Single-Trial Neural Correlates of Arm Movement Preparation PDF
Document Details
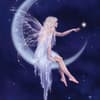
Uploaded by AppealingAmazonite
Afsheen Afshar, Gopal Santhanam, Byron M. Yu, Stephen I. Ryu, Maneesh Sahani, Krishna V. Shenoy
Tags
Summary
This paper examines the neural mechanisms behind arm movement preparation. It introduces a novel “initial condition hypothesis”, suggesting that the timing of movements can be predicted by firing rates and changes in firing rates of neurons in premotor cortex. The study uses simultaneous recordings from monkey brains; the model accurately predicts reaction times, outperforming alternative methods by 4x.
Full Transcript
Neuron Article Single-Trial Neural Correlates of Arm Movement Preparation Afsheen Afshar,1,2 Gopal Santhanam,1 Byron M. Yu,1,5 Stephen I. Ryu,1,6 Maneesh Sahani,1,5,7 and Krishna V. Shenoy1,3,4,7,* 1Department of Electrical Engineering Scientist Training Program 3Department of Bioengineering 4Depart...
Neuron Article Single-Trial Neural Correlates of Arm Movement Preparation Afsheen Afshar,1,2 Gopal Santhanam,1 Byron M. Yu,1,5 Stephen I. Ryu,1,6 Maneesh Sahani,1,5,7 and Krishna V. Shenoy1,3,4,7,* 1Department of Electrical Engineering Scientist Training Program 3Department of Bioengineering 4Department of Neurobiology Stanford University, Stanford, CA 94305 USA 5Gatsby Computational Neuroscience Unit, University College London, London WC1N 3AR, UK 6Department of Neurosurgery, Palo Alto Medical Foundation, Palo Alto, CA 94301, USA 7These authors contributed equally to this work *Correspondence: [email protected] DOI 10.1016/j.neuron.2011.05.047 2Medical SUMMARY The process by which neural circuitry in the brain plans and executes movements is not well understood. Until recently, most available data were limited either to single-neuron electrophysiological recordings or to measures of aggregate field or metabolism. Neither approach reveals how individual neurons’ activities are coordinated within the population, and thus inferences about how the neural circuit forms a motor plan for an upcoming movement have been indirect. Here we build on recent advances in the measurement and description of population activity to frame and test an ‘‘initial condition hypothesis’’ of arm movement preparation and initiation. This hypothesis leads to a model in which the timing of movements may be predicted on each trial using neurons’ moment-by-moment firing rates and rates of change of those rates. Using simultaneous microelectrode array recordings from premotor cortex of monkeys performing delayed-reach movements, we compare such single-trial predictions to those of other theories. We show that our model can explain approximately 4-fold more arm-movement reaction-time variance than the best alternative method. Thus, the initial condition hypothesis elucidates a view of the relationship between single-trial preparatory neural population dynamics and single-trial behavior. INTRODUCTION In 1991, Leroy Burrell set a world record for the 100 m dash with a spectacular time of 9.90 s, stunning the prerace favorite Carl Lewis, who finished second with a time of 9.93 s. It was later noted, however, that Burrell was not the faster runner. Rather, his reaction time to the gun that marked the start of the race was much shorter than Lewis’s: a hair-trigger 117 ms against a relatively lethargic 166 ms. Without this difference, Lewis would have won handily. Why was Carl Lewis so much slower than Leroy Burrell to start the race that day? Of course, nonathletes also often prepare movements in anticipation of events: while preparing to swat a fly, to press a car accelerator when a traffic light turns green, or to select the appropriate button while playing a video game. Sometimes we are slow in reacting and sometimes we move before we are fully ready. This inability to precisely time the onset of a movement can often be extremely frustrating. What is the cause of this imprecision? Presumably, it is related to the operation of planning and executing movements. Voluntary movements are believed to be ‘‘prepared’’ before they are executed (e.g., Wise, 1985). Important evidence for this belief comes from behavioral tasks in which a delay period separates a stimulus instructing the goal of a reaching movement from a subsequent ‘‘go’’ cue. Reaction time (RT) is the time elapsed from the go cue until movement onset in these delayed-reach tasks, and RT is shorter when delays are longer (e.g., Rosenbaum, 1980; Riehle and Requin, 1989). This suggests that a time-consuming preparatory process is given a head start by the delay period. How is this process reflected in neural activity, and why should the preparation take time? One view, drawn by analogy to the oculomotor system (Hanes and Schall, 1996), would be that neural activity in a subpopulation of cells might be increased in order to reside near a threshold level, and that the initiation of movement would follow from the subsequent crossing of this threshold. Thus, if the distance of preparatory neural activity from this threshold were measured experimentally, it should correlate inversely with RT (Erlhagen and Schöner, 2002). Neurons in a number of brain areas, including dorsal premotor cortex (PMd), exhibit substantial activity during the delay (Tanji and Evarts, 1976; Weinrich and Wise, 1982), and this delayperiod activity changes according to the direction, distance, and speed of the upcoming movement (Messier and Kalaska, 2000; Churchland et al., 2006b). Electrical disruption of this activity in PMd largely erases the RT savings earned during the delay (Churchland and Shenoy, 2007a). PMd is thus broadly implicated in arm movement preparation. In support of the Neuron 71, 555–564, August 11, 2011 ª2011 Elsevier Inc. 555 Neuron Neural Correlates of Arm Movement Preparation neuron 3 A right reach across-trial mean left reach * * long RT ‘go’ cue short RT n (fi eur rin on g 1 ra te ) neuron 2 B target onset pgo ‘go’ cue Latent dimension 2 time target onset movement onset Latent dimension 1 long RT trial? short RT trial? C pggo *α pgo+Δt mean trajectory at t = ‘go’ cue + Δt Latent dimension 2 time across-trial mean neural trajectory * * movement boundary? Latent dimension 1 Figure 1. Illustrations of the Optimal Subspace Hypothesis, the Elaborated Optimal Subspace Hypothesis, and the Initial Condition Hypothesis Discussed in this Work (A) The optimal subspace hypothesis. The configuration of firing rates is represented in a state space, with the firing rate of each neuron contributing an axis, only three of which are drawn here. Under this hypothesis the goal of motor preparation is to optimize the configuration of firing rates so that it lies within the optimal subregion for the desired movement (small gray region with green outline). The formation of a motor plan for a given trial is represented by an individual gray trace. Adapted from Churchland et al. (2006c). (B) The elaborated optimal subspace hypothesis. The hypothesis extended to include the entire trial. The across-trial variance, represented in this illustration by the area of the colored ellipses, reduces from target onset (red) to go cue (green) to movement onset (blue). Bold dots represent individual trials at target onset, go cue, and movement onset. pgo marks the neural state of a particular trial at the time of the go cue. Adapted from Yu et al. (2009) and Churchland et al. (2010b). 556 Neuron 71, 555–564, August 11, 2011 ª2011 Elsevier Inc. ‘‘rise-to-threshold’’ hypothesis, higher firing rates in PMd are often associated with shorter RTs (Riehle and Requin, 1993; Bastian et al., 2003), although Crammond and Kalaska (2000) found that peak firing rates after the go cue, when the movement is presumably triggered, were on average lower after an instructed delay. We recently proposed an alternative hypothesis (Churchland et al., 2006c), illustrated in Figure 1. The ‘‘optimal subspace hypothesis’’ assumes that the movement produced is a function of the state of preparatory activity (pgo) at the time the movement is externally triggered. For each possible movement there would be an ‘‘optimal subspace’’: a subset of possible population firing rates that are appropriate to generate a sufficiently accurate movement. Motor preparation might therefore be an optimization in which firing rates are brought from their initial state to a state within the subregion of adequately planned movements (gray region with green outline in Figure 1A). Each point in this optimal subregion corresponds to movements that are planned equally well for the purpose of completing the behavioral task and receiving reward. Thus, firing rates would remain within this optimal region while awaiting the cue to initiate movement, so as to preserve the appropriately prepared state. This contrasts with the rise-to-threshold model, where the crossing of an appropriate threshold actually triggers the movement. The most obvious predictions of this optimal subspace hypothesis are well established: delay-period firing rates are concentrated in a subregion of the accessible space, and this subregion is different for each instructed movement. However, if evidence could be found to show that the brain actively attempted to contain firing rates within that subregion, and that a penalty was paid for failing to do so, then the optimal subspace hypothesis could prove to be a valuable framework for further investigation of arm movement preparation. Three recent experiments have probed the process of motor preparation further, yielding averaged measurements consistent with the optimal subspace hypothesis and motivating the present study of single-trial neural correlates of behavior. First, we found that movement speed is predicted by the state of preparatory activity at the time of presentation of the go cue (Churchland et al., 2006a, 2006b). Second, we found that the across-trial Fano Factor (FF; the variance in spike count normalized by the mean rate) in neural activity decreases after target onset and results in low across-trial FF at the time of the go cue (Churchland et al., 2010b). In Figure 1B, this is closely related to the reduction of across-trial scatter from the time the target (C) The initial condition hypothesis. In this work, we hypothesize that if the neural state on a given trial at the time of the go cue were far along the mean neural trajectory across all trials to that target, then that trial would have a short reaction time. This is possible due to the neural activity being closer to a movement boundary (dashed line). This corresponds to a given trial’s RT correlating with a (length of bold line segment), which is the projection of pgo along pgo + Dt. Vector pgo connects the mean neural activity at the go cue across all trials to a given target (green asterisk) to the neural activity measured at the go cue on a given trial. Vector pgo + Dt connects the mean neural activity at the go cue across all trials to a given target (green asterisk) to the mean neural activity at some offset after the go cue (Dt = 100 ms; see Figure S1B). Bold line is the mean neural trajectory. Colored asterisks are the mean neural state across all trials at target onset, go cue, and movement onset. Neuron Neural Correlates of Arm Movement Preparation A B delay period fixate, touch target onset reach ‘go’ cue movement onset o Preferred direction 0 o 360 hand eye 200 ms Figure 2. Task Design and Neural Data (A) Monkeys performed a delayed-reach task, similar to that described previously (Santhanam et al., 2006; Churchland et al., 2006c) while simultaneous neural data were recorded via a 96-channel microelectrode array (Blackrock Microsystems, Salt Lake City, Utah). Task illustration corresponds to the time of go cue onset. (B) One of 53 trials to a given target (G20040123, target 5, which is at a distance of 60 mm and angle 225 ). Gray corresponds to the baseline period (before a target is presented), red to the delay period (after target presentation but before go cue), green to the reaction time period (after go cue but before movement onset), and blue to movement period (after recorded movement onset). A spike raster is shown, which is organized with one neuron per row and with each tick corresponding to a spike time for a given neuron. Neurons are organized by preferred direction as determined by plan period activity. Hand and eye traces are also shown. appears (red dots) to the time that the go cue appears (green dots). Consistent with the idea that the brain actively attempts to bring firing rates to a focal subregion during the planning period, the variance between trials with RTs shorter than the median value was smaller at the go cue (lower FF) than that between trials with RTs in the upper half of the distribution (Churchland et al., 2006c). Finally, when the exact state of the preparatory activity is perturbed with electrical microstimulation, which most likely moves pgo in Figure 1B to outside of the optimal subregion, we found that the RT savings created by the delay period (i.e., presumed motor preparation) are largely erased (Churchland and Shenoy, 2007a). These initial experiments studied the process of preparation by averaging measures across multiple trials. Their consistency with the optimal subspace hypothesis motivated us to now ask how individual movements are prepared on individual trials and how the initiation of the movement is related to transition of activity from preparatory to movement states. More specifically, we asked how the preparatory activity at the time of the go cue is related to the reaction time on each individual trial. Our earlier work (Yu et al., 2009; Churchland et al., 2010b) revealed that neural activity across different trials to the same reach target becomes progressively more stereotyped during the planning and movement periods (Figure 1B). We wondered whether we could exploit this increasing stereotypy to predict single-trial behavior, by studying even subtle deviations from the mean. To see how this might be possible, consider the average neural activity across all trials to the given target, shown by the bold trace in Figure 1C. This can be viewed as a lowdimensional representation of the mean neural activity that creates the motor plan for, and generates the arm movement to, a given target. We hypothesized that if the point corresponding to the neural population activity were farther along this mean path on a given trial at the time of the go cue, but still within the optimal subspace, then that trial would have a correspondingly fast RT (compare points labeled ‘‘short RT’’ versus ‘‘long RT’’ in Figure 1C). This view is consistent with the hypothesis that there exists a boundary along the mean neural trajectory (dotted line in the figure), which is crossed at the initiation of a movement. Importantly, as discussed below, this is a different ‘‘threshold’’ than that of the rise-to-threshold models. This view augments the optimal subspace hypothesis, which does not suggest that different neural states within the optimal subregion would correspond to different RTs. We call this augmented view the ‘‘initial condition hypothesis,’’ as it is consistent with the idea that differences in RT reflect the different times taken for the motor network to evolve from each state of the optimal subregion to the states associated with motor initiation. To test this hypothesis we conducted experiments with rhesus monkeys performing a delayed-reach task while we recorded from tens to hundreds of neurons simultaneously (Churchland et al., 2007). Our subjects performed multiple reaches to different targets throughout the workspace (see Experimental Procedures for details). The task design is shown in Figure 2. Simultaneous measurement of multiple neurons is essential to gather enough information about the population preparatory state on a millisecond timescale to make it feasible to account for individual trial RTs. We found that visualizing these neural data in a lower dimensional space helped reveal a stereotyped ‘‘neural trajectory’’ (Yu et al., 2009; Churchland et al., 2010b) and helped lead to a new neural measure (based on our initial condition hypothesis) that predicts roughly four times more RT variance than previously published methods. RESULTS Neural Activity Predicts Trial-by-Trial RT A low-dimensional representation of neural data from our experiments is shown in Figure 3. Figure 3A shows neural data from three reaches to a given target, while Figure 3B shows all of the 49 reaches. Dimensionality reduction was performed using Gaussian-process factor analysis (GPFA); see Experimental Neuron 71, 555–564, August 11, 2011 ª2011 Elsevier Inc. 557 Neuron Neural Correlates of Arm Movement Preparation 0.6 target onset 0.4 Latent Dimension 2 0.8 B Latent Dimension 2 A 0.2 movement onset 0 −0.2 −0.4 0.4 0 −0.4 ‘go’ cue −0.6 −0.8 −0.5 0 −0.8 1 0.5 −0.4 0 Latent Dimension 1 0.4 0.8 1.2 Latent Dimension 1 Normalized Drift Speed (spikes/s/ms/neuron) C 0.005 0 −200 −100 0 100 200 300 400 500 600 700 time relative to target onset (ms) Target Onset D Monkey H Monkey G 14 12 6 p = 6.42e−08 5 p = 0.00137 count count 10 8 6 4 3 4 2 2 1 0 −0.5 0 0.5 correlation coeff 0 −0.5 0 correlation coeff 0.5 Figure 3. Low-Dimensional Representations of Recorded Neural Data and Correlations of Single-Trial RT Predictions with RT (A and B) GPFA reductions of neural data recorded for three randomly selected (A) and for all 49 (B) preparations and movement initiations to the same target (G20040123, target 13, which is at a distance of 100 mm and angle 45 ). Same color code is used here as in the previous figure. The neural states at the time of target onset are bold red dots, at the time of the go cue are bold green dots, and at the time of measured movement onset are bold blue dots. Lighter dots are separated by 20 ms. (C) Normalized path neural speed in GPFA space as a function of time relative to target onset. Same color code used here as in previous figures. Dark black trace is the mean speed across all trials. Note that this speed increases after target onset and decreases to near zero until the go cue (green portion of traces). (D) Histograms of correlations coefficients of neural metric described in Figure 1C with Dt = 100 ms across all reach targets performed by two monkeys (G and H). The medians of both distributions (marked with arrows) are not 0 with p < 0.01 (Wilcoxon signed-rank test). Colored bars represent those correlations that are statistically significant (p < 0.05). Procedures for details (also Yu et al., 2009; Churchland et al., 2010b). Note that qualitatively similar results are obtained when using principal components analysis (PCA), but in general 558 Neuron 71, 555–564, August 11, 2011 ª2011 Elsevier Inc. PCA can be erroneously dominated by just a few high-firing-rate neurons (Yu et al., 2009). As in the illustrations in Figure 1, the neural activity seems to behave in a stereotyped way during Neuron Neural Correlates of Arm Movement Preparation pgo+Δt Latent dimension 2 time across-trial mean neural trajectory * Monkey G B 25 mean trajectory at t = ‘go’ cue + Δt count * 20 p = 0.00129 15 10 5 * 0 −0.5 0 0.5 correlation coeff Monkey H 7 6 5 4 3 2 1 0 −0.5 p = 4.33e-06 count vgo A 0 0.5 correlation coeff Latent dimension 1 Figure 4. Illustration Depicting Neural Velocity Correlate and Resulting Histogram of Correlation Coefficients When Using Neural Velocity to Predict RT for All Targets by Two Monkeys (A) Neural velocity at time t, labeled vt, was defined as the neural position at t + 10 ms – position at t – 10 ms. The component of vgo along the mean neural trajectory across trials was correlated with that trial’s RT. (B) Histogram of correlation coefficients from all comparisons of projections of neural velocity with trial-by-trial RT for monkeys G and H when segregating by delay period in 100 ms bins. Medians are denoted by arrows and gray bars represent significant correlations (p < 0.05). The medians of both distributions (marked with arrows) are not 0 with p < 0.01 (Wilcoxon sign-rank test). motor planning and execution. Notably, the three trials shown are in approximately the same location in the GPFA state-space at the time of target onset (red points in Figure 1A). The neural states during all three trials then move together along the second latent dimension during the plan period (red traces) before changing direction after the go cue is given (green and blue traces are along a different direction than red traces). This stereotypy is also evident even when looking at all trials to a given reach target (Figure 3B). Furthermore, the drift speed in neural space (calculated by measuring the distance traveled per time step along the mean neural trajectory) also has a stereotyped shape and decreases markedly after about 200 ms, which is the approximate presumed length of time required for motor preparation (Figure 3C). As described above, we reasoned that the degree to which the neural state had advanced by the time of the go cue along the mean neural path across similar trials would be predictive of RT (Figure 1C). To test this, we calculated the projection of an individual trial’s neural activities along the mean neural path (the ‘‘mean neural trajectory’’) for the appropriate target. This is shown in Figure 1C as a, which is the length of the bold line segment. This segment is the projection of the vector pgo along the vector pgo + Dt ; pgo links the target’s mean neural activities at the go cue to the activity on a single trial at the go cue, while pgo + Dt links the target’s mean neural activities at the go cue to the mean neural activities at a time Dt later for this target. This projection was correlated with the reaction time for all trials to the same target on a trial-by-trial basis. The offset Dt was chosen to maximize the average RT variance explained across all data sets (100 ms for our data; see Figure S1B). The exact Dt used does not appear to be critical, as any from a range of values yields similar results (Figure S1B). This analysis and all subsequent analyses were performed without dimensionality reduction so as to preserve complete information about firing rates from all neurons recorded. Histograms of correlation coefficients across all reach targets for both monkeys are shown in Figure 3D. For both monkeys, the histograms are shifted significantly to the negative values, with medians less than zero (p < 0.01; Wilcoxon signed-rank test). This is consistent with the hypothesis that trials with neural activities that are farther along the mean neural trajectory at the time of the go cue have shorter RTs, which predicts that correlation coefficients should be negative. Thus, these data are consistent with the hypothesis as depicted in Figure 1C. We performed several controls, as described in Figure S1, to rule out some alternative hypotheses, as well as potential artifacts in the experimental design or analysis. Specifically, we found that a model based on the distance between the neural state and an arbitrary reference point performed more poorly (Figures S1A and S1B); our results did not depend on the inclusion of multineuron units (Figure S1C and qualitative observations that spike sorting was of good quality); subjects remained motivated during the planning period (Figures S1D and S1E); the smoothing used to create continuous firing rates from spike times did not introduce an artifact (Figure S1F); and the results could not be explained by a systematic change of neural position with delay period (Figure S1G), by small anticipatory arm movements during the delay period (Figures S1H– S1J), or by small muscle contractions as measured by EMG (Figures S1J–S1L). Rate of Change of Neural Activity Improves Trial-by-Trial RT Predictions The results of the previous section show that the degree to which the neural activity has progressed along the average neural trajectory by the time of the go cue (the ‘‘neural position’’) is predictive of RT trial-by-trial. We further hypothesized that the direction and rate of change of neural activity at the time of the go cue (the ‘‘neural velocity’’) also relates to that trial’s RT. We investigated this possibility using a similar analysis to that above, but now correlating the neural velocity at the time of the go cue (vgo) projected onto the mean neural trajectory with RT (Figure 4A). In order to isolate the effects of neural velocity from position, we grouped trials together that had similar neural positions, which was done by further segregating our data by delay period into 100 ms bins (justified by results in Figure S1G). As shown in Figure 4B, for both monkeys the histograms have medians significantly less than zero (p < 0.01; Wilcoxon Neuron 71, 555–564, August 11, 2011 ª2011 Elsevier Inc. 559 Neuron Neural Correlates of Arm Movement Preparation 0.2 fraction of targets with significant (p400 ms for monkey G and > 700 ms for monkey H). These data sets come from experiments that were designed to address a number of questions, only some of which are considered in the current study. For this reason, the different data sets differ modestly in the task details. For data sets G20040120–G20040123, targets were presented in seven directions (45 , 90 , 135 , 180 , 225 , and 315 ) and two distances (e.g., 60 and 100 mm). For data set G20040119, targets were located in a grid 20 3 20 cm at 5 cm increments. Three targets that were covered by the outstretched arm (located on the bottom half of the vertical column in the middle of the grid) were removed, thereby making 22 total possible targets. For monkey H, targets were located at eight possible directions (0 , 45 , 70 , 110 , 150 , 190 , 230 , 310 , and 350 ) and two possible distances (70 and 120 mm) for H20041119 or one distance (100 mm) for H20041217. These variations in design across data sets serve, if anything, to strengthen the result of this study because similar effects were found regardless of the details of the task. Note that some of these data sets are the same ones used in previous studies (Churchland et al., 2006c; Santhanam et al., 2006). For all data sets, trials that had outlier RTs (>500 ms and < 150 ms) were not analyzed. This comprised a small percentage (