would probably never find its way out of the parking lot, let alone to Bucharest. Level of abstraction Can we be more precise about the appropriate level of abstraction? Think of the abstract states and actions we have chosen as corresponding to large sets of detailed world states and detailed action sequences. Now consider a solution to the abstract problem: for example, the path from Arad to Sibiu to Rimnicu Vilcea to Pitesti to Bucharest. This abstract solution corresponds to a large number of more detailed paths. For example, we could drive with the radio on between Sibiu and Rimnicu Vilcea, and then switch it off for the rest of the trip. The abstraction is valid if we can elaborate any abstract solution into a solution in the more detailed world; a sufficient condition is that for every detailed state that is “in Arad,” there is a detailed path to some state that is “in Sibiu,” and so on.4 The abstraction is useful if carrying out each of the actions in the solution is easier than the original problem; in our case, the action “drive from Arad to Sibiu” can be carried out without further search or planning by a driver with average skill. The choice of a good abstraction thus involves removing as much detail as possible while retaining validity and ensuring that the abstract actions are easy to carry out. Were it not for the ability to construct useful abstractions, intelligent agents would be completely swamped by the real world. 3.2 Example Problems The problem-solving approach has been applied to a vast array of task environments. We list some of the best known here, distinguishing between standardized and real-world problems. Standardized problem A standardized problem is intended to illustrate or exercise various problem-solving meth- ods. It can be given a concise, exact description and hence is suitable as a benchmark for Real-world problem researchers to compare the performance of algorithms. A real-world problem, such as robot navigation, is one whose solutions people actually use, and whose formulation is idiosyn- cratic, not standardized, because, for example, each robot has different sensors that produce different data. 3.2.1 Standardized problems Grid world A grid world problem is a two-dimensional rectangular array of square cells in which agents can move from cell to cell. Typically the agent can move to any obstacle-free adjacent cell— horizontally or vertically and in some problems diagonally. Cells can contain objects, which 4 See Section 11.4. Section 3.2 Example Problems 67 R L R L S S R R L R L R L L S S S S R L R L S S Figure 3.2 The state-space graph for the two-cell vacuum world. There are 8 states and three actions for each state: L = Left, R = Right, S = Suck. the agent can pick up, push, or otherwise act upon; a wall or other impassible obstacle in a cell prevents an agent from moving into that cell. The vacuum world from Section 2.1 can be formulated as a grid world problem as follows: States: A state of the world says which objects are in which cells. For the vacuum world, the objects are the agent and any dirt. In the simple two-cell version, the agent can be in either of the two cells, and each call can either contain dirt or not, so there are 2 · 2 · 2 = 8 states (see Figure 3.2). In general, a vacuum environment with n cells has n · 2n states. Initial state: Any state can be designated as the initial state. Actions: In the two-cell world we defined three actions: Suck, move Left, and move Right. In a two-dimensional multi-cell world we need more movement actions. We could add Upward and Downward, giving us four absolute movement actions, or we could switch to egocentric actions, defined relative to the viewpoint of the agent—for example, Forward, Backward, TurnRight, and TurnLeft. Transition model: Suck removes any dirt from the agent’s cell; Forward moves the agent ahead one cell in the direction it is facing, unless it hits a wall, in which case the action has no effect. Backward moves the agent in the opposite direction, while TurnRight and TurnLeft change the direction it is facing by 90◦. Goal states: The states in which every cell is clean. Action cost: Each action costs 1. Another type of grid world is the sokoban puzzle, in which the agent’s goal is to push a Sokoban puzzle number of boxes, scattered about the grid, to designated storage locations. There can be at most one box per cell. When an agent moves forward into a cell containing a box and there is an empty cell on the other side of the box, then both the box and the agent move forward. 68 Chapter 3 Solving Problems by Searching 7 2 4 1 2 5 6 3 4 5 8 3 1 6 7 8 Start State Goal State Figure 3.3 A typical instance of the 8-puzzle. The agent can’t push a box into another box or a wall. For a world with n non-obstacle cells and b boxes, there are n × n!/(b!(n − b)!) states; for example on an 8 × 8 grid with a dozen boxes, there are over 200 trillion states. Sliding-tile puzzle In a sliding-tile puzzle, a number of tiles (sometimes called blocks or pieces) are ar- ranged in a grid with one or more blank spaces so that some of the tiles can slide into the blank space. One variant is the Rush Hour puzzle, in which cars and trucks slide around a 6 × 6 grid in an attempt to free a car from the traffic jam. Perhaps the best-known variant is 8-puzzle the 8-puzzle (see Figure 3.3), which consists of a 3 × 3 grid with eight numbered tiles and 15-puzzle one blank space, and the 15-puzzle on a 4 × 4 grid. The object is to reach a specified goal state, such as the one shown on the right of the figure. The standard formulation of the 8 puzzle is as follows: States: A state description specifies the location of each of the tiles. Initial state: Any state can be designated as the initial state. Note that a parity prop- erty partitions the state space—any given goal can be reached from exactly half of the possible initial states (see Exercise 3.PART ). Actions: While in the physical world it is a tile that slides, the simplest way of describ- ing an action is to think of the blank space moving Left, Right, Up, or Down. If the blank is at an edge or corner then not all actions will be applicable. Transition model: Maps a state and action to a resulting state; for example, if we apply Left to the start state in Figure 3.3, the resulting state has the 5 and the blank switched. Goal state: Although any state could be the goal, we typically specify a state with the numbers in order, as in Figure 3.3. Action cost: Each action costs 1. Note that every problem formulation involves abstractions. The 8-puzzle actions are ab- stracted to their beginning and final states, ignoring the intermediate locations where the tile is sliding. We have abstracted away actions such as shaking the board when tiles get stuck and ruled out extracting the tiles with a knife and putting them back again. We are left with a description of the rules, avoiding all the details of physical manipulations. Our final standardized problem was devised by Donald Knuth (1964) and illustrates how infinite state spaces can arise. Knuth conjectured that starting with the number 4, a sequence Section 3.2 Example Problems 69 of square root, floor, and factorial operations can reach any desired positive integer. For example, we can reach 5 from 4 as follows: vs u r ju t qp k (4!)! = 5. The problem definition is simple: States: Positive real numbers. Initial state: 4. Actions: Apply square root, floor, or factorial operation (factorial for integers only). Transition model: As given by the mathematical definitions of the operations. Goal state: The desired positive integer. Action cost: Each action costs 1. The state space for this problem is infinite: for any integer greater than 2 the factorial oper- ator will always yield a larger integer. The problem is interesting because it explores very large numbers: the shortest path to 5 goes through (4!)! = 620,448,401,733,239,439,360,000. Infinite state spaces arise frequently in tasks involving the generation of mathematical expres- sions, circuits, proofs, programs, and other recursively defined objects. 3.2.2 Real-world problems We have already seen how the route-finding problem is defined in terms of specified lo- cations and transitions along edges between them. Route-finding algorithms are used in a variety of applications. Some, such as Web sites and in-car systems that provide driving directions, are relatively straightforward extensions of the Romania example. (The main complications are varying costs due to traffic-dependent delays, and rerouting due to road closures.) Others, such as routing video streams in computer networks, military operations planning, and airline travel-planning systems, involve much more complex specifications. Consider the airline travel problems that must be solved by a travel-planning Web site: States: Each state obviously includes a location (e.g., an airport) and the current time. Furthermore, because the cost of an action (a flight segment) may depend on previous segments, their fare bases, and their status as domestic or international, the state must record extra information about these “historical” aspects. Initial state: The user’s home airport. Actions: Take any flight from the current location, in any seat class, leaving after the current time, leaving enough time for within-airport transfer if needed. Transition model: The state resulting from taking a flight will have the flight’s desti- nation as the new location and the flight’s arrival time as the new time. Goal state: A destination city. Sometimes the goal can be more complex, such as “arrive at the destination on a nonstop flight.” Action cost: A combination of monetary cost, waiting time, flight time, customs and immigration procedures, seat quality, time of day, type of airplane, frequent-flyer re- ward points, and so on. 70 Chapter 3 Solving Problems by Searching Commercial travel advice systems use a problem formulation of this kind, with many addi- tional complications to handle the airlines’ byzantine fare structures. Any seasoned traveler knows, however, that not all air travel goes according to plan. A really good system should in- clude contingency plans—what happens if this flight is delayed and the connection is missed? Touring problem Touring problems describe a set of locations that must be visited, rather than a single Traveling goal destination. The traveling salesperson problem (TSP) is a touring problem in which salesperson problem every city on a map must be visited. The aim is to find a tour with cost < C (or in the (TSP) optimization version, to find a tour with the lowest cost possible). An enormous amount of effort has been expended to improve the capabilities of TSP algorithms. The algorithms can also be extended to handle fleets of vehicles. For example, a search and optimization algorithm for routing school buses in Boston saved $5 million, cut traffic and air pollution, and saved time for drivers and students (Bertsimas et al., 2019). In addition to planning trips, search algorithms have been used for tasks such as planning the movements of automatic circuit-board drills and of stocking machines on shop floors. VLSI layout A VLSI layout problem requires positioning millions of components and connections on a chip to minimize area, minimize circuit delays, minimize stray capacitances, and maximize manufacturing yield. The layout problem comes after the logical design phase and is usually split into two parts: cell layout and channel routing. In cell layout, the primitive components of the circuit are grouped into cells, each of which performs some recognized function. Each cell has a fixed footprint (size and shape) and requires a certain number of connections to each of the other cells. The aim is to place the cells on the chip so that they do not overlap and so that there is room for the connecting wires to be placed between the cells. Channel routing finds a specific route for each wire through the gaps between the cells. These search problems are extremely complex, but definitely worth solving. Robot navigation Robot navigation is a generalization of the route-finding problem described earlier. Rather than following distinct paths (such as the roads in Romania), a robot can roam around, in effect making its own paths. For a circular robot moving on a flat surface, the space is essentially two-dimensional. When the robot has arms and legs that must also be controlled, the search space becomes many-dimensional—one dimension for each joint angle. Advanced techniques are required just to make the essentially continuous search space finite (see Chap- ter 26). In addition to the complexity of the problem, real robots must also deal with errors in their sensor readings and motor controls, with partial observability, and with other agents that might alter the environment. Automatic assembly sequencing Automatic assembly sequencing of complex objects (such as electric motors) by a robot has been standard industry practice since the 1970s. Algorithms first find a feasible assembly sequence and then work to optimize the process. Minimizing the amount of manual human labor on the assembly line can produce significant savings in time and cost. In assembly problems, the aim is to find an order in which to assemble the parts of some object. If the wrong order is chosen, there will be no way to add some part later in the sequence without undoing some of the work already done. Checking an action in the sequence for feasibility is a difficult geometrical search problem closely related to robot navigation. Thus, the generation of legal actions is the expensive part of assembly sequencing. Any practical algorithm must avoid exploring all but a tiny fraction of the state space. One important assembly problem is Protein design protein design, in which the goal is to find a sequence of amino acids that will fold into a three-dimensional protein with the right properties to cure some disease. Section 3.3 Search Algorithms 71 3.3 Search Algorithms A search algorithm takes a search problem as input and returns a solution, or an indication of Search algorithm failure. In this chapter we consider algorithms that superimpose a search tree over the state- space graph, forming various paths from the initial state, trying to find a path that reaches a goal state. Each node in the search tree corresponds to a state in the state space and the edges Node in the search tree correspond to actions. The root of the tree corresponds to the initial state of the problem. It is important to understand the distinction between the state space and the search tree. The state space describes the (possibly infinite) set of states in the world, and the actions that allow transitions from one state to another. The search tree describes paths between these states, reaching towards the goal. The search tree may have multiple paths to (and thus multiple nodes for) any given state, but each node in the tree has a unique path back to the root (as in all trees). Figure 3.4 shows the first few steps in finding a path from Arad to Bucharest. The root node of the search tree is at the initial state, Arad. We can expand the node, by considering Expand Arad Sibiu Timisoara Zerind Arad Fagaras Oradea Rimnicu Vilcea Arad Lugoj Arad Oradea Arad Sibiu Timisoara Zerind Arad Fagaras Oradea Rimnicu Vilcea Arad Lugoj Arad Oradea Arad Sibiu Timisoara Zerind Arad Fagaras Oradea Rimnicu Vilcea Arad Lugoj Arad Oradea Figure 3.4 Three partial search trees for finding a route from Arad to Bucharest. Nodes that have been expanded are lavender with bold letters; nodes on the frontier that have been generated but not yet expanded are in green; the set of states corresponding to these two types of nodes are said to have been reached. Nodes that could be generated next are shown in faint dashed lines. Notice in the bottom tree there is a cycle from Arad to Sibiu to Arad; that can’t be an optimal path, so search should not continue from there. 72 Chapter 3 Solving Problems by Searching Figure 3.5 A sequence of search trees generated by a graph search on the Romania problem of Figure 3.1. At each stage, we have expanded every node on the frontier, extending every path with all applicable actions that don’t result in a state that has already been reached. Notice that at the third stage, the topmost city (Oradea) has two successors, both of which have already been reached by other paths, so no paths are extended from Oradea. (a) (b) (c) Figure 3.6 The separation property of graph search, illustrated on a rectangular-grid prob- lem. The frontier (green) separates the interior (lavender) from the exterior (faint dashed). The frontier is the set of nodes (and corresponding states) that have been reached but not yet expanded; the interior is the set of nodes (and corresponding states) that have been expanded; and the exterior is the set of states that have not been reached. In (a), just the root has been expanded. In (b), the top frontier node is expanded. In (c), the remaining successors of the root are expanded in clockwise order. the available ACTIONS for that state, using the R ESULT function to see where those actions Generating lead to, and generating a new node (called a child node or successor node) for each of the Child node resulting states. Each child node has Arad as its parent node. Successor node Now we must choose which of these three child nodes to consider next. This is the Parent node essence of search—following up one option now and putting the others aside for later. Sup- pose we choose to expand Sibiu first. Figure 3.4 (bottom) shows the result: a set of 6 unex- Frontier panded nodes (outlined in bold). We call this the frontier of the search tree. We say that any Reached state that has had a node generated for it has been reached (whether or not that node has been expanded).5 Figure 3.5 shows the search tree superimposed on the state-space graph. Separator Note that the frontier separates two regions of the state-space graph: an interior region where every state has been expanded, and an exterior region of states that have not yet been reached. This property is illustrated in Figure 3.6. 5 Some authors call the frontier the open list, which is both geographically less evocative and computationally less appropriate, because a queue is more efficient than a list here. Those authors use the term closed list to refer to the set of previously expanded nodes, which in our terminology would be the reached nodes minus the frontier. Section 3.3 Search Algorithms 73 function B EST-F IRST-S EARCH (problem, f ) returns a solution node or failure node← N ODE(S TATE=problem.INITIAL) frontier ← a priority queue ordered by f , with node as an element reached ← a lookup table, with one entry with key problem.I NITIAL and value node while not I S -E MPTY(frontier) do node← P OP(frontier) if problem.I S -G OAL(node.S TATE) then return node for each child in E XPAND(problem, node) do s ← child.S TATE if s is not in reached or child.PATH -C OST < reached[s].PATH -C OST then reached[s] ← child add child to frontier return failure function E XPAND( problem, node) yields nodes s ← node.S TATE for each action in problem.ACTIONS(s) do s′ ← problem.R ESULT(s, action) cost ← node.PATH -C OST + problem.ACTION -C OST(s, action, s′ ) yield N ODE(S TATE=s′ , PARENT=node, ACTION=action, PATH -C OST=cost) Figure 3.7 The best-first search algorithm, and the function for expanding a node. The data structures used here are described in Section 3.3.2. See Appendix B for yield. 3.3.1 Best-first search How do we decide which node from the frontier to expand next? A very general approach is called best-first search, in which we choose a node, n, with minimum value of some Best-first search evaluation function, f (n). Figure 3.7 shows the algorithm. On each iteration we choose Evaluation function a node on the frontier with minimum f (n) value, return it if its state is a goal state, and otherwise apply E XPAND to generate child nodes. Each child node is added to the frontier if it has not been reached before, or is re-added if it is now being reached with a path that has a lower path cost than any previous path. The algorithm returns either an indication of failure, or a node that represents a path to a goal. By employing different f (n) functions, we get different specific algorithms, which this chapter will cover. 3.3.2 Search data structures Search algorithms require a data structure to keep track of the search tree. A node in the tree is represented by a data structure with four components: node.S TATE : the state to which the node corresponds; node.PARENT: the node in the tree that generated this node; node.ACTION : the action that was applied to the parent’s state to generate this node; node.PATH -C OST : the total cost of the path from the initial state to this node. In math- ematical formulas, we use g(node) as a synonym for PATH -C OST. Following the PARENT pointers back from a node allows us to recover the states and actions along the path to that node. Doing this from a goal node gives us the solution. 74 Chapter 3 Solving Problems by Searching Queue We need a data structure to store the frontier. The appropriate choice is a queue of some kind, because the operations on a frontier are: I S -E MPTY (frontier) returns true only if there are no nodes in the frontier. P OP(frontier) removes the top node from the frontier and returns it. T OP(frontier) returns (but does not remove) the top node of the frontier. A DD(node, frontier) inserts node into its proper place in the queue. Three kinds of queues are used in search algorithms: Priority queue A priority queue first pops the node with the minimum cost according to some evalu- ation function, f. It is used in best-first search. FIFO queue A FIFO queue or first-in-first-out queue first pops the node that was added to the queue first; we shall see it is used in breadth-first search. LIFO queue A LIFO queue or last-in-first-out queue (also known as a stack) pops first the most Stack recently added node; we shall see it is used in depth-first search. The reached states can be stored as a lookup table (e.g. a hash table) where each key is a state and each value is the node for that state. 3.3.3 Redundant paths The search tree shown in Figure 3.4 (bottom) includes a path from Arad to Sibiu and back to Repeated state Arad again. We say that Arad is a repeated state in the search tree, generated in this case by Cycle a cycle (also known as a loopy path). So even though the state space has only 20 states, the Loopy path complete search tree is infinite because there is no limit to how often one can traverse a loop. Redundant path A cycle is a special case of a redundant path. For example, we can get to Sibiu via the path Arad–Sibiu (140 miles long) or the path Arad–Zerind–Oradea–Sibiu (297 miles long). This second path is redundant—it’s just a worse way to get to the same state—and need not be considered in our quest for optimal paths. Consider an agent in a 10 × 10 grid world, with the ability to move to any of 8 adjacent squares. If there are no obstacles, the agent can reach any of the 100 squares in 9 moves or fewer. But the number of paths of length 9 is almost 89 (a bit less because of the edges of the grid), or more than 100 million. In other words, the average cell can be reached by over a million redundant paths of length 9, and if we eliminate redundant paths, we can complete a ◮ search roughly a million times faster. As the saying goes, algorithms that cannot remember the past are doomed to repeat it. There are three approaches to this issue. First, we can remember all previously reached states (as best-first search does), allowing us to detect all redundant paths, and keep only the best path to each state. This is appropriate for state spaces where there are many redundant paths, and is the preferred choice when the table of reached states will fit in memory. Second, we can not worry about repeating the past. There are some problem formulations where it is rare or impossible for two paths to reach the same state. An example would be an assembly problem where each action adds a part to an evolving assemblage, and there is an ordering of parts so that it is possible to add A and then B, but not B and then A. For those problems, we could save memory space if we don’t track reached states and we don’t check Graph search for redundant paths. We call a search algorithm a graph search if it checks for redundant Tree-like search paths and a tree-like search6 if it does not check. The B EST-F IRST-S EARCH algorithm in Section 3.3 Search Algorithms 75 Figure 3.7 is a graph search algorithm; if we remove all references to reached we get a tree- like search that uses less memory but will examine redundant paths to the same state, and thus will run slower. Third, we can compromise and check for cycles, but not for redundant paths in general. Since each node has a chain of parent pointers, we can check for cycles with no need for additional memory by following up the chain of parents to see if the state at the end of the path has appeared earlier in the path. Some implementations follow this chain all the way up, and thus eliminate all cycles; other implementations follow only a few links (e.g., to the parent, grandparent, and great-grandparent), and thus take only a constant amount of time, while eliminating all short cycles (and relying on other mechanisms to deal with long cycles). 3.3.4 Measuring problem-solving performance Before we get into the design of various search algorithms, we will consider the criteria used to choose among them. We can evaluate an algorithm’s performance in four ways: Completeness: Is the algorithm guaranteed to find a solution when there is one, and to Completeness correctly report failure when there is not? Cost optimality: Does it find a solution with the lowest path cost of all solutions?7 Cost optimality Time complexity: How long does it take to find a solution? This can be measured in Time complexity seconds, or more abstractly by the number of states and actions considered. Space complexity: How much memory is needed to perform the search? Space complexity To understand completeness, consider a search problem with a single goal. That goal could be anywhere in the state space; therefore a complete algorithm must be capable of systematically exploring every state that is reachable from the initial state. In finite state spaces that is straightforward to achieve: as long as we keep track of paths and cut off ones that are cycles (e.g. Arad to Sibiu to Arad), eventually we will reach every reachable state. In infinite state spaces, more care is necessary. For example, an algorithm that repeatedly applied the “factorial” operator in Knuth’s “4” problem would follow an infinite path from 4 to 4! to (4!)!, and so on. Similarly, on an infinite grid with no obstacles, repeatedly moving forward in a straight line also follows an infinite path of new states. In both cases the algo- rithm never returns to a state it has reached before, but is incomplete because wide expanses of the state space are never reached. To be complete, a search algorithm must be systematic in the way it explores an infinite Systematic state space, making sure it can eventually reach any state that is connected to the initial state. For example, on the infinite grid, one kind of systematic search is a spiral path that covers all the cells that are s steps from the origin before moving out to cells that are s + 1 steps away. Unfortunately, in an infinite state space with no solution, a sound algorithm needs to keep searching forever; it can’t terminate because it can’t know if the next state will be a goal. Time and space complexity are considered with respect to some measure of the problem difficulty. In theoretical computer science, the typical measure is the size of the state-space graph, |V | + |E|, where |V | is the number of vertices (state nodes) of the graph and |E| is 6 We say “tree-like search” because the state space is still the same graph no matter how we search it; we are just choosing to treat it as if it were a tree, with only one path from each node back to the root. 7 Some authors use the term “admissibility” for the property of finding the lowest-cost solution, and some use just “optimality,” but that can be confused with other types of optimality. 76 Chapter 3 Solving Problems by Searching the number of edges (distinct state/action pairs). This is appropriate when the graph is an explicit data structure, such as the map of Romania. But in many AI problems, the graph is represented only implicitly by the initial state, actions, and transition model. For an implicit Depth state space, complexity can be measured in terms of d, the depth or number of actions in an optimal solution; m, the maximum number of actions in any path; and b, the branching Branching factor factor or number of successors of a node that need to be considered. 3.4 Uninformed Search Strategies An uninformed search algorithm is given no clue about how close a state is to the goal(s). For example, consider our agent in Arad with the goal of reaching Bucharest. An uninformed agent with no knowledge of Romanian geography has no clue whether going to Zerind or Sibiu is a better first step. In contrast, an informed agent (Section 3.5) who knows the location of each city knows that Sibiu is much closer to Bucharest and thus more likely to be on the shortest path. 3.4.1 Breadth-first search Breadth-first search When all actions have the same cost, an appropriate strategy is breadth-first search, in which the root node is expanded first, then all the successors of the root node are expanded next, then their successors, and so on. This is a systematic search strategy that is therefore com- plete even on infinite state spaces. We could implement breadth-first search as a call to B EST-F IRST-S EARCH where the evaluation function f (n) is the depth of the node—that is, the number of actions it takes to reach the node. However, we can get additional efficiency with a couple of tricks. A first-in-first-out queue will be faster than a priority queue, and will give us the correct order of nodes: new nodes (which are always deeper than their parents) go to the back of the queue, and old nodes, which are shallower than the new nodes, get expanded first. In addition, reached can be a set of states rather than a mapping from states to nodes, because once we’ve reached a state, Early goal test we can never find a better path to the state. That also means we can do an early goal test, Late goal test checking whether a node is a solution as soon as it is generated, rather than the late goal test that best-first search uses, waiting until a node is popped off the queue. Figure 3.8 shows the progress of a breadth-first search on a binary tree, and Figure 3.9 shows the algorithm with the early-goal efficiency enhancements. Breadth-first search always finds a solution with a minimal number of actions, because when it is generating nodes at depth d, it has already generated all the nodes at depth d − 1, so if one of them were a solution, it would have been found. That means it is cost-optimal A A A A B C B C B C B C D E F G D E F G D E F G D E F G Figure 3.8 Breadth-first search on a simple binary tree. At each stage, the node to be ex- panded next is indicated by the triangular marker. Section 3.4 Uninformed Search Strategies 77 function B READTH -F IRST-S EARCH ( problem) returns a solution node or failure node← N ODE(problem.INITIAL) if problem.I S -G OAL(node.S TATE) then return node frontier ← a FIFO queue, with node as an element reached ← {problem.INITIAL} while not I S -E MPTY(frontier) do node← P OP(frontier) for each child in E XPAND(problem, node) do s ← child.S TATE if problem.I S -G OAL(s) then return child if s is not in reached then add s to reached add child to frontier return failure function U NIFORM -C OST-S EARCH ( problem) returns a solution node, or failure return B EST-F IRST-S EARCH (problem, PATH -C OST) Figure 3.9 Breadth-first search and uniform-cost search algorithms. for problems where all actions have the same cost, but not for problems that don’t have that property. It is complete in either case. In terms of time and space, imagine searching a uniform tree where every state has b successors. The root of the search tree generates b nodes, each of which generates b more nodes, for a total of b2 at the second level. Each of these generates b more nodes, yielding b3 nodes at the third level, and so on. Now suppose that the solution is at depth d. Then the total number of nodes generated is 1 + b + b2 + b3 + · · · + bd = O(bd ) All the nodes remain in memory, so both time and space complexity are O(bd ). Exponential bounds like that are scary. As a typical real-world example, consider a problem with branch- ing factor b = 10, processing speed 1 million nodes/second, and memory requirements of 1 Kbyte/node. A search to depth d = 10 would take less than 3 hours, but would require 10 terabytes of memory. The memory requirements are a bigger problem for breadth-first search than the execution time. But time is still an important factor. At depth d = 14, even with ◭ infinite memory, the search would take 3.5 years. In general, exponential-complexity search problems cannot be solved by uninformed search for any but the smallest instances. ◭ 3.4.2 Dijkstra’s algorithm or uniform-cost search When actions have different costs, an obvious choice is to use best-first search where the evaluation function is the cost of the path from the root to the current node. This is called Di- jkstra’s algorithm by the theoretical computer science community, and uniform-cost search Uniform-cost search by the AI community. The idea is that while breadth-first search spreads out in waves of uni- form depth—first depth 1, then depth 2, and so on—uniform-cost search spreads out in waves of uniform path-cost. The algorithm can be implemented as a call to B EST-F IRST-S EARCH with PATH -C OST as the evaluation function, as shown in Figure 3.9. 78 Chapter 3 Solving Problems by Searching Sibiu Fagaras 99 80 Rimnicu Vilcea Pitesti 211 97 101 Bucharest Figure 3.10 Part of the Romania state space, selected to illustrate uniform-cost search. Consider Figure 3.10, where the problem is to get from Sibiu to Bucharest. The succes- sors of Sibiu are Rimnicu Vilcea and Fagaras, with costs 80 and 99, respectively. The least- cost node, Rimnicu Vilcea, is expanded next, adding Pitesti with cost 80 + 97 = 177. The least-cost node is now Fagaras, so it is expanded, adding Bucharest with cost 99 + 211 = 310. Bucharest is the goal, but the algorithm tests for goals only when it expands a node, not when it generates a node, so it has not yet detected that this is a path to the goal. The algorithm continues on, choosing Pitesti for expansion next and adding a second path to Bucharest with cost 80 + 97 + 101 = 278. It has a lower cost, so it replaces the previous path in reached and is added to the frontier. It turns out this node now has the lowest cost, so it is considered next, found to be a goal, and returned. Note that if we had checked for a goal upon generating a node rather than when expanding the lowest-cost node, then we would have returned a higher-cost path (the one through Fagaras). The complexity of uniform-cost search is characterized in terms of C∗ , the cost of the optimal solution,8 and ǫ, a lower bound on the cost of each action, with ǫ > 0. Then the ∗ algorithm’s worst-case time and space complexity is O(b1+⌊C /ǫ⌋ ), which can be much greater than bd. This is because uniform-cost search can explore large trees of actions with low costs before exploring paths involving a high-cost and perhaps useful action. When all action costs ∗ are equal, b1+⌊C /ǫ⌋ is just bd+1 , and uniform-cost search is similar to breadth-first search. Uniform-cost search is complete and is cost-optimal, because the first solution it finds will have a cost that is at least as low as the cost of any other node in the frontier. Uniform- cost search considers all paths systematically in order of increasing cost, never getting caught going down a single infinite path (assuming that all action costs are > ǫ > 0). 3.4.3 Depth-first search and the problem of memory Depth-first search Depth-first search always expands the deepest node in the frontier first. It could be imple- mented as a call to B EST-F IRST-S EARCH where the evaluation function f is the negative of the depth. However, it is usually implemented not as a graph search but as a tree-like search that does not keep a table of reached states. The progress of the search is illustrated in Figure 3.11; search proceeds immediately to the deepest level of the search tree, where the nodes have no successors. The search then “backs up” to the next deepest node that still has 8 Here, and throughout the book, the “star” in C∗ means an optimal value for C. Section 3.4 Uninformed Search Strategies 79 A A A B C B C B C D E F G D E F G D E F G H I J K L M N O H I J K L M N O H I J K L M N O A A A B C B C B C ✕ ✕ ✕ ✕ D E F G D E F G D E F G ✕✕ ✕✕ ✕✕ ✕✕ ✕✕ ✕ ✕ ✕ H I J K L M N O H I J K L M N O H I J K L M N O ✕ ✕ ✕ A A ✕ A ✕ ✕ ✕ ✕ ✕ B C B C B ✕ C ✕ ✕ ✕ ✕ ✕ ✕ ✕ ✕ ✕ ✕ ✕ ✕ ✕ ✕ ✕ ✕ D E F G D E F G D E F G ✕ ✕ ✕ ✕ ✕ ✕ ✕ ✕ ✕ ✕ ✕ ✕ ✕ ✕ ✕ ✕ ✕ ✕ ✕ ✕ ✕ ✕ ✕ ✕ ✕ ✕ ✕ ✕ ✕ ✕ ✕ ✕ ✕ H I J K L M N O H I J K L M N O H I J K L M N O ✕ ✕ ✕ ✕ ✕ ✕ ✕ ✕ ✕ ✕ A ✕ ✕ A ✕ ✕ ✕ A ✕ ✕ ✕ ✕ ✕ ✕ ✕ ✕ ✕ ✕ ✕ ✕ B ✕ C B ✕ C B ✕ C ✕ ✕ ✕ ✕ ✕ ✕ ✕ ✕ ✕ ✕ ✕ ✕ ✕ ✕ ✕ ✕ ✕ ✕ ✕ ✕ ✕ ✕ ✕ ✕ D E F G D E F G D E F G ✕ ✕ ✕ ✕ ✕ ✕ ✕ ✕ ✕ ✕ ✕ ✕ ✕ ✕ ✕ ✕ ✕ ✕ ✕ ✕ ✕ ✕ ✕ ✕ ✕ ✕ ✕ ✕ ✕ ✕ ✕ ✕ ✕ ✕ ✕ ✕ ✕ ✕ ✕ ✕ ✕ ✕ ✕ ✕ ✕ ✕ H I J K L M N O H I J K L M N O H I J K L M N O ✕ ✕ ✕ ✕ ✕ ✕ ✕ ✕ ✕ ✕ ✕ ✕ ✕ Figure 3.11 A dozen steps (left to right, top to bottom) in the progress of a depth-first search on a binary tree from start state A to goal M. The frontier is in green, with a triangle marking the node to be expanded next. Previously expanded nodes are lavender, and potential future nodes have faint dashed lines. Expanded nodes with no descendants in the frontier (very faint lines) can be discarded. unexpanded successors. Depth-first search is not cost-optimal; it returns the first solution it finds, even if it is not cheapest. For finite state spaces that are trees it is efficient and complete; for acyclic state spaces it may end up expanding the same state many times via different paths, but will (eventually) systematically explore the entire space. In cyclic state spaces it can get stuck in an infinite loop; therefore some implementations of depth-first search check each new node for cycles. Finally, in infinite state spaces, depth- first search is not systematic: it can get stuck going down an infinite path, even if there are no cycles. Thus, depth-first search is incomplete. With all this bad news, why would anyone consider using depth-first search rather than breadth-first or best-first? The answer is that for problems where a tree-like search is feasible, depth-first search has much smaller needs for memory. We don’t keep a reached table at all, and the frontier is very small: think of the frontier in breadth-first search as the surface of an ever-expanding sphere, while the frontier in depth-first search is just a radius of the sphere. 80 Chapter 3 Solving Problems by Searching For a finite tree-shaped state-space like the one in Figure 3.11, a depth-first tree-like search takes time proportional to the number of states, and has memory complexity of only O(bm), where b is the branching factor and m is the maximum depth of the tree. Some problems that would require exabytes of memory with breadth-first search can be handled with only kilobytes using depth-first search. Because of its parsimonious use of memory, depth-first tree-like search has been adopted as the basic workhorse of many areas of AI, including constraint satisfaction (Chapter 6), propositional satisfiability (Chapter 7), and logic programming (Chapter 9). Backtracking search A variant of depth-first search called backtracking search uses even less memory. (See Chapter 6 for more details.) In backtracking, only one successor is generated at a time rather than all successors; each partially expanded node remembers which successor to generate next. In addition, successors are generated by modifying the current state description directly rather than allocating memory for a brand-new state. This reduces the memory requirements to just one state description and a path of O(m) actions; a significant savings over O(bm) states for depth-first search. With backtracking we also have the option of maintaining an efficient set data structure for the states on the current path, allowing us to check for a cyclic path in O(1) time rather than O(m). For backtracking to work, we must be able to undo each action when we backtrack. Backtracking is critical to the success of many problems with large state descriptions, such as robotic assembly. 3.4.4 Depth-limited and iterative deepening search To keep depth-first search from wandering down an infinite path, we can use depth-limited Depth-limited search search, a version of depth-first search in which we supply a depth limit, ℓ, and treat all nodes at depth ℓ as if they had no successors (see Figure 3.12). The time complexity is O(bℓ ) and the space complexity is O(bℓ). Unfortunately, if we make a poor choice for ℓ the algorithm will fail to reach the solution, making it incomplete again. Since depth-first search is a tree-like search, we can’t keep it from wasting time on re- dundant paths in general, but we can eliminate cycles at the cost of some computation time. If we look only a few links up in the parent chain we can catch most cycles; longer cycles are handled by the depth limit. Sometimes a good depth limit can be chosen based on knowledge of the problem. For example, on the map of Romania there are 20 cities. Therefore, ℓ = 19 is a valid limit. But if we studied the map carefully, we would discover that any city can be reached from any other Diameter city in at most 9 actions. This number, known as the diameter of the state-space graph, gives us a better depth limit, which leads to a more efficient depth-limited search. However, for most problems we will not know a good depth limit until we have solved the problem. Iterative deepening Iterative deepening search solves the problem of picking a good value for ℓ by trying search all values: first 0, then 1, then 2, and so on—until either a solution is found, or the depth- limited search returns the failure value rather than the cutoff value. The algorithm is shown in Figure 3.12. Iterative deepening combines many of the benefits of depth-first and breadth-first search. Like depth-first search, its memory requirements are modest: O(bd) when there is a solution, or O(bm) on finite state spaces with no solution. Like breadth-first search, iterative deepening is optimal for problems where all actions have the same cost, and is complete on finite acyclic state spaces, or on any finite state space when we check nodes for cycles all the way up the path. Section 3.4 Uninformed Search Strategies 81 function I TERATIVE -D EEPENING -S EARCH( problem) returns a solution node or failure for depth = 0 to ∞ do result ← D EPTH -L IMITED -S EARCH( problem, depth) if result 6= cutoff then return result function D EPTH -L IMITED -S EARCH( problem, ℓ) returns a node or failure or cutoff frontier ← a LIFO queue (stack) with N ODE(problem.I NITIAL) as an element result ← failure while not I S -E MPTY(frontier) do node← P OP(frontier) if problem.I S -G OAL(node.S TATE) then return node if D EPTH(node) > ℓ then result ← cutoff else if not I S -C YCLE(node) do for each child in E XPAND(problem, node) do add child to frontier return result Figure 3.12 Iterative deepening and depth-limited tree-like search. Iterative deepening re- peatedly applies depth-limited search with increasing limits. It returns one of three different types of values: either a solution node; or failure, when it has exhausted all nodes and proved there is no solution at any depth; or cutoff , to mean there might be a solution at a deeper depth than ℓ. This is a tree-like search algorithm that does not keep track of reached states, and thus uses much less memory than best-first search, but runs the risk of visiting the same state mul- tiple times on different paths. Also, if the I S -C YCLE check does not check all cycles, then the algorithm may get caught in a loop. The time complexity is O(bd ) when there is a solution, or O(bm ) when there is none. Each iteration of iterative deepening search generates a new level, in the same way that breadth- first search does, but breadth-first does this by storing all nodes in memory, while iterative- deepening does it by repeating the previous levels, thereby saving memory at the cost of more time. Figure 3.13 shows four iterations of iterative-deepening search on a binary search tree, where the solution is found on the fourth iteration. Iterative deepening search may seem wasteful because states near the top of the search tree are re-generated multiple times. But for many state spaces, most of the nodes are in the bottom level, so it does not matter much that the upper levels are repeated. In an iterative deepening search, the nodes on the bottom level (depth d) are generated once, those on the next-to-bottom