ANCOVA and MANCOVA Discussion PDF
Document Details
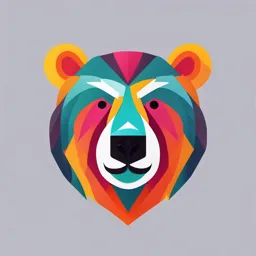
Uploaded by CourageousCyclops
Rizal Technological University
Tags
Summary
This document discusses ANCOVA and MANCOVA, including assumptions and procedures for conducting these analyses. It covers various aspects of experimental design and data analysis, especially when dealing with multiple dependent variables. The document highlights the importance of checking assumptions like normality and homogeneity of variance before performing the analyses.
Full Transcript
# BONFERRONI CORRECTION (Source: https://www.statisticssolutions.com/bonferroni-correction/) Bonferroni Correction is also known as Bonferroni type adjustment Made for inflated Type I error (the higher the chance for a false positive; rejecting the null hypothesis when you should not) When conduc...
# BONFERRONI CORRECTION (Source: https://www.statisticssolutions.com/bonferroni-correction/) Bonferroni Correction is also known as Bonferroni type adjustment Made for inflated Type I error (the higher the chance for a false positive; rejecting the null hypothesis when you should not) When conducting multiple analyses on the same dependent variable, the chance of committing a Type I error increases, thus increasing the likelihood of coming about a significant result by pure chance. To correct for this, or protect from Type I error, a Bonferroni correction is conducted. Bonferroni correction is a conservative test that, although protects from Type I Error, is vulnerable to Type II errors (failing to reject the null hypothesis when you should in fact reject the null hypothesis) same dependent variable However, when reporting the new p-value, the rounded version (of 3 decimal places) is typically reported. This rounded version is not technically correct; a rounding error. Example: 13 correlation analyses on the same dependent variable would indicate the need for a Bonferroni correction of (xaltered =.05/13) = .004 (rounded), but acritical = 1 - (1-.004)^13 = 0.051, which is not less than 0.05. But with the non-rounded version: (xaltered =.05/13) = .003846154, and acritical = 1 – (1 – .003846154)^13 = 0.048862271, which is in-fact less than 0.05! SPSS does not currently have the capability to set alpha levels beyond 3 decimal places, so the rounded version is presented and used. ## DISCUSSION (Refer to Data and Data (2) Tabs) ### ANCOVA - No. of IV = 1 - No of CV =2 - No of DV =1 - Number of Analyses for DV = 2 (because of 2 covariates present for univariate analysis - conservative approach) - Original p - value = 0.05; Adjusted p-value = 0.05/2 = 0.025 ### MANCOVA - No. of IV = 1 - No of CV =1 - No of DV =2 - Number of Analyses for DV = 2 (because of 2 DVs are present) - Original p - value = 0.05; Adjusted p-value = 0.05/2 = 0.025 ## ASSUMPTIONS | Assumption | ANCOVA/Factorial | MANCOVA/ Factorial | ANCOVA | |---|---|---|---| | Independent Variable | (one-way); 2 or more (factorial); categorical | (one-way); 2 or more (factorial); categorical | | | Dependent Variable | 1 continuous | 2 or more; continuous | | | Covariate/ Control Variables | 1 or more; continuous | 1 or more; continuous | | | Study Design | Independence of Observation (Diff participants in each group with no one being in more than one group) | | | | Outliers | no significant unusual points; three main types of unusual point- outliers, leverage points and influential points; tested by inspecting the values of the studentized residuals, the leverage values and Cook's distance values. | 1) No significant univariate outliers in the groups of your independent variable in terms of each dependent variable. Univariate outliers can be detected by inspecting the standardized residuals that can be produced using SPSS Statistics. 2) No significant multivariate outliers in the groups of your independent variable in terms of each dependent variable. Mahalanobis distance used to determine whether a particular case might be a multivariate outlier. | 1) Cook's distance - cut-off is 4/n where n = sample size 2) Standardized residuals - within +/- 1.96 to +/-3 (extremes); to detect outliers 3) Standardized residuals - cut off is 3*(k+1)/n where k is the # of Ivs; to detect leverage | | Normality | Yes; to test using Shapiro-Wilk| | Yes; to test using Shapiro-Wilk | | Homogeneity of Variance | Not needed | Yes, to test using Box's M Test of Equality of Covariance Matrices | Not needed | | Homogeneity of Covariance | Yes; to test using Levene's test | not a priority; univariate analysis | Yes; to test using Levene's test | | Homoscedasticity | values for each cell of the design (i.e., each combination of groups of the two independent variables). Can be tested using Shapiro-Wilk test | assessed by the regression slope, is the same in each group of the independent variable. Test by plotting a scatterplot included in the test of normality of residuals using Shapiro | | | Homogeneity of Regression Slopes | no interaction between the covariate and the independent variable test by plotting a grouped scatterplot and adding less lines to make the interpretation easier | within each group of the independent variable. Test by plotting a scatterplot | | | Linear correlation between Cv and each group (combination) of IV | | | | | Randomness of error | mere should be multivanate normalmy, best guess using Shapiro-vvik test lof normality (residuals) | | |